- 1Laboratory of Veterinary Bacteriology, Department of Animal Infectious Diseases, Sciensano, Brussels, Belgium
- 2Laboratory of Biochemistry and Genetics of Microorganisms, Louvain Institute of Biomolecular Science and Technology, Université Catholique de Louvain, Louvain-la-Neuve, Belgium
- 3Transversal Activities in Applied Genomics, Sciensano, Brussels, Belgium
- 4VISAVET Health Surveillance Centre, Complutense University of Madrid, Madrid, Spain
- 5Experimental Center Machelen, Sciensano, Machelen, Belgium
In recent years, there has been an increase in the number of reported cases of Mycobacterium microti infection in various animals, which can interfere with the ante-mortem diagnosis of animal tuberculosis caused by Mycobacterium bovis. In this study, whole genome sequencing (WGS) was used to search for protein-coding genes to distinguish M. microti from M. bovis. In addition, the population structure of the available M. microti genomic WGS datasets is described, including three novel Belgian isolates from infections in alpacas. Candidate genes were identified by examining the presence of the regions of difference and by a pan-genome analysis of the available WGS data. A total of 80 genes showed presence-absence variation between the two species, including genes encoding Proline-Glutamate (PE), Proline-Proline-Glutamate (PPE), and Polymorphic GC-Rich Sequence (PE-PGRS) proteins involved in virulence and host interaction. Filtering based on predicted subcellular localization, sequence homology and predicted antigenicity resulted in 28 proteins out of 80 that were predicted to be potential antigens. As synthetic peptides are less costly and variable than recombinant proteins, an in silico approach was performed to identify linear and discontinuous B-cell epitopes in the selected proteins. From the 28 proteins, 157 B-cell epitope-based peptides were identified that discriminated between M. bovis and M. microti species. Although confirmation by in vitro testing is still required, these candidate synthetic peptides containing B-cell epitopes could potentially be used in serological tests to differentiate cases of M. bovis from M. microti infection, thus reducing misdiagnosis in animal tuberculosis surveillance.
Introduction
Animal tuberculosis (TB) is a chronic infectious disease caused by members of the Mycobacterium tuberculosis complex (MTBC), whose natural hosts are wild and domestic mammals (1). Mycobacterium bovis is the primary cause of TB in cattle (bovine TB, bTB) and is capable of infecting a wide range of other animal species as well as humans (2). This zoonotic pathogen therefore poses a high risk to animal and human health and to international trade in domestic animals and animal products, highlighting the need for surveillance.
The first reported case of M. bovis infection on an alpaca farm in Belgium occurred in 2015 (personal communication from M. Mori, Sciensano, Belgium), increasing the interest in ante-mortem diagnostics in New World Camelids (NWCs), such as llamas and alpacas. Diagnostic tests for animal TB detection based on cell-mediated immunity (CMI), such as the intradermal tuberculin test or the IFNɤ assay, have been reported to have poor accuracy in NWCs (3, 4). Serological diagnostic assays may be a promising alternative, as shown by (5). Over the last decade, several serological assays have been developed to detect animal TB in camelids, such as the ELISA INgezim Tuberculosis DR multispecies test (Ingenasa, Spain) and the multiplexed Enferplex Camelids TB test (Enfer Scientific, Ireland) (6, 7).
In 2020, alpacas from two different Belgian farms, one of which was involved in the 2015 animal TB outbreak, were suspected of being infected with M. bovis based on positive serological diagnostic results (INgezim Tuberculosis DR multispecies and Enferplex Camelids TB). Necropsy of these animals revealed lesions typical of tuberculosis, and the real-time PCR (qPCR), performed on the organs and using the IS6110 insertion sequence for identifying MTBC members, was positive. However, the qPCR test to differentiate the MTBC members, based on polymorphisms of the gyrB gene and performed from bacterial culture (8), identified M. microti (data from the National Reference Laboratory for Bovine Tuberculosis, Sciensano, Belgium).
First identified in voles, M. microti is another, less virulent, member of MTBC that can infect a wide range of animal species and cause tuberculous lesions (9–11). Furthermore, the impact of the epidemiological situation of M. microti in some areas does not seem to be irrelevant (11–13). However, the European Animal Health Law (EU 2016/429) does not include M. microti as an etiological agent of animal TB, which according to the legislation only includes M. bovis, M. caprae and M. tuberculosis. Consequently, M. microti infections are non-notifiable and do not lead to the same sanitary measures (e.g., removed animals, blocked farms) as M. bovis or M. caprae outbreak declarations. M. microti, therefore, represents a risk of interference in the animal TB diagnosis.
Research into biomarkers to accurately distinguish M. bovis from M. microti infection at ante-mortem examination in animals has received limited attention. While the serological tests used to diagnose animal TB in NWCs and other species, such as cattle, are effective in detecting infected animals, they are not specific enough to distinguish between M. bovis and M. microti infection (14–16). It is therefore important to identify specific antigenic proteins that can elicit an antibody response and that can be used in serological tests. B-cell epitopes are segments of antigenic proteins that can be recognized by antibodies (17), and are categorized into linear (i.e., continuous amino acid sequences) and conformational (i.e., folded three-dimensional structures) epitopes. Of particular interest is the identification of specific B-cell epitopes that could be used to construct synthetic peptides as biomarkers.
Members of the MTBC have over 99.9% DNA sequence identity to each other, but they differ in phenotype, virulence and host species. Differences between species are mostly limited to deletions of regions of difference (RDs), single nucleotide polymorphisms (SNPs) in protein-coding genes and hypervariable regions. Molecular typing of MTBC members is often performed using spoligotyping, a polymerase chain reaction-based method that examines the deletion of spacer sequences in the Direct Repeat (DR) region (18). The resulting spoligotype profiles can be used to understand the genetic diversity and relatedness of MTBC strains. Diverse spoligotypes were reported for M. microti strains (11) among them the more dominant are the “llama-type” and “vole-type” spoligotypes, named after the host animals in which they were first observed (19). Studies have shown that M. microti strains are characterized by M. microti-specific deletion regions (MiD) together with the deletion of part of the RD1 region (RD1mic), which includes open reading frames (ORF) of known virulence genes (20). These genomic variations may be useful for the epidemiological surveillance and diagnosis of animal TB (21–23). The genomic organization of members of the MTBC, mainly M. bovis and M. tuberculosis, has been further explored using whole-genome sequencing (WGS), allowing a better understanding of the pathology and the identification of biomarkers for the diagnosis and management of TB (24–27). However, to date, there has been very little research on M. microti, and very few WGS datasets are currently available in public databases.
This study was initiated to identify potential biomarkers for distinguishing M. microti from M. bovis using WGS. The genomic information was used to screen for variations affecting proteins that could form the basis of serological diagnostic methods. An in silico approach was used to extract B-cell epitopes from the identified putative antigenic proteins. In addition, this study describes the genomic diversity of M. microti based on publicly available data, including the recently collected strains from Belgium.
Materials and methods
Bacterial strains and DNA extraction
Three M. microti strains were isolated from the organs of alpacas from Belgian farms. Two were isolated on the same farm from animals with a positive result in serological tests for animal TB (i.e., isolates MI20-1 and MI20-2). Both alpacas showed tuberculous lesions at necropsy. The third isolate was collected from an alpaca that was found dead (with tuberculous lesions) and originating from another farm (i.e., isolate VAR696). There was no apparent geographical or epidemiological link between the two farms. All samples were collected in 2020.
M. microti strains MI20-1 and MI20-2 were grown either on a solid stone-brink medium or using a BACTEC™ MGIT™ automated liquid system (Becton Dickinson, Sparks, MD, USA) at the European Reference Laboratory for bovine tuberculosis at the VISAVET Health Center (Madrid, Spain) and incubated at 37°C for several weeks. M. microti VAR696 was processed in a similar manner at the Belgian Reference Laboratory for bovine tuberculosis at the Belgian Institute of Health (Sciensano, Brussels, Belgium) and genomic DNA was extracted using the QIAGEN DNeasy Blood and Tissue kit (Qiagen, Hilden, Germany) according to the manufacturer's instructions for Gram-positive bacteria with a prior mechanical grinding step to meet the specific requirements for DNA extraction in the case of Mycobacteria spp. Genomic DNA of strains MI20-1 and MI20-2 was extracted from a pure culture grown in Middlebrook 7H9 medium supplemented with oleic albumin dextrose catalase (OADC) using phenol-chloroform-isoamyl alcohol (PCI) and was then purified using ethanol, as previously described by (28). The resulting DNA pellet was dried at room temperature and re-suspended in 50–200 μL of ultrapure distilled water (Sigma-Aldrich, St. Louis, MO, USA).
DNA quality and concentration were measured by spectrophotometry using the Nanodrop® 2000 (Thermo Fisher Scientific, Waltham, MA, USA) and DNA purity was assessed using the A260/A280 and A260/A230 ratios.
Detection of members of the MTBC was performed by a qPCR based on the IS6110 element (29). The molecular characterization of MTBC mycobacteria was performed using a qPCR based on polymorphism of the gyrB gene (8) and/or direct variable repeat (DVR)-spoligotyping (30).
Whole-genome sequencing
The DNA extracts from isolates MI20-1 and MI20-2 were sent to the National Veterinary Services Laboratory in Ames, Iowa (USA) for library preparation and whole genome sequencing, whereas the VAR696 isolate was sequenced at Sciensano (Belgium). Library preparation was achieved using the Nextera XT library preparation kit (Illumina, San Diego, CA, USA) according to manufacturer's instructions. Sequencing was carried out in both cases on a MiSeq system with the V3 chemistry, obtaining 250 bp paired-end reads, aiming for a theoretical coverage of 60X based on the expected genome size of ~ 4.4 Mbp of M. microti.
Pre-processing and de novo assembly
Pre-processing and de novo assembly were performed as previously described (31). Briefly, raw reads were trimmed using Trimmomatic 0.38 (32) with the following options: “LEADING” set to 10, “TRAILING” set to 10, “SLIDINGWINDOW” set to “4:20,” “MINLEN” set to 40, and “ILLUMINACLIP” set to “NexteraPE-PE.fa:2:30:10.” Processed reads were then assembled de novo using SPAdes 3.13.0 (33) with the “—careful” option enabled and “–cov-cutoff” set to 10. Contigs smaller than 1,000 bp were removed using the “seq” function of Seqtk 1.3 (available at https://github.com/lh3/seqtk). The quality of the assemblies was evaluated using QUAST 4.4 (34), with the filtered assemblies as input. Several additional quality checks included in the workflow were also evaluated: (1) screening for contaminants using Kraken 2 v2.0.7 (35); (2) evaluation of median sequencing depth and mapping rate using Bowtie 2 v2.4.1 (36) and SAMtools v1.9 (37); and (3) various quality checks on the input reads with FastQC v0.11.7 (https://www.bioinformatics.babraham.ac.uk/projects/fastqc/). Figure 1 illustrates the flow of the full bioinformatics analysis.
