- 1Department of Comparative, Diagnostic, and Population Medicine, College of Veterinary Medicine, University of Florida, Gainesville, FL, United States
- 2Independent Researcher, Livorno, Italy
Facial expressions are essential for communication and emotional expression across species. Despite the improvements brought by tools like the Horse Grimace Scale (HGS) in pain recognition in horses, their reliance on human identification of characteristic traits presents drawbacks such as subjectivity, training requirements, costs, and potential bias. Despite these challenges, the development of facial expression pain scales for animals has been making strides. To address these limitations, Automated Pain Recognition (APR) powered by Artificial Intelligence (AI) offers a promising advancement. Notably, computer vision and machine learning have revolutionized our approach to identifying and addressing pain in non-verbal patients, including animals, with profound implications for both veterinary medicine and animal welfare. By leveraging the capabilities of AI algorithms, we can construct sophisticated models capable of analyzing diverse data inputs, encompassing not only facial expressions but also body language, vocalizations, and physiological signals, to provide precise and objective evaluations of an animal's pain levels. While the advancement of APR holds great promise for improving animal welfare by enabling better pain management, it also brings forth the need to overcome data limitations, ensure ethical practices, and develop robust ground truth measures. This narrative review aimed to provide a comprehensive overview, tracing the journey from the initial application of facial expression recognition for the development of pain scales in animals to the recent application, evolution, and limitations of APR, thereby contributing to understanding this rapidly evolving field.
1 Introduction
In animals and humans, facial expressions play a crucial role as a primary non-verbal method for managing peer interactions and conveying information about emotional states (1). Scientific interest in facial expressions was initiated in the 1860s by Duchenne de Boulogne. However, it is in the last two decades that the utilization of facial expressions for understanding emotional conditions, such as pain, has expanded in both humans and non-human species (2). Notably, it was demonstrated that facial expressions of pain show consistency across ages, genders, cognitive states (e.g., non-communicative patients), and different types of pain and may correlate with self-report of pain in humans (3, 4). Analyzing facial expressions and body language in animals poses unique challenges absent in human medicine, like data collection, establishing ground truth—that is, determining whether or not the animal is experiencing pain or distress, and navigating the vast array of morphological differences, shapes, and colors present within and across animal species (5, 6). Various scales for interpreting facial expressions in animals have been created in the past decade. The Mouse Grimace Scale (MGS) was the first facial grimace scale for animal pain assessment, developed from studies on emotional contagion in mice, and led to the creation of similar scales for other species, such as the Rat Grimace Scale (RGS) (7, 8). These scales, now developed for 11 species, have been used in various pain models, including surgical procedures and husbandry practices. Despite their usefulness, limitations include the fact that most of these pain scales were developed based on a restricted number of action units (AUs) retrieved from picture-based recognition patterns, as described in more details later.
Computational tools, especially those based on computer vision (CV), provide an attractive alternative. Automated Pain Recognition (APR) is an innovative technology that utilizes image sensors and pain algorithms that employ Artificial Intelligence (AI) techniques to recognize pain in individuals (9, 10). These systems are based on machine learning (ML) techniques to recognize and classify facial expressions associated with pain (11). Machine learning consists of training an algorithm to discern various categories or events (classes). Subsequently, this trained algorithm is utilized to identify categories or events within a new or unknown data set. The application of AI optimized the research on classification algorithms of ML, increasing recognition rates, computing speed and preventing system crashes.
Machine learning and AI can radically change how we recognize and treat pain in non-verbal patients, including animals, with an immense impact on veterinary medicine and animal welfare. By harnessing the power of ML algorithms, we can create sophisticated models that analyze various data inputs, not only facial expressions but also body posture and gesture (12), vocalizations (13), and physiological parameters, to accurately and objectively assess an animal's pain level. This approach will enhance our ability to provide timely and effective pain management, and it will be pivotal in minimizing suffering and improving the overall quality of life for animals under our care.
Therefore, this narrative review aims to focus on the impact of automation in the recognition of animal somatosensory emotions like pain and to provide an update on APR methodologies tested in the veterinary medical field, as well as their differences, advantages, and limitations to date.
2 Facial expression-based (grimace) scales for animal pain assessment
A grimace pain scale assesses animals' pain by evaluating changes in their facial expressions. It is developed through systematic observation and analysis of facial expressions exhibited by animals in response to pain-inducing stimuli. Researchers identify specific facial features associated with pain and create a coding system to quantify these responses objectively. The scale then undergoes validation to establish its reliability and sensitivity.
The MGS was the pioneering facial grimace scale for pain assessment developed for animals, emerging from investigations exploring the possibility of emotional contagion in mice (14). These studies exposed the capacity of mice to discern pain in their counterparts through subtle changes in body language and facial expressions after they were injected intraperitoneally with 0.9% acetic acid (7). Within a short span, the RGS followed suit, its inception marked by experiments conducted on appendicular inflammatory models and a laparotomy model (8, 15). Demonstrating features mirroring those of the MGS—such as orbital tightening, ear changes, and whisker alterations—the RGS exhibited comparable reliability and accuracy. Moreover, it showcased sensitivity to morphine and the ability to quantify pain stemming from inflammatory sources (8). Since their development, rodent grimace scales have been tested in several preclinical pain models, including post-laparotomy (16), post-vasectomy (17), post-thoracotomy (18). Following the initial publications, there has been a swift expansion in both the conversation and application of grimace scales. Grimace scales have been developed for 11 distinct species, including rodents, lagomorph (19), feline (20, 21), equine (22, 23), bovine (24), swine (25, 26), ovine (27, 28), ferrets (29), harbor seals (30), and donkeys (31, 32). As castration is considered one of the most common surgical procedures practiced by veterinarians, it is not surprising that several of these models were based on difference in behavior and posture before and after castration (22, 24, 26, 31, 33–35). However, other husbandry procedures have been used, like tail docking, ear-tagging and microchipping (25, 28, 30). A complete overview of the facial grimace scales developed to date and the painful stimulus used has been reported in Table 1.
These studies collectively share several common limitations. Primarily, a significant inconsistency exists in developing species-specific ethograms associated with pain. An ethogram is a descriptive inventory or catalog of all behaviors or actions exhibited by a particular species or group of animals under specific conditions. But many of these investigations were conducted before establishing a formal codification system for facial expressions in the relevant species, such as the Facial Action Coding System (FACS), which will be elaborated upon in the subsequent paragraph. A wide range of pain models has been employed across these studies, including experimental models (7, 8, 23, 29), clinical or husbandry procedures (24, 26, 31, 34, 35, 40) and observations of spontaneous pain (20, 21, 36–39). Notably, it has been demonstrated that the duration of the noxious stimulus affects the facial expression of pain (14). Langford et al. (7) showed that noxious stimuli lasting between 10 min and 4 h were most likely to elicit a “pain face.” Consequently, this would render most transient pain models (30) and chronic pain models (39, 41) inadequate for facial pain detection. Interesting ear notching did not evocate grimace in mice (42) but it did in rabbits (19). Furthermore, potential overlap between pain and other states (sleep, grooming, and illness) has been observed (43, 44). In many cases, animals were assessed both before and after procedures requiring general anesthesia (8, 22, 27, 29, 31). However, studies have shown that the facial expression of pain can remain altered for several hours after inhalant anesthesia in both experimental mice and rats (45, 46) and in horses (47). This effect likely holds for other animal species.
The collection of images for facial expression scoring lacked consistency across studies. Despite trained personnel being capable of regularly recording and evaluating animal pain intensity in clinical settings, continuous annotation still needs to be attainable (48). Many studies relied on static images, often arbitrarily extracted from videos of varying durations, or real-time scoring, with manual annotation performed by human researchers. This approach introduced the risk of bias and subjective judgment. Furthermore, researchers emphasized the necessity of using high-definition video cameras or still cameras to ensure optimal image quality (7, 22, 25, 27, 43) but to avoid the use of bright light or camera flashes (20). The development of the RGS coincided with the introduction of the Rodent Face Finder® free software, designed to streamline the conversion of videos into scorable photographs by capturing frames with optimal optical quality and head positioning (8). Similar approaches have been developed also for horses (49). Typically, images were then pre-processed, with cropping around the head and removal of the background being common practices. However, the impact of background on image interpretation remains untested (43). Subsequently, these still images were presented to blind observers with varying levels of experience to assess inter- and intra-rater variabilities. Notably, observer experience significantly impacted the ability to discern facial features (7, 28, 31, 39). Given animals' inability to verbalize pain and the variability in employed pain models, researchers have typically identified facial changes occurring in more than 25–50% of animals following a painful stimulus as indicative of pain (22, 24, 29). Alternatively, they have relied on the coding of pain AUs recognized by experts in human facial pain expression (7, 23). But it is known that human observers often categorize facial expressions based on emotion, which can influence the process of comparing expressions across different species (50).
