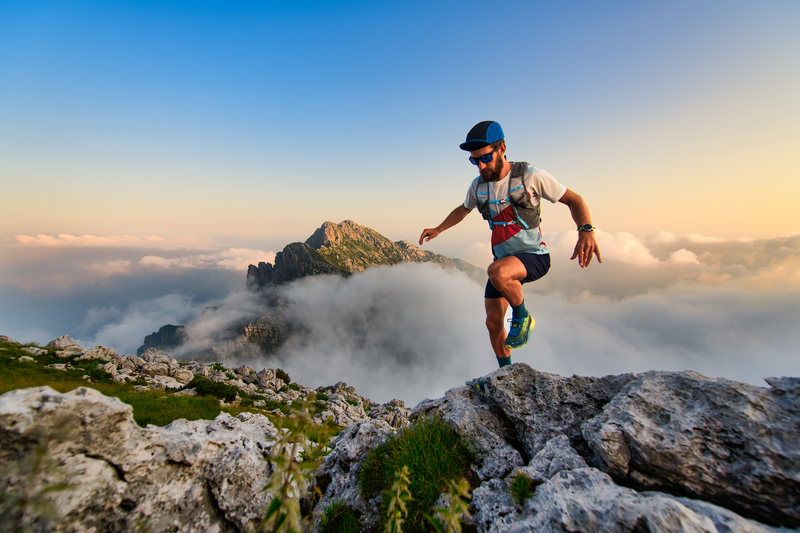
94% of researchers rate our articles as excellent or good
Learn more about the work of our research integrity team to safeguard the quality of each article we publish.
Find out more
ORIGINAL RESEARCH article
Front. Virol. , 10 June 2022
Sec. Translational Virology
Volume 2 - 2022 | https://doi.org/10.3389/fviro.2022.894531
This article is part of the Research Topic Role of genetic and structural insights of neutralizing antibodies to guide viral vaccine design View all 5 articles
High transmissibility is a hallmark of the Omicron variant of SARS-CoV-2. Understanding the molecular determinants of Omicron’s transmissibility will impact development of intervention strategies. Here we map the electrostatic potential surface of the Spike protein to show that major SARS-CoV-2 variants have accumulated positive charges in solvent-exposed regions of the Spike protein, especially its ACE2-binding interface. Significantly, the Omicron Spike-ACE2 complex has complementary electrostatic surfaces. In contrast, interfaces between Omicron and neutralizing antibodies tend to have similar positively charged surfaces. Structural modeling demonstrates that the electrostatic property of Omicron’s Spike receptor binding domain (S RBD) plays a role in enhancing ACE2 recognition and destabilizing Spike-antibody complexes. Specifically, the Omicron S RBD has favorable electrostatic interaction energy with ACE2 that is 3-5 times greater than the Delta variant over a range of 20 Å, implying efficient recognition of host receptors. Computed binding affinities of six representative S RBD-antibody complexes show that Omicron can escape most antibodies targeting the ACE2-binding region of S RBD. Interestingly, a straightforward assessment of the electrostatic surfaces of 18 neutralizing antibodies correctly predicted the Omicron escape status of 80% of cases. Collectively, our structural analysis implies that Omicron S RBD interaction interfaces have been optimized to simultaneously promote access to human ACE2 receptors and evade antibodies. These findings suggest that electrostatic interactions are a major contributing factor for increased Omicron transmissibility relative to other variants.
The emergence of highly transmissible Omicron variant of SARS-CoV-2 challenges our understanding of the underlying causes of its spread and clinical manifestations. Omicron is characterized by a jump in the number mutations in the crucial Spike protein with thirty amino acid changes, three small deletions, and one small insertion. In contrast, the Delta variant only has eight amino acid changes, a single amino acid deletion, and a small deletion in Spike. Indeed, tracking of SARS-CoV-2 mutations (nextstrain.org database (1)) indicates that the number of mutations in variants has been increasing over time. Since viral transmissibility also has been increasing throughout the COVID-19 pandemic, it is intriguing to speculate whether there is a mutational pattern that can provide some insight into the trajectory of emerging variants. Deciphering the links between mutations, variant evolution and transmissibility could help anticipate new variants and assist design of effective therapeutics.
Mutations in the Spike protein of the Omicron variant (BA.1 lineage) are in three major regions: two in the Spike receptor binding domain (S RBD, residues 333–527) and one in the “fusion” domain (2). Crucially, a cluster of ten mutations is in the Spike receptor binding motif (S RBM, residues 438–506) or its ACE2-binding interface. There is also a smaller cluster of four mutations around the S1/S2 (or furin) cleavage site. The Spike N-terminal domain (S NTD, residues 13-303) contains three deletions and an insertion in addition to four point mutations. The two other Omicron lineages (BA.2 and BA.3) share twenty point mutations in Spike with the BA.1 lineage, eight of which are in S RBM. Unlike the previous SARS-CoV-2 variants, mutations in Omicron lineages tend to occur in clusters, a scenario suggested in a structural modeling analysis (3).
The occurrence of mutation clusters challenges our understanding about their influence on the biological properties of Omicron such as antibody resistance (4–6), mode of host cell entry (7), viral evolution (2), virulence (8), and transmission. Several large-scale experimental studies have demonstrated that Omicron S RBD can evade most neutralizing antibodies, especially those targeting S RBM including some therapeutic monoclonal antibodies (4, 5). Although some Omicron S RBD mutations found in other variants are known to evade antibodies (K417N, N440K, E484K/Q), the roles of Omicron-specific mutations are not clear. According to a recent study (2), some novel Omicron mutations (G339D, S371L, S373P, S375F, Y505H) have a negligible effect on the strength of antibody escape; such mutations are also considered rare in sarbecoviruses (SARS-CoV-1/2) and not positively selected. Antibody escape potential of multiple, clustered mutations cannot be easily assessed based on single mutation data because of likely significant conformational changes at antibody binding sites. These considerations suggest a need for new approaches to analysis of Omicron mutations, both individually and collectively.