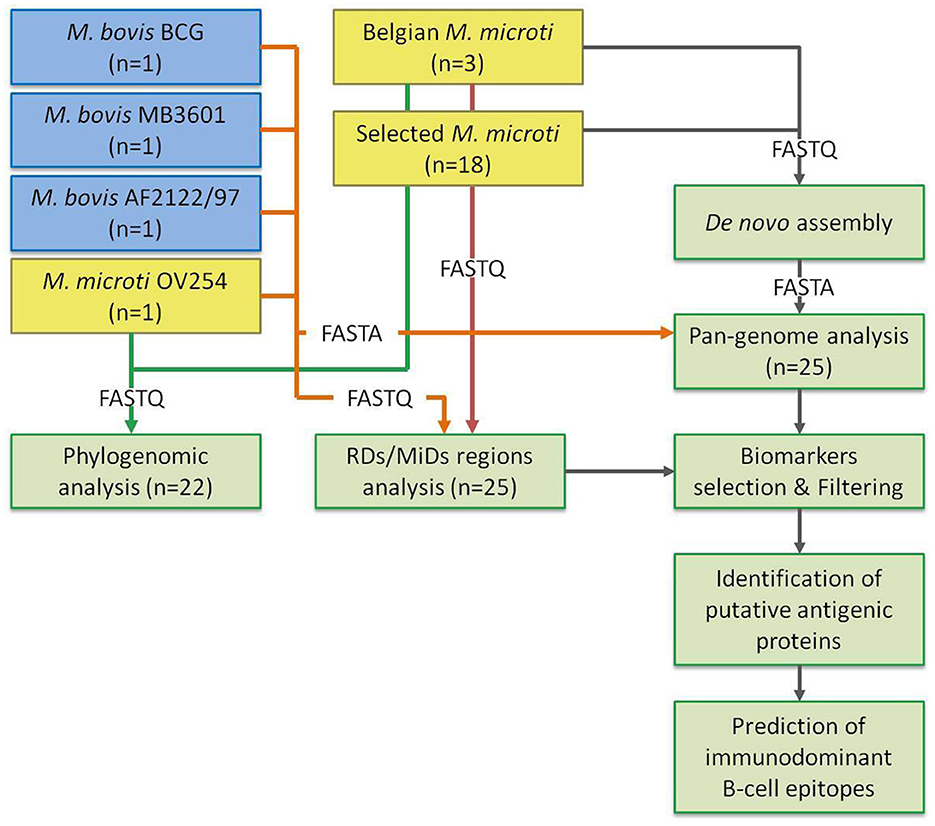
Figure 1. Overview of the bioinformatics processing. Overview of the study design. M. bovis and M. microti data are shown in blue and yellow boxes, respectively. The number of strains is indicated. The parts of the analysis are shown in green boxes. The arrows indicate the flow of the data, with the format indicated by the label. The green arrow shows the input FASTQ files for the phylogenomic analysis. The orange arrow indicates the data for the selected reference genomes: (1) the FASTQ files are used for the RD/MiD analysis, (2) the genome FASTA files are used as input for the pan-genome analysis. The red arrow indicates the FASTQ input of the Belgian and selected M. microti strains for the RD/MiD analysis. The gray arrow indicates the flow of data between the sub-analyses.
Phylogenomic analysis
All available M. microti datasets in the Sequence Read Archive (SRA) at the National Center for Biotechnology Information (NCBI) that were generated by Illumina sequencing were retrieved (accessed on the 24th of January 2022), for which an overview is provided in Table 1. Three datasets were available for isolate 8753/00, of which ERR551113 was selected because it had the highest coverage. The ERR553377 dataset was also excluded from the analysis as it clustered identically in the SNP analysis with ERR553376, which was collected in the same study. Similarly, the ERR2659165 dataset was excluded from analysis as it is a replicate of the ERR2659166 dataset. Datasets with an estimated coverage >100x, calculated based on the number of bases in the FASTQ files and the genome size of the M. microti OV254 genome (RefSeq accession GCF_904810325.1), were downsampled to ~100x using the “sample” function of seqtk 1.3. The SNP phylogeny was then performed using PACU 0.0.5 (44), which uses BCFtools 1.17 (45) for variant calling and Gubbins 3.3.1 (46) to remove SNPs in recombinant regions. First, the processed reads were mapped to the M. microti OV254 genome (RefSeq accession GCF_904810325.1), using the PACU_map function with the “–read-type” parameter set to “illumine” and other options left at their default values. The resulting BAM files were used as input for the PACU SNP workflow. The online PHASTER tool (47) was used to generate a BED file with (pro-) phage regions in the reference genome and provided to PACU with the “–ref-bed” option. The –use-mega' option was enabled to perform automatic model selection and tree building with MEGA X (48). The best-fit model was selected as the substitution model with the lowest Bayesian information criterion (BIC) value, selecting the Tamura 3-parameter model. Spoligotypes were determined in silico using SpoTyping 2.1 (49) and added as annotations to the phylogeny. The “—min” and “—rmin” parameter values were set to 1 for datasets with an estimated coverage of < 30x, estimated based on the mapping to the reference genome. For other datasets, the default parameter values were used.
Genomic characterization of RDs and MiDs regions
The presence of RDs and MiDs was assessed using a read mapping-based approach. The RD and MiD nomenclature used in this study is based on that of (20, 21, 50–52) and differs from that proposed by (53). An overview of the evaluated RDs and MiDs is provided in Table 2. Bowtie2 2.4.1 was used with the “–very-sensitive-local” preset enabled to map the processed reads to the sequences of the RD regions, obtained from the M. tuberculosis H37Rv reference genome (RefSeq accession NC_000962.3). The analysis included the Illumina FASTQ datasets for the M. bovis BCG Danish 1311 (SRR7983756), M. bovis AF2122/97 (ERR1744454), M. bovis MB3601 (ERR3825346), and M. microti OV254 (ERR027295) reference genomes and all M. microti strains included in the phylogenomic analysis. Regions that were covered for at least 75% and with a median depth >5x were considered to be present.
Pan-genome analysis
Roary was used for the pan-genomic analysis. Three M. bovis reference strains (i.e., the M. bovis BCG vaccine strain and the two virulent M. bovis strains AF2122/97 and MB3601) and one M. microti reference strain (OV254) were used, for which the circular genomes were downloaded from NCBI RefSeq. In addition, the assembled genomes of the M. microti strains used in the phylogenomic analysis were also used, including those of the three Belgian strains. Prokka 1.14.6 (54) was used with default options for gene prediction and functional annotation. The proteins from the M. tuberculosis H37Rv, M. microti OV254, M. bovis AF2122/97, and M. bovis BCG Danish 1311 reference genomes were provided with the “—proteins” options as a basis for gene annotation. Roary 3.12.0 (55) was then used for the pan-genome analysis with the “—mafft” option enabled, minimum identity set to 90%, and paralogs not split (“-s”). UpSet plots were generated using the UpSetPlot python package v0.8.0 (available at https://github.com/jnothman/UpSetPlot) to show the overlap of genes between species and strains. The results of the pan genome analysis were used to identify protein-encoding genes that could be used as potential biomarkers for the differentiation of M. microti from virulent M. bovis (i.e., excluding the M. bovis Danish 1311 BCG vaccine strain).
Selection of potential biomarkers
Proteins, from the RD/MiDs and pan-genome analyses, present in the virulent M. bovis strains (i.e., not the M. bovis BCG vaccine strain) and absent in all M. microti strains, and vice-versa, were selected as potential biomarkers. They were then evaluated according to the following three criteria: subcellular localization, sequence homology and antigenicity, as elaborated below.
Prediction of subcellular localization
SignalP 6.0 was used to predict the presence of sec- and tat-dependent signal peptides in proteins (56). These short N-terminal amino acid sequences are recognized by the classical sec or tat translocation machinery and thus control protein secretion and translocation in bacteria. SecretomeP 2.0 was used for the prediction of non-classical secreted proteins (57). Both programs are freely available on the website of the Center for Biological Sequence Analysis at the Technical University of Denmark (http://www.cbs.dtu.dk/services). In addition, Psortb v3.0.3 [(58); https://www.psort.org/psortb/], Gpos-mPLoc [(59); http://www.csbio.sjtu.edu.cn/bioinf/Gpos-multi/] and CELLO2.5 [(60); http://cello.life.nctu.edu.tw/] were used for predictions of the subcellular localization of the candidate proteins. CELLO2.5 is a multi-class Support vector machine (SVM) classification system using four types of sequence coding schemes. Psortb3.0.3 uses a combination of modules, each of which is known to influence subcellular location of the proteins (e.g., the number of transmembrane helices, signal peptides, outermembrane motifs, ...). Gpos-mPLoc identifies subcellular localization of Gram positive bacterial proteins by fusing the information of gene ontology, as well as the functional domain information and sequential evolution information. Amino acid sequences were retrieved from the NCBI protein database and used as input for all tools. The UniProt database (http://www.uniprot.org/) was also queried to obtain the subcellular localization of the proteins.
Sequence homology analysis
Putative biomarkers with high sequence similarity to proteins from members of the Mycobacterium avium-intracellulare complex (MAC; M. avium subspecies paratuberculosis, “M. avium subspecies hominissuis,” M. avium subspecies avium, and M. intracellulare) were removed. These environmental mycobacteria are commonly isolated from cattle and share proteins with members of MTBC that can cross-react in diagnosis (61–63). Sequence similarities of all proteins were evaluated using the non-redundant protein sequence database of the NCBI BLASTp tool (https://blast.ncbi.nlm.nih.gov/). Putative proteins with percent identity ≥55% and query coverage ≥40% to those in the NCBI database were discarded.
Prediction of antigenicity
The antigenicity of all proteins was estimated using ANTIGENpro (http://scratch.proteomics.ics.uci.edu/) and VaxiJen v2.0 (https://www.ddg-pharmfac.net/vaxijen/VaxiJen/VaxiJen.html). The predictions from ANTIGENpro are based on a two-step approach, combining multiple representations of the primary sequence and machine learning algorithms. A final support vector machine (SVM) classifier summarizes the resulting predictions and provides a probability of whether the protein is likely to be antigenic (64). VaxiJen 2.0 is an alignment-free approach for antigen prediction, which is based on auto cross covariance transformation of protein sequences into uniform vectors of key amino acid properties (65). A prediction threshold of 0.5 was used for both programs, as recommended in literature (64, 65). Proteins predicted to be antigenic by at least one of these two tools were considered likely to be antigenic.
Prediction of linear and conformational B-cell epitopes
The prediction of linear and conformational B-cell epitopes was performed for all proteins predicted as extracellular, low conserved in environmental mycobacteria and antigenic.
For the prediction of linear B-cell epitopes, protein sequences in FASTA format were submitted to five different tools available in the Epitope Database and Analysis Resource (IEDB; https://www.iedb.org/), based on the following scales: beta turn (66), surface accessibility (67), flexibility (68), hydrophilicity (69), and antigenicity (70). For each tool, the window size was set to seven amino acids and the default threshold was used. BepiPred-2.0, available on the IEDB server, was also used to predict linear B-cell epitopes from amino acid sequences in FASTA format. The Bepipred 2.0 tool is based on a random forest algorithm trained on epitope and non-epitope amino acids as determined from crystal structures. The default threshold value of 0.50 was used (71). All results (i.e., all linear B-cell epitopes predicted) obtained with the IEDB tools were compared to find similar epitopes using the Epitope Cluster Analysis v2.0 tool (http://tools.iedb.org/cluster/), with a minimum sequence identity threshold of 70%. The consensus epitopes obtained with the Epitope Cluster tool were selected as the final linear B-cell epitopes. Prediction of linear B-cell epitopes via multiple tools and peptides clustering was limited for protein sequences with a high number of amino acids (>1,500), meanly PE_PGRS50 (Rv3345c) and PPE55 (Rv3347c). Consequently, only antigenic linear B-cell epitopes predicted by the Kolaskar and Tongaonkar method were used for these proteins.
