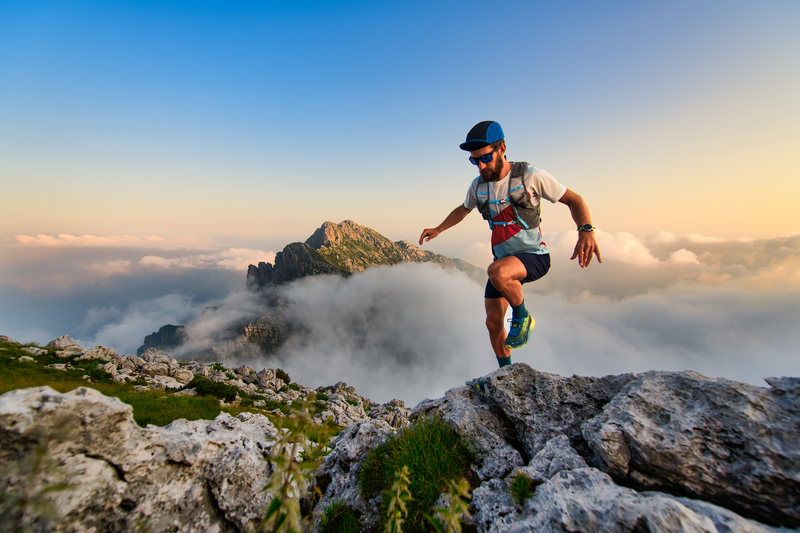
95% of researchers rate our articles as excellent or good
Learn more about the work of our research integrity team to safeguard the quality of each article we publish.
Find out more
ORIGINAL RESEARCH article
Front. Vet. Sci. , 24 October 2024
Sec. Livestock Genomics
Volume 11 - 2024 | https://doi.org/10.3389/fvets.2024.1461075
This article is part of the Research Topic Enhancing Livestock Breeding through Advanced Genetic Tools and Phenotyping Systems View all 5 articles
The study presents a comprehensive investigation into the genetic determinants of 100-day milk yield (100DMY), 305-day milk yield (305DMY), total milk yield (TMY), and persistency using first lactation records of 374 Holstein heifers reared in a private farm at Çanakkale province of Türkiye, employing a genome-wide association study (GWAS) approach. The research underscores the substantial genetic component underlying these economically important traits through detailed descriptive statistics and heritability estimations. The estimated moderate to high heritabilities (0.32–0.54) for milk production traits suggest the feasibility of targeted genetic improvement strategies. By leveraging GWAS, the study identifies many significant and suggestively significant single nucleotide polymorphisms (SNP) associated with studied traits. Noteworthy genes have identified in this analysis include BCAS3, MALRD1, CTNND2, DOCK1, TMEM132C, NRP1, CNTNAP2, GPRIN2, PLEKHA5, GLRA1, SCN7A, HHEX, KTM2C, RAB40C, RAB11FIP3, and FXYD6. These findings provide valuable understandings of the genetic background of milk production and persistency in Holstein cattle, shedding light on specific genomic regions and candidate genes playing pivotal roles in these traits. This research contributes valuable knowledge to the field of dairy cattle genetics and informs future breeding efforts to improve milk production sustainability and efficiency in Holstein cattle populations.
Milk is an essential natural source of valuable animal nutriments that is vital to human nutrition worldwide. Worldwide population growth necessitates the ongoing prioritization of milk yield in cattle breeding while concurrently minimizing reproductive and health issues. Oltenacu and Broom (1) highlight that regions such as Europe, North America, and Nordic Countries have already integrated the enhancement of fertility and reduction of mastitis incidence into their breeding objectives. This strategic approach underscores the importance of addressing key health and reproductive concerns in dairy cattle populations to enhance overall herd performance and welfare. By prioritizing these objectives in breeding programs, stakeholders aim to foster more resilient and productive dairy herds, ultimately contributing to sustainable and profitable dairy farming practices. Wiggans et al. (2) highlighted a significant transformation in dairy cattle breeding attributable to implementing genomic selection. The adoption of this approach has resulted in a doubling of the genetic progress rate, facilitated by the enhanced accuracy in estimating the genetic merit of young animals. The intensified utilization of genetically superior young male and female animals identified at an early age decreases the generation interval (3). Numerous researchers are dedicated to delving into animal genomes, with a particular emphasis on the multitude of genes that play significant roles in regulating production traits in dairy cattle. Candidate gene identification is crucial for the genomic background underlying the interested traits, and various methods are employed for this purpose. One of the most recent methods is the Genome-wide Association Study (GWAS), as highlighted by Taherkhani et al. (4). Several GWAS studies have been conducted with the aim of identifying quantitative trait loci (QTL), genomic regions, and/or single nucleotide polymorphisms (SNPs) associated with milk production traits in different Holstein populations (5–10). However, there is a notable lack of studies focusing on the genetic architecture of milk production traits in Holstein cattle within the specific context of Türkiye. Türkiye presents a unique environment for dairy production due to its diverse climatic regions, which can significantly influence the expression of genetic traits in cattle. These environmental variations may lead to different gene expressions affecting milk production, necessitating research that specifically addresses the genomic characteristics of Holstein cattle in Türkiye. Türkiye’s primary focus remains on enhancing milk yield, making it imperative to understand the genetic factors influencing this trait within the local context. By investigating the genetic parameters associated with milk production in Holstein cattle raised in Türkiye, this study aims to fill a critical gap in the literature and provide valuable insights for improving dairy productivity through targeted breeding programs. The objectives of this study are to estimate the genetic parameters associated with key milk production traits, including 100-day milk yield (100DMY), 305-day milk yield (305DMY), total milk yield (TMY), and persistency, and to identify novel SNPs and candidate genes linked to these traits. By employing a GWAS approach, this research looks forward to enhance our understanding of the genetic foundation of milk production in Holstein cattle under the specific conditions of Türkiye, ultimately contributing to the development of more efficient and sustainable dairy farming practices in the region.
Ethical approval is not needed for this study as the animals were genotyped using routinely collected blood samples from the farm where the research was conducted.
The first lactation records of 374 American originated Holstein heifers raised in a private farm located in Çanakkale province were used in this study. The heifers calved between 2017 and 2021. These animals were daughters of 40 sires and 345 dams. All the data were acquired from the daily milk records collected by an automatic farm management system (GEA Farm Technologies). The cattle were fed using a standardized feeding system tailored to meet their specific needs for growth, reproduction, and milk production, and housed in well-ventilated barns with access to clean water and suitable bedding. Only the animals with lactation lengths of 200–500 days were included in the analyses. Persistency was calculated as follows:
DNA samples of the animals were used for genotyping by Illumina BovineSNP50K BeadChip. The reference genome assembly was based on cattle genome UMD 3.1 (NCBI-http://www.ncbi.nlm.nih.gov). Before GWAS, imputation was performed using an in-home Excel VBA Add-in, which utilize the kNN algorithm. QC filtering was applied to imputed data to decrease type 1 errors. The raw data comprised 62,902 SNPs for each of the 377 cows at the end of genotyping. SNPs on sex and do not belong to any chromosomes were excluded. SNPs were excluded from subsequent analysis if they had a call rate below 95%, a minor allele frequency under 5%, a Hardy–Weinberg equilibrium (HWE) test p-value less than 1E-04 or were identified as duplicate SNPs. Additionally, three samples were excluded due to having over 10% missing SNP genotypes. Following data filtering, a total of 42,444 single nucleotide polymorphisms (SNPs) and 374 individual animals were retained for subsequent genome-wide association studies (GWAS) analyses. This rigorous selection process ensures that only high-quality genetic markers and a representative sample size are utilized, allowing for robust and reliable investigations into the genetic basis of the traits under study.