A feature of Omicron and other variants is that most of their defining mutations are found on the surface of the Spike protein. Surface mutations affect Spike’s interactions with antibodies, ACE2 receptors and proteases to determine infectivity. To decipher the global effects of Omicron mutations, we map the electrostatic potential surface of Spike. We show that the Omicron Spike trimer surface has transitioned to a strongly positive electrostatic surface relative to the reference (Wuhan-Hu-1) Spike, especially in S RBM, which interfaces with the negatively charged ACE2 receptor. In addition, we use structural modeling to demonstrate that Omicron S RBD is attracted to ACE2 receptors by long-range electrostatic forces and can destabilize five out of six representative neutralizing antibodies tested. Thus, our computational analyses suggest that Omicron mutations collectively enhance ACE2 recognition and antibody escape.
Use of electrostatic potential surfaces is a common approach for mapping complementary interaction interfaces in biomolecular complexes (9, 10). Relative to the reference Spike, the electrostatic surface of the Omicron Spike protein shows a marked transition to positive surface charges, especially on the top face or S RBM and near the furin (S1/S2) cleavage site (Figure 1A); the S1/S2 region too has become less negatively charged. The additional positive surface charges in Omicron S RBM are acquired through N440K, T478K, Q493R, Q498R and Y505H mutations; the solvent-accessible loop containing the furin cleavage site added two positive charges from N679K and P681H mutations. The increase in surface charges of Omicron S RBD has been speculated to influence ACE2 recognition and antibody binding (11).
Figure 1 Electrostatic potential surfaces of Spike, Spike trimer, and ACE2 proteins. (A) Electrostatic surfaces of reference and modeled Omicron Spike proteins. (B) Electrostatic surfaces of reference and modeled Omicron Spike trimers. (C) Electrostatic surface of extracellular domain of human ACE2 with Spike RBD (PDB ID: 6m17). (D) Electrostatic binding energy as a function of separation distance for the reference, Delta and Omicron Spike RBD-ACE2 complexes. Separation distance is along the axis perpendicular to the interaction interface. The color scale of the electrostatic potential surface is in units of kT/e at T = 37°C, or 26.7mV.
Since the Spike protein is assembled into Spike trimers on the viral membrane of SARS-CoV-2, the electrostatic surface of Spike trimer is a direct indication of its functional implications. The reference Spike trimer complex has a slightly positive head or top region, consisting of S NTD and RBD, while the surface of the S1/S2 region below it is mostly negatively charged (Figure 1B, side view). In sharp contrast, the Omicron Spike trimer has a strongly positive head region as well as the S1/S2 region. The Omicron Spike trimer was obtained using a predicted Spike structure (Materials and Methods) and then assembled using a solved reference Spike trimer template (PDB ID: 6vyb). A modeled Spike trimer was used for this purpose because current solved S trimers contain many (120-450) missing residues, especially in the solvent-exposed loop regions (PBD ID: 7t9j, 7tgw, 7wpd) (12–14). The Spike structure predicted by AlphaFold2 is highly accurate: structural deviations of <2 Å for S RBD (Figure S1A) and ~3 Å for full Spike structure (RBD in the down conformation), or about the same as deviations between solved structures. The change in the electrostatic surface of Omicron Spike trimer is especially dramatic at the ACE2-binding interface or S RBM (Figure 1B, top view). Since the extracellular domain of human ACE2 (residues 1-614) has an entirely negative electrostatic potential surface (Figure 1C), Omicron S RBD is strongly attracted to target ACE2 receptors by long-range electrostatic forces. Protein electrostatic surfaces, as described above, are shielded by ions in physiological environments. Effective surface potentials of Spike trimer and ACE2 at different ionic concentrations (50, 100 and 150 mM) indicate attenuation of positive surfaces (Figures S1B, C), which must be accounted for in quantitative assessment of Spike’s interactions with ACE2 and antibodies.
We quantified the electrostatic interactions between S RBD and the extracellular domain of human ACE2 by computing their electrostatic binding energy as a function of separation distance for reference, Delta and Omicron Spike proteins (Figure 1D). To simulate physiological conditions, the energies were computed using APBS v1.5 at 150 mM monovalent ions (15). The computed electrostatic binding energies show that Omicron S RBD has a considerably greater affinity for ACE2 over all distances (8-20 Å) compared with Delta and reference S RBDs (Figure 1D). Quantitatively, over the separation distances compared, Omicron’s electrostatic energies are 3-9 times greater than those for the reference S RBD and 3-5 times greater than Delta S RBD. Even at a distance of 20 Å Omicron S RBD is still influenced by the attractive electrostatic force emanating from the negatively charged extracellular ACE2 domain. The attractive force is expected to be even stronger if the Omicron Spike trimer, instead of S RBD, is considered because of the combined positive charges on the Spike trimer’s surface. These findings suggest that Omicron Spike trimers are guided by long range electrostatic forces to the vicinity of ACE2 leading to efficient recognition of the receptor.
The advantage conferred by Omicron’s electrostatic property to infect host cells may indicate an adaptive feature of variant evolution. To reveal this plausible driver of viral dynamics, we analyzed S RBM mutations, charges, and electrostatic surfaces across SARS-CoV-2 phylogeny. To uncover biological relationships, we superposed information about the electrostatic surfaces of S RBD on the phylogeny of SARS-CoV-2 variants (Nextstrain) as a function of viral emergence (earliest sampling date, cov-lineages.org). The Nextstrain SARS-CoV-2 phylogenetic tree represents mutation lineages and assigns each clade based on its combination of signature mutations.
The evolution of the electrostatic surface shows that there is a gradual accumulation of positive surface charges in S RBD over time and that related clades have similar electrostatic surfaces (Figure 2). To quantify the changes, we divided the surface charge transitions into two time periods: from beginning to Fall of 2020 and late 2020 to late 2021. In the first period, Alpha, Beta, Epsilon and Lambda variants acquired moderately more positive surface charges in S RBD compared with the reference Spike. More significant accumulation of positive surface charges occurred in the second period with the emergence of Gamma, Delta, Eta, Iota, Kappa and Mu variants. The large change in surface charges in the Omicron variant appears to mark another surface charge transition from those in the second time period; Omicron sub-variants BA.2 and BA.3 have essentially the same S RBD mutations associated with charge changes.