Construct validity of the pain scale is typically assessed by comparing the scores of animals experiencing pain vs. those undergoing sham procedures and by reassessing the painful animal before and after treatment. However, in the existing literature these comparisons were often omitted due to ethical concerns with performing invasive veterinary procedures without analgesia (22). Dalla Costa et al. (22) found no differences in the Horse Grimace Scale (HGS) among horses undergoing castration under general anesthesia, regardless of receiving one or two doses of flunixin meglumine. Similarly, there were no differences in the Piglet Grimace Scale (PGS) scores between piglets castrated with and without receiving meloxicam (34) or piglets receiving buprenorphine injections whether undergoing castration or not (35). Even when PGS was refined through 3D landmark geometric morphometrics, neither the PGS nor 3D landmark-based geometric morphometrics were able to identify facial indicators of pain in piglets undergoing castration (51). These findings raise questions about the potential confounding effects of drugs and the reliability of the scale in assessing post-castration pain. While this is not substantiated by the current literature, it is also possible that expressions may not always be an accurate indicator of pain in animals or researchers did not identify the pain ethogram for the species yet.
While animals cannot communicate their pain perception directly, the criterion validity of a pain scale can be assessed by testing it against a gold standard. However, this validation method was rarely conducted in previous studies (21, 27, 39, 41). A pain scale's internal consistency measures its components' coherence. In pain assessment, a scale demonstrates internal consistency if it consistently yields similar scores for the same aspect of pain across its various items or questions. This ensures that all items reliably measure the exact dimension of pain. Internal consistency is typically assessed using statistical methods like Cronbach's α coefficient, with higher values indicating more robust agreement among scale items and more reliable pain measurement. However, internal consistency has been reported only for the Feline Grimace Scale (21). Inter- and intra-rater reliability assess the agreement among different raters (inter-rater reliability) or the same rater over multiple assessments (intra-rater reliability) when using the scale to evaluate pain. Inter-rater reliability ensures consistent results regardless of who administers the scale, ensuring validity and generalizability across different observers. Intra-rater reliability confirms the stability and consistency of the scale's measurements over time, indicating that a rater's assessments are not influenced by variability or bias. The Intraclass Correlation Coefficient (ICC) is widely used to measure reliability, with values <0.50 indicating poor agreement, between 0.50 and 0.75 indicating moderate agreement, between 0.75 and 0.90 indicating good agreement, and above 0.90 indicating excellent agreement (52). Inter-rater ICC values for current facial expression pain scales ranged between 0.57 (26) and 0.92 (27), while intra-rater ICC ranged between 0.64 (24) and 0.90 (21), with considerable variability across facial features. But presenting good rater agreement on a given behavior does not mean that the behavior actually measures a given emotion. Another significant limitation of existing facial pain scales is the need for a cutoff value for treatment determination. van Loon and Van Dierendonck (36) reported that the EQUUS FAP had sensitivity and sensibility for distinguishing colic from no-colic of 87.5 and 88% using a cut-off value of 4 in a scale 0–18, but only of 30 and 64.3% for distinguishing surgical and medical colic with a cut-off at 6. Häger et al. (27) and McLennan and Mahmoud (53) both reported a discrimination accuracy below 70% using two different facial pain scales developed for sheep, denouncing a high number of false positive results and highlighting the need for further refinement and standardization in this area.
3 Facial Action Coding System
The gold standard for objectively assessing changes in facial expressions in human emotion research is the FACS, first published almost half a century ago (54). FACS is a comprehensive, anatomically based system that taxonomizes all visible human facial movements (55, 56). In FACS, the authors assign numbers to refer to the appearance changes associated with 33 facial muscle contractions to each specific facial movement, termed AUs. Each AU is linked to mimetic muscles innervated by the facial nerve and characterized by corresponding changes in facial appearance. Additionally, the system introduced 25 more general head/eye movements termed Action Descriptors (AD), representing broader movements from non-mimetic muscles, which could impact AU identification. Recognizing the interplay between AUs and ADs is emphasized, as their concurrent presence could modify the visual expression of individual movements. The FACS manual offers guidelines for scoring these AUs, supported by a collection of photographs and illustrations for reference. The FACS system revolutionized human research based on facial expression interpretation, finding extensive application in psychology, sociology, and communication. It enabled the objective and systematic recognition of individual facial movements based on facial anatomy and steered the field away from subjective interpretations of visual displays known for their unreliability. Following the FACS approach, researchers have developed the same system for non-human primates, including orangutans [Pongo spp: OrangFACS (55)], chimpanzees chimpanzees [Pan troglodytes: ChimpFACS (56)], rhesus macaques [Macaca mulatta: MaqFACS (57, 58)], gibbons [Hylobatids, GibbonFACS (59)], marmosets (60), and domesticated mammals such as horses [Equus caballlus: EquiFACS (61, 62)], dogs (Canis familiaris: DogFACS) (63), and cats (Felis catus: CatFACS) (64).
Developing species-specific AnimalFACS involved identifying and documenting every potential facial movement of the species based on observable changes in appearance, consistent with the FACS terminology. Subsequently, the muscular foundation of each movement was confirmed through rigorous anatomical studies (56, 61, 63). This extensive work has interestingly unveiled phylogenetic similarities across species, with those already analyzed for FACS demonstrating a shared muscular foundation of at least 47% of their facial muscles (65). While species may share similar anatomical structures, this correspondence does not invariably translate into analogous facial movements. Specific muscles may be implicated in multiple AUs, while others may exhibit infrequent use, complicating the relationship between anatomy and expression (65). For a more detailed description of all the AUs identified in the different species, the reader is referred to Waller et al. (65). But, while FACS is generally considered reliable for gauging human perception due to the presumed alignment between facial expression production and interpretation, its applicability to non-human animals may be less precise, as third party evaluation is always required. Therefore, it's vital to approach its application cautiously and gather empirical data to ascertain how animals respond to stimuli.
Despite the growing interest in facial expression analysis for evaluating pain and emotion, only a few animal studies applied AnimalFACS. Among small animal species, the FACS system has been scarcely used. In dogs and cats, FACS has been used more commonly for emotion interpretation than specifically for pain determination (66–68). In one study, 932 images from 29 cats undergoing ovariohysterectomy were extracted and manually annotated using 48 landmarks selected according to CatFACS criteria (69). A significant relationship was found between pain-associated Principal Components, which capture facial shape variations, and the UNESP-Botucatu Multidimensional Composite Pain Scale tool (69). However, an intrinsic bias of the study was that the first postoperative assessment, prior to administration of analgesia, was recorded between 30 min and 1 h after general anesthesia, and the role of general anesthesia on facial expression cannot be excluded as it has been previously discussed. A groundbreaking methodology for investigating the facial expressions of ridden horses, known as Facial Expressions of Ridden Horses (FEReq) (70, 71), was developed by integrating species-specific ethograms from previous studies (22, 23) with components of the EquiFACS codification system (61). This ethogram represented a pioneering effort in characterizing changes in facial expressions among ridden horses, demonstrating reasonable consistency across diverse professional backgrounds post-adaptation and training. Although initially limited to analyzing still photographs capturing singular moments, the ethogram was subsequently enhanced with additional markers for assessing general body language and behavior in ridden horses (72). Despite no observed correlation between this improved Ridden Horse Pain Ethogram (RHpE) score and maximum lameness grade before diagnostic anesthesia (Spearman's rho = 0.09, P = 0.262) (73), the scale has proven effective in detecting musculoskeletal pain in competitively ridden horses (74, 75). These studies uncovered variations in consistency across horse facial features, particularly noting the eye and muzzle as displaying the least reliability. This stands in contrast to findings by Rashid et al. (62), who repurposed data from Gleerup et al. (23) to employ EquiFACS in describing facial features in pain-related videos. The group suggested that inner brow raiser (AU101), half blink (AU47), chin raiser (AU17), ear rotator (EAD104), eye white increase (AD1), and nostril dilator (AD38) were frequently linked with pain. Moreover, these findings were echoed by a recent study by Ask et al. (76), investigating pain indicators in horses with experimentally induced orthopedic pain. Employing the Composite Orthopedic Pain Scale (77) as the gold standard, the group identified numerous lip and eye-related AUs and ADs as robust predictors of pain. Noteworthy indicators included frequency and duration of eye closure (AU143), duration of blink (AU145), upper lid raiser (AU5), duration of lower jaw thrust (AD29), frequency and duration of lower lip relax (AD160), frequency of lower lip depressor (AU16), frequency of upper lip raiser (AU10), frequency and duration of AU17, duration of lip presser (AU24), frequency and duration of AD38, and frequency and duration of lips part (AU25), among others. Additionally, AU16, AU25, AU47, single ear forward (SEAD101), and EAD104 co-occurred more frequently in horses experiencing orthopedic pain. The study by Rashid et al. (62) also noted an interesting discrepancy in pain detection rates. In still images or video segments lasting 0.04 s, the likelihood of detecting more than three pain AUs was extremely low, contrasting with higher detection rates with a 5 s observation window. This may be explained by the fact that 75% of pain-related AUs in horses lasts between 0.3 and 0.7 s (76). This finding underscores the potential value of using video footage over randomly selected images for pain assessment. However, it's essential to acknowledge limitations in these studies, such as the small number of experimental horses used to build the models and the presumption of pain based solely on evaluations by clinically experienced observers, potentially overlooking influences of stress, tiredness and malaise (44).