MINITAB software was used to obtain descriptive statistics and determine the significancy of the environmental factors for the traits. The animals with outlier records (μ ± 3 standard deviations) were discarded from the data. We estimated the variance components for each trait using average information restricted maximum likelihood (AI-REML) accommodating genomic relationship matrix by following mixed model:
where y was the vector of phenotypic records; μ was the population mean related to the traits; β was the vector of significant environmental fixed effects including calving year (2017–2021) and season (winter, spring, summer, and fall); a was the vector of random animal effects; e was the random residual error; and X and Z were incidence matrices attributing phenotypic observations to fixed and additive genetic effects. The lactation length was added to the model as a covariate in the run of TMY analysis. WOMBAT (Version 07/03/2023) (11) was used in the formation of a genomic relationship matrix and estimation of variance components and multiple SNP effects with the guide of the software handbook. Genome-wide (1.17 x 10E-06, = 0.05/42444) and chromosome-wide [3.42 x 10E-05, = 0.05/(42,444/29)] Bonferroni thresholds were used for statistical significance. Additionally, suggestive threshold of <3.00 x 10E-04 was considered suggestively significant for associations. Manhattan plots to display the -log10 (p-value) of SNP effects and Quantile-Quantile (Q-Q) plots to compare the genome-wide distribution of the test statistics with the expected null distribution were created using the qqman package (12) version 0.1.8 in R version 4.3.0 (The R Foundation, Vienna, Austria). The median of the observed chi-squared test statistic divided by the expected median of the corresponding chi-squared distribution is the definition of genomic inflation factor (λ). According to Williams et al. (13), a genomic inflation factor (λ) close to 1 indicates no significant evidence of inflation, suggesting that the statistical analysis is not biased by population stratification or other confounding factors. In the context of genome-wide association studies (GWAS), values of λ up to 1.10 are typically deemed acceptable, as they indicate minimal inflation and thus maintain the integrity of the results.
National Center for Biotechnology Information database1 were used to obtain nearby genes related to each SNP. Genes including or nearest to significant SNPs were considered as candidates.
Gene Ontology (GO) functional annotation and KEGG signaling pathway analysis were performed to gain deeper insights into the biological significance of the candidate regions in various biological processes, molecular functions, and cellular components. The genes located within 200 kb upstream and downstream of these SNPs were submitted to the Database for Annotation, Visualization and Integrated Discovery (DAVID) platform (14, 15). A p-value below 0.05 was deemed statistically significant in the enrichment analysis.
The descriptive statistics for milk production traits and persistency of Holstein cattle are shown in Table 1. Additive and residual variance components are shown in Table 2.
Estimated direct heritabilities for the 100DMY, 305DMY, TMY, and persistency were 0.42, 0.54, 0.47, and 0.32, respectively. These estimations were moderately high and implied a possibility for faster genetic progress. Some cows start the lactation with a high production capacity. However, inadequate energy intake in high-yielding cows during early lactation can elevate the risk of ketosis, which arises from an energy imbalance (16). According to Gustafsson et al. (16), ketotic animals exhibited lactation curves with an abnormal shape, characterized by an inverted peak during early lactation. Most of the decrease in milk yield for these animals likely occurred within the first 100 days of lactation. Moderate genetic variability for this trait may provide an opportunity to improve the starting yields of the cows and/or heifers. Utilizing both genomic relationship and pedigree-based information in heritability estimation may lead to more accurate and higher heritability estimates for 305DMY. Our study yielded a notably higher estimate (0.54) for the 305DMY. This finding contrasts with previous research by Nayeri et al. (17), Atashi et al. (6), Eiríksson et al. (18), and Zhou et al. (19), who reported higher and closer heritabilities for 305DMY, ranging from 0.37 to 0.43 in Holstein and Xinjiang Brown breeds. Conversely, several studies documented lower heritability estimates, ranging from 0.03 to 0.29, across various cattle breeds (5, 20–23). Additionally, Schmidtmann et al. (24) reported a heritability of 0.333 for the 305-day milk yield in the first lactation of German Holstein cattle. Regarding the TMY trait, our study found a moderate heritability estimate of 0.47, contrasting with a lower estimate of 0.24 reported by Eiríksson et al. (18) in Icelandic dairy cows. These variations in heritability estimates across studies underscore the influence of factors such as breed, management practices, and environmental conditions on milk production traits in cattle populations. The difference could be due to the genomic relationship matrix used in this study, breed, and statistical methods. Heifers that demonstrate lower milk production during the first 100 days of lactation, in contrast to the rest of the lactation period, typically show greater persistency. The heritability estimate for persistency was 0.32, indicating the potential for a more robust selection response. Madsen (25) and Tekerli et al. (26) underlined that the moderate yield at the beginning of lactation with moderate persistency minimizes the problems leading to reproductive or metabolic disorders. Focusing solely on milk yield during selection can lead to a significant negative energy balance in cows. This imbalance prompts cows to mobilize body fat reserves to compensate for the lack of nutrients required for milk production (27, 28). Moreover, Pereira et al. (28) cautioned against solely focusing on total milk yield in selection processes, emphasizing the importance of considering lactation persistency in dairy breeding programs. These insights highlight the need for a more holistic approach to breeding strategies, one that accounts for both milk quantity and persistency to ensure the long-term health and productivity of dairy herds. Cows with a flat curve require less concentrate feed during lactation, so we suggest keeping these animals in the herd. Therefore, an index considering milk yield, persistency, milk fat, protein and other sustainable criteria could be used for the improving of dairy cows. In Table 3, genetic and phenotypic correlations among all traits are presented. These correlations were notably strong and positive, ranging between 0.70 and 0.99 for both genetic and phenotypic measures. Strong correlations between 100DMY and 305DMY or TMY indicated that the cows starting lactation with a high yield have the genetic potential to give more milk in the remaining part of the lactation. Genetic and phenotypic correlations of persistency with 100DMY were negative and implied the cows giving most of the lactation milk yield in the first 100 days could have a low persistency. The positively moderate genetic and phenotypic correlations observed between persistency and 305DMY and TMY suggest that selecting for higher persistency may lead to the emergence of cows with increased milk yield.
Table 3. Genetic (below diagonal) and phenotypic correlations amongst 100DMY, 305DMY, TMY, and persistency.
p-values obtained from GWAS were used to visualize the Q-Q plots. False positive results in which many SNP departed from expected probability in genome-wide association study may be due to stratification in population (7, 29). The Manhattan and Quan-tile-Quantile (Q-Q) plots illustrating the Genome-wide Association Study (GWAS) results are depicted in Figures 1–4. These plots provide visual representations of the statistical significance and distribution of genetic variants associated with the traits under investigation. Q-Q plots revealed acceptable patterns of associations and indicated that significant associated SNPs were obtained for all traits. Lambda inflation factors for 100DMY, 305DMY, TMY, and persistency were 1.02, 1.02, 0.99, and 1.01, respectively. These findings indicate the absence of notable inflations, and no corrections were per-formed for lambda since the values are very close to 1 or just slightly above. Information for the SNPs associated with studied traits were presented in Table 4. Genes within the proximity of associated SNPs were significantly enriched in some biological process (BP), molecular functions (MF), and cellular components (CC), and presented in Table 5.
Figure 1. Manhattan and QQ plots of p values for 100DMY. The red and blue lines represents the chromosome-wide (3.42 x 10E-05) and suggestive significance (3.00 x 10E-04) thresholds, respectively.
