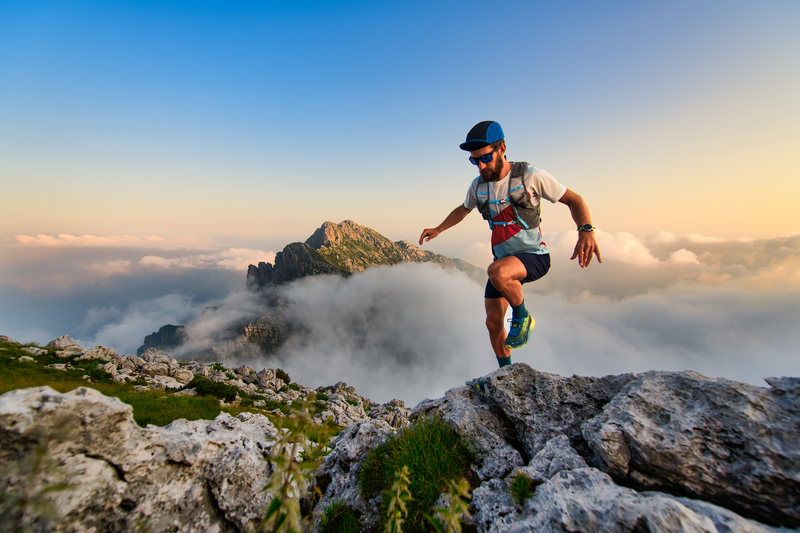
94% of researchers rate our articles as excellent or good
Learn more about the work of our research integrity team to safeguard the quality of each article we publish.
Find out more
BRIEF RESEARCH REPORT article
Front. Virol. , 01 December 2022
Sec. Bioinformatic and Predictive Virology
Volume 2 - 2022 | https://doi.org/10.3389/fviro.2022.1028335
This article is part of the Research Topic Methods in Bioinformatic and Predictive Virology View all 4 articles
SARSNTdb offers a curated, nucleotide-centric database for users of varying levels of SARS-CoV-2 knowledge. Its user-friendly interface enables querying coding regions and coordinate intervals to find out the various functional and selective constraints that act upon the corresponding nucleotides and amino acids. Users can easily obtain information about viral genes and proteins, functional domains, repeats, secondary structure formation, intragenomic interactions, and mutation prevalence. Currently, many databases are focused on the phylogeny and amino acid substitutions, mainly in the spike protein. We took a novel, more nucleotide-focused approach as RNA does more than just code for proteins and many insights can be gleaned from its study. For example, RNA-targeted drug therapies for SARS-CoV-2 are currently being developed and it is essential to understand the features only visible at that level. This database enables the user to identify regions that are more prone to forming secondary structures that drugs can target. SARSNTdb also provides illustrative mutation data from a subset of ~25,000 patient samples with a reliable read coverage across the whole genome (from different locations and time points in the pandemic. Finally, the database allows for comparing SARS-CoV-2 and SARS-CoV domains and sequences. SARSNTdb can serve the research community by being a curated repository for information that gives a jump start to analyze a mutation’s effect far beyond just determining synonymous/non-synonymous substitutions in protein sequences.
The COVID-19 pandemic caused by the SARS-CoV-2 virus has generated a massive amount of data, which needs to be organized in order to understand the virus biology. This data includes sequences, papers, medical information, and proteomics data. Several databases related to SARS-CoV-2 have studied and reported on the evolution of the virus and identifying variants (1). For example, GISAID (2), the important primary repository of assembled SARS-CoV-2 genomes at the time of writing, has over 11 million genome sequence samples submitted and list on their website several databases that use this new data to track the evolution of the virus over time. However, interpreting a reported nucleotide or amino acid substitution often requires sifting through pieces of information related to affected proteins of the virus. Such information is scattered throughout the web in many papers and databases. If a mutation is found at a certain coordinate, a thorough investigation delving into multiple papers is required to understand the functional importance of that one nucleotide and its surroundings. To remedy this, we created SARSNTdb, a compact database of highly interlinked data records that can allow the user to rapidly navigate from genome positions to functional/selective constraints on the corresponding nucleotides and amino acids.
