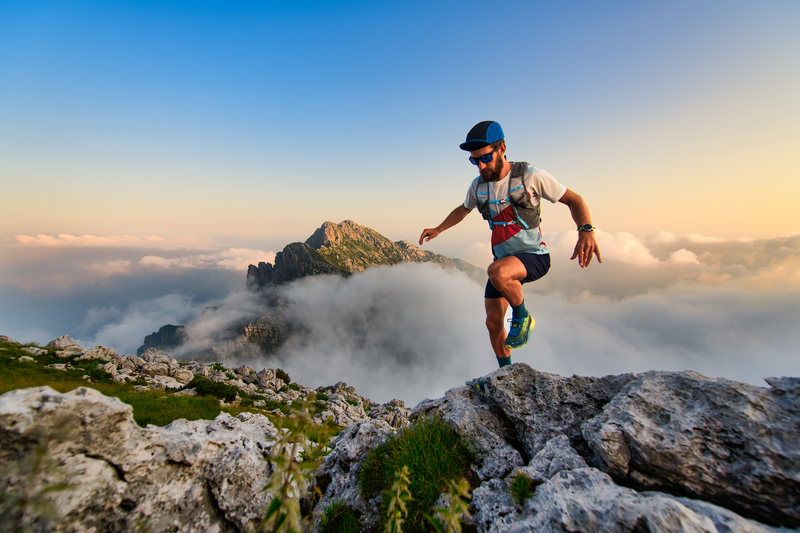
94% of researchers rate our articles as excellent or good
Learn more about the work of our research integrity team to safeguard the quality of each article we publish.
Find out more
ORIGINAL RESEARCH article
Front. Vet. Sci.
Sec. Animal Behavior and Welfare
Volume 12 - 2025 | doi: 10.3389/fvets.2025.1404564
This article is part of the Research Topic Artificial Intelligence in Animal Behaviour, Veterinary Behaviour and Neurology View all 9 articles
The final, formatted version of the article will be published soon.
You have multiple emails registered with Frontiers:
Please enter your email address:
If you already have an account, please login
You don't have a Frontiers account ? You can register here
To enable intelligent management of large-scale farms, a deep learning-based approach for monitoring individual livestock is proposed, together with a three-phase intelligent monitoring system designed for sheep flocks. The system incorporates multipart recognition, facial classification, and facial expression analysis, thereby facilitating inventory counting, facial identity recognition, and health monitoring for sheep within the flock environment. A multi-site detection network was designed for flock inventory counting, achieving detection of both lamb and adult sheep faces, with an emphasis on the rear of the sheep to facilitate counting. The YOLOv5s path aggregation network topology was modified through the incorporation of a multi-link convolution fusion block (MCFB), thereby improving fine-grained feature extraction for objects of different sizes. To further enhance the detection of dense small targets, a Re-Parameterizable Convolution (Repconv) structure was integrated into the YOLOv5s head. The network's ability to extract key facial features was further improved by replacing the sixth-stage structure in GhostNet with a four-layer spatially separable selfattention mechanism (SSSA) to enhance facial identity recognition. Additionally, model compression experiments were performed on the facial expression analysis network to improve detection speed and efficiency. A transfer learning strategy was employed for weight pre-training, with FPS, model weight, mean average precision (mAP), and test set accuracy as the primary evaluation metrics. The results showed that the enhanced multi-part identification network effectively extracts features from different regions of the sheep flock, achieving an average detection accuracy of 95.84%.In comparison to YOLOv5s, mAP improved by 2.55%. The improved facial classification network achieved a test set accuracy of 98.9%, a 3.1% increase compared to GhostNet. Furthermore, the facial expression analysis network reached a test set accuracy of 99.2%, representing a 3.6% increase compared to EfficientNet, thus enabling real-time facial expression detection.
Keywords: YOLOv5, MCFB, SSSA, Compression of model, Transfer Learning
Received: 21 Mar 2024; Accepted: 10 Feb 2025.
Copyright: © 2025 Zhang, Zhao, Wang, Qiu, Fu and Zhang. This is an open-access article distributed under the terms of the Creative Commons Attribution License (CC BY). The use, distribution or reproduction in other forums is permitted, provided the original author(s) or licensor are credited and that the original publication in this journal is cited, in accordance with accepted academic practice. No use, distribution or reproduction is permitted which does not comply with these terms.
* Correspondence:
Fu Zhang, Henan University of Science and Technology, Luoyang, China
Sanling Fu, Henan University of Science and Technology, Luoyang, China
Disclaimer: All claims expressed in this article are solely those of the authors and do not necessarily represent those of their affiliated organizations, or those of the publisher, the editors and the reviewers. Any product that may be evaluated in this article or claim that may be made by its manufacturer is not guaranteed or endorsed by the publisher.
Research integrity at Frontiers
Learn more about the work of our research integrity team to safeguard the quality of each article we publish.