Figure 2. Manhattan and QQ plots of p values for 305DMY. The red and blue lines represents the chromosome-wide (3.42 x 10E-05) and suggestive significance (3.00 x 10E-04) thresholds, respectively.
Figure 3. Manhattan and QQ plots of p values for TMY. The red and blue lines represents the chromosome-wide (3.42 x 10E-05) and suggestive significance (3.00 x 10E-04) thresholds, respectively.
Figure 4. Manhattan and QQ plots of p values for Persistency. The red and blue lines represents the chromosome-wide (3.42 x 10E-05) and suggestive significance (3.00 x 10E-04) thresholds, respectively.
Table 5. Enrichment of gene ontology (GO) terms and functional annotations of the candidate genes included in the 200 kb upstream and downstream of the identified SNPs.
Following the genome-wide association studies, 27 SNPs were identified as potential candidates associated with 100DMY, 305DMY, TMY, and persistency in Holstein heifers (Table 4). There was a total of eight SNPs associated with 100DMY trait. One SNP exceeded the chromosome-wide significance threshold, and seven SNPs were found to be suggestively significant. The SNP rs109082401 had achieved chromosome-wide significance and is located within the VPS53 gene. Martins et al. (30) have identified this gene as pivotal for back fat thickness in Nellore cattle reared in a pasture-based system. This finding under-scores the significance of the gene in regulating adipose tissue deposition, a trait of considerable importance in cattle production systems. The second highly significant SNP (rs110392985) was detected in BCAS3 (Breast carcinoma-amplified sequence 3 homolog) gene on chromosome 19. Marina et al. (31) identified this gene as a candidate gene through a genome-wide association study investigating the genetic basis of cheese yield in Churra sheep. Parmigiano Reggiano cheese as opposed to others.
In a study by Massender et al. (32), the BCAS3 gene was additionally linked to fat percentage in the initial lactation of Saanen goats. Similarly, Persichilli et al. (33) reported that the BCAS3 gene exhibited significance among Italian Holstein populations, particularly in those involved in the production of Also, Kim et al. (34) suggested BCAS3 gene as a candidate in a selection signature study using three Korean cattle breeds. In our study, another suggestively significant single nucleotide polymorphism (SNP), rs41624433, was identified within the MALRD1 (MAM and LDL receptor class A domain containing 1) gene. This gene has also been previously highlighted by Di Gerlando et al. (35) in their investigation focusing on milk production traits in Valle del Belice dairy sheep. Their study identified MALRD1 as a significant candidate associated with milk and fat yields, mirroring the findings observed in our research. This consistency across studies underscores the potential importance of MALRD1 in modulating milk production traits in dairy sheep populations and highlights its candidacy as a target for further investigation and breeding efforts aimed at improving milk yield and quality. This gene also reported as a potential candidate for gestation length of Large White pig population (36) and rear leg rear view of Korean Holstein cattle (37). Another suggestively significant SNP (rs41616381) is 500 kb away from the CTNND2 gene, which have been suggested to have association with milk yield for first lactation in water buffaloes (38). Gan et al. (39) reported that the CTNND2 gene was found to be related to the thyroxine hormone, which was reported to increase the milk yield, fat, and lactose content (40). Venturini et al. (41) conducted a study on Brazilian buffalo, revealing the CTNND2 gene’s association with milk, fat, and protein yields. Liu et al. (42) obtained results regarding the CTNND2 gene as a candidate for protein yield in Holsteins. Zhao et al. (43) identified this gene as linked to litter birth weight in pigs in a previous investigation. Considering the literature, this gene’s involvement in milk, fat, and protein yields suggests its potential importance in regulating milk production metabolism. Our findings are supported by an alternative SNP (rs110280081) that exceeds the suggestive threshold and is in the DOCK1 gene, which has previously been reported to be involved in milk synthesis (44, 45). We found a SNP (rs42295395) in the CADM1 gene which is assigned as a candidate gene for C18:1n9 fatty acid by Buitenhuis et al. (46). Last suggestively significant SNP for 100DMY, was in the upstream of TMEM132C gene. Interestingly, this gene has been identified in the region harboring selection signatures for Sarabi cattle in Iran, and researchers have revealed that this gene could be associated with economically important traits (47). Similarly, Yodklaew et al. (48) found that the TMEM132C gene was in the near of top 10 significant SNP markers for milk yield. In the Shanghai Holstein population, Liu et al. (42) proposed the TMEM132C gene as a potential candidate influencing protein yield.
After the GWAS, we obtained eight suggestively significant SNPs for 305DMY. One of these SNPs (rs110332624) was in the RAB40C gene that is noted as a candidate for reproduction traits in pigs (49) and cattle (50). Gene ontology analysis revealed that the genes located around this SNP are involved in 2,4-dienoyl-CoA reductase (NADPH) activity (GO:0008670) and fatty acid catabolic process (GO:0009062). It was reported that the cows with a high liver fat content during early lactation negatively influenced the NADPH-dependent glutathione system (51). In addition, NADPH is crucial for milk fatty acid synthesis (52, 53). Genetic variations in this region may influence key pathways involved in fatty acid metabolism and redox balance, potentially impacting traits such as milk production and liver function in livestock. On the other hand, the SNP coded as rs41631692 was in the NRP1 gene, which has been previously linked with milk production and composition traits (31, 42, 54). Another SNPs, rs41639316 and rs109145830 related to the PXYLP1 and LBD2 genes that are already reported for eye muscle area of Hanwoo cattle (55) and carcass traits of Beijing-You chickens (56). The SNP rs110760798 was located within RAB11FIP3 gene. The 200 kb upstream and downstream of the locus contains several genes such as HBM, HBA, HBQ1, and HBA1 which are significantly (p < 0.05) enriched in two biological process named as oxygen transport (GO:0015671) and hydrogen peroxide catabolic process (GO:0042744), six molecular functions entitled as haptoglobin binding (GO:0031720), organic acid binding (GO:0043177), oxygen carrier activity (GO:0005344), oxygen binding (GO:0019825), peroxidase activity (GO:0004601), and heme binding (GO:0020037), and three cellular components called as hemoglobin complex (GO:0005833), haptoglobin-hemoglobin complex (GO:0031838), and blood microparticle (GO:0072562). Zeng et al. (57) reported that the oxygen transport of high-yielding dairy cows under heat stress has deteriorated. Moreover, blood oxygen metabolism is confirmed to be significantly connected with high production of milk in Holstein-Friesian Dairy cows (58). High haptoglobin values in dairy cows have been shown to be strongly correlated with white blood cells, indicating an inflammatory response (59). The haptoglobin concentration in dairy cattle showed a downward trend throughout lactation, and high concentrations in fresh cows could result from inflammatory illnesses without clinical disease, stress, or variations within the physiological statement (60). It is hypothesized that this region may influence oxidative stress responses and oxygen metabolism, which could subsequently impact milk production efficiency and dairy cows’ inflammatory status, particularly under conditions of heat stress and high production demands. Interestingly, another suggestively significant SNP (rs41624433) was found in the MALRD1 (MAM and LDL receptor class A domain containing 1) gene, which was also significant for 100DMY in the current study. This gene has previously explored as a candidate for milk and fat yields of Valle del Belice sheep (35), gestation length in Large White pigs (36) and rear leg rear view for Korean Holstein (37). This SNP has emerged as significant with the suggestive threshold for both 100DMY and 305DMY traits in the study. So, QTLs that significantly affect milk yield traits could be hypothesized to exist within or around the MALRD1 gene. Lastly in 305DMY, the SNP (rs42760684) located in the CNTNAP2 gene has been detected to be related with the 305DMY trait, and some literature about the CNTNAP2 revealed supportive results regarding the effects on C18:3 n3 fatty acids (61), 305-d fat yield in dairy cattle (62), scrotal circumference in Italian Simmental (63), fat yield in Murrah buffalo (64), and 305-d milk yield in Murrah buffaloes (65). So, gene could play a role in the metabolic processes related to milk production and is important for further investigations.