Genome databases typically list coordinates of coding and non-coding regions and provide their annotations per such region. In contrast, SARSNTdb is nucleotide-centric, it allows querying annotations for every position in the genome from the perspective of potential selection factors affecting the corresponding nucleotide. Public attention to SARS-CoV-2 virus has generally focused on mutations occurring in its genome (and their impact on vaccine efficacy and virus spread). Most frequently, SARS-CoV-2 mutations are viewed through the prism of immune system evasion (3, 4). While this is relevant for the (most widely known) viral spike protein, the general public and scientific community are often at a loss when other substitutions are considered, especially silent ones or short insertions/deletions (indels). Given the significant interest in variants of concern (VOC), strong focus on selection would provide complementary functional context for respective VOC mutations, beyond the trivial synonymous/non-synonymous designations. Examples of such context include repeats, secondary structure formation, intragenomic interactions, nucleotide and amino acid conservation, and mutation prevalence. For example, it is known that repeats and their variations play a critical role in production of subgenomic mRNAs in coronaviruses (5), and recent VOCs, such as Omicron, display large number of both spike (6) and non-spike substitutions or indels.
To ensure the consistent cataloguing of the nucleotide and amino acid substitutions, we re-evaluated mutations across ~25,000 patient samples, for which raw metatranscriptome datasets of sufficient quality were available in NCBI’s SRA (7). We avoided taking mutations reported in GISAID, which contained already assembled genomes. Analysis of raw data for some of these genomes reveals cases of incomplete and often peculiar patterns of genome coverage (Supplementary Figure 1). Such genomes often contained segments of low genome coverage (jeopardizing mutation calling or producing massive sections with missing data) and it was not possible to tell how reliable these assemblies were. We did not aim for (and did not expect) detecting new variants but focused on cataloguing mutations in representative well-sequenced samples. By calling mutations from genomes with controlled coverage we increased the consistency of the substitution data collected, that is provided in SARSNTdb to illustrate substitution trends. In addition to selection acting upon immunity evasion or similar fitness gains, such trends may reveal interesting patterns related to a balance of selective forces versus random mutations related to basic viral processes, as shown in SARS-CoV (8).
We downloaded mutation data from the NCBI’s SRA using the prefetch feature to download SRA files. Selection of files was complicated by divergent numbers of samples submitted in different projects, so we selected them from several labs across the world, which provided large batches of samples over extended periods of time. We reasoned such labs would have likely perfected the sequencing process and provided reliable samples for different VOCs.
Thus we obtained >35,000 samples and filtered them as follows. Samples under 10MB in size were excluded as they had low read depth overall, preventing reliable detection of single-nucleotide variants (SNVs). We selected samples with <700 nt of zero read depth over the whole genome, to avoid effects of biased coverage and of lack of coverage for potential SNVs (Supplementary Figure 1). To process the data in consistent way, we used fastq files (when available) or unaligned the reads from BAM files using Samtools (9) to convert them to fastq files. We then aligned the fastq files to the SARS-CoV-2 reference sequence (10) (NC_045512.2) using BWA mem.
We then used GROM (11) to find SNVs in the data. In total we found ~25,000 unique SNVs using GROM across >25,000 samples. GROM was run using default settings and the “remove duplicates” option, to minimize PCR duplicates. Relevant data from output VCF files were then consolidated to SQL files detailing the sequencing platform, coordinates, and alternate nucleotides for each sample.
To identify repeats in the SARS-CoV-2 genome, we analyzed the Wuhan reference sequence (10) using UGENE (12) with the default settings and selected repeats >5 nt long. We then identified super-repeats of one another (superstrings of shorter repeat strings) using in-house scripts.
Table 1 describes datatypes, sources, and tools used to generate the data that populates the database. Protein structures were obtained from the Zhang group who has used I-TASSER to predict protein structure for all SARS-CoV-2 proteins (13). Their predictions are highly accurate for the SARS-CoV-2 proteins despite relatively few homologous sequences with available protein structures.
Table 1 A display of the types, sources, and tools used to generate data that populate the database.
To show the secondary structure of SARS-CoV-2 genomic RNA we collected several datasets from groups that have measured the viral RNA accessibility at a single base resolution. The first of these was taken from Manfredonia et al. (16) who has used SHAPE and DMS mutational profiling to find secondary structure maps with single base resolution. Yang et al. (14) has used SHAPE-MaP to find the reactivities of the reference sequence as well as a delta variant sequence. Finally, Sun et al. (15) has used icSHAPE to map reactivities.