As most of B-cell Epitopes in native proteins are conformational (72), the conformational B-cell epitopes were predicted by using the Discotope 3.0 (https://services.healthtech.dtu.dk/services/DiscoTope-3.0/) and ElliPro http://tools.iedb.org/ellipro/; (73) servers. Proteins structures were downloaded from the PDB database (https://www.rcsb.org/) or the AlphaFold protein structure database [https://alphafold.ebi.ac.uk/; (74)]. The default parameters were used. Amino acid sequences, ranging from 8 to 25 residues, that were common to the predicted B-cell linear and conformational epitopes, were retained. The antigenicity of B-cell Epitopes predicted as linear and conformational was analyzed with Vaxijen2.0. The epitopes with a value >0.5 were considered as the best B-cell epitope peptides.
Data availability
The datasets supporting the conclusions of this study have been deposited in the NCBI SRA under accession number PRJNA1000766. Individual accession numbers are provided in Table 1.
Results
Pre-processing and data quality
Data quality metrics for the de novo assembly and read mapping analysis of the in-house generated datasets (i.e., the Belgian M. microti isolates) are provided in Supplementary Table 1. All three datasets passed the quality checks of the workflow (31), indicating that the data were suitable for further analysis.
Phylogenomic analysis of available M. microti genomes
Of the 27 M. microti datasets collected from NCBI, 19 were included in the phylogenomic analysis, with eight excluded due to low quality. These data were supplemented by the three M. microti strains sequenced in this study, resulting in a total of 22 M. microti strains (Table 1). The output of the maximum likelihood analysis is shown in Figure 2 and pairwise SNP distances are shown in Supplementary Table 2. The isolates collected in this study show very high genomic similarity, with only eight SNP differences between MI20-1 and MI20-2, and 18 SNPs between these two isolates and VAR696. However, a direct epidemiological link between these strains seems unlikely given the low mutation rate of MTBC (75). The closest strain collected from SRA was M. microti-45, isolated from a pig in the United Kingdom (UK), which differed by 57 SNPs from all three Belgian strains in this study. The M. microti-36 strain, isolated from a domestic cat in the UK, showed 241–243 SNP differences with the three Belgian strains and 244 SNP differences with M. microti-45. While the bootstrap values for the longer branches were generally high, the bootstrap values also indicated low confidence in the relative position of these branches.
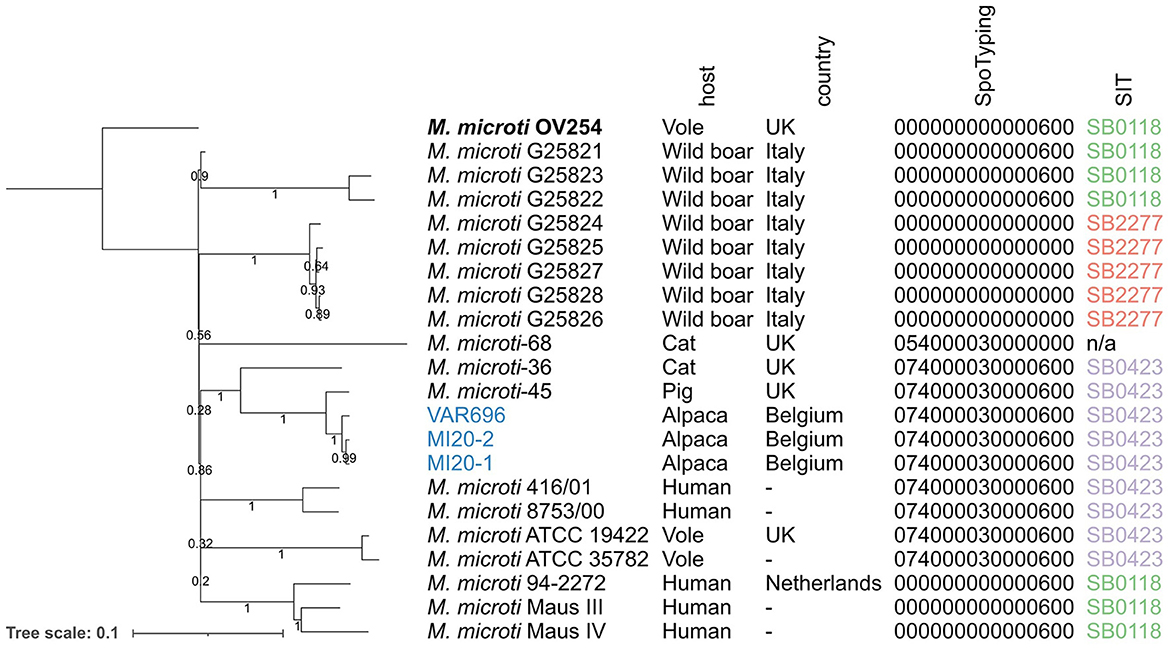
Figure 2. Maximum likelihood phylogeny of the M. microti strains collected from NCBI and the isolates collected in this study. Branch lengths and the scale bar are expressed as average substitutions per site. Bootstrap support is indicated by the numbers on the branches (proportions based on 1,000 replicates). M. microti OV254 was used as the reference genome for the phylogeny (highlighted in bold). The three isolates collected as part of this study are shown in blue. Annotations are (from left to right): strain name, host species, country, spoligotype determined by SpoTyping in octal format, and SIT number. The phylogeny was mid-point rooted. UK, United Kingdom; n/a, not available; SIT, Spoligotype International Type.
The three Belgian strains were assigned to the same spoligotype as the M. microti-45 and M. microti-36 strains in the same clade (the “llama-type” spoligotype SB0423). The same spoligotype was observed in two other clades: (1) the clade carrying M. microti ATCC 19422 and ATCC35782, collected from voles, and (2) the clade carrying M. microti 416/01 and 8753/00, both isolated from humans. The M. microti-68 strain had a similar spacer pattern, but lacked spacers 37 and 38, resulting in a different spoligotype. All other isolates were assigned to SB0118, known as the “vole-type” spoligotype, except for the clade containing five isolates collected from wild boars in Italy, which lacked all spacers, assigning them to SB2277.
Genomic characterization of RDs and MiDs regions
Twenty out of the 40 RDs/MiDs regions analyzed were present in the three Belgian and the OV254 reference M. microti strains along with in the three M. bovis reference strains studied, as shown in Figure 3. The RD7, RD8, RD9, and RD10 regions were (mostly) absent in all strains, except for M. tuberculosis H37Rv. The RD4, RD6, RD11 (prophage phiRv2), RD12bov, RD13, and N-RD25bov/cap regions were absent in all M. bovis strains (virulent and vaccine strains), but present in the four M. microti strains. However, ~60% (below the detection threshold of 75%) of the N-RD25bov/cap region was present in the M. bovis strains, with the PPE66 (Rv3738c) ORF interrupted and the PPE67 (Rv3739c) locus completely absent in these strains. No intact ORFs were found in the fractions (< 25% coverage) of the RD4, RD6, RD11, and RD13 regions that were present in the M. bovis strains. Similarly, no intact ORFs were found for the fraction of the RD12bov region (~36% coverage) in the M. bovis strains, except for the Rv3117 locus. Interestingly, the RD5mic region was largely absent from the M. bovis and M. microti OV254 genomes, but was almost completely covered in the three Belgian M. microti strains. In contrast to M. microti OV254, the plcC (Rv2349c), plcB (Rv2350c), plcA (Rv2351c), and PPE38 (Rv2352c) loci were completely covered in the three Belgian strains. The MiD3 region was present in all M. bovis strains under study, but was below the detection threshold in all M. microti strains. However, the M. microti strains contained a large fraction of the MiD3 region, but no intact ORFs for PE_PGRS50 (Rv3345c), a transmembrane protein (Rv3346c), PPE55 (Rv3347c), and a transposase (Rv3349c). Only the transposase Rv3348c had an intact ORF. The RD1mic, RD1bcg, RD3, and RD149 regions were present in M. bovis AF2122/97 and M. bovis MB3601, but were absent in all other strains (M. bovis BCG and M. microti strains). The beginning of the RD1bcg region (Rv3871-Rv3879c) was missing in the M. microti strains, as was most of the RD1mic region (Rv3864-Rv3876). For the RD3 region (prophage phiRv1; Rv1573-Rv1586c), which partially overlaps with the RD149 region (Rv1585c-Rv1587c), only the Rv1587c ORF was intact in M. bovis BCG and the M. microti strains. The RD2 region was present in all strains, except for M. bovis BCG Danish 1311. Lastly, the partially overlapping RD207 and MiD1 regions were present in all MTBC strains except for M. microti OV254, resulting in missing or interrupted CRISPR-Cas loci cas2 (Rv2816c), cas1 (Rv2817c), csm6 (Rv2818c), and csm5 (Rv2819c). Similarly, the MiD2 region (Rv3188-Rv3189) was present in all analyzed MTBC strains, except for M. microti OV254.