One of the limitations of AnimalFACS consist in the limited availability across species and the reliance on manual annotation, necessitating rigorous human training to ensure acceptable inter-rater reliability (78, 79). Debates arose regarding distinctive individual differences, encompassing variations in muscle presence, size, symmetry, disparities in adipose tissue distribution, and even inherent facial asymmetry (65, 80). Notably, present studies using AnimalFACS are limited to quantifying the number of AUs, their combinations, and their temporal duration within a confined observation period (62, 72). However, this approach falls short of capturing the intricate complexity of facial movements. Another fundamental limitation of FACS-based systems is their failure to account for the dynamic shifts in movement or posture that often accompany and enrich facial expressions. So, some studies have assessed behavioral indicators such as changes in consumption behaviors (time activity budgets for eating, drinking, or sleeping, etc.) (81–83); anticipatory behaviors (84), affiliative behavior (85), agonistic behaviors, and displacement behaviors, amongst others (86).
4 Automated pain recognition
Automated Pain Recognition is a cutting-edge technology aiming to develop objective, standardized, and generalizable instruments for pain assessment in numerous clinical contexts. This innovative approach has the potential to significantly enhance the pain recognition process. Automated Pain Recognition leverages image sensors and pain algorithms, powered by AI techniques, to identify pain in individuals (9, 10). AI, a field encompassing a broad range of symbolic and statistical approaches to learning and reasoning, mimics various aspects of human brain function. Data-driven AI models, such as those used in APR, can overcome the limitations of subjective pain evaluation. Machine learning, CV, fuzzy logic (FL), and natural language processing (NLP) are commonly considered subsets of AI. However, with technological advancements and interdisciplinary research, the boundaries between these subsets often blur. Machine learning, a branch of AI, enables systems to learn and improve their performance through experience without explicit programming. It involves training a computer model on a dataset, allowing it to make predictions or decisions independently. Automated Pain Recognition research has focused on discerning pain and pain intensity within clinical settings (87) and assessing responses to quantitative sensory testing in preclinical research (88, 89). The following paragraphs will briefly outline and summarize the steps involved in APR.
4.1 Data collection
The initial step toward implementing APR involves data collection, a significant challenge in the veterinary field due to the scarcity of available datasets (90). Animals exhibit considerable variability even within the same species, influenced by factors such as breed, age, sex, and neuter status, that may affect the morphometry of the face, especially in adult males (91). These variables can impact the pain-related facial information extracted from images (6, 92, 93). This variability, however, can enhance the learning process of deep learning (DL) models. Exposure to diverse examples and scenarios allows models trained on a broad spectrum of data to generalize well to unseen examples, improving performance in real-world applications. Additionally, variability aids in acquiring robust features applicable across different contexts. With the availability of high-definition cameras and the relatively low demand for image or video quality in CV, recording has become less problematic compared to the past (49). Studies suggest that resolutions of 224 × 224 pixels and frame rates of 25 FPS are sufficient for processing images and videos in modern CV systems (49). Multicamera setups are ideal, especially for coding both sides of the face, as required in laterality studies or to avoid invisibility. Different animal species pose unique challenges. Laboratory animals are usually confined to a limited environment, allowing more control over data acquisition and video recording quality (89, 94, 95). Horses can be manually restrained or confined in a stall (96). Data acquisition for farm animals often occurs in open spaces or farms with uncontrolled light conditions (53, 97).
4.2 Data labeling
The absence of verbal communication in veterinary APR introduces a unique challenge in establishing a ground truth label of pain or emotional state. Unlike human medicine, where self-reporting of pain is feasible, veterinary APR requires third-party assessment of the pain status, preferably utilizing a validated pain scale, but commonly not (Table 2). This has led to the categorization of pain labeling methods in animal APR into behavior-based or stimulus-based annotations (90). The former relies solely on observed behaviors and is typically assessed by human experts (5, 6, 97, 99, 104–106). In contrast, the latter determines the ground truth based on whether the data were recorded during an ongoing stimulus or not (5, 10, 49, 76, 94–96, 99, 100, 107–109). Stimulus-based annotations enable recording the same animal under pain and no pain conditions and offer a potential solution to the challenge of variability in pain perceptions across individuals (110). Therefore, CV and ML methods must acknowledge the inherent bias in their algorithms until a definitive marker for pain is identified.
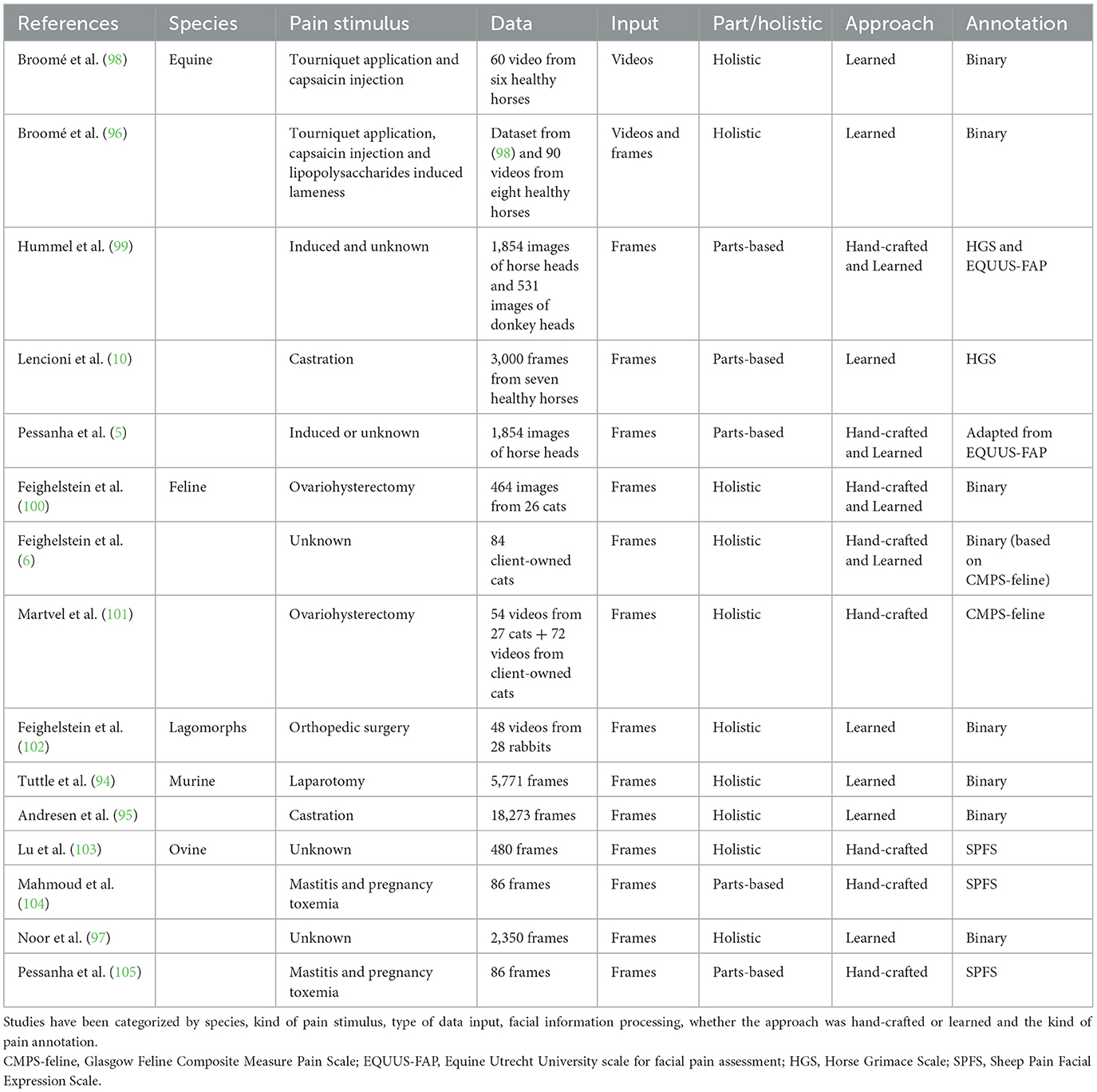
Table 2. Overview of datasets featuring facial expressions of pain for automated animal pain assessment to date.
