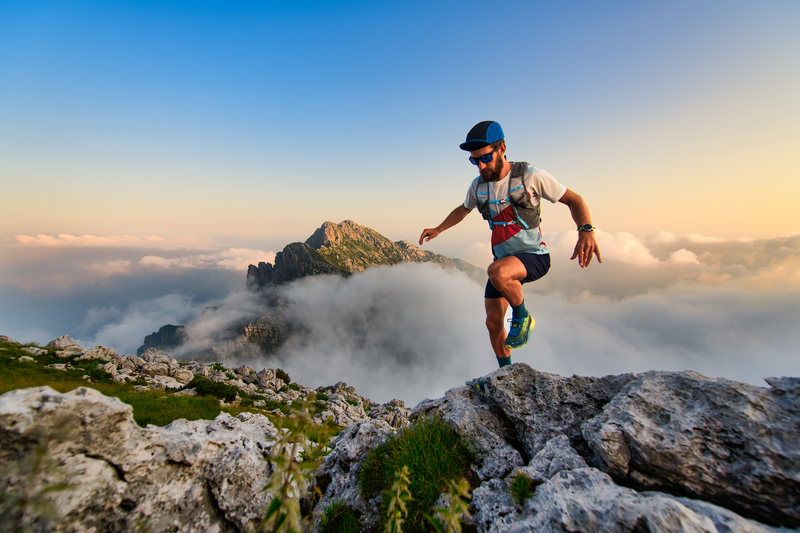
94% of researchers rate our articles as excellent or good
Learn more about the work of our research integrity team to safeguard the quality of each article we publish.
Find out more
ORIGINAL RESEARCH article
Front. Vet. Sci.
Sec. Animal Nutrition and Metabolism
Volume 12 - 2025 | doi: 10.3389/fvets.2025.1551327
This article is part of the Research Topic Dietary Supplements for Optimizing Rumen Health and Nutrient Digestibility in Livestock View all 4 articles
The final, formatted version of the article will be published soon.
You have multiple emails registered with Frontiers:
Please enter your email address:
If you already have an account, please login
You don't have a Frontiers account ? You can register here
The duodenum and its resident microbiota play crucial roles in the process of nutrient digestion and absorption. However, the temporal dynamics of duodenal microbiota in response to different dietary regimes remain are not yet fully understood.The aim of the present study was to explore the effects of high-forage (HF) and highconcentration (HS) diets on the circadian rhythm variation of duodenal fermentation and microbial communities in dairy cattle. Six duodenum-cannulated Holstein dairy cows were assigned to HF and HS diets according to a crossover design with two periods, with each period lasting 21d (18 d for adaptation, 3 d for sampling). Duodenal content samples were collected at six time points (07:00, 11:00, 15:00, 19:00, 23:00, and 03:00) for the analysis of volatile fatty acids (VFA) and characterisation of microbial characterization. The times of 11:00, 15:00, and 19:00 were recorded as the light phase and 23:00, 03:00 and 07:00 were recorded as the dark phase. The results showed that TVFA displayed a significant circadian rhythm following the introduction of the HS diet (p < 0.01). The concentration of TVFA (p < 0.01) and acetate (p < 0.01) were significantly higher during the light than the dark phase, regardless of diet type.PERMANOVA analysis revealed that diet and diet × time interaction strongly influenced the composition of duodenal microbiota. The relative abundance of Lachnospiraceae_ND3007_group showed a positive correlation with the propionate proportions under the HS diet during the light phase, while the HS diet significantly increased the abundance of Bifidobacterium and norank_f__Lachnospiraceae. These findings provide novel insights into diet-dependent circadian regulation of duodenal fermentation in dairy cattle.
Keywords: duodenal microbiota, dairy cattle, Circadian Rhythm, Dietary regimes, Forage-to-concentration ratio
Received: 25 Dec 2024; Accepted: 18 Feb 2025.
Copyright: © 2025 Wang, Xie, Ji, Xia, Hao, Cao, Wang, Gao, Li and Yang. This is an open-access article distributed under the terms of the Creative Commons Attribution License (CC BY). The use, distribution or reproduction in other forums is permitted, provided the original author(s) or licensor are credited and that the original publication in this journal is cited, in accordance with accepted academic practice. No use, distribution or reproduction is permitted which does not comply with these terms.
* Correspondence:
Shengli Li, China Agricultural University, Beijing, China
Kailun Yang, Xinjiang Agricultural University, Ürümqi, 830052, Xinjiang Uyghur Region, China
Disclaimer: All claims expressed in this article are solely those of the authors and do not necessarily represent those of their affiliated organizations, or those of the publisher, the editors and the reviewers. Any product that may be evaluated in this article or claim that may be made by its manufacturer is not guaranteed or endorsed by the publisher.
Research integrity at Frontiers
Learn more about the work of our research integrity team to safeguard the quality of each article we publish.