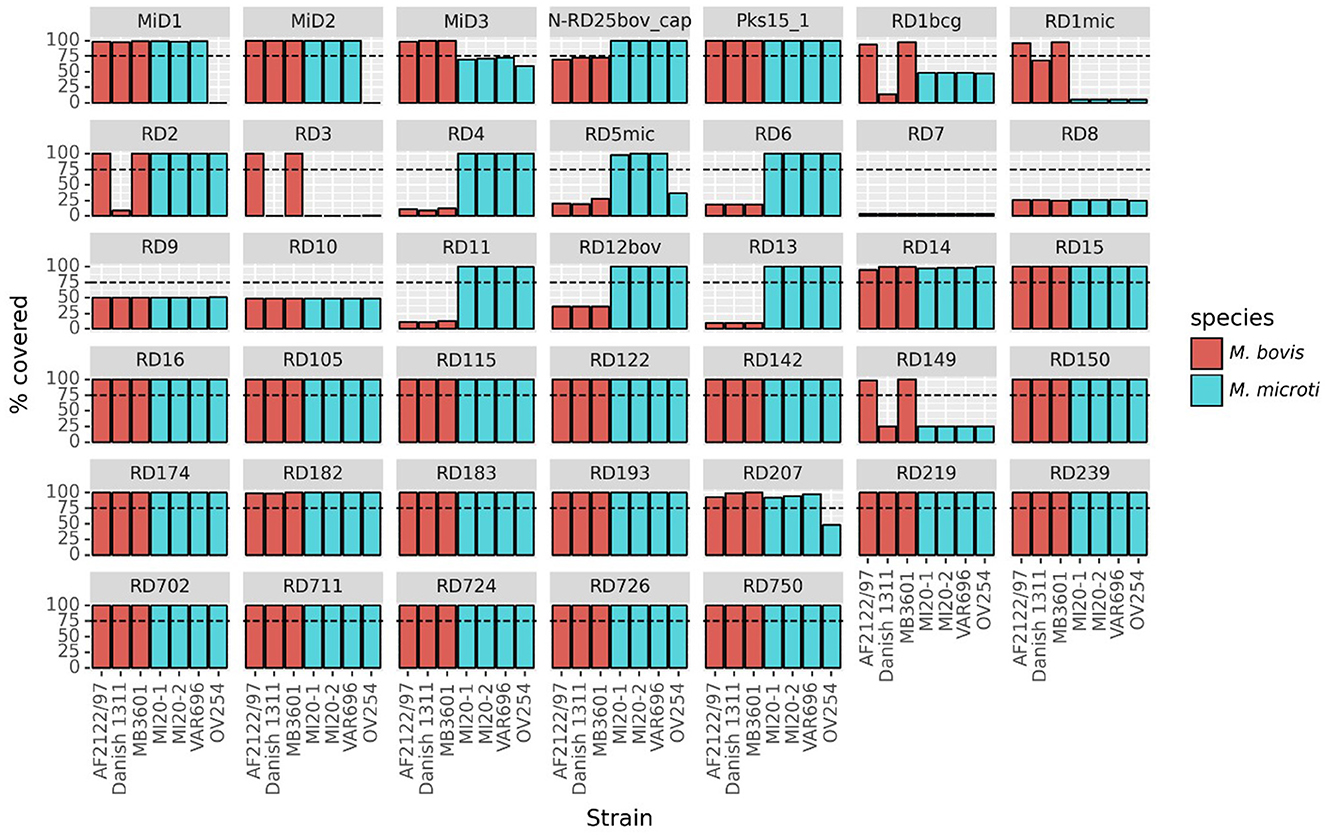
Figure 3. Presence of RDs and MiDs regions in the Belgian M. microti datasets and the selected reference genomes. This graph shows the coverage percentage of the regions of difference (RDs) and M. microti-specific deleted regions (MiDs). The y-axis indicates the percentage of the region that was covered by reads, the x-axis indicates the different strains. The bars are colored by species as indicated in the legend. The dashed line indicates the 75% threshold that was used to classify regions as present or absent. The following reference and Belgian strains were included: M. bovis BCG Danish 1311 (SRR7983756), M. bovis AF2122/97 (ERR1744454), M. bovis MB3601 (ERR3825346), M. microti OV254 (ERR027295), M. microti VAR696 (SRR25473322), M. microti MI20-1 (SRR25473321), and M. microti MI20-2 (SRR25473320).
The presence of the RDs and MiDs in all M. microti strains used in the phylogenomic analysis (i.e., the 22 M. microti strains) is shown in Supplementary Figure 1. For most of the regions tested, the coverage was very similar between all M. microti strains. However, some variability between strains were observed for the RD5mic and RD6 regions, which were almost completely covered in Belgian strains but partially missing or absent in some other strains. The MiD2 region was present in all M. microti strains, except the reference OV254. Similarly, the RD724 region was present in all M. microti strains except the strain G25822. Differences were observed for the CRISPR-Cas loci (i.e., MiD1/RD207 regions) which were fully present in ATCC 35782, ATCC 19422, 416/01, 8753/00, microti-45, and three Belgian strains, but (partially) absent in other strains, including the 94-2272, Maus III, Maus IV, microti-36, microti-68 and G25821-G25828 strains (i.e., the cas2 and cas1 genes absent and the csm6 gene interrupted) and the OV254 reference strain. While this analysis reveals the presence of genomic regions, it does not provide information on the expression of the ORFs located in these regions, as they may be affected by SNPs and indels.
Pan-genome analysis and identification of polymorphism signals
The pan-genome analysis detected a total of 4,668 genes, of which 1,401 were annotated as hypothetical proteins. A schematic representation of the overlap between species is shown in Supplementary Figure 2. To obtain genes that could be potential biomarkers to discriminate M. microti from M. bovis, the genes present exclusively in the two virulent M. bovis or in all M. microti (n = 22) strains studied were extracted (Supplementary Figure 2). Note that, for the purpose of this study, the results for the M. bovis BCG Danish 1311 strains were not included in this analysis, because this vaccine strain differs from the traditional virulent strains. The resulting selection is shown in Table 3 and was performed from 47 and 34 genes exclusively present in virulent M. bovis and M. microti strains, respectively. Of these 81 genes, 30 were annotated as hypothetical proteins (i.e., 19 M. bovis and 11 M. microti exclusive genes).
Among the 47 genes exclusively present in virulent M. bovis (including ORFs located in RD1mic, MiD3 and RD3 regions) were several genes encoding for proline-glutamate (PE) and proline-proline-glutamate (PPE) proteins, as well as the EsxR (Rv3019c) and EsxS (Rv3020c) proteins. This deletion, corresponding to the MiD4 region (Rv3018c-Rv3022c), was previously reported in the M. microti OV254 strain and other M. microti strains isolated from patients (40). Other protein-encoding genes, such as PE_PGRS21 (FCU26_1135), the hypothetical protein Rv0530A (locus_tag BQ2027_RS02780), a transposase (BQ2027_RS17270), and the hypothetical protein (WP_015631092.1; locus_tag BQ2027_RS20600) were also absent in all 22 M. microti strains studied and present in the virulent M. bovis strains (Table 3, point A).
In addition to the genes associated with the RD4, RD11, RD12bov, and RD13 regions (mostly absent in M. bovis), three other additional genes were identified among 34 genes that were exclusively present in all M. microti. These genes encode the hypothetical protein Rv1508A, a hypothetical protein (JN986_RS12585) and the integral membrane protein Rv3162c. Further investigation revealed that the Rv3162c gene was present in the M. bovis genomes, but it contained a single nucleotide deletion resulting in an inactive gene product (Table 3, point B). Of note, some loci recorded as deleted in M. microti or M. bovis strains in the pan-genome are likely artifacts related to the Roary method used in this work, which is based on (imperfect) assembled genomes and annotations. Other genes were present in multiple copies, with different variants, and their presence or absence could not be confirmed.
Furthermore, we investigated the differences between the M. microti strains studied in this work by focusing on the three Belgian strains and the OV254 reference strain. Three ORFs (ORF2-ORF4) were found to be deleted or interrupted in the Belgian M. microti strains. These were associated with the RvD2 region including plcD, a glycosyltransferase (ORF2), a sulfite oxidase (ORF3), and a RND family transporter (ORF4), known to be absent in the M. tuberculosis H37Rv strain (H37Rv related deletion region or RvD). The region adjacent to RvD2, called RD152, was completely absent in these three strains. These two regions were also found to be partially (RvD2) or completely (RD152) absent in the M. microti-45 strain, the phylogenetically closest strain of the three Belgian strains. In addition, the RD152 region was found to be absent in the G25824 strain. Other genes were found to be deleted in the three Belgian strains, but present in the OV254 and the M. bovis strains (Table 3, point C). In addition to the genes associated with RDs/MiDs exclusively absent in M. microti OV254, five other genes were identified during the pan-genome analysis as shown in Table 3, point D.
Identification of protein-encoding genes as potential diagnosis markers
Proteins encoded by the genes from RDs present in virulent M. bovis strains and absent in all M. microti strains studied (RD1mic, RD3, and MiD3) and vice versa (RD4, RD11, RD12bov, RD13, and N-RD25bov/cap), as well as the proteins with presence/absence variation between virulent M. bovis and M. microti strains were further analyzed. As the aim was to identify “universal” antigens, genes that exhibited presence/absence variation between the different M. microti strains were not considered.
Of these 80 proteins, 52 were predicted to have a signal peptide, to be non-classically secreted and/or, to be located in the extracellular matrix by the SignalP6.0, SecretomeP2.0, Psortb3.0, Gpos-mPLoc, and CELLO2.5 tools. Protein-BLAST revealed that 27 of these 52 proteins had little or no homology to any sequence of MAC members in the database. Three proteins belonging to the PE/PPE family protein (PE_PGRS50, PPE55 and PE_PGRS21) showed sequence similarity between 40 and 60% but a very low query coverage (5–18%) in the aligned sequences, and were retained in this work. The antigenic propensity of all of these 30 proteins was then evaluated using the VaxiJen 2.0 and ANTIGENpro servers.
After these selection steps, a total of 28 proteins remained that were predicted by at least one of the tools to be secreted or located on the cell surface, with low conservation in environmental mycobacteria and putative antigenic properties (Table 4 and Supplementary Tables 3–5).
Prediction of sero-dominant epitopes
A total of 157 regions predicted as both linear and conformational epitopes with an antigenicity score >0.5 in the VaxiJen 2.0 server were identified and considered as promising epitopes for serodiagnosis. The peptide sequences with the highest antigenicity score are shown in Table 5 for the 28 proteins analyzed.
Discussion
M. microti is less virulent in humans and livestock than other members of the MTBC (51), and is not considered as a causative agent of TB in animals under the new animal health law (EU 2016/429). However, its spread and the increasing number of reported cases of infection in different hosts (9–11, 13, 76, 77) is a concern for the identification and management of control strategies for TB in animals, especially when the prevalence of animal TB is low. Field data suggest that M. microti may interfere with various ante-mortem diagnostic tests used in animal TB surveillance programs in livestock, camelids and other species [unpublished data from the Belgian NRL; (11)].
In this study, we first performed WGS on three M. microti field strains and compared the genomic diversity within the publicly available M. microti WGS data. Secondly, we compared the complete genomes of different M. microti and M. bovis strains, through characterization of large sequence polymorphisms (RDs, MiDs, and RvD) and pan-genomic analysis to identify potential markers for discriminating between the two mycobacteria in animal TB diagnosis. The analysis focused on proteins that are likely to be suitable for serological diagnostic tests. Serological assays are the primary ante-mortem tests used to detect TB in camelids [due to the poor performance of the IFNɤ and SICCT assays in these hosts; (4)] but, there are currently no assays available to distinguish between M. microti and M. bovis infections. An in silico approach was then used to select the best B-cell epitopes from these proteins for use in serodiagnosis.
The phylogenomic analysis provided insight into the genetic diversity within the M. microti species, including differences between isolates collected from different host species. As previously shown by (39), M. microti strains tended to cluster based on their spoligopattern rather than their host species. However, the bootstrap support for the relationships between clades was very low, indicating low confidence in the relative placement of the clades, possibly due to the limited WGS data available for M. microti. We chose to harmonize the spoligotype detection by determining it in silico using the WGS datasets to reduce bias between samples. In some cases, the spoligotypes that were extracted from the WGS data differed slightly from those previously reported (20, 38), which may be due to the inherent difficulty in determining spoligotypes, both in dry-lab and wet-lab techniques. SNP distances between M. microti strains isolated from Belgian alpaca farms could provide an indication of potential epidemiological links between strains. The mean substitution rate of M. tuberculosis has been estimated to be 0.3–0.5 SNPs per genome per year and SNP thresholds ranging from 5 (stringent, most likely) to 12 SNPs (more putative) have been defined to infer transmission events (78, 79). Several studies carried out on M. bovis isolates suggest that a similar rate might be applicable for animal-adapted mycobacterial species (78, 80–83). Based on these SNP thresholds, M. microti strains VAR696 and MI20-1, MI20-2, isolated from two different Belgian farms and differing by 18 SNPs, were unlikely to be epidemiologically linked. In contrast, the two M. microti strains MI20-1 and MI20-2 isolated from the same farm and showing 8 SNPs differences, could be related to a past transmission event or past epidemiological interactions within the same farm. However, these SNP profiles could also be caused by different past introductions, by the introduction of infected animals (with different genotypes) from different locations, or by different strains circulating on the same farm or in the region.