A total of six SNPs were identified to be associated with total milk yield (TMY) in the first lactation. The most significant marker (rs42149741) exceed the chromosome-wide threshold and is in the GPRIN2 gene on chromosome 28. According to Anton et al. (66), the GPRIN2 gene was associated with fertility in Hungarian Simmental cattle. Remarkably, Lee et al. (67) identified the GPRIN2 gene as one of the genes associated with breast cancer in humans. This underscores the potential significance of this gene in the underlying mechanism of mammary functionality. The SNP (rs43110224) located in the upstream of the FXYD6 gene with a distance of 2 kb was suggested as a candidate for TMY in Holstein cattle. This gene, in conjunction with the FXYD2 gene situated in proximity, plays a role in the biological processes of monoatomic ion transport (GO:0006811), regulation of monoatomic ion transport (GO:0043269), and regulation of sodium ion transmembrane transporter activity (GO:2000649). In another GWAS research, FXYD6 gene shows an association with Yak’s body height and researchers (68) reported that this gene encodes a transmembrane protein mediating the Na/K ion pump and accelerates the Na + deactivation and Na + pump. In alignment with our findings, Teng et al. (69) documented a significant association between the FXYD6 gene and protein percentage in Holstein cattle. This corroborates the importance of FXYD6 in regulating milk composition traits, particularly protein content, which is crucial for dairy product quality and nutritional value. Another suggestive SNP (rs41611290) is in the PLEKHA5 gene and pointed out this gene could be involved in the milk related traits. Pedrosa et al. (70) associated this gene with the fat yield of North American Holstein cattle. Furthermore, Wang et al. (9) identified the PLEKHA5 gene as being linked with both fat yield and percentage in cattle, suggesting its involvement in regulating milk composition traits. This research also reported pleiotropic effects of PLEKHA5 on various aspects of milk production, indicating that this gene may exert influence over multiple traits simultaneously. This gene has also been linked to total collagen content in Hanwoo cattle meat (71) and carcass weight in Hawai’i cattle (72). Our research indicates that the PLEKHA5 gene could be considered a potential candidate for influencing milk production in cattle. The SNP (rs42644939) located in upstream of GLRA1 gene was found to be suggestively significant for TMY in our study. In Lázaro et al.’s recent study (64), the GLRA1 gene emerged as a promising candidate influencing milk protein yield in Murrah buffaloes. This finding underscores the genetic complexity underlying the production of milk not only in cattle but also in other dairy species like buffaloes. Additionally, Makina et al. (73) and Saravanan et al. (74) both recognized this gene as a candidate in selection signature studies conducted on Holstein and Sahiwal cattle, respectively. The other suggestive SNP (rs137603336) associated with TMY of Holstein heifers is in the DTNA gene. While this gene has not been previously associated with milk yield traits, recent studies have identified it as a potential candidate for other important traits. Manca et al. (75) found evidence suggesting its involvement in residual concentrate intake in Italian Brown Swiss, while Serão et al. (76) identified it as a potential candidate for residual average daily gain in beef cattle. Moreover, Chen et al. (77) reported differential expression of the PLEKHA5 gene in cattle exhibiting high and low residual feed intake. This suggests that PLEKHA5 may play a role not only in milk production traits but also in feed efficiency, indicating its broader influence on cattle metabolism and performance. Understanding the relationship between PLEKHA5 expression and feed intake can provide valuable insights into the genetic basis of feed efficiency and guide efforts to improve feed utilization and reduce pro-duction costs in cattle farming.
Five SNPs were found suggestively significant in persistency. The first SNP (rs109742720) is in the NEU3 gene on chromosome 15. In a study conducted by Cochran et al. (78), the NEU3 gene was identified as being associated with several important agricultural traits in cows. These traits include daughter pregnancy rate, cow conception rate, productive life, and net merit. This research provides valuable insights into the genetic factors influencing the reproductive efficiency and overall productivity of dairy cattle. By identifying genes associated with important traits such as milk production, feed efficiency, daughter pregnancy rate, and cow conception rate, researchers acquire a more profound comprehension of the fundamental genetic mechanisms governing these traits. Such insights are essential for developing targeted breeding programs aimed at enhancing the reproductive performance and overall productivity of dairy cattle populations. By leveraging this knowledge, breeders can make informed decisions to selectively breed animals with desirable genetic traits, ultimately leading to more efficient and profitable dairy farming practices. The second SNP (rs109266162) for persistency is in the SCN7A gene on chromosome two. This gene plays a role in cellular homeostasis (GO:0019725), which is essential for maintaining equilibrium within an animal’s physiological state (79). It is particularly important for dairy cattle that produce large quantities of milk, as it helps maintain calcium (Ca) homeostasis through the absorption of Ca from the intestine and the mobilization of Ca from bone tissue (80). The SCN7A gene, which is essential for maintaining cellular homeostasis, highlights its potential involvement in calcium regulation and overall physiological balance, which are crucial for supporting high milk production and maintaining health in dairy cattle. According to findings of Rahmatalla et al. (81), the SCN7A gene may have potential associations with milk traits in cattle. This suggests a genetic link between SCN7A, and various characteristics related to milk production, which could have implications for breeding strategies aimed at enhancing milk quality and quantity in dairy cattle populations. The third SNP (rs41626189) located in C16H1orf100 gene, but no literature reports are available for the gene. Another SNP called rs109008551 was located near the HHEX gene. Pedrosa et al. (70) discovered that the SCN7A gene is linked to milk fat yield specifically in North American Holstein cattle. This suggests a significant role for SCN7A in influencing the production of milk fat in this breed, supplying valuable insights into the genetic mechanisms that dictate milk composition traits within dairy cattle populations. Mohammadi et al. (82) had suggested the HHEX gene for days open in Iranian Holstein cattle. It was determined that the gene could have effects on type 2 diabetes, insulin mechanism, and milk production (83–85) in last decades. So, Schmidt (86) previously showed that insulin injection into lactating cows interestingly caused a decrease in milk production, milk lactose content, and blood glucose levels, and an increase in milk fat and protein percentages. According to Puppin et al. (87), the HHEX gene possesses the potential to serve a critical function in the differentiation of mammary epithelial cells. This finding suggests that HHEX may contribute significantly to the complex regulatory processes involved in the function and development of mammary tissue, which is essential for milk production in dairy cattle. Understanding the role of HHEX in mammary epithelial cell differentiation could offer valuable insights into the molecular mechanisms underlying lactation and potentially inform strategies for improving milk production efficiency in dairy farming. Furthermore, the HHEX gene has been reported to be an important transcriptional regulator for miRNA regulatory networks that control milk yield (85). Therefore, the HHEX gene could be considered a candidate gene due to its possible involvement in the regulation of milk production. The last SNP (rs41618099), suggestively associated with persistency, was 12 kb away from the KTM2C gene. According to Wang et al. (88) and Wang et al. (89), this gene is associated with milk protein and calcium accumulation mechanisms. This study suggests that it may be a candidate gene for persistence.