Figure 2 Electrostatic surfaces of Spike RBD variants organized by Nextstrain SARS-CoV-2 phylogeny. Tracking of total charge changes with accompanying mutations in S RBM suggests a gradual increase of positive charges over time. The color scale of the electrostatic potential surface is in units of kT/e at T = 37°C.
These observations are supported by the accumulated charges in S RBM, an ACE-binding interface and target of many neutralizing antibodies. The early variants (Alpha, Beta, Epsilon and Lambda) gained an average of one elementary electric charge (+1e). In contrast, variants in the second time period (Gamma, Delta, Eta, Iota, Kappa and Mu) all gained +2e. Intriguingly, the gain in positive charges up to late 2021 is largely accounted for by only three mutations (L452R, T478K and E484K), suggesting close links between these variants. The Omicron variant gained +5e from N440K, T478K and three novel mutations Q493R, Q498R and Y505H. Thus, there is roughly a doubling of positive charges in S RBM in each of the time periods described (0➔+1e➔+2e➔+5e).
Even though the SARS-CoV-2 clades are defined without S RBM charge considerations, their phylogeny reveals a close relationship with S RBD electrostatic surfaces. For example, Eta (clade 21D), Iota (21F) and Mu (21H) variants have similar S RBD electrostatic surfaces. Also evident is the similarity of the electrostatic surfaces of related Delta (21J) and Kappa (21B) variants. Moreover, distantly related clades (Delta/Kappa, Eta/Iota/Mu and Omicron) all acquired positive charges. These observations suggest that the increase of total positive charges in S RBM is a general adaptive feature of variant evolution to enhance ACE2 association.
Although hundreds of antibodies against SARS-CoV-2 have been sequenced, most known neutralizing antibodies target S RBD (16–18). To assess the response of neutralizing antibodies to Omicron SARS-CoV-2, we selected six representative antibodies from high-resolution (<3 Å) S RBD-antibody complexes that bind to different S RBD sites (Figure 3A, Materials and Methods). Specifically, antibodies P2B-2F6 and COVOX-88,-150,-158,-316 bind S RBM sites, whereas S2X259 targets outside of S RBM. Electrostatic potential surfaces of these six antibodies indicate that their Spike-interacting interfaces are predominantly positively charged, especially for P2B-2F6 and COVOX-316 (Figure 3B). In contrast, antibody S2X259 has a negatively charged interaction interface. As shown (Figure 3C), the antibody-binding interfaces of Spike are all positively charged. Qualitatively, this indicates that antibodies P2B-2F6 and COVOX-88,-150,-158,-316 are likely to have unfavorable electrostatic binding energies with Omicron S RBD, whereas S2X259 is expected to have a favorable binding energy. Overall, the electrostatic property of Omicron S RBD is likely to increase resistance against most classes of antibodies, as found in recent experimental studies (4, 5). Below, we present a quantitative assessment of antibody response to S RBD mutations from different variants using an antibody escape score based on binding affinity changes relative to the reference S RBD.
Figure 3 Spike-antibody complexes and their electrostatic potential surfaces. (A) Solved structures of neutralizing antibodies targeting different sites on S RBD used for computational modeling. (B) Electrostatic surfaces of antibodies complexed with reference Spike. (C) Electrostatic surfaces of Omicron Spike modeled with different antibodies using templates in (A). Spike-interacting interfaces on antibodies are predominantly positively charged which contribute to antibody resistance by Omicron. The color scale of the electrostatic potential surface is in units of kT/e at T = 37°C.
Current experimental approaches employ infectivity (5, 19) and yeast-display/FACS (4, 20) assays as measures of destabilization of antibody binding due to Spike mutations. Here, we use computational modeling to directly quantify the effects of Spike mutations on antibody binding. This approach is feasible because many Spike-antibody complexes have been solved in the last two years (17, 18, 21), enabling structural modeling of mutational effects (22, 23). Briefly, we employed six representative neutralizing antibodies (Figure 3, Materials and Methods) and considered mutations in direct contact with or in close proximity of antibodies. We define the strength of antibody escape as the destabilization of antibody binding. Specifically, our antibody escape score is defined as the normalized affinity change, (ΔGmut - ΔGref)/|ΔGref|, where ΔGref and ΔGmut are affinities of reference and mutant Spike, respectively. Thus, positive scores indicate reduced antibody binding, whereas negative scores imply increased antibody binding. Here, we use the score to evaluate antibody escape induced by single mutations of the reference Spike, a method we described previously (3).
Computed antibody escape scores show that the most prominent escape peaks across all antibodies are from E484K/Q mutations, which are confirmed escape mutants found in Beta, Gamma, Zeta and Kappa variants (Figure 4) (20). Mutation N501Y, occurring in Alpha, Beta, Gamma and Mu variants, only exhibits a moderate escape from COVOX-158 and COVOX-88. Mutations K417N/T from Beta and Gamma variants exhibit varying degrees of resistance to COVOX-150, COVOX-158 and COVOX-88. By contrast, S477N, a frequent mutation in GISAID database, has a weak escape from most antibodies except possibly COVOX-316. A deep mutagenesis study of single S RBD mutations based on ten antibodies determined that S477N is not an escape mutant (20). The Delta variant has a L452Q/T487K mutation combination in S RBD. Based on our predictions, mutation L452Q can escape all tested antibodies except S2X259, and mutation T478K escapes COVOX-316 and COVOX-88. The predicted extensive antibody escape profile for the Delta variant correlates well with experimental studies (24) and its dominance.
Figure 4 Antibody escape scores of point mutations from non-Omicron variants. (A) Escape scores of point mutations for six neutralizing antibodies targeting S RBD. Occurrence of mutations in different variants is indicated. (B) Correlation between predicted and experimentally measured antibody escape scores. The two approaches used different sets of antibodies targeting S RBD. The experimental score is the sum of contributions from ten antibodies.