4.3 Data analysis
Computer vision-based methods operate using data in the form of images or image sequences (videos). This suggests that the system can utilize single frames, aggregate frames (10) or incorporate spatiotemporal representations to account for temporality (94, 98, 105). Utilizing single frames offers greater control and facilitates explainability, although it may result in information loss. Researchers demonstrated that the likelihood of observing more than three pain AUs was negligible in still images extracted from videos of horses undergoing moderate experimental nociceptive stimulus (62). On the other hand, based on Martvel et al. (101), different frame extraction rates may affect the accuracy of the results. Preliminary results in mice (94), horses (98), sheep (105), and cats (101) suggest that extracting spatiotemporal patterns from video data may increase the performance of the model. However, working with videos rather than single-frame input requires substantial computational resources.
The data processing pipeline is developed after the images are collected and the input is either images or videos. The output is typically pain classification, which can be binary pain/no pain or multi-class degree assessment. Often, outputs based on grimace pain scale taxonomy encompass at least three scales [pain not present (0), pain moderately present (1), or pain present (2)] (10, 103). The pipeline can encompass multiple steps and may analyze the entire body or face or focus on specific parts. These two approaches, differing in processing facial information, have been defined as parts-based and holistic methods. For instance, Hummel et al. (99) cropped the equine face based on several Regions of Interest (ROIs); the eyes, ears, nostrils, and mouth, respectively, and analyzed them with HOG (Histogram of Oriented Gradients), Local Binary Pattern (LBP), Scale Invariant Feature Transform (SIFT), and DL approach using VGG-16 Convolutional Neural Network (CNN). Similarly, Lencioni et al. (10) employed a parts-based approach in annotating 3,000 images from seven horses of similar breeds and ages undergoing castration. They utilized the HGS (22), where the six parameters were grouped into three different facial parts: ears, eyes, and muzzle. Subsequently, three pain classifier models based on CNN architecture were developed. The outputs of these models were then fused using a fully connected network for an overall pain classification. Recent research employing explainable AI methods to investigate different regions of cat faces suggested that features related to the ears may be the least important (111). In contrast, those associated with the mouth movement were considered the most crucial (6, 49). Similarly, Lu et al. (103) have developed a multilevel pipeline to assess pain in sheep, utilizing the Sheep Facial Expression Pain Scale (39). The authors divided the sheep's face into regions, including eyes, ears, and nose, with further subdivision of the ears into left and right. Symmetric features such as eyes and ears were scored separately and then averaged, while scores for all three facial features (ears, eyes, nose) were averaged again to derive the overall pain score. The task of automatically identifying and localizing specific points or features on an animal's face, such as the eyes, nose, mouth corners, etc., known in CV as recognition of key facial points, poses the initial challenge due to limited datasets in animals (95). Researchers have proposed adapting animal training data to a pre-trained human key point detector to address this issue. The approach involved morphing animal faces into human faces and fine-tuning a CNN developed for human key point recognition. Surprisingly, this approach has demonstrated promising performance in both equine and ovine faces (112).
4.4 Hand-crafted vs. deep learning
Automated Pain Recognition identifies, understands, and enhances image pain features. Two main approaches have been used for feature extraction.
4.4.1 Hand-crafted features extraction
Before the advent of DL, classical ML relied on hand-crafted features (90). The process involves extracting characteristics from the data using previous knowledge to capture pain-related patterns with facial or bodily landmarks, grimace scale elements, or pose representations. For example, Blumrosen et al. (113) studied four fundamental facial movements to recognize facial actions in macaques: neutral expression, lip smacking, chewing, and random mouth opening. They used unsupervised learning, which does not require manually labeling or annotating the data. In their approach, they utilized eigenfaces to extract features from facial images. Eigenfaces use a mathematical method called Principal Component Analysis (PCA) to capture the statistical patterns present in facial images. Another standard method is the landmark-based (LM-based) approach, which identifies pain-related AUs through manual annotation (7, 10, 94, 103). It provides a mathematical representation of previous findings by human experts concerning certain facial expressions. The system requires preliminary efforts to detect and locate the animal face in an image or video clip and to detect individual AUs. Face detection and alignment are achieved by detecting key facial points, which are then transformed into multi-region vectors and fed to a multi-layer perceptron neural network (MLP). For example, Andersen et al. (49) trained individual classifiers to detect 31 AUs, including ADs and ear EADs, in 20,000 EquiFACS-labeled short video clips after cropping the images around a pre-defined ROI to help the classifier focus on the correct anatomical region. But the model did not work for the ear action descriptors. The authors attributed this discrepancy to the many different positions possible for ears, suggesting that ears' position should be examined in spatiotemporal data acquisition (49, 90). Similarly, Feighelstein et al. (100) utilized 48 facial landmarks selected based on the CatFACS and manually annotated for developing their automated model. Landmark-based approaches are by their nature better able to directly measure and thus better account for morphological variability. However, the downside of this route is the resource and effort needed for landmark annotation, given that this requires manual completion (114).
4.4.2 Deep learning approach
Deep Learning approaches are gaining popularity in APR due to their reduced need for annotation and manual feature crafting. Unlike LM-based methods, DL is less sensitive to facial alignment (100), although the accuracy of the models improves with data cleaning (102). Deep learning trains artificial neural networks with many layers to automatically extract hierarchical features from vast datasets like video data. Convolutional Neural Networks (CNNs) are particularly effective for image processing tasks like classification and object recognition, offering superior performance by mapping individual inputs to single outputs. Deep learning relies heavily on large volumes of video data for training (6). Continual advancements in DL methods for APR are expanding the possibilities in the field. CNNs, inspired by the functioning of the retina, consist of various layers, including convolutional layers for feature detection, non-linearity layers to introduce non-linearity, and pooling layers for down-sampling parameters. This architecture culminates in a fully connected layer for final processing, where each node in the output layer connects directly to a node in the previous layer. Among the diverse CNN architectures, the Visual Geometry Group (VGG) 16 architecture, with its 16 convolutional layers, each equipped with 3 × 3 filters, is particularly notable for its extensive utilization in CV applications. Other advanced neural networks, such as deep residual networks (ResNets), enable the handling of deeper architectures and improved performance (95, 100, 102). These advancements in DL methods have equipped researchers and practitioners with more powerful tools for APR. It is crucial to emphasize the significance of large and diverse datasets in DL methods for APR. While DL methods are often effective, they frequently lack interpretability, which poses a challenge for humans to comprehend their decision-making process.
Building upon the work of Finka et al. (69), Feighelstein et al. (100) explored both LM-based and DL methods in APR for cats, achieving comparable accuracies of around 72%. However, DL approaches faced challenges with highly homogeneous datasets, which affected their performance. The model showed improvement when applied to a more diverse population. A similar limitation was observed by Lencioni et al. (10), who extracted 3,000 frames from seven horses of similar breed and age to classify pain following a painful stimulus and general anesthesia. Using CNN-based individual training models for each facial part, they achieved an accuracy of 90.3% for the ears, 65.5% for the eyes, and 74.5% for the mouth and nostrils, and an overall accuracy of 75.8%. This underscores the need for diverse datasets to enhance the performance of DL methods in APR. When Feighelstein et al. (102) used a DL approach for recognizing pain in 28 rabbits undergoing an orthopedic procedure, the initial “naïve” model trained on all frames achieved an accuracy of over 77%. The performance improved to over 87% when a frame selection method was applied to reduce noise in the dataset (102). Another notable DL model is the deep recurrent video model used by Broomé et al. (96, 98), which utilizes a ConvLSTM layer to analyze spatial and temporal features simultaneously, yielding better results in spatiotemporal representations. Steagall et al. (106) and Martvel et al. (114) introduced a landmark detection CNN-based model to predict facial landmark positions and pain scores based on the manually annotated FGS.
4.5 Limitations and downfalls in animal APR
Data imbalance is a significant challenge in both classic ML and DL methods. This issue, as highlighted by Broomé et al. (90), occurs when they are fewer instances of one class compared to another, potentially skewing the model accuracy, especially in extreme categories. In the case of animal pain recognition, there are often fewer instances of animals in pain compared to non-painful animals (97, 98). The use of data augmentation techniques, such as synthesizing additional data using 3D models and generative AI (5) has been proposed to address this imbalance. However, the highly individualized nature of pain perception and expression in animals may limit the clinical value of these techniques in animal pain recognition.
Overfitting and underfitting are frequently encountered problems in ML. Overfitting happens when a model excessively learns from the training data, resulting in inadequate performance when applied to new data. On the other hand, underfitting occurs when a model does not perform well even on the training set. Cross-validation techniques mitigate these problems by splitting the data into training, validation, and testing sets. For smaller sample sizes, ensuring that each subject appears in only one part of the data (training, validation, or testing) can be beneficial (6, 102). In DL, it is crucial to reserve a fully held-out test set comprising data from subjects not seen during training to ensure unbiased evaluation. Techniques like leave-one-subject-out cross-validation can help reduce bias by rotating subjects between the training and testing sets (96, 100). Additionally, when training DL models from scratch, the initial setup can be influenced by a random number called a “seed.” Different seeds can lead to slightly different results each time the model is trained. To ensure robustness, training and testing are often repeated with different random seeds, and the outcomes are averaged to minimize the impact of random variations. Addressing data imbalance, overfitting, and underfitting is not just a choice but a necessity for improving the accuracy and robustness of ML and DL models in applications such as animal pain recognition. It is a crucial step that cannot be overlooked.