Genomic variations, such as RD or SNP, are markers used to study the evolution or epidemiological relationship existing between the species of the MTBC or the strains of the same species (82, 84). These variations may also be useful as diagnostic markers. In this study, the genomic differences between the tested M. microti strains were first investigated by comparing the RDs and MiDs. Little variation was observed between the M. microti strains for most of the tested regions. As previously reported, the M. microti strains are all characterized by the deletion of the RD1mic region, which contains several genes encoding the ESX-1 secretion system, including the virulence factors ESAT6 (Rv3875) and CFP10 (Rv3874). The MiD3, MiD4, and RD3 regions were also absent in all tested M. microti strains, including the Belgian M. microti strains (20, 39, 40). However, differences were observed between the different M. microti strains for some RDs/MiD, such as RD5mic, RD6, RD207/MiD1, and MiD2. The RD5 region contains the phospholipase C loci (plcA, plcB, and plcC), and is highly variable between different species of tuberculous mycobacteria but also between strains of the same species as shown by (21) and (39). The RD6 region, containing the IS1532 element, is also described as highly variable among MTBC strains (21). The presence or (partial) deletion of the RD207 region (which includes the MiD1 region) in the M. microti strains results in the loss of spacers in the DR locus and would therefore be associated with the llama and vole spoligotypes observed (20, 85). Since screening for RDs is limited to regions of the H37Rv M. tuberculosis genome, a pan-genomic analysis was performed in order to reveal additional differences. This analysis revealed the absence of several genes which, after more detailed investigation, were identified to be associated with RvD2 and RD152 regions. These two regions were only found (partially) deleted in the Belgian strains and M. microti-45, but were fully present in the reference OV254 and other M. microti strains studied (except for the RD152 region found to be also deleted in the G25824 strain). The RvD2 region is located downstream of the plcD gene (Rv1755c) and encodes three ORFs (Mb1785-Mb1787) predicted to be a hypothetical protein, a putative sulfite oxidase and a putative transmembrane transport protein (86–88). This region is known to be absent in M. tuberculosis H37Rv but is present in other members of the MTBC such as M. bovis. The RD152 region encodes genes Rv1758-Rv1765c (89), including a putative cutinase (cut1) and a member of the PE_PGRS protein family (wag22). Deletions of the RD152 region have previously been identified in M. africanum lineage nine strains (90) and M. tuberculosis Beijing/W strains (91). To our knowledge, these deletions have never been reported in M. microti or other animal-adapted MTBC species. Both regions have been reported as hotspots for IS6110 insertion sequences, which may facilitate recombination events (20, 85, 92). Thus, an IS6110-mediated deletion mechanism could be responsible for the loss of the RvD2 and RD152 regions in these strains. However, strains with these deletions were still capable of causing tuberculosis lesions in alpacas, as observed with Belgian M. microti strains, suggesting that these regions may not be essential for virulence.
Genes showing presence-absence variation were selected as potential protein markers to distinguish M. bovis from M. microti infections. A total of 80 protein-encoding genes were identified that were exclusively present in all M. microti strains tested and absent in all virulent M. bovis strains tested, or vice versa. The gene detection method employed in the pan-genome analysis was, in some cases, based on fragmented genome assemblies. Therefore, genes may have been missed due to assembly fractures, or other inaccuracies in the assembled genomes or gene predictions, highlighting the need for in vitro verification. Nevertheless, these types of analyses are a powerful and rapid screening method that can be used to generate peptide libraries that can be tested in vitro. Many potential biomarkers identified in the present pan-genome analysis have previously been reported as potential antigens to improve the serological diagnosis of human and/or bovine tuberculosis: Rv3872 (PE35), Rv3874 (CFP10), Rv3875 (ESAT6), Rv3876 (EspI) in RD1 (93–96), Rv1573, Rv1577c in RD3 (97), or Rv1508c, Rv1509, Rv1514c, Rv1516c in RD4 (93, 97). In addition, several of the genes with presence-absence variation identified in this study encode proteins belonging to the PE, PPE, and Polymorphic GC-Rich Sequence (PE_PGRS) families, including PE_PGRS21, PPE55, and PE_PGRS50. The proteins of these families, which constitute ~10% of the M. bovis genome (22), have been described as virulence factors involved in the pathogenesis of tuberculosis, but also as having a potential role in the antigenic variability within the MTBC (98, 99). In addition, several proteins of the PE/PPE/PE_PGRS family have been reported to be immunogenic and may be useful in the diagnosis of human and/or animal tuberculosis (98, 100). It is noteworthy that the antigens ESAT6 and CFP10, which are used in various assays for diagnosis of TB in animals (e.g., the IFNɤ assay and the Enferplex Camelid TB test), are absent in the M. microti strains but present in the M. bovis strains (7, 101, 102). Consequently, the absence of an antibody response to these antigens does not confirm the absence of M. microti infection, highlighting the importance of M. microti-specific antigens.
The main problem in establishing immunodiagnostic systems is the identification of immunogenic proteins specific to the target species. Several additional in silico analyses were performed to obtain a set of optimal potential immunogen proteins, including an evaluation of sequence homology, antigenic probability and accessibility by antibodies. Proteins with high sequence similarity/identity to environmental mycobacteria, such as members of the MAC commonly isolated from cattle, may cross-react in serological tests, and were therefore excluded (61, 63). After this additional filtering, a total of 28 candidate proteins were retained. However, it is possible that effective antigens were removed by this filtering. For example, proteins such as Rv3871 (103), Rv1509, Rv2658c (97), and Rv1586c (104) were not predicted to be secreted/located on the cell surface and/or antigenic in this work, whereas they have been reported to induce an antibody response in TB patients. As our aim was to maximize the detection of M. bovis vs. M. microti infection cases, only “universal” protein antigens present in all M. microti strains under study but absent in all virulent M. bovis strains (and vice-versa) were considered. Although genes that were absent/present in the Belgian M. microti strains (and some other strains) were not considered as potential antigens in this work, further investigation of these proteins may be valuable.
The production of recombinant proteins to obtain mycobacterial antigens is a challenging task associated with high costs and antigen variability related to expression systems and purification steps for recombinant proteins. The use of synthetic peptides can provide uniformity in antigen preparations and thus in assays (105). Therefore, the identification of peptides containing immunodominant B-cell epitopes may be essential for the development of diagnostic strategies aimed at antibody detection. An additional advantage is the reduced cost associated with the number of peptides for which antibody reactivity must be evaluated experimentally. Many studies have focused primarily on linear B-cell epitopes, whereas an important fraction of the B-cell epitopes are conformational (106). Until recently, the prediction of conformational B-cell epitopes required the protein structure to be deposited in the PDB database or the use of homology modeling tools (106, 107). However, the recently released neural network-based AlphaFold is a powerful alternative that enables highly accurate protein structure prediction (74). In this work, peptides based on both linear and conformational B-cell epitopes were sought using various in silico tools available online to predict B-cell epitopes based on amino acid sequences and/or protein structural data (74, 108). Therefore, a total of 157 peptides containing predicted B-cell epitopes were identified from the 28 previously selected proteins using the in silico approach. Interestingly, some peptides, which were derived from the full sequence of the candidate proteins, such as the peptide EISTNIRQAGVQYSRADEEQ for RV3874 (109) or the peptide AGMKLGWHPYHFPDEPDSKQ for Rv2653c (110), were previously reported to induce a moderate to strong CMI response in tuberculous animals or patients, suggesting that these peptides could potentially activate both humoral and cellular immunity and have a potential in various diagnostic applications. However, no information was found in the scientific literature on the recognition of these specific peptides by antibodies in a serological assay.
In silico assays can guide the experimental testing to screen for putative antigens more efficiently. In this paper, an in silico approach was used to identify putative antigenic peptides containing B-cell epitopes that discriminate between M. bovis and M. microti infections in animal TB serodiagnosis. In vitro analyses are nevertheless required to identify immunologically relevant peptides among the ones selected in silico. The immunoreactivity of the peptides should be evaluated by immunoassay (e.g., ELISA) with a sufficient number of reference sera, i.e. sera from presumed uninfected animals with a known status and sera from animals with M. bovis or M. microti infection confirmed by bacterial culture and molecular methods. The peptides with the highest sensitivity and specificity values will be further assessed for their serodiagnostic ability in a larger panel of sera. Previous data reported that the antibody response to antigens can be boosted in cattle, alpacas, goats following the intradermal tuberculin test, which can impact the performance of serological tests, in particular sensitivity (4, 14, 109). This should be considered in the future studies. Furthermore, although the use of carefully selected synthetic peptides decrease the inclusion of cross-reactive region of the protein antigens, it might be necessary to confirm the absence of aspecific response in the test using sera from NTM-infected animals. As combinations of mycobacterial antigens (peptides or recombinant proteins) are indicated to improve serodiagnosis of tuberculosis in humans and animals (16, 105, 111), the diagnostic performance of peptides confirmed as immunogenic in preliminary assays could be further evaluated in multiplexed serological assays or by polypeptide antigens.
Using WGS, we were able to extract potential biomarkers across the entire genome. In addition, whole-genome SNP-based analysis allowed us to characterize relationships between strains. As the number of M. microti WGS datasets increases, to which our study contributes, the effectiveness of these WGS-based analyses may increase further. In conclusion, the results presented in this study may contribute to the development of serological tests that can differentiate between M. bovis and M. microti in the future, thus avoiding misdiagnosis in the control TB in animals.
Data availability statement
The datasets presented in this study can be found in online repositories. The names of the repository/repositories and accession number(s) can be found in the article/Supplementary material.
Ethics statement
Ethical approval was not required for the study involving animals in accordance with the local legislation and institutional requirements because organs from the alpacas were obtained as part of the Belgian national bovine tuberculosis (bTB) control program, in accordance with the official guidelines of the Federal Authority for the control of bTB in Belgium [i.e., the Federal Agency for the Safety of the Food Chain [FASFC] and veterinary services].
Author contributions
CM: Conceptualization, Data curation, Formal analysis, Investigation, Methodology, Software, Writing – original draft, Writing – review & editing. BB: Conceptualization, Data curation, Formal analysis, Investigation, Methodology, Software, Writing – original draft, Writing – review & editing. VL-L: Writing – review & editing. KV: Methodology, Supervision, Writing – review & editing. SD: Methodology, Writing – review & editing. NR: Writing – review & editing. LM: Writing – review & editing. DF: Writing – review & editing. SM: Conceptualization, Supervision, Writing – review & editing.
Funding
The author(s) declare that no financial support was received for the research, authorship, and/or publication of this article.
Acknowledgments
We thank Wiebke Jansen and Philippe Vannoorenberghe for technical assistance along with Animal and Plant Health Agency (APHA) for information on the M. microti strains isolated from animals in UK. We also thank the technicians of the service transversal activities in Applied Genomics in Sciensano, Belgium for performing one of the whole-genome sequencing runs. In addition, we would like to thank the European Reference Laboratory for bovine TB for their assistance in detecting the infection by the MTBC.
Conflict of interest
The authors declare that the research was conducted in the absence of any commercial or financial relationships that could be construed as a potential conflict of interest.
