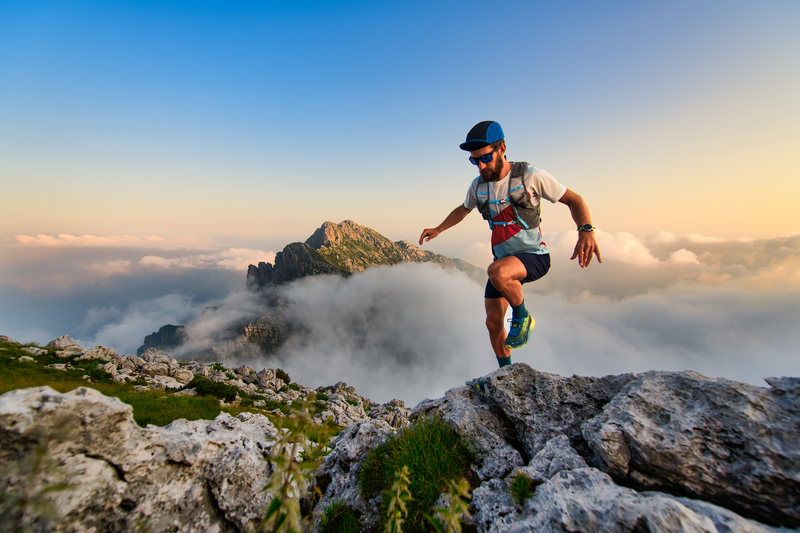
94% of researchers rate our articles as excellent or good
Learn more about the work of our research integrity team to safeguard the quality of each article we publish.
Find out more
ORIGINAL RESEARCH article
Front. Vet. Sci.
Sec. Animal Nutrition and Metabolism
Volume 12 - 2025 | doi: 10.3389/fvets.2025.1569196
The final, formatted version of the article will be published soon.
You have multiple emails registered with Frontiers:
Please enter your email address:
If you already have an account, please login
You don't have a Frontiers account ? You can register here
Tibetan pigs, native to the Qinghai-Tibet Plateau, have adapted over millennia to extreme conditions such as low oxygen, harsh cold, and high UV radiation, impacting their muscle characteristics and digestive tract microbiota. The quality of pork from Tibetan pigs (TP) and black pigs (BP) is influenced by various factors, including genetics, diet, and environmental adaptation. However, the specific influence of digestive tract microbiota metabolites on muscle traits remains poorly understood. Our goal was to correlate omic variations with meat quality traits and identify potential biomarkers predictive of superior meat quality, elucidate the regulatory effects of digestive tract microbial metabolites on Tibetan pig muscle characteristics, and reveal the genetic and nutritional mechanisms that promote adaptation to extreme environmental conditions. This analysis encompassed metabolomic profiling of the entire digestive tract—including the stomach, jejunum, cecum, colon, and rectum—as well as histological, amino acid, fatty acid composition, and transcriptomic assessments of the longissimus dorsi muscle tissues to investigate how digestive tract microbial metabolites influence muscle adaptation to high altitudes. Analyses revealed that Tibetan pig muscles contain smaller, more oxidative fibers enriched with flavor-enhancing amino acids. This was accompanied by a more favorable n-6/n-3 fatty acid ratio. Distinct patterns of microbial metabolites were observed in the digestive tract, influencing protein digestion and purine metabolism, and correlating with muscle glycine levels. Transcriptomic data showed varied gene expression in metabolic pathways related to salivary and pancreatic secretion, as well as carbohydrate and fatty acid metabolism. Integrated multi-omics approaches linked stomach metabolism, particularly through bile secretion pathways influenced by acetylcholine, to muscle functionality, highlighting the important role played by the ATP1B4 gene in enabling muscle physiology in Tibetan pigs. This study highlights the importance of targeted dietary interventions in improving meat quality for specific pig breeds. It also provides a theoretical foundation for precision agriculture strategies aimed at enhancing the meat quality of both TP and BP pigs.
Keywords: Tibetan pig, Black pig, Metabolome, altitude, Muscle
Received: 31 Jan 2025; Accepted: 05 Mar 2025.
Copyright: © 2025 Bai, Huang, Tan, Gu, Wang, Jin, Shang, Long, Li and Li. This is an open-access article distributed under the terms of the Creative Commons Attribution License (CC BY). The use, distribution or reproduction in other forums is permitted, provided the original author(s) or licensor are credited and that the original publication in this journal is cited, in accordance with accepted academic practice. No use, distribution or reproduction is permitted which does not comply with these terms.
* Correspondence:
Diyan Li, Antibiotics Research and Re-evaluation Key Laboratory of Sichuan Province, Chengdu University, Chengdu, China
Mingzhou Li, College of Animal Science and Technology, Sichuan Agricultural University, Chengdu, China
Disclaimer: All claims expressed in this article are solely those of the authors and do not necessarily represent those of their affiliated organizations, or those of the publisher, the editors and the reviewers. Any product that may be evaluated in this article or claim that may be made by its manufacturer is not guaranteed or endorsed by the publisher.
Research integrity at Frontiers
Learn more about the work of our research integrity team to safeguard the quality of each article we publish.