5 Discussion
This narrative review aimed to offer a comprehensive journey through the progression of research on recognizing facial expressions of pain in animals. It began with the rapid advancement of grimace pain scales, moved through the refinement of FACS for various animal species, and culminated in APR. Although APR extends beyond facial cues (98, 101), existing studies' predominant focus has been analyzing pain AUs in datasets crafted through prior facial expression research and annotation.
Pessanha et al. (5) underscored several significant challenges encountered in detecting APR in animals. The first among these challenges is the scarcity of available datasets, a notable contrast to the abundance of databases in the human domain (115). Very few current datasets have been created specifically for CV and APR studies. The majority of researchers have out-sourced their dataset from previous studies (6, 84, 96, 98, 99, 101, 104, 105), with the significant advantage was that most of these dataset were already annotated for pain AUs. However, as highlighted, most of the previously published pain scales based on facial expressions were based on unspecified or artificially created ethograms and they did not undergo complete validation, except for Evangelista et al. (21). Most interestingly, they were developed before or independently from the development of the AnimalFACS dataset for the species. While agreement was often found for many AUs developed before and after AnimalFACS (62, 76), this issue may introduce inherent bias. One solution proposed for overcoming the scarcity of data is data augmentation (5, 100). However, one of the primary ethical concerns is the integrity and representativeness of the augmented data. Augmented data should accurately represent real-world variations, and care should be taken to ensure that the augmented data does not lead to misinterpretations that could result in harm or unnecessary interventions (116). To address the issue of small datasets, open access to datasets and sharing between researchers is crucial. Fairly implementing AI in veterinary care requires integrating inclusivity, openness, and trust principles in biomedical datasets by design. The concept of openly sharing multiple facets of the research process—including data, methods, and results—under terms that allow reuse, redistribution, and reproduction of all findings has given birth to open science, a practice strongly supported by several institutions and funding agencies (49, 117). Secondly, animals may have much more significant facial texture and morphology variation than humans. While initially perceived as a challenge, this may be advantageous when employing a DL approach. Finally and foremost, a significant limitation in animal APR is the need for consistent ground truth. Unlike in humans, where self-reporting of the internal affective state is commonly used, there is no verbal basis for establishing a ground truth label of pain or emotional state in animals. Consequently, animal pain detection heavily relies on third-party (human expert) interpretation, introducing intrinsic bias that cannot be bypassed entirely. One possible strategy for establishing ground truth involves designing or timing the experimental setup to induce pain. However, since pain is a subjective experience, this approach may not eliminate bias. Additionally, the type and duration of pain need to be researched further, as there are postulations about differences in facial expressions of pain between acute nociceptive and chronic pain and on the effects of general anesthesia (14, 47, 95). These hypotheses, for example, could be tested to improve the understanding and detection of pain in animals. Currently, the best way to address this problem is by using fully validated pain scales to discriminate the pain status.
In conclusion, the advancement of animal APR has immense potential for assessing and treating animal pain. However, it requires addressing data scarcity, ensuring the ethical use of augmented data, and developing consistent and validated ground truth assessments. Open science practices and collaboration will be crucial in overcoming these challenges, ultimately improving the welfare of animals in research and clinical settings.
Author contributions
LC: Conceptualization, Data curation, Funding acquisition, Investigation, Resources, Writing – original draft, Writing – review & editing. AG: Investigation, Methodology, Writing – original draft, Writing – review & editing. GC: Investigation, Methodology, Writing – original draft, Writing – review & editing.
Funding
The author(s) declare financial support was received for the research, authorship, and/or publication of this article. The project was partially funded by the International Veterinary Academy for Pain Management (PRO00054545).
Conflict of interest
The authors declare that the research was conducted in the absence of any commercial or financial relationships that could be construed as a potential conflict of interest.
Publisher's note
All claims expressed in this article are solely those of the authors and do not necessarily represent those of their affiliated organizations, or those of the publisher, the editors and the reviewers. Any product that may be evaluated in this article, or claim that may be made by its manufacturer, is not guaranteed or endorsed by the publisher.
References
1. Ferretti V, Papaleo F. Understanding others: emotion recognition in humans and other animals. Genes Brain Behav. (2019) 18:e12544. doi: 10.1111/gbb.12544
2. Williams AC. Facial expression of pain: an evolutionary account. Behav Brain Sci. (2002) 25:439–55. doi: 10.1017/S0140525X02000080
3. Kappesser J. The facial expression of pain in humans considered from a social perspective. Philos Trans R Soc Lond B Biol Sci. (2019) 374:20190284. doi: 10.1098/rstb.2019.0284
4. Chambers CT, Mogil JS. Ontogeny and phylogeny of facial expression of pain. Pain. (2015) 156:133. doi: 10.1097/j.pain.0000000000000133
5. Pessanha F, Ali Salah A, van Loon T, Veltkamp R. Facial image-based automatic assessment of equine pain. IEEE Trans Affect Comput. (2023) 14:2064–76. doi: 10.1109/TAFFC.2022.3177639
6. Feighelstein M, Henze L, Meller S, Shimshoni I, Hermoni B, Berko M, et al. Explainable automated pain recognition in cats. Sci Rep. (2023) 13:8973. doi: 10.1038/s41598-023-35846-6
7. Langford DJ, Bailey AL, Chanda ML, Clarke SE, Drummond TE, Echols S, et al. Coding of facial expressions of pain in the laboratory mouse. Nat Methods. (2010) 7:447–9. doi: 10.1038/nmeth.1455
8. Sotocinal SG, Sorge RE, Zaloum A, Tuttle AH, Martin LJ, Wieskopf JS, et al. The rat grimace scale: a partially automated method for quantifying pain in the laboratory rat via facial expressions. Mol Pain. (2011) 7:55. doi: 10.1186/1744-8069-7-55
9. Walter S, Gruss S, Frisch S, Liter J, Jerg-Bretzke L, Zujalovic B, et al. “what about automated pain recognition for routine clinical use?” a survey of physicians and nursing staff on expectations, requirements, and acceptance. Front Med. (2020) 7:566278. doi: 10.3389/fmed.2020.566278
10. Lencioni GC, de Sousa RV, de Souza Sardinha EJ, Corrêa RR, Zanella AJ. Pain assessment in horses using automatic facial expression recognition through deep learning-based modeling. PLoS ONE. (2021) 16:e0258672. doi: 10.1371/journal.pone.0258672
11. Werner P, Al-Hamadi A, Limbrecht-Ecklundt K, Walter S, Gruss S, Traue HC. Automatic pain assessment with facial activity descriptors. IEEE Transact Affect Comp. (2016) 8:286–99. doi: 10.1109/TAFFC.2016.2537327
12. Olugbade TA, Bianchi-Berthouze N, Marquardt N, Williams AC, editors. Pain level recognition using kinematics and muscle activity for physical rehabilitation in chronic pain. In: 2015 International Conference on Affective Computing and Intelligent Interaction (ACII). Xi'an: IEEE (2015).
13. Thiam P, Kessler V, Amirian M, Bellmann P, Layher G, Zhang Y, et al. Multi-modal pain intensity recognition based on the senseemotion database. IEEE Transact Affect Comp. (2019) 12:743–60. doi: 10.1109/TAFFC.2019.2892090
14. Mogil JS, Pang DSJ, Silva Dutra GG, Chambers CT. The development and use of facial grimace scales for pain measurement in animals. Neurosci Biobehav Rev. (2020) 116:480–93. doi: 10.1016/j.neubiorev.2020.07.013
15. Oliver V, De Rantere D, Ritchie R, Chisholm J, Hecker KG, Pang DS. Psychometric assessment of the rat grimace scale and development of an analgesic intervention score. PLoS ONE. (2014) 9:e97882. doi: 10.1371/journal.pone.0097882
16. Matsumiya LC, Sorge RE, Sotocinal SG, Tabaka JM, Wieskopf JS, Zaloum A, et al. Using the Mouse Grimace Scale to reevaluate the efficacy of postoperative analgesics in laboratory mice. J Am Assoc Lab Anim Sci. (2012) 51:42–9.