Publisher's note
All claims expressed in this article are solely those of the authors and do not necessarily represent those of their affiliated organizations, or those of the publisher, the editors and the reviewers. Any product that may be evaluated in this article, or claim that may be made by its manufacturer, is not guaranteed or endorsed by the publisher.
Supplementary material
The Supplementary Material for this article can be found online at: https://www.frontiersin.org/articles/10.3389/fvets.2024.1446930/full#supplementary-material
Supplementary Figure 1 | Presence of RDs and MiDs regions in the M. microti datasets. This figure shows the percentage of the corresponding RDs and MiDs regions that were covered by the read mapping-based approach for each dataset.
Supplementary Figure 2 | Results of the pan-genome analysis, showing the number of genes detected exclusively in all M. microti and all virulent M. bovis strains. Note that all 22 M. microti strains and the two virulent M. bovis strains (AF2122/97 and MB3601) were considered. The horizontal bars indicate the total number of genes detected in the corresponding species by the pan-genome analysis. The vertical lines indicate the size of the overlap as indicated by the dots in membership matrix.
References
1. WOAH. Infection with Mycobacterium tuberculosis complex. In: World Organisation for Animal Health—Terrestrial Animal Health Code, Chapter 8.12. Paris (2023).
2. Palmer MV, Thacker TC, Waters WR, Gortázar C, Corner LAL. Mycobacterium bovis: a model pathogen at the interface of livestock, wildlife, and humans. Vet Med Int. (2012) 2012:e236205. doi: 10.1155/2012/236205
3. García-Bocanegra I, Barranco I, Rodríguez-Gómez IM, Pérez B, Gómez-Laguna J, Rodríguez S, et al. Tuberculosis in alpacas (Lama pacos) caused by Mycobacterium bovis. J Clin Microbiol. (2010) 48:1960–4. doi: 10.1128/JCM.02518-09
4. Rhodes S, Holder T, Clifford D, Dexter I, Brewer J, Smith N, et al. Evaluation of gamma interferon and antibody tuberculosis tests in alpacas. Clin Vac Immunol. (2012) 19:1677–83. doi: 10.1128/CVI.00405-12
5. Lyashchenko KP, Greenwald R, Esfandiari J, Meylan M, Burri IH, Zanolari P. Antibody responses in New World camelids with tuberculosis caused by Mycobacterium microti. Vet Microbiol. (2007) 125:265–73. doi: 10.1016/j.vetmic.2007.05.026
6. Bezos J, Casal C, Álvarez J, Díez-Guerrier A, Rodríguez-Bertos A, Romero B, et al. Evaluation of the performance of cellular and serological diagnostic tests for the diagnosis of tuberculosis in an alpaca (Vicugna pacos) herd naturally infected with Mycobacterium bovis. Prev Vet Med. (2013) 111:304–13. doi: 10.1016/j.prevetmed.2013.05.013
7. Krajewska-Wedzina M, Didkowska A, Sridhara AA, Elahi R, Johnathan-Lee A, Radulski Ł, et al. Transboundary tuberculosis: importation of alpacas infected with Mycobacterium bovis from the United Kingdom to Poland and potential for serodiagnostic assays in detecting tuberculin skin test false-negative animals. Transbound Emerg Dis. (2020) 67:1306–14. doi: 10.1111/tbed.13471
8. Kasai H, Ezaki T, Harayama S. Differentiation of phylogenetically related slowly growing mycobacteria by their gyrB sequences. J Clin Microbiol. (2000) 38:301–8. doi: 10.1128/JCM.38.1.301-308.2000
9. Ghielmetti G, Kupca AM, Hanczaruk M, Friedel U, Weinberger H, Revilla-Fernández S, et al. Mycobacterium microti infections in free-ranging red deer (Cervus elaphus). Emerg Infect Dis. (2021) 27:2025–32. doi: 10.3201/eid2708.210634
10. Michelet L, de Cruz K, Zanella G, Aaziz R, Bulach T, Karoui C, et al. Infection with Mycobacterium microti in animals in France. J Clin Microbiol. (2015) 53:981–5. doi: 10.1128/JCM.02713-14
11. Michelet L, de Cruz K, Tambosco J, Hénault S, Boschiroli M-L. Mycobacterium microti interferes with bovine tuberculosis surveillance. Microorganisms. (2020) 8:1850. doi: 10.3390/microorganisms8121850
12. Smith NH, Crawshaw T, Parry J, Birtles RJ. Mycobacterium microti: more diverse than previously thought. J Clin Microbiol. (2009) 47:2551–9. doi: 10.1128/JCM.00638-09
13. Tagliapietra V, Boniotti MB, Mangeli A, Karaman I, Alborali G, Chiari M, et al. Mycobacterium microti at the environment and wildlife interface. Microorganisms. (2021) 9:2084. doi: 10.3390/microorganisms9102084
14. Infantes-Lorenzo JA, Whitehead CE, Moreno I, Bezos J, Roy A, Dominguez L, et al. Development and evaluation of a serological assay for the diagnosis of tuberculosis in alpacas and llamas. Front Vet Sci. (2018) 5:189. doi: 10.3389/fvets.2018.00189
15. Infantes-Lorenzo JA, Dave D, Moreno I, Anderson P, Lesellier S, Gormley E, et al. New serological platform for detecting antibodies against Mycobacterium tuberculosis complex in European badgers. Vet Med Sci. (2019) 5:61–9. doi: 10.1002/vms3.134
16. O'Brien A, Clarke J, Hayton A, Adler A, Cutler K, Shaw DJ, et al. Diagnostic accuracy of the Enferplex Bovine Tuberculosis antibody test in cattle sera. Sci Rep. (2023) 13:1875. doi: 10.1038/s41598-023-28410-9
17. Lo Y-T, Shih T-C, Pai T-W, Ho L-P, Wu J-L, Chou H-Y. Conformational epitope matching and prediction based on protein surface spiral features. BMC Genom. (2021) 22:116. doi: 10.1186/s12864-020-07303-5
18. Aranaz A, Liébana E, Mateos A, Dominguez L, Vidal D, Domingo M, et al. Spacer oligonucleotide typing of Mycobacterium bovis strains from cattle and other animals: a tool for studying epidemiology of tuberculosis. J Clin Microbiol. (1996) 34:2734–40. doi: 10.1128/jcm.34.11.2734-2740.1996
19. Kremer K, van Soolingen D, van Embden J, Hughes S, Inwald J, Hewinson G. Mycobacterium microti: more widespread than previously thought. J Clin Microbiol. (1998) 36:2793–4. doi: 10.1128/JCM.36.9.2793-2794.1998
20. Brodin P, Eiglmeier K, Marmiesse M, Billault A, Garnier T, Niemann S, et al. Bacterial artificial chromosome-based comparative genomic analysis identifies Mycobacterium microti as a natural ESAT-6 deletion mutant. Infect Immun. (2002) 70:5568–78. doi: 10.1128/IAI.70.10.5568-5578.2002
21. Brosch R, Gordon SV, Marmiesse M, Brodin P, Buchrieser C, Eiglmeier K, et al. A new evolutionary scenario for the Mycobacterium tuberculosis complex. Proc Natl Acad Sci USA. (2002) 99:3684–9. doi: 10.1073/pnas.052548299
22. Guimaraes AMS, Zimpel CK. Mycobacterium bovis: from genotyping to genome sequencing. Microorganisms. (2020) 8:667. doi: 10.3390/microorganisms8050667
23. Landolt P, Stephan R, Scherrer S. Development of a new High Resolution Melting (HRM) assay for identification and differentiation of Mycobacterium tuberculosis complex samples. Sci Rep. (2019) 9:1850. doi: 10.1038/s41598-018-38243-6
24. De la Fuente J, Diez-Delgado I, Contreras M, Vicente J, Cabezas-Cruz A, Tobes R, et al. Comparative genomics of field isolates of Mycobacterium bovis and M. caprae provides evidence for possible correlates with bacterial viability and virulence. PLoS Negl Trop Dis. (2015) 9:e0004232. doi: 10.1371/journal.pntd.0004232
25. Meehan CJ, Goig GA, Kohl TA, Verboven L, Dippenaar A, Ezewudo M, et al. Whole genome sequencing of Mycobacterium tuberculosis: current standards and open issues. Nat Rev Microbiol. (2019) 17:533–45. doi: 10.1038/s41579-019-0214-5
26. Reis AC, Cunha MV. Genome-wide estimation of recombination, mutation and positive selection enlightens diversification drivers of Mycobacterium bovis. Sci Rep. (2021) 11:18789. doi: 10.1038/s41598-021-98226-y
27. Reis AC, Cunha MV. The open pan-genome architecture and virulence landscape of Mycobacterium bovis. Microb Genom. (2021) 7:000664. doi: 10.1099/mgen.0.000664
28. Lorente-Leal V, Liandris E, Pacciarini M, Botelho A, Kenny K, Loyo B, et al. Direct PCR on tissue samples to detect Mycobacterium tuberculosis complex: an alternative to the bacteriological culture. J Clin Microbiol. (2021) 59:20. doi: 10.1128/JCM.01404-20
29. Thierry D, Cave MD, Eisenach KD, Crawford JT, Bates JH, Gicquel B, et al. IS6110, an IS-like element of Mycobacterium tuberculosis complex. Nucl Acids Res. (1990) 18:188. doi: 10.1093/nar/18.1.188
30. Kamerbeek J, Schouls L, Kolk A, Van Agterveld M, van Soolingen D, Kuijper S, et al. Simultaneous detection and strain differentiation of Mycobacterium tuberculosis for diagnosis and epidemiology. J Clin Microbiol. (1997) 35:907–14. doi: 10.1128/jcm.35.4.907-914.1997
31. Bogaerts B, Delcourt T, Soetaert K, Boarbi S, Ceyssens PJ, Winand R, et al. A bioinformatics whole-genome sequencing workflow for clinical Mycobacterium tuberculosis complex isolate analysis, validated using a reference collection extensively characterized with conventional methods and in silico approaches. J Clin Microbiol. (2021) 59:21. doi: 10.1128/JCM.00202-21
32. Bolger AM, Lohse M, Usadel B. Trimmomatic: a flexible trimmer for illumina sequence data. Bioinformatics. (2014) 30:2114–20. doi: 10.1093/bioinformatics/btu170
33. Prjibelski A, Antipov D, Meleshko D, Lapidus A, Korobeynikov A. Using SPAdes de novo assembler. Curr Protocol Bioinformat. (2020) 70:e102. doi: 10.1002/cpbi.102
34. Gurevich A, Saveliev V, Vyahhi N, Tesler G. QUAST: quality assessment tool for genome assemblies. Bioinformatics. (2013) 29:1072–5. doi: 10.1093/bioinformatics/btt086
35. Wood DE, Lu J, Langmead B. Improved metagenomic analysis with Kraken 2. Genome Biol. (2019) 20:257. doi: 10.1186/s13059-019-1891-0
36. Langmead B, Salzberg SL. Fast gapped-read alignment with Bowtie 2. Nat Methods. (2012) 9:357–9. doi: 10.1038/nmeth.1923