A further test of predicted antibody escape scores is to compare with those measured experimentally using deep mutagenesis scanning (20). The experimental method used ten antibodies targeting S RBD, but the specific antibodies do not overlap with those used in structural modeling (scores defined in Materials and Methods). Still, this comparison is meaningful because many S RBD-targeting antibodies bind the crucial S RBM region, as demonstrated by many solved complexes (Figure 3) (5, 17). By using antibody escape scores for groups of antibodies, we find that the predicted scores are moderately correlated (R2 = 0.51) with those from deep mutagenesis for single mutations (Figure 4B). As shown, E484K/Q/R and L452R have high antibody escape scores, whereas S477N has a low score. Collectively, our predicted antibody responses to mutations in variants indicate broad qualitative agreement with experimental studies.
Although recent experimental works have examined antibody escape by the Omicron variant (4, 5), computational modeling allows identification of specific energetic contributors of antibody resistance. Our antibody escape measure predicted that the Omicron S RBD with 15 mutations can escape all antibodies except S2X259 whose target site is outside of S RBM (Figure 5A). The antibody escape scores for 5 out of the 6 neutralizing antibodies examined are in the upper range (scores of 2 to 4) of those predicted for single mutations from previous variants (Figure 4A). In fact, computed affinities show that Omicron Spike cannot form complexes (i.e., ΔG>0) with these tested antibodies. In contrast, the Omicron mutations have a negligible effect on S2X259’s binding to S RBD. These results are in overall agreement with a recent experimental antibody escape study showing that most neutralizing antibodies targeting S RBM are ineffective against Omicron and that effective antibodies target sites outside of S RBM (5).
Figure 5 Antibody escape scores of Omicron S RBD mutations. (A) Antibody escape scores of six antibody-Omicron S RBD complexes. (B) Electrostatic component of antibody escape scores of six antibody-Omicron S RBD complexes. (C) Antibody escape score matrix of six antibodies against 15 individual Omicron mutations. (D) Average antibody escape scores of six antibodies induced by individual Omicron mutations (derived from C). (E) Antibody escape scores of individual Omicron mutations averaged over six antibodies. (F) Comparison of model and experimental relative antibody escape scores of Omicron S RBD mutations grouped by selection regime. To enable a comparison of predicted and experimental scores, the group escape scores were normalized by the score of the positive group to yield relative escape scores. Two separate experimental datasets were used for comparison: one generated using 10 antibodies and another using 247 antibodies.
Omicron Spike-antibody affinities were computed based on refined complexes obtained using Monte Carlo Minimization (MCM, see Materials and Methods). Complexes were refined in a stepwise manner from initial template Spike-antibody structures toward lower energy configurations. Structural deviations as a function of MCM steps for Omicron Spike RBD-P2B-2F6/S2X259/COVOX-150 complexes show that the final root-mean-square deviation (RMSD) values vary between 0.8 to 1.6 Å (Figures S2A, B). We observed the largest RMSD deviation for the S RBD-S2X259 complex because the flexible S RBM loop is not the site of antibody binding, allowing the loop to fluctuate. Thus, its S RBD has larger RMSD values than S2X259 during longer MCM simulations (inset Figure S2A). Overall, structural changes are small occurring mostly in the loop regions reflecting their conformational flexibility and the effects of Omicron mutations. Although these results indicate conformational sampling simulations maintain the stability of individual proteins, longer simulations are needed to achieve more consistent equilibration of complexes studied.
To unravel the energetic factors causing antibody resistance, we examined the major energy components contributing to binding affinity, including short-range van der Waals, electrostatic, and entropy terms (25). We then computed the antibody escape scores for each energy component (i.e., substituting ΔGref and ΔGmut with component energies). For the electrostatic energy component, which includes the effects of protein charge and ionic interactions among Spike, antibody and counter-ions, we again observed the same pattern of antibody affinity destabilization by Omicron (Figure 5B): P2B-2F6 and COVOX-316,-150,-158,-88 have unfavorable electrostatic binding energies, but the effect on S2X259 is nearly unchanged. Electrostatic destabilization is especially strong (score of ~15) for COVOX-316, which has a positive electrostatic surface like S RBM (Figures 3B, C). The destabilizing effects of electrostatics are also significant for P2B-2F6 and COVOX-158 (scores of ~5). Using the same analysis, the effects of other energy components on overall affinity are considerably less. In particular, the changes in escape scores due to van der Waals energy are less than 10%, and there is no specific direction of influence. The entropy factor also contributes to antibody resistance but the effects are weak (escape score of 0.4 for COVOX-88 and much less for other antibodies). This suggests that Omicron mutations introduce some entropic costs to antibody binding. Overall, our quantitative affinity assessment implies that electrostatic interactions are the main contributor to antibody escape by Omicron. This confirms our assessment based on electrostatic surfaces of Spike-antibody complexes (Figure 3).
To further analyze the contribution of each Omicron mutation to antibody escape, we computationally predicted the escape scores for all combinations of six antibodies and 15 single S RBD mutations (Figure 5C). As shown, antibody response varies significantly across the 15 mutations and six antibodies. However, a couple of response patterns emerged. Mutation-averaged escape scores show that all antibodies except S2X259 are resisted by individual Omicron mutations (Figure 5D), consistent with scores modeled with full Omicron S RBD (Figure 5A). These scores are lower than that for combined Omicron mutations (Figure 5A), indicating the additive effects of individual mutations. The antibody-averaged scores of individual mutations show that all Omicron mutations can escape antibodies to some extent (Figure 5E). This conclusion is in agreement with a recent study of 247 antibodies showing that almost all individual Omicron mutations were shown to escape some antibodies (4). The predicted stronger escapers (scores > 0.5) are G446S, T478K, E484A, Q493R, G496S, Q498R, N501Y and Y505H and the weaker escapers (scores < 0.5) are G339D, S371L, S373P, S375F, K417N and N440K. Our modeling indicates that these mutations induce a range of positive antibody escape scores, implying they contribute to the overall fitness of the Omicron variant. Additionally, a recently solved Omicron Spike-ACE2 complex shows that Q493R and Q498R also contribute to affinity by forming two new salt bridges with ACE2 (14), a finding that reinforces the role of electrostatics in Omicron’s interactions.