Data presented as Intragenome Interaction Data represent regions of pairwise RNA interactions across the genome. Such regions have been detected via proximity ligation sequencing was performed using SPLASH to find these regions in Vero-E6 infected cell (14).
We obtained the coordinates of viral non-coding regions, its genes and proteins, their respective nucleotide and amino acid sequences from the NCBI record of the SARS-CoV-2 (NC_045512.2). SARS-CoV’s information was retrieved in the same way from the NCBI record of the Tor reference sequence (NC_004718.3). We then performed a thorough literature review (across hundreds of papers) of proteins in SARS-CoV-2 and SARS-CoV to obtain their functional descriptions. Next, we identified the available coordinates of functional domains in both viruses. Using BLAST (17) and CLUSTAL-W (18), we further performed pairwise alignments of the proteins of SARS-CoV-2 and SARS-CoV to evaluate the levels of amino acid identity of the homologous functional domains. We manually curated mismatched coordinates of such homologous domains between different studies, produced reconciled coordinates and transferred the domain annotations, further accompanied on respective pages by the publications describing them.
Succinctly, the data in the database is retrieved by users via two main query hubs. One is the Genome Search page and is comprised of several datasets and information retrieved from literature. The other is made available in the Mutation Search page (and Repeat page), presenting results of our re-analysis of >25,000 patient samples obtained from NCBI’s publicly available SRA SARS-CoV-2 genomes. We interlinked these sections comprehensively in order to provide the user an easy way to carry over the findings gained in one section to another.
See Figure 1 (top) for an overview of the data sources and functionality of the database.
Figure 1 Overview of the data sources and functionalities (top). A walkthrough of the case study described in text (bottom).
Users can access SARSNTdb at https://grigoriev-lab.camden.rutgers.edu/sarsntdb/
The website is implemented in PHP (version 7.4.29) and the SQL server through mysql (version 15.1) with MariaDB (distribution 10.3.34).
The interface of the database consists of several tabs. The Search tab has a dropdown menu that brings the user to a Genome Search, Mutation Search, and a Repeat Search. These searches are interconnected to allow the user to take information gleaned from one search into another. The Help tab instructs the user on how to use the website by providing an example. The Reference tab brings the user to this article where they can learn about the data sources and how the website was constructed.
The Genome Search page allows the user to specify nucleotide coordinate intervals and find information about functionally relevant regions of the SARS-CoV-2 virus that overlap or are contained between these coordinate pairs. Such regions most often correspond to genes and functional domains they encode. Also, this search reports about nearby repeats and intragenomic interactions obtained using a SPLASH technique (14).
One can also select a single ORF or Nsp from a menu to get to such genes. Their protein products are described on the Protein Detail page (Figure 2). In addition to images of the predicted structure of the SARS-CoV-2 proteins, their functional domains, smaller motifs, and certain amino acid residues with annotated functionality are also displayed graphically. At the bottom of this page there are the relevant RNA and Protein sequences derived from the respective NCBI reference.
Figure 2 The Genome Detail page of the S protein. Data presented includes a protein structure simulation, functional detail, coordinates for start and end, domain map/ranges, and more. This page links to the SARS-CoV vs SARS-CoV-2 comparison page and reference sequences for the gene and protein.
Similar to evaluations of SARS-CoV-2 mutations using variant effect predictors (19), a link to online analysis of all possible amino acid substitutions for a given protein by SNAP2 (20) is also provided here. A single click would copy the protein FASTA sequence and redirect to the SNAP2 server, so users just need to paste it there, start the analysis and view its results.
Knowing that a substitution occurred in a protein domain with certain function may provide more specific information on the functional effect. Since SARS-CoV-2 domains are typically derived from the previous body of work on SARS-CoV, we devoted a special page for each protein in both viruses for comparing domains. This page is linked form the Protein page and contains a table detailing the similarities of the two viruses and an alignment of both protein sequences created using CLUSTALW (18) and BLAST (17). The coordinates in the table are derived from primary literature and review papers (that can be accessed by clicking the hyperlinks on the coordinates) and sometimes they differ, despite being reasonably well aligned. These pages also illustrate the degree of conservation of the two viruses and links to mutations for each domain are also provided.