17. Leach MC, Klaus K, Miller AL, Scotto di Perrotolo M, Sotocinal SG, Flecknell PA. the assessment of post-vasectomy pain in mice using behaviour and the Mouse Grimace Scale. PLoS ONE. (2012) 7:e35656. doi: 10.1371/journal.pone.0035656
18. Faller KM, McAndrew DJ, Schneider JE, Lygate CA. Refinement of analgesia following thoracotomy and experimental myocardial infarction using the Mouse Grimace Scale. Exp Physiol. (2015) 100:164–72. doi: 10.1113/expphysiol.2014.083139
19. Keating SC, Thomas AA, Flecknell PA, Leach MC. Evaluation of emla cream for preventing pain during tattooing of rabbits: changes in physiological, behavioural and facial expression responses. PLoS ONE. (2012) 7:e44437. doi: 10.1371/journal.pone.0044437
20. Holden E, Calvo G, Collins M, Bell A, Reid J, Scott E, et al. Evaluation of facial expression in acute pain in cats. J Small Anim Pract. (2014) 55:615–21. doi: 10.1111/jsap.12283
21. Evangelista MC, Watanabe R, Leung VSY, Monteiro BP, O'Toole E, Pang DSJ, et al. Facial expressions of pain in cats: the development and validation of a Feline Grimace Scale. Sci Rep. (2019) 9:19128. doi: 10.1038/s41598-019-55693-8
22. Dalla Costa E, Minero M, Lebelt D, Stucke D, Canali E, Leach MC. Development of the Horse Grimace Scale (Hgs) as a pain assessment tool in horses undergoing routine castration. PLoS ONE. (2014) 9:e92281. doi: 10.1371/journal.pone.0092281
23. Gleerup KB, Forkman B, Lindegaard C, Andersen PH. An equine pain face. Vet Anaesth Analg. (2015) 42:103–14. doi: 10.1111/vaa.12212
24. Yamada PH, Codognoto VM, de Ruediger FR, Trindade PHE, da Silva KM, Rizzoto G, et al. Pain assessment based on facial expression of bulls during castration. Appl Anim Behav Sci. (2021) 236:105258. doi: 10.1016/j.applanim.2021.105258
25. Di Giminiani P, Brierley VL, Scollo A, Gottardo F, Malcolm EM, Edwards SA, et al. The assessment of facial expressions in piglets undergoing tail docking and castration: toward the development of the Piglet Grimace Scale. Front Vet Sci. (2016) 3:100. doi: 10.3389/fvets.2016.00100
26. Viscardi AV, Hunniford M, Lawlis P, Leach M, Turner PV. Development of a Piglet Grimace Scale to evaluate piglet pain using facial expressions following castration and tail docking: a pilot study. Front Vet Sci. (2017) 4:51. doi: 10.3389/fvets.2017.00051
27. Häger C, Biernot S, Buettner M, Glage S, Keubler LM, Held N, et al. The Sheep Grimace Scale as an indicator of post-operative distress and pain in laboratory sheep. PLoS ONE. (2017) 12:e0175839. doi: 10.1371/journal.pone.0175839
28. Guesgen MJ, Beausoleil NJ, Leach M, Minot EO, Stewart M, Stafford KJ. Coding and quantification of a facial expression for pain in lambs. Behav Processes. (2016) 132:49–56. doi: 10.1016/j.beproc.2016.09.010
29. Reijgwart ML, Schoemaker NJ, Pascuzzo R, Leach MC, Stodel M, de Nies L, et al. The composition and initial evaluation of a Grimace Scale in ferrets after surgical implantation of a telemetry probe. PLoS ONE. (2017) 12:e0187986. doi: 10.1371/journal.pone.0187986
30. MacRae AM, Makowska IJ, Fraser D. Initial evaluation of facial expressions and behaviours of harbour seal pups (Phoca Vitulina) in response to tagging and microchipping. Appl Anim Behav Sci. (2018) 205:167–74. doi: 10.1016/j.applanim.2018.05.001
31. Orth EK, Navas Gonzalez FJ, Iglesias Pastrana C, Berger JM, Jeune SSL, Davis EW, et al. Development of a Donkey Grimace Scale to recognize pain in donkeys (Equus Asinus) post castration. Animals. (2020) 10:1411. doi: 10.3390/ani10081411
32. van Dierendonck MC, Burden FA, Rickards K, van Loon J. Monitoring acute pain in donkeys with the Equine Utrecht University Scale for Donkeys Composite Pain Assessment (EQUUS-Donkey-Compass) and the Equine Utrecht University Scale for Donkey Facial Assessment of Pain (EQUUS-Donkey-Fap). Animals. (2020) 10:354. doi: 10.3390/ani10020354
33. Dalla Costa E, Dai F, Lecchi C, Ambrogi F, Lebelt D, Stucke D, et al. Towards an improved pain assessment in castrated horses using facial expressions (Hgs) and circulating mirnas. Vet Rec. (2021) 188:e82. doi: 10.1002/vetr.82
34. Viscardi AV, Turner PV. Use of meloxicam or ketoprofen for piglet pain control following surgical castration. Front Vet Sci. (2018) 5:299. doi: 10.3389/fvets.2018.00299
35. Viscardi AV, Turner PV. Efficacy of buprenorphine for management of surgical castration pain in piglets. BMC Vet Res. (2018) 14:1–12. doi: 10.1186/s12917-018-1643-5
36. van Loon JP, Van Dierendonck MC. Monitoring acute equine visceral pain with the Equine Utrecht University Scale for Composite Pain Assessment (EQUUS-Compass) and the Equine Utrecht University Scale for Facial Assessment Of Pain (EQUUS-Fap): a scale-construction study. Vet J. (2015) 206:356–64. doi: 10.1016/j.tvjl.2015.08.023
37. VanDierendonck MC, van Loon JP. Monitoring acute equine visceral pain with the Equine Utrecht University Scale for Composite Pain Assessment (EQUUS-Compass) and the Equine Utrecht University Scale for Facial Assessment of Pain (EQUUS-Fap): a validation study. Vet J. (2016) 216:175–7. doi: 10.1016/j.tvjl.2016.08.004
38. Dalla Costa E, Bracci D, Dai F, Lebelt D, Minero M. Do different emotional states affect the horse Grimace Scale Score? A pilot study. J Eq Vet Sci. (2017) 54:114–7. doi: 10.1016/j.jevs.2017.03.221
39. McLennan KM, Rebelo CJB, Corke MJ, Holmes MA, Leach MC, Constantino-Casas F. Development of a facial expression scale using footrot and mastitis as models of pain in sheep. Appl Anim Behav Sci. (2016) 176:19–26. doi: 10.1016/j.applanim.2016.01.007
40. de Oliveira FA, Luna SP, do Amaral JB, Rodrigues KA, Sant'Anna AC, Daolio M, et al. Validation of the UNESP-Botucatu Unidimensional Composite Pain Scale for assessing postoperative pain in cattle. BMC Vet Res. (2014) 10:200. doi: 10.1186/s12917-014-0200-0
41. Dalla Costa E, Stucke D, Dai F, Minero M, Leach MC, Lebelt D. Using the Horse Grimace Scale (Hgs) to assess pain associated with acute laminitis in horses (Equus caballus). Animals. (2016) 6:47. doi: 10.3390/ani6080047
42. Miller A, Leach M. Using the Mouse Grimace Scale to assess pain associated with routine ear notching and the effect of analgesia in laboratory mice. Lab Anim. (2015) 49:117–20. doi: 10.1177/0023677214559084
43. McLennan KM, Miller AL, Dalla Costa E, Stucke D, Corke MJ, Broom DM, et al. Conceptual and methodological issues relating to pain assessment in mammals: the development and utilisation of Pain Facial Expression Scales. Appl Anim Behav Sci. (2019) 217:1–15. doi: 10.1016/j.applanim.2019.06.001
44. Trindade PHE, Hartmann E, Keeling LJ, Andersen PH, Ferraz GC, Paranhos da Costa MJR. Effect of work on body language of ranch horses in Brazil. PLoS ONE. (2020) 15:e0228130. doi: 10.1371/journal.pone.0228130
45. Miller AL, Golledge HD, Leach MC. The influence of isoflurane anaesthesia on the Rat Grimace Scale. PLoS ONE. (2016) 11:e0166652. doi: 10.1371/journal.pone.0166652
46. Miller A, Kitson G, Skalkoyannis B, Leach M. The effect of isoflurane anaesthesia and buprenorphine on the Mouse Grimace Scale and Behaviour in Cba and Dba/2 Mice. Appl Anim Behav Sci. (2015) 172:58–62. doi: 10.1016/j.applanim.2015.08.038
47. Reed RA, Krikorian AM, Reynolds RM, Holmes BT, Branning MM, Lemons MB, et al. Post-anesthetic Cps and Equus-Fap scores in surgical and non-surgical equine patients: an observational study. Front Pain Res. (2023) 4:1217034. doi: 10.3389/fpain.2023.1217034
48. Gkikas S, Tsiknakis M. Automatic assessment of pain based on deep learning methods: a systematic review. Comput Methods Progr Biomed. (2023) 231:107365. doi: 10.1016/j.cmpb.2023.107365
49. Andersen PH, Broomé S, Rashid M, Lundblad J, Ask K, Li Z, et al. Towards machine recognition of facial expressions of pain in horses. Animals. (2021) 11:1643. doi: 10.3390/ani11061643
50. Waller BM, Bard KA, Vick S-J, Smith Pasqualini MC. Perceived differences between chimpanzee (Pan Troglodytes) and human (Homo Sapiens) facial expressions are related to emotional interpretation. J Comp Psychol. (2007) 121:398. doi: 10.1037/0735-7036.121.4.398
51. Lou ME, Porter ST, Massey JS, Ventura B, Deen J, Li Y. The application of 3d landmark-based geometric morphometrics towards refinement of the Piglet Grimace Scale. Animals. (2022) 12:1944. doi: 10.3390/ani12151944
52. Koo TK, Li MY. A Guideline of selecting and reporting intraclass correlation coefficients for reliability research. J Chiropr Med. (2016) 15:155–63. doi: 10.1016/j.jcm.2016.02.012
53. McLennan K, Mahmoud M. Development of an automated pain facial expression detection system for sheep (Ovis Aries). Animals. (2019) 9:196. doi: 10.3390/ani9040196