37. Li H, Handsaker B, Wysoker A, Fennell T, Ruan J, Homer N, et al. The sequence alignment/map format and SAMtools. Bioinformatics. (2009) 25:2078–9. doi: 10.1093/bioinformatics/btp352
38. van Soolingen D, van der Zanden AGM, de Haas PEW, Noordhoek GT, Kiers A, Foudraine NA, et al. Diagnosis of Mycobacterium microti infections among humans by using novel genetic markers. J Clin Microbiol. (1998) 36:1840–5. doi: 10.1128/JCM.36.7.1840-1845.1998
39. Orgeur M, Frigui W, Pawlik A, Clark S, Williams A, Ates LS, et al. Pathogenomic analyses of Mycobacterium microti, an ESX-1- deleted member of the Mycobacterium tuberculosis complex causing disease in various hosts. Microbial Genom. (2021) 7:e000505. doi: 10.1099/mgen.0.000505
40. Garcia-Pelayo MC, Caimi KC, Inwald JK, Hinds J, Bigi F, Romano MI, et al. Microarray analysis of Mycobacterium microti reveals deletion of genes encoding PE-PPE proteins and ESAT-6 family antigens. Tubercul Microarrays Mycobacterium tuberculosis. (2004) 84:159–66. doi: 10.1016/j.tube.2003.12.002
41. Malm S, Linguissi LSG, Tekwu EM, Vouvoungui JC, Kohl TA, Beckert P, et al. New Mycobacterium tuberculosis complex sublineage, Brazzaville, Congo. Emerg Infect Dis. (2017) 23:423–9. doi: 10.3201/eid2303.160679
42. Boniotti MB, Gaffuri A, Gelmetti D, Tagliabue S, Chiari M, Mangeli A, et al. Detection and molecular characterization of Mycobacterium microti isolates in wild boar from Northern Italy. J Clin Microbiol. (2014) 52:2834–43. doi: 10.1128/JCM.00440-14
43. Brites D, Loiseau C, Menardo F, Borrell S, Boniotti MB, Warren R, et al. A new phylogenetic framework for the animal-adapted Mycobacterium tuberculosis complex. Front Microbiol. (2018) 9:2820. doi: 10.3389/fmicb.2018.02820
44. Bogaerts B, Van den Bossche A, Verhaegen B, Delbrassinne L, Mattheus W, Nouws S, et al. Closing the gap: Oxford Nanopore Technologies R10 sequencing allows comparable results to illumina sequencing for SNP-based outbreak investigation of bacterial pathogens. J Clin Microbiol. (2024) 62:e01576–23. doi: 10.1128/jcm.01576-23
45. Danecek P, Bonfield JK, Liddle J, Marshall J, Ohan V, Pollard MO, et al. Twelve years of SAMtools and BCFtools. GigaScience. (2021) 10:giab008. doi: 10.1093/gigascience/giab008
46. Croucher NJ, Page AJ, Connor TR, Delaney AJ, Keane JA, Bentley SD, et al. Rapid phylogenetic analysis of large samples of recombinant bacterial whole genome sequences using Gubbins. Nucleic Acids Res. (2015) 43:e15. doi: 10.1093/nar/gku1196
47. Arndt D, Grant JR, Marcu A, Sajed T, Pon A, Liang Y, et al. PHASTER: a better, faster version of the PHAST phage search tool. Nucleic Acids Res. (2016) 44:W16–21. doi: 10.1093/nar/gkw387
48. Kumar S, Stecher G, Li M, Knyaz C, Tamura K. MEGA X: molecular evolutionary genetics analysis across computing platforms. Mol Biol Evol. (2018) 35:1547–9. doi: 10.1093/molbev/msy096
49. Xia E, Teo Y-Y, Ong RT-H. SpoTyping: fast and accurate in silico Mycobacterium spoligotyping from sequence reads. Genome Med. (2016) 8:19. doi: 10.1186/s13073-016-0270-7
50. Cole ST. Comparative and functional genomics of the Mycobacterium tuberculosis complex. Microbiology. (2002) 148:2919–28. doi: 10.1099/00221287-148-10-2919
51. Frota CC, Hunt DM, Buxton RS, Rickman L, Hinds J, Kremer K, et al. Genome structure in the vole bacillus, Mycobacterium microti, a member of the Mycobacterium tuberculosis complex with a low virulence for humans. Microbiology. (2004) 150:1519–27. doi: 10.1099/mic.0.26660-0
52. Gagneux S, DeRiemer K, Van T, Kato-Maeda M, de Jong BC, Narayanan S, et al. Variable host-pathogen compatibility in Mycobacterium tuberculosis. Proc Natl Acad Sci USA. (2006) 103:2869–73. doi: 10.1073/pnas.0511240103
53. Behr MA, Wilson MA, Gill WP, Salamon H, Schoolnik GK, Rane S, et al. Comparative genomics of BCG vaccines by whole-genome DNA microarray. Science. (1999) 284:1520–3. doi: 10.1126/science.284.5419.1520
54. Seemann T. Prokka: rapid prokaryotic genome annotation. Bioinformatics. (2014) 30:2068–9. doi: 10.1093/bioinformatics/btu153
55. Page AJ, Cummins CA, Hunt M, Wong VK, Reuter S, Holden MTG, et al. Roary: rapid large-scale prokaryote pan genome analysis. Bioinformatics. (2015) 31:3691–3. doi: 10.1093/bioinformatics/btv421
56. Teufel F, Almagro Armenteros JJ, Johansen AR, Gíslason MH, Pihl SI, Tsirigos KD, et al. SignalP 6.0 predicts all five types of signal peptides using protein language models. Nat Biotechnol. (2022) 40:1023–5. doi: 10.1038/s41587-021-01156-3
57. Bendtsen JD, Kiemer L, Fausbøll A, Brunak S. Non-classical protein secretion in bacteria. BMC Microbiol. (2005) 5:58. doi: 10.1186/1471-2180-5-58
58. Yu NY, Wagner JR, Laird MR, Melli G, Rey S, Lo R, et al. PSORTb 3.0: improved protein subcellular localization prediction with refined localization subcategories and predictive capabilities for all prokaryotes. Bioinformatics. (2010) 26:1608–15. doi: 10.1093/bioinformatics/btq249
59. Shen H-B, Chou K-C. Gpos-mPLoc: a top-down approach to improve the quality of predicting subcellular localization of gram-positive bacterial proteins. PPL. (2009) 16:1478–84. doi: 10.2174/092986609789839322
60. Yu C-S, Chen Y-C, Lu C-H, Hwang J-K. Prediction of protein subcellular localization. Proteins. (2006) 64:643–51. doi: 10.1002/prot.21018
61. Biet F, Boschiroli ML. Non-tuberculous mycobacterial infections of veterinary relevance. Res Vet Sci. (2014) 97:S69–77. doi: 10.1016/j.rvsc.2014.08.007
62. Infantes-Lorenzo JA, Moreno I, Risalde MÁ, Roy Á, Villar M, Romero B, et al. Proteomic characterisation of bovine and avian purified protein derivatives and identification of specific antigens for serodiagnosis of Bovine tuberculosis. Clin Proteom. (2017) 14:36. doi: 10.1186/s12014-017-9171-z
63. Varela-Castro L, Barral M, Arnal MC, Fernández de Luco D, Gortázar C, Garrido JM, et al. Beyond tuberculosis: diversity and implications of non-tuberculous mycobacteria at the wildlife-livestock interface. Transbound Emerg Dis. (2022) 69:e2978-93. doi: 10.1111/tbed.14649
64. Magnan CN, Zeller M, Kayala MA, Vigil A, Randall A, Felgner PL, et al. High-throughput prediction of protein antigenicity using protein microarray data. Bioinformatics. (2010) 26:2936–43. doi: 10.1093/bioinformatics/btq551
65. Doytchinova IA, Flower DR. VaxiJen: a server for prediction of protective antigens, tumour antigens and subunit vaccines. BMC Bioinform. (2007) 8:4. doi: 10.1186/1471-2105-8-4
66. Chou PY, Fasman GD. Prediction of the secondary structure of proteins from their amino acid sequence. Adv Enzymol Relat Areas Mol Biol. (1978) 47:145–8. doi: 10.1002/9780470122921.ch2
67. Emini EA, Hughes JV, Perlow DS, Boger J. Induction of hepatitis A virus-neutralizing antibody by a virus-specific synthetic peptide. J Virol. (1985) 55:836–9. doi: 10.1128/jvi.55.3.836-839.1985
68. Karplus PA, Schulz GE. Prediction of chain flexibility in proteins. Naturwissenschaften. (1985) 72:212–3. doi: 10.1007/BF01195768
69. Parker JMR, Guo D. New hydrophilicity scale derived from high-performance liquid chromatography peptide retention data: correlation of predicted surface residues with antigenicity and X-ray-derived accessible sites? Biochemistry. (1986) 25:5425–32. doi: 10.1021/bi00367a013
70. Kolaskar AS, Tongaonkar PC. A semi-empirical method for prediction of antigenic determinants on protein antigens. FEBS Lett. (1990) 276:172–4. doi: 10.1016/0014-5793(90)80535-Q
71. Jespersen MC, Peters B, Nielsen M, Marcatili P. BepiPred-2.0: improving sequence-based B-cell epitope prediction using conformational epitopes. Nucl Acids Res. (2017) 45:W24–29. doi: 10.1093/nar/gkx346
72. Andersen PH, Nielsen M, Lund O. Prediction of residues in discontinuous B-cell epitopes using protein 3D structures. Protein Sci. (2006) 15:2558–67. doi: 10.1110/ps.062405906
73. Ponomarenko J, Bui H-H, Li W, Fusseder N, Bourne PE, Sette A, et al. ElliPro: a new structure-based tool for the prediction of antibody epitopes. BMC Bioinform. (2008) 9:514. doi: 10.1186/1471-2105-9-514
74. Jumper J, Evans R, Pritzel A, Green T, Figurnov M, Ronneberger O, et al. Highly accurate protein structure prediction with AlphaFold. Nature. (2021) 596:583–9. doi: 10.1038/s41586-021-03819-2
75. Barbier M, Wirth T. The evolutionary history, demography, and spread of the Mycobacterium tuberculosis complex. Microbiol Spectr. (2016) 4:8. doi: 10.1128/microbiolspec.TBTB2-0008-2016
76. Michelet L, Richomme C, Réveillaud E, Cruz KD, Moyen J-L, Boschiroli ML. Mycobacterium microti infection in red foxes in France. Microorganisms. (2021) 9:1257. doi: 10.3390/microorganisms9061257
77. Pérez de Val B, Sanz A, Soler M, Allepuz A, Michelet L, Boschiroli ML, et al. Mycobacterium microti infection in free-ranging wild boar, Spain, 2017–2019. Emerg Infect Dis. (2019) 25:2152–4. doi: 10.3201/eid2511.190746
78. Crispell J, Zadoks RN, Harris SR, Paterson B, Collins DM, de-Lisle GW, et al. Using whole genome sequencing to investigate transmission in a multi-host system: Bovine tuberculosis in New Zealand. BMC Genom. (2017) 18:180. doi: 10.1186/s12864-017-3569-x
79. Merker M, Kohl TA, Niemann S, Supply P. The evolution of strain typing in the Mycobacterium tuberculosis complex. In:Gagneux S, , editor. Strain Variation in the Mycobacterium tuberculosis Complex: Its Role in Biology, Epidemiology and Control, Advances in Experimental Medicine and Biology. Cham: Springer (2017). p. 43–78.