The Mutation Search page allows the user to search for mutations in a nucleotide range or within a gene. The search results are bar graphs depicting the number and type of substitutions in the range. The bars are also subdivided by sequencing platforms. If a more granular view of the mutations is needed the user can click on Mutation Detail to see expanded information related to the mutations in the that nucleotide range. Below the Substitution Frequency table there is a histogram that displays SNV frequency across the nucleotide range selected.
Also on this page is SHAPE data that may help inform the user why certain regions may be conserved due to the secondary structure constraints. When the size of the searched region is large, the SHAPE Data is displayed in intervals where the SHAPE value is averaged across that region. If the size of the searched interval is under 100 nucleotides, each position and the SHAPE value is displayed individually. If the shape value is above 0.5 it is displayed in blue indicating a high reactivity while below 0.5 is displayed in red and indicates a low reactivity. We selected several SHAPE datasets and displayed them in separate graphs. These data make up our Mutation Search page and it is visualized on the page using CanvasJS (Fenopix Inc.).
The Repeat Page (Figure 3) allows the user to search the SARS-CoV-2 reference sequence for repeats of size 6 nucleotides or greater. Displayed on this page is the genome schematic with proteins colored distinctly. When a repeat is found red lines appear on the genome indicating repeat locations, and a table displaying the coordinates of the repeats as well as which protein they appear in is displayed. Also available are repeats, which are super-strings containing the searched repeat; these are deemed super-repeats. For example, the repeat AACAGGA is a super-repeat of AACAGG as the former is a super-string of (i.e., contains) the latter. Clicking on these super-repeats brings the user to a Repeat Page for the super-repeat (with their respective super-repeats, if available). For the default search on the Repeat Page and a clear biological example, we provide the minimal repeat of the transcription regulatory sequence (TRS) from the SARS-CoV-2 virus (5), with all locations of canonical TRS visualized throughout the genome for the user.
Figure 3 Visualization of the leader sequence repeat ACGAAC across the genome. This page visualizes the location of repeats across the genome visible as red ticks on top of the genome. In addition, the locations of repeats within coding regions are listed in a table where each protein name is a link to its genome detail page.
As stated previously there are many databases tracking the waves of VOCs and their typical mutations. The virus continues to evolve, and even the general public is made aware of new substitutions in the best-annotated spike protein. When new mutations appear, it is important to be able to quickly identify where they occur and analyze their effects by detecting genome features nearby. Furthermore, substitutions take place not only the spike protein, yet those affecting other parts of the genome are typically ignored in the databases and many analyzes.
In contrast, SARSNTdb could be an excellent starting point for such quick evaluation, and a schematic walkthrough is shown in Figure 1 (bottom). Consider the mutation C28311T, found in Omicron. Let us first go to our Genome Search page and input the coordinate 28311 and find it is part of two overlapping genes, encoding the Nucleocapsid (N) protein as well as ORF9b. In N it is located in the N-terminal arm/Intrinsically disordered region. In ORF9b we see it is part of the site that interacts with the host protein NEMO. We also see that it is a part of some common repeats and has intragenomic interactions at the 5’ end of the protein as well as a region 200nt away that it binds with. These close intragenomic interacting regions could form pockets that may become therapeutic targets (15). Clicking View Details for ORF9b, we find its function and see it supresses the innate immune system through regulating Mitochondrial Antiviral Signaling pathways (21). In comparing it to SARS-CoV we find this domain is not well conserved with only 63% similarity overall. In the paper linked via the domain coordinates in the table, we find that this region, when deleted, resulted in a loss of function of the protein and its interaction with NEMO (21). If this nucleotide change results in a non-synonymous mutation, it could affect the function of the protein. By clicking Mutations on the table, we are brought to the mutation page showing the NEMO interaction region’s mutation frequencies, SHAPE scores and, if we click Detail, a breakdown if that specific mutation has been found. If it has been found the detail page will also show the type of variant it creates. In this case the SNP has been found previously in thousands of samples, where it changed a proline to a serine. In addition, the SHAPE score of this nucleotide is low according to all datasets, indicating that it may be prone to forming secondary structures within the RNA. Overall, with all such results about this mutation we can conclude that it should be monitored as it has been persisting over time and now, with Omicron spreading rapidly, may be gaining increased prevalence. This mutation could affect the ability of ORF9b to supress the innate immune system through interacting with NEMO and its effects should be explored further.