54. Ekman P, Friesen WV. Manual for the Facial Action Code. Palo Alto, CA: Consulting Psychologists Press (1978).
55. Caeiro CC, Waller BM, Zimmermann E, Burrows AM, Davila-Ross M. Orangfacs: a muscle-based facial movement coding system for orangutans (Pongo spp). Int J Primatol. (2013) 34:115–29. doi: 10.1007/s10764-012-9652-x
56. Parr LA, Waller BM, Vick SJ, Bard KA. Classifying chimpanzee facial expressions using muscle action. Emotion. (2007) 7:172–81. doi: 10.1037/1528-3542.7.1.172
57. Correia-Caeiro C, Holmes K, Miyabe-Nishiwaki T. Extending the maqfacs to measure facial movement in Japanese Macaques (Macaca Fuscata) reveals a wide repertoire potential. PLoS ONE. (2021) 16:e0245117. doi: 10.1371/journal.pone.0245117
58. Clark PR, Waller BM, Burrows AM, Julle-Danière E, Agil M, Engelhardt A, et al. Morphological variants of silent bared-teeth displays have different social interaction outcomes in crested macaques (Macaca nigra). Am J Phys Anthropol. (2020) 173:411–22. doi: 10.1002/ajpa.24129
59. Waller BM, Lembeck M, Kuchenbuch P, Burrows AM, Liebal K. Gibbonfacs: a muscle-based facial movement coding system for hylobatids. Int J Primatol. (2012) 33:809–21. doi: 10.1007/s10764-012-9611-6
60. Correia-Caeiro C, Burrows A, Wilson DA, Abdelrahman A, Miyabe-Nishiwaki T. Callifacs: The common marmoset facial action coding system. PLoS ONE. (2022) 17:e0266442. doi: 10.1371/journal.pone.0266442
61. Wathan J, Burrows AM, Waller BM, McComb K. Equifacs: the equine facial action coding system. PLoS ONE. (2015) 10:e0131738. doi: 10.1371/journal.pone.0131738
62. Rashid M, Silventoinen A, Gleerup KB, Andersen PH. Equine facial action coding system for determination of pain-related facial responses in videos of horses. PLoS ONE. (2020) 15:e0231608. doi: 10.1371/journal.pone.0231608
63. Waller BM, Peirce K, Caeiro CC, Scheider L, Burrows AM, McCune S, et al. Paedomorphic facial expressions give dogs a selective advantage. PLoS ONE. (2013) 8:e82686. doi: 10.1371/journal.pone.0082686
64. Caeiro CC, Burrows AM, Waller BM. Development and application of catfacs: are human cat adopters influenced by cat facial expressions? Appl Anim Behav Sci. (2017) 189:66–78. doi: 10.1016/j.applanim.2017.01.005
65. Waller BM, Julle-Daniere E, Micheletta J. Measuring the evolution of facial ‘expression' using multi-species facs. Neurosci Biobehav Rev. (2020) 113:1–11. doi: 10.1016/j.neubiorev.2020.02.031
66. Mota-Rojas D, Marcet-Rius M, Ogi A, Hernández-Ávalos I, Mariti C, Martínez-Burnes J, et al. Current advances in assessment of dog's emotions, facial expressions, and their use for clinical recognition of pain. Animals. (2021) 11:3334. doi: 10.3390/ani11113334
67. Bennett V, Gourkow N, Mills DS. Facial correlates of emotional behaviour in the domestic cat (Felis catus). Behav Processes. (2017) 141:342–50. doi: 10.1016/j.beproc.2017.03.011
68. Vojtkovská V, Voslárová E, Večerek V. Methods of assessment of the welfare of shelter cats: a review. Animals. (2020) 10:1–34. doi: 10.3390/ani10091527
69. Finka LR, Luna SP, Brondani JT, Tzimiropoulos Y, McDonagh J, Farnworth MJ, et al. Geometric morphometrics for the study of facial expressions in non-human animals, using the domestic cat as an exemplar. Sci Rep. (2019) 9:9883. doi: 10.1038/s41598-019-46330-5
70. Mullard J, Berger JM, Ellis AD, Dyson S. Development of an ethogram to describe facial expressions in ridden horses (Fereq). J Vet Behav. (2017) 18:7–12. doi: 10.1016/j.jveb.2016.11.005
71. Dyson S, Berger JM, Ellis AD, Mullard J. Can the presence of musculoskeletal pain be determined from the facial expressions of ridden horses (Fereq)? J Vet Behav. (2017) 19:78–89. doi: 10.1016/j.jveb.2017.03.005
72. Dyson S, Berger J, Ellis AD, Mullard J. Development of an ethogram for a pain scoring system in ridden horses and its application to determine the presence of musculoskeletal pain. J Vet Behav. (2018) 23:47–57. doi: 10.1016/j.jveb.2017.10.008
73. Dyson S, Pollard D. Application of the ridden horse pain ethogram to 150 horses with musculoskeletal pain before and after diagnostic anaesthesia. Animals. (2023) 13:1940. doi: 10.3390/ani13121940
74. Dyson S, Pollard D. Application of the ridden horse pain ethogram to horses competing in British eventing 90, 100 and novice one-day events and comparison with performance. Animals. (2022) 12:590. doi: 10.3390/ani12050590
75. Dyson S, Pollard D. Application of the ridden horse pain ethogram to elite dressage horses competing in world cup grand prix competitions. Animals. (2021) 11:1187. doi: 10.3390/ani11051187
76. Ask K, Rhodin M, Rashid-Engström M, Hernlund E, Andersen PH. Changes in the equine facial repertoire during different orthopedic pain intensities. Sci Rep. (2024) 14:129. doi: 10.1038/s41598-023-50383-y
77. Bussières G, Jacques C, Lainay O, Beauchamp G, Leblond A, Cadoré JL, et al. Development of a composite orthopaedic pain scale in horses. Res Vet Sci. (2008) 85:294–306. doi: 10.1016/j.rvsc.2007.10.011
78. Christov-Moore L, Simpson EA, Coudé G, Grigaityte K, Iacoboni M, Ferrari PF. Empathy: gender effects in brain and behavior. Neurosci Biobehav Rev. (2014) 46 (Pt 4):604–27. doi: 10.1016/j.neubiorev.2014.09.001
79. Zhang EQ, Leung VS, Pang DS. Influence of rater training on inter-and intrarater reliability when using the Rat Grimace Scale. J Am Assoc Lab Anim Sci. (2019) 58:178–83. doi: 10.30802/AALAS-JAALAS-18-000044
80. Schanz L, Krueger K, Hintze S. Sex and age don't matter, but breed type does-factors influencing eye wrinkle expression in horses. Front Vet Sci. (2019) 6:154. doi: 10.3389/fvets.2019.00154
81. de Oliveira AR, Gozalo-Marcilla M, Ringer SK, Schauvliege S, Fonseca MW, Trindade PHE, et al. Development, validation, and reliability of a sedation scale in horses (Equised). Front Vet Sci. (2021) 8:611729. doi: 10.3389/fvets.2021.611729
82. Kelemen Z, Grimm H, Long M, Auer U, Jenner F. Recumbency as an equine welfare indicator in geriatric horses and horses with chronic orthopaedic disease. Animals. (2021) 11:3189. doi: 10.3390/ani11113189
83. Maisonpierre IN, Sutton MA, Harris P, Menzies-Gow N, Weller R, Pfau T. Accelerometer activity tracking in horses and the effect of pasture management on time budget. Equine Vet J. (2019) 51:840–5. doi: 10.1111/evj.13130
84. Podturkin AA, Krebs BL, Watters JV, A. Quantitative approach for using anticipatory behavior as a graded welfare assessment. J Appl Anim Welf Sci. (2023) 26:463–77. doi: 10.1080/10888705.2021.2012783
85. Clegg IL, Rödel HG, Delfour F. Bottlenose dolphins engaging in more social affiliative behaviour judge ambiguous cues more optimistically. Behav Brain Res. (2017) 322(Pt A):115–22. doi: 10.1016/j.bbr.2017.01.026
86. Foris B, Thompson AJ, von Keyserlingk MAG, Melzer N, Weary DM. Automatic detection of feeding- and drinking-related agonistic behavior and dominance in dairy cows. J Dairy Sci. (2019) 102:9176–86. doi: 10.3168/jds.2019-16697
87. Fontaine D, Vielzeuf V, Genestier P, Limeux P, Santucci-Sivilotto S, Mory E, et al. Artificial intelligence to evaluate postoperative pain based on facial expression recognition. Eur J Pain. (2022) 26:1282–91. doi: 10.1002/ejp.1948