This study represents the pioneering effort to detect candidate regions related with milk yield and persistency in Holstein cattle raised in Türkiye. Several SNPs were identified to be significant with these traits. Genetic parameters were estimated, revealing moderate to high heritabilities for all traits. It is noteworthy that novel candidate genes were identified, which shed light on some of the molecular pathways underlying these traits. Such discoveries significantly contribute to unravelling the genetic underpinnings of milk production traits in cattle. They offer crucial insights that could guide future breeding programs aimed at optimizing productivity and profitability within the dairy industry. By understanding the genetic factors influencing milk traits like fat yield, breeders can make informed decisions to select for desirable characteristics, ultimately leading to more efficient and economically viable dairy cattle populations.
The data presented in the study are deposited in APERTA open archive repository of The Scientific and Technological Research Council of Türkiye (TÜBİTAK) with accession number 274008. https://aperta.ulakbim.gov.tr/record/274008.
Ethical approval was not required for the studies involving animals in accordance with the local legislation and institutional requirements because the animals were genotyped with the routinely collected blood samples on the farm where the animals were reared. Written informed consent was obtained from the owners for the participation of their animals in this study.
ME: Conceptualization, Investigation, Methodology, Validation, Writing – review & editing. SÇ: Conceptualization, Data curation, Formal analysis, Investigation, Methodology, Resources, Visualization, Writing – original draft. BB: Investigation, Resources, Writing – review & editing. KÇ: Investigation, Resources, Validation, Writing – review & editing. MD: Data curation, Formal analysis, Investigation, Resources, Writing – review & editing. SS: Investigation, Resources, Writing – review & editing. MT: Conceptualization, Data curation, Formal analysis, Investigation, Methodology, Project administration, Resources, Supervision, Validation, Writing – review & editing.
The author(s) declare that no financial support was received for the research, authorship, and/or publication of this article.
The authors are grateful to the KAANLAR Corporation, ANIMAGEN and MST Medical Companies for providing data and assisting in this study. In order to enhance the quality of the writing, the author(s) were utilised the OpenAI/Chat GPT-4 (https://chatgpt.com/) tool to refine the grammar and style.
SS was employed by Kaanlar Agriculture and Livestock Farm.
The remaining authors declare that the research was conducted in the absence of any commercial or financial relationships that could be construed as a potential conflict of interest.
The author(s) declared that they were an editorial board member of Frontiers, at the time of submission. This had no impact on the peer review process and the final decision.
All claims expressed in this article are solely those of the authors and do not necessarily represent those of their affiliated organizations, or those of the publisher, the editors and the reviewers. Any product that may be evaluated in this article, or claim that may be made by its manufacturer, is not guaranteed or endorsed by the publisher.
1. Oltenacu, PA, and Broom, DM. The impact of genetic selection for increased milk yield on the welfare of dairy cows. Anim Welf. (2010) 19:39–49. doi: 10.1017/S0962728600002220
2. Wiggans, GR, Cole, JB, Hubbard, SM, and Sonstegard, TS. Genomic selection in dairy cattle: the USDA experience. Annu Rev Anim Biosci. (2017) 5:309–27. doi: 10.1146/annurev-animal-021815-111422
3. Ma, Y, Khan, MZ, Xiao, J, Alugongo, GM, Chen, X, Chen, T, et al. Genetic markers associated with milk production traits in dairy cattle. Agriculture. (2021) 11:1018. doi: 10.3390/agriculture11101018
4. Taherkhani, L, Banabazi, MH, Emamjomeh-Kashan, N, Noshary, A, and Imumorin, I. The candidate chromosomal regions responsible for Milk yield of cow: a GWAS Meta-analysis. Animals. (2022) 12:582. doi: 10.3390/ani12050582
5. Iung, LHS, Petrini, J, Ramírez-Díaz, J, Salvian, M, Rovadoscki, GA, Pilonetto, F, et al. Genome-wide association study for milk production traits in a Brazilian Holstein population. J Dairy Sci. (2019) 102:5305–14. doi: 10.3168/jds.2018-14811
6. Atashi, H, Salavati, M, De Koster, J, Ehrlich, J, Crowe, M, Opsomer, G, et al. Genome-wide association for milk production and lactation curve parameters in Holstein dairy cows. J Anim Breed Genet. (2020) 137:292–304. doi: 10.1111/jbg.12442
7. Liu, L, Zhou, J, Chen, CJ, Zhang, J, Wen, W, Tian, J, et al. GWAS-based identification of new loci for milk yield, fat, and protein in Holstein cattle. Animals. (2020) 10:2048. doi: 10.3390/ani10112048
8. Wang, T, Li, J, Gao, X, Song, W, Chen, C, Yao, D, et al. Genome-wide association study of milk components in Chinese Holstein cows using single nucleotide polymorphism. Livest Sci. (2020) 233:103951. doi: 10.1016/j.livsci.2020.103951
9. Wang, P, Li, X, Zhu, Y, Wei, J, Zhang, C, Kong, Q, et al. Genome-wide association analysis of milk production, somatic cell score, and body conformation traits in Holstein cows. Front Vet Sci. (2022) 9:932034. doi: 10.3389/fvets.2022.932034
10. Su, M, Lin, X, Xiao, Z, She, Y, Deng, M, Liu, G, et al. Genome-wide association study of lactation traits in Chinese Holstein cows in southern China. Animals. (2023) 13:2545. doi: 10.3390/ani13152545
11. Meyer, K. WOMBAT: a tool for mixed model analyses in quantitative genetics by restricted maximum likelihood (REML). J Zhejiang Univ Sci B. (2007) 8:815–21. doi: 10.1631/jzus.2007.B0815
12. Stephen, D. Qqman: an R package for visualizing GWAS results using Q-Q and Manhattan plots. J Open Source Softw. (2018) 3:731. doi: 10.21105/joss.00731
13. Williams, CJ, Li, Z, Harvey, N, Lea, RA, Gurd, BJ, Bonafiglia, JT, et al. Genome wide association study of response to interval and continuous exercise training: the predict-HIIT study. J Biomed Sci. (2021) 28:37. doi: 10.1186/s12929-021-00733-7
14. Huang, DW, Sherman, BT, and Lempicki, RA. Systematic and integrative analysis of large gene lists using DAVID bioinformatics resources. Nat Protoc. (2009) 4:44–57. doi: 10.1038/nprot.2008.211
15. Sherman, BT, Hao, M, Qiu, J, Jiao, X, Baseler, MW, Lane, HC, et al. DAVID: a web server for functional enrichment analysis and functional annotation of gene lists (2021 update). Nucleic Acids Res. (2022) 50:W216–21. doi: 10.1093/nar/gkac194
16. Gustafsson, AH, Andersson, L, and Emanuelson, U. Effect of hyperketonaemia, feeding frequency and intake of concentrate and energy on milk yield in dairy cows. Anim Prod. (1993) 56:51–60. doi: 10.1017/S0003356100006152
17. Nayeri, S, Sargolzaei, M, Abo-Ismail, MK, May, N, Miller, SP, Schenkel, F, et al. Genome-wide association for milk production and female fertility traits in Canadian dairy Holstein cattle. BMC Genet. (2016) 17:75. doi: 10.1186/s12863-016-0386-1
18. Eiríksson, JH, Sigurdsson, Á, Jóhannesson, G, and Eythórsdóttir, E. Genetic parameters for Icelandic dairy cows using a random regression test-day model. Icel Agric Sci. (2019) 32:3–16. doi: 10.16886/IAS.2019.01