SARSNTdb is a database for users of varying levels of knowledge about virology or genomics. It provides nucleotide-level functional information about various aspects of the SARS-CoV-2 genome. It features a quick and easy coordinate-based search for SARS-CoV-2 gene and protein functions, mutations found in patient samples, structural and sequence elements of the virus RNA and several other features. We reviewed, analyzed, and provided visualization for data that could help users to better understand the virus, and to do this rapidly. We will continue to add mutation data as we process other representative samples from the NCBI to detect SNVs in those with GROM on a regular basis. In addition, should new domain definitions be discovered they will also be added to the database.
The original contributions presented in the study are included in the article/Supplementary Material. Further inquiries can be directed to the corresponding author.
JO - developed code and server, produced content, tested database, wrote paper; JK - collected and analyzed patient samples, analyzed repeats, tested database; OB - produced domain annotations and alignments, contributed to Help section, tested database; SV - contributed to domain annotations, tested database; AG - conceived project, obtained funding, oversaw project execution, tested database, wrote paper. All authors contributed to the article and approved the submitted version.
The work in AG’s lab is supported by the National Science Foundation [MCB-2027611 to AG] and National Institutes of Health [R15CA220059 to AG]. Funding for open access publication fees - National Science Foundation [MCB-2027611 to AG].
We thank Jim Schmincke for excellent technical help and Sudheshna Bodapati for contributions to an early prototype.
The authors declare that the research was conducted in the absence of any commercial or financial relationships that could be construed as a potential conflict of interest.
All claims expressed in this article are solely those of the authors and do not necessarily represent those of their affiliated organizations, or those of the publisher, the editors and the reviewers. Any product that may be evaluated in this article, or claim that may be made by its manufacturer, is not guaranteed or endorsed by the publisher.
The Supplementary Material for this article can be found online at: https://www.frontiersin.org/articles/10.3389/fviro.2022.1028335/full#supplementary-material
1. Hodcroft EB. CoVariants: SARS-CoV-2 mutations and variants of interest (2021). Available at: https://covariants.org/.
2. Khare S, Gurry C, Freitas L, Schultz MB, Bach G, Diallo A, et al. GISAID's role in pandemic response. China CDC Wkly (2021) 3(49):1049–51. doi: 10.46234/ccdcw2021.255
3. Cao Y, Wang J, Jian F, Xiao T, Song W, Yisimayi A, et al. Omicron escapes the majority of existing SARS-CoV-2 neutralizing antibodies. Nature (2022) 602(7898):657–63. doi: 10.1038/s41586-021-04385-3
4. Karim SSA, Karim QA. Omicron SARS-CoV-2 variant: a new chapter in the COVID-19 pandemic. Lancet (2021) 398(10317):2126–8. doi: 10.1016/S0140-6736(21)02758-6
5. Kim D, Lee JY, Yang JS, Kim JW, Kim VN, Chang H. The architecture of SARS-CoV-2 transcriptome. Cell (2020) 181(4):914–21.e10. doi: 10.1016/j.cell.2020.04.011
6. Gobeil SMC, Henderson R, Stalls V, Janowska K, Huang X, May A, et al. Structural diversity of the SARS-CoV-2 omicron spike. Mol Cell (2022) 82(11):2050–68.e6. doi: 10.1016/j.molcel.2022.03.028
7. Sayers EW, Bolton EE, Brister JR, Canese K, Chan J, Comeau DC, et al Database resources of the national center for biotechnology information. Nucleic Acids Res (2016) 44(D1):D7–19. doi: 10.1093/nar/gkab1112
8. Grigoriev A. Mutational patterns correlate with genome organization in SARS and other coronaviruses. Trends Genet (2004) 20(3):131–5. doi: 10.1016/j.tig.2004.01.009
9. Li H, Handsaker B, Wysoker A, Fennell T, Ruan J, Homer N, et al. The sequence Alignment/Map format and SAMtools. Bioinformatics (2009) 25(16):2078–9. doi: 10.1093/bioinformatics/btp352