80. Akhmetova A, Guerrero J, McAdam P, Salvador LCM, Crispell J, Lavery J, et al. Genomic epidemiology of Mycobacterium bovis infection in sympatric badger and cattle populations in Northern Ireland. Microb Genom. (2023) 9:mgen001023. doi: 10.1099/mgen.0.001023
81. Duault H, Michelet L, Boschiroli M-L, Durand B, Canini L. A Bayesian evolutionary model towards understanding wildlife contribution to F4-family Mycobacterium bovis transmission in the South-West of France. Vet Res. (2022) 53:28. doi: 10.1186/s13567-022-01044-x
82. Perea C, Ciaravino G, Stuber T, Thacker TC, Robbe-Austerman S, Allepuz A, et al. Whole-genome SNP analysis identifies putative Mycobacterium bovis transmission clusters in livestock and wildlife in Catalonia, Spain. Microorganisms. (2021) 9:1629. doi: 10.3390/microorganisms9081629
83. van Tonder AJ, Thornton MJ, Conlan AJK, Jolley KA, Goolding L, Mitchell AP, et al. Inferring Mycobacterium bovis transmission between cattle and badgers using isolates from the Randomised Badger Culling Trial. PLoS Pathog. (2021) 17:e1010075. doi: 10.1371/journal.ppat.1010075
84. Dippenaar A, Parsons SDC, Sampson SL, van der Merwe RG, Drewe JA, Abdallah AM, et al. Whole genome sequence analysis of Mycobacterium suricattae. Tuberculosis. (2015) 95:682–8. doi: 10.1016/j.tube.2015.10.001
85. Cerezo-Cortés M, Rodríguez-Castillo J, Hernández-Pando R, Murcia M. Circulation of M. tuberculosis Beijing genotype in Latin America and the Caribbean. Pathog Glob Health. (2019) 113:336–51. doi: 10.1080/20477724.2019.1710066
86. Zheng H, Lu L, Wang B, Pu S, Zhang X, Zhu G, et al. Genetic basis of virulence attenuation revealed by comparative genomic analysis of Mycobacterium tuberculosis strain H37Ra versus H37Rv. PLoS ONE. (2008) 3:e2375. doi: 10.1371/journal.pone.0002375
87. Stavrum R, Valvatne H, Bø TH, Jonassen I, Hinds J, Butcher PD, et al. Genomic diversity among Beijing and non-Beijing Mycobacterium tuberculosis isolates from Myanmar. PLoS ONE. (2008) 3:e1973. doi: 10.1371/journal.pone.0001973
88. Gordon SV, Brosch R, Billault A, Garnier T, Eiglmeier K, Cole ST. Identification of variable regions in the genomes of tubercle bacilli using bacterial artificial chromosome arrays. Mol Microbiol. (1999) 32:643–55. doi: 10.1046/j.1365-2958.1999.01383.x
89. Gómez-González PJ, Campino S, Phelan JE, Clark TG. Portable sequencing of Mycobacterium tuberculosis for clinical and epidemiological applications. Brief Bioinform. (2022) 23:bbac256. doi: 10.1093/bib/bbac256
90. Coscolla M, Gagneux S, Menardo F, Loiseau C, Ruiz P, Borrell S, et al. Phylogenomics of Mycobacterium africanum reveals a new lineage and a complex evolutionary history. Microbiol Genom. (2021) 7:e000477. doi: 10.1099/mgen.0.000477
91. Tsolaki AG, Gagneux S, Pym AS, Goguet de la Salmoniere YOL, Kreiswirth BN, Van Soolingen D, et al. Genomic deletions classify the Beijing/W strains as a distinct genetic lineage of Mycobacterium tuberculosis. J Clin Microbiol. (2005) 43:3185–91. doi: 10.1128/JCM.43.7.3185-3191.2005
92. Sampson SL, Warren RM, Richardson M, Victor TC, Jordaan AM. IS6110-mediated deletion polymorphism in the direct repeat region of clinical isolates of Mycobacterium tuberculosis. J Bacteriol. (2003) 185:2856–66. doi: 10.1128/JB.185.9.2856-2866.2003
93. Al-Khodari NY, Al-Attiyah R, Mustafa AS. Identification, diagnostic potential, and natural expression of immunodominant seroreactive peptides encoded by five Mycobacterium tuberculosis-specific genomic regions. Clin Vac Immunol. (2011) 18:477–82. doi: 10.1128/CVI.00405-10
94. Lyashchenko KP, Grandison A, Keskinen K, Sikar-Gang A, Lambotte P, Esfandiari J, et al. Identification of novel antigens recognized by serum antibodies in Bovine tuberculosis. Clin Vac Immunol. (2017) 24:e00259–17. doi: 10.1128/CVI.00259-17
95. Mukherjee P, Dutta M, Datta P, Dasgupta A, Pradhan R, Pradhan M, et al. The RD1-encoded antigen Rv3872 of Mycobacterium tuberculosis as a potential candidate for serodiagnosis of tuberculosis. Clin Microbiol Infect. (2007) 13:146–52. doi: 10.1111/j.1469-0691.2006.01660.x
96. Xu J-N, Chen J-P, Chen D-L. Serodiagnosis efficacy and immunogenicity of the fusion protein of Mycobacterium tuberculosis composed of the 10-kilodalton culture filtrate protein, ESAT-6, and the extracellular domain fragment of PPE68. Clin Vac Immunol. (2012) 19:536–44. doi: 10.1128/CVI.05708-11
97. Ren N, JinLi J, Zhou X, Wang J, Ge P, Khan FA, et al. Identification of new diagnostic biomarkers for Mycobacterium tuberculosis and the potential application in the serodiagnosis of human tuberculosis. Microb Biotechnol. (2018) 11:893–904. doi: 10.1111/1751-7915.13291
98. D'Souza C, Kishore U, Tsolaki AG. The PE-PPE family of Mycobacterium tuberculosis: proteins in disguise. Immunobiology. (2023) 228:152321. doi: 10.1016/j.imbio.2022.152321
99. Karboul A, Mazza A. Frequent homologous recombination events in Mycobacterium tuberculosis PE/PPE multigene families: potential role in antigenic variability. J Bacteriol. (2008) 190:7838–46. doi: 10.1128/JB.00827-08
100. Moens C, Filée P, Boes A, Alie C, Dufrasne F, André E, et al. Identification of new Mycobacterium bovis antigens and development of a multiplexed serological bead-immunoassay for the diagnosis of Bovine tuberculosis in cattle. PLoS ONE. (2023) 18:e0292590. doi: 10.1371/journal.pone.0292590
101. Clifford V, He Y, Zufferey C, Connell T, Curtis N. Interferon gamma release assays for monitoring the response to treatment for tuberculosis: a systematic review. Tuberculosis. (2015) 95:639–50. doi: 10.1016/j.tube.2015.07.002
102. Nuñez-Garcia J, Downs SH, Parry JE, Abernethy DA, Broughan JM, Cameron AR, et al. Meta-analyses of the sensitivity and specificity of ante-mortem and post-mortem diagnostic tests for bovine tuberculosis in the UK and Ireland. Prev Vet Med. (2018) 153:94–107. doi: 10.1016/j.prevetmed.2017.02.017
103. Greenaway C, Lienhardt C, Adegbola R, Brusasca P, McAdam K, Menzies D. Humoral response to Mycobacterium tuberculosis antigens in patients with tuberculosis in the Gambia. Int J Tuberc Lung Dis. (2005) 9:1112–9.
104. Rosenkrands I, Aagaard C, Weldingh K, Brock I, Dziegiel MH, Singh M, et al. Identification of Rv0222 from RD4 as a novel serodiagnostic target for tuberculosis. Tuberculosis. (2008) 88:335–43. doi: 10.1016/j.tube.2007.12.001
105. Baassi L, Sadki K, Seghrouchni F, Contini S, Cherki W, Nagelkerke N, et al. Evaluation of a multi-antigen test based on B-cell epitope peptides for the serodiagnosis of pulmonary tuberculosis. Int J Tuberc Lung Dis. (2009) 13:848–54.
106. Sun P, Ju H, Liu Z, Ning Q, Zhang J, Zhao X, et al. Bioinformatics resources and tools for conformational B-cell epitope prediction. Comput Math Methods Med. (2013) 2013:e943636. doi: 10.1155/2013/943636
107. Zargaran FN, Akya A, Rezaeian S, Ghadiri K, Lorestani RC, Madanchi H, et al. B cell epitopes of four fimbriae antigens of Klebsiella pneumoniae: a comprehensive in silico study for vaccine development. Int J Pept Res Ther. (2021) 27:875–86. doi: 10.1007/s10989-020-10134-3
108. Potocnakova L, Bhide M, Pulzova LB. An introduction to B-cell epitope mapping and in silico epitope prediction. J Immunol Res. (2016) 2016:e6760830. doi: 10.1155/2016/6760830
109. Roupie V, Alonso-Velasco E, Van Der Heyden S, Holbert S, Duytschaever L, Berthon P, et al. Evaluation of mycobacteria-specific gamma interferon and antibody responses before and after a single intradermal skin test in cattle naturally exposed to M. avium subsp paratuberculosis and experimentally infected with M. bovis. Vet Immunol Immunopathol. (2018) 196:35–47. doi: 10.1016/j.vetimm.2017.12.007
110. Aagaard C, Brock I, Olsen A, Ottenhoff THM, Weldingh K, Andersen P. Mapping immune reactivity toward Rv2653 and Rv2654: two novel low-molecular-mass antigens found specifically in the Mycobacterium tuberculosis complex. J Infect Dis. (2004) 189:812–9. doi: 10.1086/381679
Keywords: Mycobacterium microti, Mycobacterium bovis, tuberculosis, B-cell epitopes, diagnosis, whole-genome sequencing, antigens
Citation: Moens C, Bogaerts B, Lorente-Leal V, Vanneste K, De Keersmaecker SCJ, Roosens NHC, Mostin L, Fretin D and Marché S (2024) Genomic comparison between Mycobacterium bovis and Mycobacterium microti and in silico analysis of peptide-based biomarkers for serodiagnosis. Front. Vet. Sci. 11:1446930. doi: 10.3389/fvets.2024.1446930
Received: 10 June 2024; Accepted: 28 August 2024;
Published: 20 September 2024.
Edited by:
Lorraine Michelet, Agence Nationale de Sécurité Sanitaire de l'Alimentation, de l'Environnement et du Travail (ANSES), FranceReviewed by:
Maria Beatrice Boniotti, Experimental Zooprophylactic Institute of Lombardy and Emilia Romagna (IZSLER), ItalyWynand Johan Goosen, Stellenbosch University, South Africa
Copyright © 2024 Moens, Bogaerts, Lorente-Leal, Vanneste, De Keersmaecker, Roosens, Mostin, Fretin and Marché. This is an open-access article distributed under the terms of the Creative Commons Attribution License (CC BY). The use, distribution or reproduction in other forums is permitted, provided the original author(s) and the copyright owner(s) are credited and that the original publication in this journal is cited, in accordance with accepted academic practice. No use, distribution or reproduction is permitted which does not comply with these terms.
*Correspondence: Charlotte Moens, Y2hhcmxvdHRlLm1vZW5zQHVjbG91dmFpbi5iZQ==
†These authors have contributed equally to this work