19. Zhou, J, Liu, L, Chen, CJ, Zhang, M, Lu, X, Zhang, Z, et al. Genome-wide association study of milk and reproductive traits in dual-purpose Xinjiang Brown cattle. BMC Genomics. (2019) 20:827. doi: 10.1186/s12864-019-6224-x
20. Stoop, WM, Van Arendonk, JAM, Heck, JML, Van Valenberg, HJF, and Bovenhuis, H. Genetic parameters for major milk fatty acids and milk production traits of dutch Holstein-Friesians. J Dairy Sci. (2008) 91:385–94. doi: 10.3168/jds.2007-0181
21. Stefani, G, El Faro, L, Santana Júnior, ML, and Tonhati, H. Association of longevity with type traits, milk yield and udder health in Holstein cows. Livest Sci. (2018) 218:1–7. doi: 10.1016/j.livsci.2018.10.007
22. Paiva, JT, Peixoto, MGCD, Bruneli, FAT, Alvarenga, AB, Oliveira, HR, Silva, AA, et al. Genetic parameters, genome-wide association and gene networks for milk and reproductive traits in Guzerá cattle. Livest Sci. (2020) 242:104273. doi: 10.1016/j.livsci.2020.104273
23. Ilie, DE, Mizeranschi, AE, Mihali, CV, Neamț, RI, Goilean, GV, Georgescu, OI, et al. Genome-wide association studies for milk somatic cell score in Romanian dairy cattle. Genes. (2021) 12:1495. doi: 10.3390/genes12101495
24. Schmidtmann, C, Segelke, D, Bennewitz, J, Tetens, J, and Thaller, G. Genetic analysis of production traits and body size measurements and their relationships with metabolic diseases in German Holstein cattle. J Dairy Sci. (2023) 106:421–38. doi: 10.3168/jds.2022-22363
25. Madsen, O. A comparison of some suggested measures of persistency of milk yield in dairy cows. Anim Prod. (1975) 20:191–7. doi: 10.1017/S0003356100035182
26. Tekerli, M, Akinci, Z, Dogan, I, and Akcan, A. Factors affecting the shape of lactation curves of Holstein cows from the Balikesir Province of Turkey. J Dairy Sci. (2000) 83:1381–6. doi: 10.3168/jds.S0022-0302(00)75006-5
27. Harder, I, Stamer, E, Junge, W, and Thaller, G. Lactation curves and model evaluation for feed intake and energy balance in dairy cows. J Dairy Sci. (2019) 102:7204–16. doi: 10.3168/jds.2018-15300
28. Pereira, MA, El, FL, Savegnago, RP, Costa, EV, Filho, AEV, and Faria, CU. Estimates of genetic parameters and cluster analysis of the lactation curve of dairy Gyr cattle. Livest Sci. (2021) 244:104337. doi: 10.1016/j.livsci.2020.104337
29. Price, AL, Patterson, NJ, Plenge, RM, Weinblatt, ME, Shadick, NA, and Reich, D. Principal components analysis corrects for stratification in genome-wide association studies. Nat Genet. (2006) 38:904–9. doi: 10.1038/ng1847
30. Martins, R, Machado, PC, Pinto, LFB, Silva, MR, Schenkel, FS, Brito, LF, et al. Genome-wide association study and pathway analysis for fat deposition traits in Nellore cattle raised in pasture–based systems. J Anim Breed Genet. (2021) 138:360–78. doi: 10.1111/jbg.12525
31. Marina, H, Pelayo, R, Suárez-Vega, A, Gutiérrez-Gil, B, Esteban-Blanco, C, and Arranz, JJ. Genome-wide association studies (GWAS) and post-GWAS analyses for technological traits in Assaf and Churra dairy breeds. J Dairy Sci. (2021) 104:11850–66. doi: 10.3168/jds.2021-20510
32. Massender, E, Oliveira, HR, Brito, LF, Maignel, L, Jafarikia, M, Baes, CF, et al. Genome-wide association study for milk production and conformation traits in Canadian alpine and Saanen dairy goats. J Dairy Sci. (2023) 106:1168–89. doi: 10.3168/jds.2022-22223
33. Persichilli, C, Senczuk, G, Mastrangelo, S, Marusi, M, van Kaam, JT, Finocchiaro, R, et al. Exploring genome-wide differentiation and signatures of selection in Italian and north American Holstein populations. J Dairy Sci. (2023) 106:5537–53. doi: 10.3168/jds.2022-22159
34. Kim, S, Cheong, HS, Shin, HD, Lee, SS, Roh, HJ, Jeon, DY, et al. Genetic diversity and divergence among Korean cattle breeds assessed using a BovineHD single-nucleotide polymorphism chip. Asian Australas J Anim Sci. (2018) 31:1691–9. doi: 10.5713/ajas.17.0419
35. Di Gerlando, R, Sutera, AM, Mastrangelo, S, Tolone, M, Portolano, B, Sottile, G, et al. Genome-wide association study between CNVs and milk production traits in Valle del Belice sheep. PLoS One. (2019) 14:e0215204. doi: 10.1371/journal.pone.0215204
36. Wang, X, Wang, L, Shi, L, Zhang, P, Li, Y, Li, M, et al. GWAS of reproductive traits in large white pigs on Chip and Imputed whole-genome sequencing data. Int J Mol Sci. (2022) 23:13338. doi: 10.3390/ijms232113338
37. Haque, MA, Alam, MZ, Iqbal, A, Lee, YM, Dang, CG, and Kim, JJ. Genome-wide association studies for body conformation traits in Korean Holstein population. Animals. (2023) 13:2964. doi: 10.3390/ani13182964
38. Wu, JJ, Song, LJ, Wu, FJ, Liang, XW, Yang, BZ, Wathes, DC, et al. Investigation of transferability of BovineSNP50 BeadChip from cattle to water buffalo for genome wide association study. Mol Biol Rep. (2013) 40:743–50. doi: 10.1007/s11033-012-1932-1
39. Gan, Q, Li, Y, Liu, Q, Lund, M, Su, G, and Liang, X. Genome-wide association studies for the concentrations of insulin, triiodothyronine, and thyroxine in Chinese Holstein cattle. Trop Anim Health Prod. (2020) 52:1655–60. doi: 10.1007/s11250-019-02170-z
40. Davis, SR, Gluckman, PD, Hart, IC, and Henderson, HV. Effects of injecting growth hormone or thyroxine on milk production and blood plasma concentrations of insulin-like growth factors I and II in dairy cows. J Endocrinol. (1987) 114:17–24. doi: 10.1677/joe.0.1140017
41. Venturini, GC, Cardoso, DF, Baldi, F, Freitas, AC, Aspilcueta-Borquis, RR, Santos, DJA, et al. Association between single-nucleotide polymorphisms and milk production traits in buffalo. Genet Mol Res. (2014) 13:10256–68. doi: 10.4238/2014.December.4.20
42. Liu, D, Xu, Z, Zhao, W, Wang, S, Li, T, Zhu, K, et al. Genetic parameters and genome-wide association for milk production traits and somatic cell score in different lactation stages of Shanghai Holstein population. Front Genet. (2022) 13:940650. doi: 10.3389/fgene.2022.940650
43. Zhao, YX, Gao, GX, Zhou, Y, Guo, CX, Li, B, El-Ashram, S, et al. Genome-wide association studies uncover genes associated with litter traits in the pig. Animal. (2022) 16:100672. doi: 10.1016/j.animal.2022.100672
44. Otto, PI, Guimarães, SEF, Calus, MPL, Vandenplas, J, Machado, MA, Panetto, JCC, et al. Single-step genome-wide association studies (GWAS) and post-GWAS analyses to identify genomic regions and candidate genes for milk yield in Brazilian Girolando cattle. J Dairy Sci. (2020) 103:10347–60. doi: 10.3168/jds.2019-17890
45. Dong, W, Yang, J, Zhang, Y, Liu, S, Ning, C, Ding, X, et al. Integrative analysis of genome-wide DNA methylation and gene expression profiles reveals important epigenetic genes related to milk production traits in dairy cattle. J Anim Breed Genet. (2021) 138:562–73. doi: 10.1111/jbg.12530
46. Buitenhuis, B, Janss, LLG, Poulsen, NA, Larsen, LB, Larsen, MK, and Sørensen, P. Genome-wide association and biological pathway analysis for milk-fat composition in Danish Holstein and Danish Jersey cattle. BMC Genomics. (2014) 15:1112. doi: 10.1186/1471-2164-15-1112
47. Moradian, H, Esmailizadeh Koshkoiyeh, A, Mohammadabadi, M, and Asadi, FM. Whole genome detection of recent selection signatures in Sarabi cattle: a unique Iranian taurine breed. Genes Genom. (2020) 42:203–15. doi: 10.1007/s13258-019-00888-6
48. Yodklaew, P, Koonawootrittriron, S, Elzo, MA, Suwanasopee, T, and Laodim, T. Genome-wide association study for lactation characteristics, milk yield and age at first calving in a Thai multibreed dairy cattle population. Agric Nat Resour. (2017) 51:223–30. doi: 10.1016/j.anres.2017.04.002