Selection analysis of Omicron mutations has been performed to shed light on adaptive mutations and Spike function (2). Each mutation is classified as either negatively, neutral or positively selected based on analysis of SARS-CoV-2 genomes. Positive selection was analyzed using MEME (26) and negative selection sites using FEL (27); these methods evolved from earlier neutrality tests (28, 29). To analyze the relationship between selection regime and antibody escape, we group Omicron S RBD mutations by selection regime: negative (G339D, S371L, S373P, S375F, Q498R), neutral (Q493R, G496S, Y505H) and positive (K417N, N440K, G446S, S477N, T478K, E484A, N501Y). To compare with predicted escape scores, we used two experimental datasets: one generated using 10 antibodies (20) and a recent dataset using 247 antibodies (4), with only two overlapping antibodies (COV2-2479 and COV2-2499). For the larger dataset, the escape scores are characterized qualitatively, in decreasing order, as (Extended Data Figure 3 in (4)): mutation escape, site escape and no escape, which we heuristically assigned scores of 1, 0.5 and 0, respectively. To enable comparison of different escape measures, we used relative escape scores which are normalized by the score for the positively selected group.
Both experimental datasets indicate that the positive group has a considerably higher score (>5 times) than the negative group (Figure 5F). For the neutral group with only 3 mutations, the 247-antibody dataset shows a stronger average escape score than the 10-antibody dataset, which shows no change from the negative group. The predicted relative antibody escape scores also suggest the negative group has a lower score than the positive group, but less drastic than implied by experimental datasets. This suggests that positively selected mutations are adaptive, antibody resistant mutations. The neutral group has the highest predicted score not seen in experimental datasets; data for this group may be hampered by lack of available mutations. Clearly, a larger set of mutations in different selection regimes, together with quantitative scoring of the 247-antibody escape data, is needed to comprehensively test our modeling method. Moreover, the tests performed here pertain to individual mutations, which may not capture the effects of synergistic Omicron mutations (2, 4).
Since many antibodies escaped by Omicron have been determined experimentally (Supplementary Table 1 in Ref (4)), they can be used to demonstrate the role of electrostatics in antibody evasion. Here we use an expanded set of 18 solved complexes of antibodies bound to the reference strain S RBD (downloaded from PDB in November 2021) whose Omicron escape status has been determined. We grouped the S RBD-interacting surfaces of antibodies as positive/negative electrostatic surface and bind/do not bind Omicron S RBD (Figure 6). There are 12 positive and 6 negative electrostatic surfaces. As expected, all antibodies with positive surfaces are escaped by Omicron. For antibodies with negative surfaces, experimental data indicate that only 3 (S2X259, S2E12, COVOX-253H55L) out of 6 antibodies can bind Omicron S RBD, suggesting that additional factors beyond simple charge distribution, such as variation in Omicron Spike conformation, may prevent binding of Omicron Spike to antibodies S304, COVOX-75 and C110. Thus, 15 out of 18 antibodies are correctly predicted to either bind or escape Omicron S RBD based on a simple visual assessment of their electrostatic surfaces alone. In addition, five of the antibodies (P2B-2F6, S2X259, COVOX-316, -150,-88) whose escape status was predicted based on affinity calculations (above section) are in agreement with experimental data; escape data is not available for COVOX-158. These findings suggest that ~80% of antibodies that either bind or do not bind Omicron could be predicted just by inspecting their electrostatic surfaces. For more precise predictions, use of structural modeling as described above may be needed.
Figure 6 Electrostatic surfaces of 18 neutralizing antibodies with experimental Omicron escape data. Solved reference Spike-antibody complexes were used to display antibody electrostatic surfaces at Spike (cyan) interacting sites, which are sorted into positive and negative surfaces that bind or do not bind Omicron S RBD based on experimental data. In solved complexes, all antibodies except S2X259 bind S RBM. Each complex is labeled by its antibody name and PBD ID(chains). The color scale of the electrostatic potential surface is in units of kT/e at T = 37°C.
Long-range electrostatic forces play an important role in biomolecular interactions. Many biomolecular complexes form partly due to the presence of complementary electrostatic potential surfaces (9, 10). Here we show that during the course of the SARS-CoV-2 pandemic variant forms of S RBD have been evolving toward a positively charged surface that complements the ACE2 receptor, ensuring favorable association with the cell surface receptors leading to host cell infection (Figure 7). At the same time, assessment of electrostatic surfaces of neutralizing antibodies isolated early in the pandemic showed that most of the antibodies have positively charged S RBD-recognition surfaces, implying that recent SARS-CoV-2 variants could escape antibody surveillance via unfavorable electrostatic interactions. Using a computational structural modeling method, we showed this to be the case for the Omicron variant using antibodies targeting different S RBD sites. Thus, a plausible scenario is that SARS-CoV-2 variants have been optimizing their S RBM to simultaneously enhance ACE2 recognition and evade antibodies (Figure 7). The advantage conferred by the electrostatic property of Omicron S RBD may partially account for the variant’s rapid global transmission.
Figure 7 Omicron Spike’s electrostatic potential surface evades most antibodies and promotes ACE2 association for host cell entry.
These conclusions are consistent with experimental and other theoretical studies. First, experimentally measured antibody escape by Omicron is strongly correlated with the electrostatic surface of Spike binding site (15 out of 18 cases, Figure 6). Significantly, the escape status for 80% of neutralizing antibodies can be predicted by inspecting their electrostatic surfaces at Spike binding sites, which simplifies enormously the task of determining escape potential of many antibodies. Second, searches for optimal antibody binding to a specific site of reference S RBD using atomistic simulations suggest there is a roughly linear relationship between net antibody charge and binding affinity: positively charged antibodies are poorer binders than negatively charged ones (30). Third, molecular dynamics analysis of major therapeutic antibodies indicates that electrostatic energy plays a role in weakening Omicron S RBD-antibody affinity (31).