10. Wu F, Zhao S, Yu B, Chen YM, Wang W, Song ZG, et al. A new coronavirus associated with human respiratory disease in China. Nature (2020) 579(7798):265–9. doi: 10.1038/s41586-020-2008-3
11. Smith SD, Kawash JK, Grigoriev A. Lightning-fast genome variant detection with GROM. GigaScience (2017) 6(10):gix091. doi: 10.1093/gigascience/gix091
12. Okonechnikov K, Golosova O, Fursov M, team tU. Unipro UGENE: a unified bioinformatics toolkit. Bioinformatics (2012) 28(8):1166–7. doi: 10.1093/bioinformatics/bts091
13. Zheng W, Zhang C, Li Y, Pearce R, Bell EW, Zhang Y. Folding non-homologous proteins by coupling deep-learning contact maps with I-TASSER assembly simulations. Cell Rep Methods (2021) 1(3):100014. doi: 10.1016/j.crmeth.2021.100014
14. Yang SL, DeFalco L, Anderson DE, Zhang Y, Aw JGA, Lim SY, et al. Comprehensive mapping of SARS-CoV-2 interactions in vivo reveals functional virus-host interactions. Nat Commun (2021) 12(1):5113. doi: 10.1038/s41467-021-25357-1
15. Sun L, Li P, Ju X, Rao J, Huang W, Ren L, et al. In vivo structural characterization of the SARS-CoV-2 RNA genome identifies host proteins vulnerable to repurposed drugs. Cell (2021) 184(7):1865–83.e20. doi: 10.1016/j.cell.2021.02.008
16. Manfredonia I, Nithin C, Ponce-Salvatierra A, Ghosh P, Wirecki TK, Marinus T, et al. Genome-wide mapping of SARS-CoV-2 RNA structures identifies therapeutically-relevant elements. Nucleic Acids Res (2020) 48(22):12436–52. doi: 10.1093/nar/gkaa1053
17. Camacho C, Coulouris G, Avagyan V, Ma N, Papadopoulos J, Bealer K, et al. BLAST+: architecture and applications. BMC Bioinf (2009) 10:421. doi: 10.1186/1471-2105-10-421
18. Thompson JD, Higgins DG, Gibson TJ. CLUSTAL W: improving the sensitivity of progressive multiple sequence alignment through sequence weighting, position-specific gap penalties and weight matrix choice. Nucleic Acids Res (1994) 22(22):4673–80. doi: 10.1093/nar/22.22.4673
19. Mishra D, Suri GS, Kaur G, Tiwari M. Comparative insight into the genomic landscape of SARS-CoV-2 and identification of mutations associated with the origin of infection and diversity. J Med Virol (2021) 93(4):2406–19. doi: 10.1002/jmv.26744
20. Hecht M, Bromberg Y, Rost B. Better prediction of functional effects for sequence variants. BMC Genomics (2015) 16(8):S1. doi: 10.1186/1471-2164-16-S8-S1
Keywords: SARS-CoV-2, SARS-CoV, database, genome analysis, bioinformatics
Citation: Orgera J, Kelley JJ, Bar O, Vaidhyanathan S and Grigoriev A (2022) SARSNTdb database: Factors affecting SARS-CoV-2 sequence conservation. Front. Virol. 2:1028335. doi: 10.3389/fviro.2022.1028335
Received: 25 August 2022; Accepted: 15 November 2022;
Published: 01 December 2022.
Edited by:
Evangelia Georgia Kostaki, National and Kapodistrian University of Athens, GreeceReviewed by:
Ye Qiu, Hunan University, ChinaCopyright © 2022 Orgera, Kelley, Bar, Vaidhyanathan and Grigoriev. This is an open-access article distributed under the terms of the Creative Commons Attribution License (CC BY). The use, distribution or reproduction in other forums is permitted, provided the original author(s) and the copyright owner(s) are credited and that the original publication in this journal is cited, in accordance with accepted academic practice. No use, distribution or reproduction is permitted which does not comply with these terms.
*Correspondence: Andrey Grigoriev, YW5kcmV5LmdyaWdvcmlldkBydXRnZXJzLmVkdQ==
Disclaimer: All claims expressed in this article are solely those of the authors and do not necessarily represent those of their affiliated organizations, or those of the publisher, the editors and the reviewers. Any product that may be evaluated in this article or claim that may be made by its manufacturer is not guaranteed or endorsed by the publisher.
Research integrity at Frontiers
Learn more about the work of our research integrity team to safeguard the quality of each article we publish.