49. Velasco, SCH. Genetics of litter size and prenatal survival in pigs University of Edinburgh (2011). Scotland.
50. Alves, AAC, da Costa, RM, Fonseca, LFS, Carvalheiro, R, Ventura, RV, Rosa Gj De, M, et al. A random Forest-based genome-wide scan reveals fertility-related candidate genes and potential inter-chromosomal epistatic regions associated with age at first calving in Nellore cattle. Front Genet. (2022) 13:834724. doi: 10.3389/fgene.2022.834724
51. Kuhla, B, and Ingvartsen, KL. Proteomics and the characterization of fatty liver metabolism in early lactation dairy cows. In: A Almeidade, D Eckersall, and I Miller, editors. Proteomics in domestic animals: From farm to systems biology. Springer, Cham (2018). p. 219–231.
52. Antunes-Fernandes, EC, van Gastelen, S, Dijkstra, J, Hettinga, KA, and Vervoort, J. Milk metabolome relates enteric methane emission to milk synthesis and energy metabolism pathways. J Dairy Sci. (2016) 99:6251–62. doi: 10.3168/jds.2015-10248
53. Dijkstra, J, France, J, Assis, AG, Neal, SC, and LJM, A. Simulation of digestion in cattle fed sugarcane: prediction of nutrient supply for milk production with locally available supplements. J Agric Sci. (1996) 127:247–60. doi: 10.1017/S0021859600078023
54. Do, DN, Schenkel, FS, Miglior, F, Zhao, X, and Ibeagha-Awemu, EM. Genome wide association study identifies novel potential candidate genes for bovine milk cholesterol content. Sci Rep. (2018) 8:13239. doi: 10.1038/s41598-018-31427-0
55. Naserkheil, M, Bahrami, A, Lee, D, and Mehrban, H. Integrating single-step GWAS and bipartite networks reconstruction provides novel insights into yearling weight and carcass traits in Hanwoo beef cattle. Animals. (2020) 10:1836. doi: 10.3390/ani10101836
56. Liu, R, Sun, Y, Zhao, G, Wang, F, Wu, D, Zheng, M, et al. Genome-wide association study identifies loci and candidate genes for body composition and meat quality traits in Beijing-you chickens. PLoS One. (2013) 8:e61172. doi: 10.1371/journal.pone.0061172
57. Zeng, J, Cai, J, Wang, D, Liu, H, Sun, H, and Liu, J. Heat stress affects dairy cow health status through blood oxygen availability. J Anim Sci Biotechnol. (2023) 14:112. doi: 10.1186/s40104-023-00915-3
58. Pawliński, B, Gołębiewski, M, Trela, M, and Witkowska-Piłaszewicz, O. Comparison of blood gas parameters, ions, and glucose concentration in polish Holstein-Friesian dairy cows at different milk production levels. Sci Rep. (2023) 13:1414. doi: 10.1038/s41598-023-28644-7
59. Kirbas, A, Ozkanlar, Y, Aktas, OS, and Ulas, N. Acute phase biomarkers for inflammatory response in dairy cows with traumatic Reticuloperitonitis. Israel. J Vet Med. (2015) 70:23–30.
60. Fouz, R, Rodríguez-Bermúdez, R, Rodríguez-Godina, IJ, Rodríguez-Domínguez, M, Rico, M, and Diéguez, FJ. Evaluation of haptoglobin concentration in clinically healthy dairy cows: correlation between serum and milk levels. J Appl Anim Res. (2024) 52:2300624. doi: 10.1080/09712119.2023.2300624
61. Lemos, MVA, Chiaia, HLJ, Berton, MP, Feitosa, FLB, Aboujaoud, C, Camargo, GMF, et al. Genome-wide association between single nucleotide polymorphisms with beef fatty acid profile in Nellore cattle using the single step procedure. BMC Genomics. (2016) 17:213. doi: 10.1186/s12864-016-2511-y
62. Laodim, T, Elzo, MA, Koonawootrittriron, S, Suwanasopee, T, and Jattawa, D. Identification of SNP markers associated with milk and fat yields in multibreed dairy cattle using two genetic group structures. Livest Sci. (2017) 206:95–104. doi: 10.1016/j.livsci.2017.10.015
63. Cesarani, A, Corte Pause, F, Hidalgo, J, Garcia, A, Degano, L, Vicario, D, et al. Genetic background of semen parameters in Italian Simmental bulls. Ital J Anim Sci. (2023) 22:76–83. doi: 10.1080/1828051X.2022.2160665
64. Lázaro, SF, Tonhati, H, Oliveira, HR, Silva, AA, Scalez, DCB, Nascimento, AV, et al. Genetic parameters and genome-wide association studies for mozzarella and milk production traits, lactation length, and lactation persistency in Murrah buffaloes. J Dairy Sci. (2024) 107:992–1021. doi: 10.3168/jds.2023-23284
65. George, L, Alex, R, Sukhija, N, Jaglan, K, Vohra, V, Kumar, R, et al. Genetic improvement of economic traits in Murrah buffalo using significant SNPs from genome-wide association study. Trop Anim Health Prod. (2023) 55:199. doi: 10.1007/s11250-023-03606-3
66. Anton, I, Húth, B, Füller, I, Gábor, G, Holló, G, and Zsolnai, A. Effect of single-nucleotide polymorphisms on the breeding value of fertility and breeding value of beef in hungarian simmental cattle. Acta Vet Hung. (2018) 66:215–25. doi: 10.1556/004.2018.020
67. Lee, NY, Hum, M, Amali, AA, Lim, WK, Wong, M, Myint, MK, et al. Whole-exome sequencing of BRCA-negative breast cancer patients and case–control analyses identify variants associated with breast cancer susceptibility. Hum Genomics. (2022) 16:61. doi: 10.1186/s40246-022-00435-7
68. Liu, X, Wang, M, Qin, J, Liu, Y, Chai, Z, Peng, W, et al. Identification of candidate genes associated with yak body size using a genome-wide association study and multiple populations of information. Animals. (2023) 13:1470. doi: 10.3390/ani13091470
69. Teng, J, Wang, D, Zhao, C, Zhang, X, Chen, Z, Liu, J, et al. Longitudinal genome-wide association studies of milk production traits in Holstein cattle using whole-genome sequence data imputed from medium-density chip data. J Dairy Sci. (2023) 106:2535–50. doi: 10.3168/jds.2022-22277
70. Pedrosa, VB, Schenkel, FS, Chen, SY, Oliveira, HR, Casey, TM, Melka, MG, et al. Genomewide association analyses of lactation persistency and milk production traits in Holstein cattle based on imputed whole-genome sequence data. Genes. (2021) 12:1830. doi: 10.3390/genes12111830
71. Won, KH, Kim, D, Hwang, I, Lee, HK, and Oh, JD. Genome-wide association studies on collagen contents trait for meat quality in Hanwoo. J Anim Sci Technol. (2023) 65:311–23. doi: 10.5187/jast.2022.e110
72. Adhikari, M, Kantar, MB, Longman, RJ, Lee, CN, Oshiro, M, Caires, K, et al. Genome-wide association study for carcass weight in pasture-finished beef cattle in Hawai’i. Front Genet. (2023) 14:1168150. doi: 10.3389/fgene.2023.1168150