The evasion of antibodies by the Omicron variant is not complete (4, 5). In particular, antibody S2X259 targeting outside of S RBM (site II) is not destabilized by the Omicron variant, a finding confirmed in a recent screening of antibodies using antibody escape assays (5). This is due to electrostatic complementarity in the Omicron S RBD-S2X259 complex (Figures 3B, C). The effectiveness of S2X259 antibody suggests it might be possible to engineer antibodies by exploiting electrostatic complementarity to S RBD target sites, an approach similar to design of ligands capable of binding proteins with high specificity (32). Indeed, this approach has been implemented in design of antibodies against flaviviruses (33) and SARS-CoV-2 variants (30).
The basic character of Omicron S RBD may also impact its interactions with other components of host defense. Specifically, the major components of the mucus system (mucin proteins MUC5AC and MUC5B) shield the respiratory tracts from bacterial and viral infection (34). Mucin proteins are heavily glycosylated, often with negatively charged terminal sialic acids. Thus the negatively charged polyelectrolytes (35) in the mucus matrix can in theory provide a more effective shield against Omicron than other variants. This suggests a testable molecular hypothesis for observed attenuated Omicron replication and pathogenicity in respiratory tracts (8).
In addition to ACE2, SARS-CoV-2 S RBD also interacts with cellular heparan sulfate, a highly negatively charged linear polysaccharide on cell surfaces, to strengthen cell attachment and increase infectivity (36). Docking simulations suggest that heparan sulfate and ACE2 bind to adjacent surfaces on S RBD, implying the former is a cofactor for host cell entry (36). It is thus likely the increased positive surface charges of Omicron S RBD could enhance recognition of heparan sulfate and promote viral transmissibility.
The rise of the Omicron family of variants indicates that mutational changes occur in clusters. Analysis of individual mutations may not provide a complete understanding of the biological properties of the new variants (2). To meet the new challenge, approaches that can probe the collective physical and functional properties are needed. Here we examined how mutational clusters in S RBD of the BA.1 lineage influence ACE2 association and antibody resistance. Thus, future studies on how Omicron mutations collectively affect antibody escape, replication and virulence are needed to provide a broader basis for developing effective intervention strategies.
The structures were predicted using AlphaFold2, a deep learning approach to protein structure prediction (37). It enables prediction of Spike structures with mutations, deletions and insertions. The predicted Spike variant structures differed from the solved reference Spike structure (PDB ID: 6vyb) by less than 2 Å.
Six reference Spike-antibody complexes were selected from solved structures based on their structure resolution (<3 Å) and interactions with the 15 Omicron S RBD mutations. They are (PDB ID and Fab name): 7bwj (P2B-2F6), 7m7w (S2X259), 7beh (COVOX-316), 7bei (COVOX-150), 7bek (COVOX-158), 7bel (COVOX-88). The selected antibodies bind multiple sites on S RBD (Figure 3A). Only the globular Fab domains directly interacting with Spike were retained, i.e., one structured domain each for light and heavy chains. Figure 6 contains information about 12 additional reference Spike-antibody complexes used for electrostatic surface analysis.
Electrostatic potential surfaces were computed using APBS v1.5 (at zero ionic concentration) (15) and visualized using pymol. We used default box dimensions and mesh parameters.
Distance dependence of electrostatic binding energy between two proteins was computed using the formula: ΔEbind(R) = EAB(R)–EA–EB, where R is the separation distance between the proteins and EA, EB are electrostatic energies of isolated individual proteins; R=0 is the contact distance in a solved binary protein complex. ΔEAB(R) was computed at discrete separation distances 2, 4,…,20 Å, whose complexes were generated using pymol by translating one of the proteins along an axis roughly perpendicular to the interaction interface. We then used the APBS software to compute the energy components EA, EB and EAB(R) at various distances.
Binding affinities of Spike-antibody complexes were calculated based on refined complexes. Structure refinements were performed using Monte Carlo Minimization (MCM) as implemented in Tinker v7.1 molecular modeling package (38). MCM of each complex was run for six days on four processors on HPC Linux Cluster at NYU Abu Dhabi, with the following parameters: rms gradient < 0.01 kcal/(mol.Å) and move step size of 0.5 Å. We used the all-atom AMBER99/GBSA force field. For affinity calculations, the best energy complexes were used and then subjected to a stringent local minimization (rms gradient of <0.0005 kcal/mol/Å).
Affinities of ternary Spike-antibody complexes were computed using molecular modeling methods, as described previously (22, 25). Briefly, the computed binding affinity is a sum of contributions from solvation, van der Waals, electrostatic, and entropic interactions. The electrostatic binding energies were computed using APBS v1.5. All affinities were computed at 37˚C and 150 mM of monovalent ions.
We used antibody escape scores from experimental yeast-display system (20) to compare with scores from structural modeling. Experimental scores are expressed as escape fractions for S RBM mutations against 10 antibodies (COV2-2050, COV2-2082, COV2-2094, COV2-2096, COV2-2165, COV2-2479, COV2-2499, COV2-2677, COV2-2832, rCR3022) (20). For a given mutation, we computed the total experimental escape score as the sum of all escape fractions from all 10 antibodies.
The original contributions presented in the study are included in the article/Supplementary Material. Further inquiries can be directed to the corresponding author.
HG, KG, and FP contributed to plans for the paper. HG performed structural modeling of electrostatics and antibody escape and wrote the initial draft of the paper. JZ predicted structures of Spike variants and performed data analysis. All authors contributed to editing the paper. All authors contributed to the article and approved the submitted version.
This work was supported by general research funds from NYU Abu Dhabi and by a grant from the NYU Abu Dhabi Research Institute to the NYUAD Center for Genomics and Systems Biology (ADHPG-CGSB).
The authors declare that the research was conducted in the absence of any commercial or financial relationships that could be construed as a potential conflict of interest.
All claims expressed in this article are solely those of the authors and do not necessarily represent those of their affiliated organizations, or those of the publisher, the editors and the reviewers. Any product that may be evaluated in this article, or claim that may be made by its manufacturer, is not guaranteed or endorsed by the publisher.