73. Makina, SO, Muchadeyi, FC, Van Marle-Köster, E, Taylor, JF, Makgahlela, ML, and Maiwashe, A. Genome-wide scan for selection signatures in six cattle breeds in South Africa. Genet Sel Evol. (2015) 47:92. doi: 10.1186/s12711-015-0173-x
74. Saravanan, KA, Panigrahi, M, Kumar, H, Parida, S, Bhushan, B, Gaur, GK, et al. Genomic scans for selection signatures revealed candidate genes for adaptation and production traits in a variety of cattle breeds. Genomics. (2021) 113:955–63. doi: 10.1016/j.ygeno.2021.02.009
75. Manca, E, Cesarani, A, Falchi, L, Atzori, AS, Gaspa, G, Rossoni, A, et al. Genome-wide association study for residual concentrate intake using different approaches in Italian Brown Swiss. Ital J Anim Sci. (2021) 20:1957–67. doi: 10.1080/1828051X.2021.1963864
76. Serão, NVL, González-Peña, D, Beever, JE, Faulkner, DB, Southey, BR, and Rodriguez-Zas, SL. Single nucleotide polymorphisms and haplotypes associated with feed efficiency in beef cattle. BMC Genet. (2013) 14:94. doi: 10.1186/1471-2156-14-94
77. Chen, Y, Gondro, C, Quinn, K, Herd, RM, Parnell, PF, and Vanselow, B. Global gene expression profiling reveals genes expressed differentially in cattle with high and low residual feed intake. Anim Genet. (2011) 42:475–90. doi: 10.1111/j.1365-2052.2011.02182.x
78. Cochran, SD, Cole, JB, Null, DJ, and Hansen, PJ. Discovery of single nucleotide polymorphisms in candidate genes associated with fertility and production traits in Holstein cattle. BMC Genet. (2013) 14:49. doi: 10.1186/1471-2156-14-49
79. Bauman, DE, and Bruce, CW. Partitioning of nutrients during pregnancy and lactation: a review of mechanisms involving homeostasis and Homeorhesis. J Dairy Sci. (1980) 63:1514–29. doi: 10.3168/jds.s0022-0302(80)83111-0
80. Slater, CJ, Endres, EL, Weaver, SR, Cheng, AA, Lauber, MR, Endres, SF, et al. Interaction of 5-hydroxy-L-tryptophan and negative dietary cation-anion difference on calcium homeostasis in multiparous peripartum dairy cows. J Dairy Sci. (2018) 101:5486–501. doi: 10.3168/jds.2017-13938
81. Rahmatalla, S, Arends, D, Tarekegn, G, Onzima, R, and Brockmann, G. Pairwise principal component analysis of African goat breeds identifies loci involved in meat and/or milk production. In: RF Veerkamp, de Haas, Y, editors. Proceedings of 12th world congress on genetics applied to livestock production (WCGALP) technical and species orientated innovations in animal breeding, and contribution of genetics to solving societal challenges ; 2022 Rotterdam, Netherland. Wageningen Academic Publishers (2022). p. 3012–3015.
82. Mohammadi, A, Alijani, S, Rafat, SA, and Abdollahi-Arpanahi, R. Single-step genome-wide association study and candidate genes networks affecting reproductive traits in Iranian Holstein cattle. Livest Sci. (2022) 262:104971. doi: 10.1016/j.livsci.2022.104971
83. Grarup, N, Rose, CS, Andersson, EA, Andersen, G, Nielsen, AL, Albrechtsen, A, et al. Studies of association of variants near the HHEX, CDKN2A/B, and IGF2BP2 genes with type 2 diabetes and impaired insulin release in 10,705 Danish subjects: validation and extension of genome-wide association studies. Diabetes. (2007) 56:3105–11. doi: 10.2337/db07-0856
84. Pivovarova, O, Nikiforova, VJ, Pfeiffer, AFH, and Rudovich, N. The influence of genetic variations in HHEX gene on insulin metabolism in the German MESYBEPO cohort. Diabetes Metab Res Rev. (2009) 25:156–62. doi: 10.1002/dmrr.926
85. Do, DN, Dudemaine, PL, Li, R, and Ibeagha-Awemu, EM. Co-expression network and pathway analyses reveal important modules of miRNAs regulating milk yield and component traits. Int J Mol Sci. (2017) 18:1560. doi: 10.3390/ijms18071560
86. Schmidt, GH. Effect of insulin on yield and composition of Milk of dairy cows. J Dairy Sci. (1966) 49:381–5. doi: 10.3168/jds.S0022-0302(66)87878-5
87. Puppin, C, Puglisi, F, Pellizzari, L, Manfioletti, G, Pestrin, M, Pandolfi, M, et al. Hex expression and localization in normal mammary gland and breast carcinoma. BMC Cancer. (2006) 6:192. doi: 10.1186/1471-2407-6-192
88. Wang, X, Xu, J, and Han, Z. Plasma proteomic profiling reveals the regulatory factors of Milk protein synthesis in Holstein cows. Biology. (2022) 11:1239. doi: 10.3390/biology11081239
Keywords: GWAS, candidate genes, milk production, persistency, Holstein heifers, cattle
Citation: Erdoğan M, Çinkaya S, Brenig B, Çelikeloğlu K, Demirtaş M, Sarıibrahimoğlu S and Tekerli M (2024) Genome-wide association studies for milk production traits and persistency of first calving Holstein cattle in Türkiye. Front. Vet. Sci. 11:1461075. doi: 10.3389/fvets.2024.1461075
Received: 07 July 2024; Accepted: 02 September 2024;
Published: 24 October 2024.
Edited by:
Yulin Bai, Chinese Academy of Fishery Sciences (CAFS), ChinaReviewed by:
Zhuying Wei, University of Michigan, United StatesCopyright © 2024 Erdoğan, Çinkaya, Brenig, Çelikeloğlu, Demirtaş, Sarıibrahimoğlu and Tekerli. This is an open-access article distributed under the terms of the Creative Commons Attribution License (CC BY). The use, distribution or reproduction in other forums is permitted, provided the original author(s) and the copyright owner(s) are credited and that the original publication in this journal is cited, in accordance with accepted academic practice. No use, distribution or reproduction is permitted which does not comply with these terms.
*Correspondence: Samet Çinkaya, c2NpbmtheWFAYWt1LmVkdS50cg==,; c2FtZXRjaW5rYXlhNDhAZ21haWwuY29t
†Senior authorship
Disclaimer: All claims expressed in this article are solely those of the authors and do not necessarily represent those of their affiliated organizations, or those of the publisher, the editors and the reviewers. Any product that may be evaluated in this article or claim that may be made by its manufacturer is not guaranteed or endorsed by the publisher.
Research integrity at Frontiers
Learn more about the work of our research integrity team to safeguard the quality of each article we publish.