This research was carried out on the High Performance Computing resources at New York University Abu Dhabi and New York. We thank Benoit Marchand for his encouragement and support in allocating computational resources for this project. HG is grateful to Rana Zeine for many useful discussions on immunology.
The Supplementary Material for this article can be found online at: https://www.frontiersin.org/articles/10.3389/fviro.2022.894531/full#supplementary-material
1. Hadfield J, Megill C, Bell SM, Huddleston J, Potter B, Callender C, et al. Nextstrain: Real-Time Tracking of Pathogen Evolution. Bioinformatics (2018) 34(23):4121–3. doi: 10.1093/bioinformatics/bty407
2. Martin DP, Lytras S, Lucaci AG, Maier W, Gruning B, Shank SD, et al. Selection Analysis Identifies Clusters of Unusual Mutational Changes in Omicron Lineage BA.1 That Likely Impact Spike Function. Mol Biol Evol (2022) 39(4):msac061. doi: 10.1093/molbev/msac061
3. Gan HH, Twaddle A, Marchand B, Gunsalus KC. Structural Modeling of the SARS-CoV-2 Spike/Human ACE2 Complex Interface can Identify High-Affinity Variants Associated With Increased Transmissibility. J Mol Biol (2021) 433(15):167051. doi: 10.1016/j.jmb.2021.167051
4. Cao Y, Wang J, Jian F, Xiao T, Song W, Yisimayi A, et al. Omicron Escapes the Majority of Existing SARS-CoV-2 Neutralizing Antibodies. Nature (2022) 602(7898):657–63. doi: 10.1038/s41586-021-04385-3
5. Cameroni E, Bowen JE, Rosen LE, Saliba C, Zepeda SK, Culap K, et al. Broadly Neutralizing Antibodies Overcome SARS-CoV-2 Omicron Antigenic Shift. Nature (2022) 602(7898):664–70. doi: 10.1038/s41586-021-04386-2
6. Liu L, Iketani S, Guo Y, Chan JF, Wang M, Luo Y, et al. Striking Antibody Evasion Manifested by the Omicron Variant of SARS-CoV-2. Nature (2022) 602(7898):676–81. doi: 10.1038/s41586-021-04388-0
7. Peacock TP, Brown JC, Zhou J, Thakur N, Newman J, Kugathasan R, et al. The SARS-CoV-2 Variant, Omicron, Shows Rapid Replication in Human Primary Nasal Epithelial Cultures and Efficiently Uses the Endosomal Route of Entry. bioRxiv (2022). doi: 10.1101/2021.12.31.474653
8. Shuai H, Chan JF, Hu B, Chai Y, Yuen TT, Yin F, et al. Attenuated Replication and Pathogenicity of SARS-CoV-2 B.1.1.529 Omicron. Nature (2022) 603(7902):693–9. doi: 10.1038/s41586-022-04442-5
9. Weiner PK, Langridge R, Blaney JM, Schaefer R, Kollman PA. Electrostatic Potential Molecular Surfaces. Proc Natl Acad Sci U S A (1982) 79(12):3754–8. doi: 10.1073/pnas.79.12.3754
10. McCoy AJ, Chandana Epa V, Colman PM. Electrostatic Complementarity at Protein/Protein Interfaces. J Mol Biol (1997) 268(2):570–84. doi: 10.1006/jmbi.1997.0987
11. Pascarella S, Ciccozzi M, Bianchi M, Benvenuto D, Cauda R, Cassone A. The Electrostatic Potential of the Omicron Variant Spike is Higher Than in Delta and Delta-Plus Variants: A Hint to Higher Transmissibility? J Med Virol (2022) 94(4):1277–80. doi: 10.1002/jmv.27528
12. Ye G, Liu B, Li F. Cryo-EM Structure of a SARS-CoV-2 Omicron Spike Protein Ectodomain. Nat Commun (2022) 13(1):1214. doi: 10.1038/s41467-022-28882-9
13. Yin W, Xu Y, Xu P, Cao X, Wu C, Gu C, et al. Structures of the Omicron Spike Trimer With ACE2 and an Anti-Omicron Antibody. Science (2022) 375(6584):1048–53. doi: 10.1126/science.abn8863
14. Mannar D, Saville JW, Zhu X, Srivastava SS, Berezuk AM, Tuttle KS, et al. SARS-CoV-2 Omicron Variant: Antibody Evasion and Cryo-EM Structure of Spike Protein-ACE2 Complex. Science (2022) 375(6582):760–4. doi: 10.1126/science.abn7760
15. Baker NA. Poisson-Boltzmann Methods for Biomolecular Electrostatics. Methods Enzymol (2004) 383:94–118. doi: 10.1016/S0076-6879(04)83005-2
16. Cao Y, Su B, Guo X, Sun W, Deng Y, Bao L, et al. Potent Neutralizing Antibodies Against SARS-CoV-2 Identified by High-Throughput Single-Cell Sequencing of Convalescent Patients' B Cells. Cell (2020) 182(1):73–84.e16. doi: 10.1016/j.cell.2020.05.025
17. Dejnirattisai W, Zhou D, Ginn HM, Duyvesteyn HME, Supasa P, Case JB, et al. The Antigenic Anatomy of SARS-CoV-2 Receptor Binding Domain. Cell (2021) 184(8):2183–200.e22. doi: 10.1016/j.cell.2021.02.032
18. Corti D, Purcell LA, Snell G, Veesler D. Tackling COVID-19 With Neutralizing Monoclonal Antibodies. Cell (2021) 184(17):4593–5. doi: 10.1016/j.cell.2021.07.027
19. Weisblum Y, Schmidt F, Zhang F, DaSilva J, Poston D, Lorenzi JC, et al. Escape From Neutralizing Antibodies by SARS-CoV-2 Spike Protein Variants. eLife (2020) 9:1–31. doi: 10.7554/eLife.61312
20. Greaney AJ, Starr TN, Gilchuk P, Zost SJ, Binshtein E, Loes AN, et al. Complete Mapping of Mutations to the SARS-CoV-2 Spike Receptor-Binding Domain That Escape Antibody Recognition. Cell Host Microbe (2021) 29(1):44–57.e9. doi: 10.1016/j.chom.2020.11.007
21. Mehra R, Kepp KP. Structure and Mutations of SARS-CoV-2 Spike Protein: A Focused Overview. ACS Infect Dis (2022) 8(1):29–58. doi: 10.1021/acsinfecdis.1c00433
22. Gan HH, Gunsalus KC. The Role of Tertiary Structure in MicroRNA Target Recognition. Methods Mol Biol (2019) 1970:43–64. doi: 10.1007/978-1-4939-9207-2_4
23. Flamand MN, Gan HH, Mayya VK, Gunsalus KC, Duchaine TF. A non-Canonical Site Reveals the Cooperative Mechanisms of microRNA-Mediated Silencing. Nucleic Acids Res (2017) 45(12):7212–25. doi: 10.1093/nar/gkx340
24. McCallum M, Walls AC, Sprouse KR, Bowen JE, Rosen LE, Dang HV, et al. Molecular Basis of Immune Evasion by the Delta and Kappa SARS-CoV-2 Variants. Science (2021) 374(6575):1621–6. doi: 10.1126/science.abl8506
25. Gan HH, Gunsalus KC. Tertiary Structure-Based Analysis of microRNA-Target Interactions. RNA (2013) 19(4):539–51. doi: 10.1261/rna.035691.112
26. Murrell B, Weaver S, Smith MD, Wertheim JO, Murrell S, Aylward A, et al. Gene-Wide Identification of Episodic Selection. Mol Biol Evol (2015) 32(5):1365–71. doi: 10.1093/molbev/msv035
27. Kosakovsky Pond SL, Frost SD. Not So Different After All: A Comparison of Methods for Detecting Amino Acid Sites Under Selection. Mol Biol Evol (2005) 22(5):1208–22. doi: 10.1093/molbev/msi105
28. Tajima F. Statistical Method for Testing the Neutral Mutation Hypothesis by DNA Polymorphism. Genetics (1989) 123(3):585–95. doi: 10.1093/genetics/123.3.585
29. Fay JC, Wu CI. Hitchhiking Under Positive Darwinian Selection. Genetics (2000) 155(3):1405–13. doi: 10.1093/genetics/155.3.1405
30. Neamtu A, Mocci F, Laaksonen A, Barroso da Silva FL. Towards an Optimal Monoclonal Antibody With Higher Binding Affinity to the Receptor-Binding Domain of SARS-CoV-2 Spike Proteins From Different Variant. bioRxiv (2022). doi: 10.1101/2022.01.04.474958
31. Shah M, Woo HG. Omicron: A Heavily Mutated SARS-CoV-2 Variant Exhibits Stronger Binding to ACE2 and Potently Escapes Approved COVID-19 Therapeutic Antibodies. Front Immunol (2021) 12:830527. doi: 10.3389/fimmu.2021.830527
32. Bauer MR, Mackey MD. Electrostatic Complementarity as a Fast and Effective Tool to Optimize Binding and Selectivity of Protein-Ligand Complexes. J Med Chem (2019) 62(6):3036–50. doi: 10.1021/acs.jmedchem.8b01925
33. Poveda-Cuevas SA, Etchebest C, Barroso da Silva FL. Identification of Electrostatic Epitopes in Flavivirus by Computer Simulations: The PROCEEDpKa Method. J Chem Inf Model (2020) 60(2):944–63. doi: 10.1021/acs.jcim.9b00895
34. Chatterjee M, van Putten JPM, Strijbis K. Defensive Properties of Mucin Glycoproteins During Respiratory Infections-Relevance for SARS-CoV-2. mBio (2020) 11(6):1–12. doi: 10.1128/mBio.02374-20
35. Sircar S, Keener JP, Fogelson AL. The Effect of Divalent vs. Monovalent Ions on the Swelling of Mucin-Like Polyelectrolyte Gels: Governing Equations and Equilibrium Analysis. J Chem Phys (2013) 138(1):014901. doi: 10.1063/1.4772405
36. Clausen TM, Sandoval DR, Spliid CB, Pihl J, Perrett HR, Painter CD, et al. SARS-CoV-2 Infection Depends on Cellular Heparan Sulfate and ACE2. Cell (2020) 183(4):1043–57.e15. doi: 10.1016/j.cell.2020.09.033
37. Jumper J, Evans R, Pritzel A, Green T, Figurnov M, Ronneberger O, et al. Highly Accurate Protein Structure Prediction With AlphaFold. Nature (2021) 596(7873):583–9. doi: 10.1038/s41586-021-03819-2
Keywords: omicron variant, spike protein, electrostatic surface, antibody escape, ACE2 recognition, SARS-CoV-2
Citation: Gan HH, Zinno J, Piano F and Gunsalus KC (2022) Omicron Spike Protein Has a Positive Electrostatic Surface That Promotes ACE2 Recognition and Antibody Escape. Front.Virol. 2:894531. doi: 10.3389/fviro.2022.894531
Received: 14 March 2022; Accepted: 10 May 2022;
Published: 10 June 2022.
Edited by:
Deborah Fuller, University of Washington, United StatesReviewed by:
Firdaus Samsudin, Agency for Science, Technology and Research (A*STAR), SingaporeCopyright © 2022 Gan, Zinno, Piano and Gunsalus. This is an open-access article distributed under the terms of the Creative Commons Attribution License (CC BY). The use, distribution or reproduction in other forums is permitted, provided the original author(s) and the copyright owner(s) are credited and that the original publication in this journal is cited, in accordance with accepted academic practice. No use, distribution or reproduction is permitted which does not comply with these terms.
*Correspondence: Hin Hark Gan, aGhnM0BueXUuZWR1; Kristin C. Gunsalus, a2NnMUBueXUuZWR1
Disclaimer: All claims expressed in this article are solely those of the authors and do not necessarily represent those of their affiliated organizations, or those of the publisher, the editors and the reviewers. Any product that may be evaluated in this article or claim that may be made by its manufacturer is not guaranteed or endorsed by the publisher.
Research integrity at Frontiers
Learn more about the work of our research integrity team to safeguard the quality of each article we publish.