88. Werner P, Lopez-Martinez D, Walter S, Al-Hamadi A, Gruss S, Picard RW. Automatic recognition methods supporting pain assessment: a survey. IEEE Transact Affect Comp. (2022) 13:530–52. doi: 10.1109/TAFFC.2019.2946774
89. Dedek C, Azadgoleh MA, Prescott SA. Reproducible and fully automated testing of nocifensive behavior in mice. Cell Rep Methods. (2023) 3:100650. doi: 10.1016/j.crmeth.2023.100650
90. Broomé S, Feighelstein M, Zamansky A, Carreira Lencioni G, Haubro Andersen P, Pessanha F, et al. Going deeper than tracking: a survey of computer-vision based recognition of animal pain and emotions. Int J Comput Vis. (2023) 131:572–90. doi: 10.1007/s11263-022-01716-3
91. Quinn PC, Palmer V, Slater AM. Identification of gender in domestic-cat faces with and without training: perceptual learning of a natural categorization task. Perception. (1999) 28:749–63. doi: 10.1068/p2884
92. Pompermayer E, Hoey S, Ryan J, David F, Johnson JP. Straight Egyptian Arabian skull morphology presents unique surgical challenges compared to the thoroughbred: a computed tomography morphometric anatomical study. Am J Vet Res. (2023) 84:191. doi: 10.2460/ajvr.22.11.0191
93. Southerden P, Haydock RM, Barnes DM. Three dimensional osteometric analysis of mandibular symmetry and morphological consistency in cats. Front Vet Sci. (2018) 5:157. doi: 10.3389/fvets.2018.00157
94. Tuttle AH, Molinaro MJ, Jethwa JF, Sotocinal SG, Prieto JC, Styner MA, et al. A deep neural network to assess spontaneous pain from mouse facial expressions. Mol Pain. (2018) 14:1744806918763658. doi: 10.1177/1744806918763658
95. Andresen N, Wöllhaf M, Hohlbaum K, Lewejohann L, Hellwich O, Thöne-Reineke C, et al. Towards a fully automated surveillance of well-being status in laboratory mice using deep learning: starting with facial expression analysis. PLoS ONE. (2020) 15:e0228059. doi: 10.1371/journal.pone.0228059
96. Broomé S, Ask K, Rashid-Engström M, Haubro Andersen P, Kjellström H. Sharing pain: using pain domain transfer for video recognition of low grade orthopedic pain in horses. PLoS ONE. (2022) 17:e0263854. doi: 10.1371/journal.pone.0263854
97. Noor A, Zhao Y, Koubaa A, Wu L, Khan R, Abdalla FYO. Automated sheep facial expression classification using deep transfer learning. Comp Electron Agric. (2020) 175:105528. doi: 10.1016/j.compag.2020.105528
98. Broomé S, Gleerup KB, Andersen PH, Kjellstrom H, editors. Dynamics are important for the recognition of equine pain in video. In: IEEE/CVF Conference on Computer Vision and Pattern Recognition (2019).
99. Hummel H, Pessanha F, Salah AA, van Loon JP, Veltkamp R, editors. Automatic pain detection on horse and donkey faces. In: 15th IEEE International Conference on Automatic Face and Gesture Recognition. Buenos Aires (2020).
100. Feighelstein M, Shimshoni I, Finka LR, Luna SPL, Mills DS, Zamansky A. Automated recognition of pain in cats. Sci Rep. (2022) 12:9575. doi: 10.1038/s41598-022-13348-1
101. Martvel G, Lazebnik T, Feighelstein M, Henze L, Meller S, Shimshoni I, et al. Automated pain recognition in cats using facial landmarks: dynamics matter. Sci Rep. (2023). Available online at: https://www.researchsquare.com/article/rs-3754559/v1
102. Feighelstein M, Ehrlich Y, Naftaly L, Alpin M, Nadir S, Shimshoni I, et al. Deep learning for video-based automated pain recognition in rabbits. Sci Rep. (2023) 13:14679. doi: 10.1038/s41598-023-41774-2
103. Lu Y, Mahmoud M, Robinson P, editors. Estimating sheep pain level using facial action unit detection. In: 2017 12th IEEE International Conference on Automatic Face & Gesture Recognition (FG 2017). Washington, DC: IEEE (2017).
104. Mahmoud M, Lu Y, Hou X, McLennan K, Robinson P. Estimation of pain in sheep using computer vision. In:Moore RJ, , editor. Handbook of Pain and Palliative Care: Biopsychosocial and Environmental Approaches for the Life Course. Cham: Springer International Publishing (2018). p. 145–57.
105. Pessanha F, McLennan K, Mahmoud MM. Towards automatic monitoring of disease progression in sheep: a hierarchical model for sheep facial expressions analysis from video. In: 2020 15th IEEE International Conference on Automatic Face and Gesture Recognition (FG 2020). Buenos Aires (2002). p. 387–93.
106. Steagall PV, Monteiro BP, Marangoni S, Moussa M, Sautié M. Fully automated deep learning models with smartphone applicability for prediction of pain using the Feline Grimace Scale. Sci Rep. (2023) 13:21584. doi: 10.1038/s41598-023-49031-2
107. Rashid M, Broomé S, Ask K, Hernlund E, Andersen PH, Kjellström H, et al., editors. Equine pain behavior classification via self-supervised disentangled pose representation. In: IEEE/CVF Winter Conference on Applications of Computer Vision (2021).
108. Reulke R, Rueß D, Deckers N, Barnewitz D, Wieckert A, Kienapfel K, editors. Analysis of motion patterns for pain estimation of horses. In: 15th IEEE International Conference on Advanced Video and Signal Based Surveillance (AVSS). Auckland (2018).
109. Zhu H, Salgirli Y, Can P, Atilgan D, Salah AA, editors. Video-based estimation of pain indicators in dogs. In: 2023 11th International Conference on Affective Computing and Intelligent Interaction (ACII). IEEE (2023).
110. Mischkowski D, Palacios-Barrios EE, Banker L, Dildine TC, Atlas LY. Pain or nociception? Subjective experience mediates the effects of acute noxious heat on autonomic responses. Pain. (2018) 159:699–711. doi: 10.1097/j.pain.0000000000001132
111. Minh D, Wang HX Li YF, Nguyen TN. Explainable artificial intelligence: a comprehensive review. Artif Intell Rev. (2022) 55:3503–68. doi: 10.1007/s10462-021-10088-y
112. Rashid M, Gu X, Lee YJ, editors. Interspecies knowledge transfer for facial keypoint detection. In: IEEE Conference on Computer Vision and Pattern Recognition (CVPR). Honolulu, HI (2017).
113. Blumrosen G, Hawellek D, Pesaran B, editors. Towards automated recognition of facial expressions in animal models. In: IEEE International Conference on Computer Vision Workshops. Washington, DC (2017).
114. Martvel G, Shimshoni I, Zamansky A. Automated detection of cat facial landmarks. Int J Comput Vis. (2024). doi: 10.1007/s11263-024-02006-w
115. Hassan T, Seus D, Wollenberg J, Weitz K, Kunz M, Lautenbacher S, et al. Automatic detection of pain from facial expressions: a survey. IEEE Trans Pattern Anal Mach Intell. (2021) 43:1815–31. doi: 10.1109/TPAMI.2019.2958341
116. McLennan S, Fiske A, Tigard D, Müller R, Haddadin S, Buyx A. Embedded ethics: a proposal for integrating ethics into the development of medical AI. BMC Med Ethics. (2022) 23:6. doi: 10.1186/s12910-022-00746-3
Keywords: AnimalFACS, computer vision, Convolutional Neural Networks (CNNs), deep learning, facial expressions, machine learning, pain recognition
Citation: Chiavaccini L, Gupta A and Chiavaccini G (2024) From facial expressions to algorithms: a narrative review of animal pain recognition technologies. Front. Vet. Sci. 11:1436795. doi: 10.3389/fvets.2024.1436795
Received: 22 May 2024; Accepted: 03 July 2024;
Published: 17 July 2024.
Edited by:
Caterina Di Bella, University of Camerino, ItalyReviewed by:
Annika Bremhorst, University of Bern, SwitzerlandGiorgia della Rocca, University of Perugia, Italy
Copyright © 2024 Chiavaccini, Gupta and Chiavaccini. This is an open-access article distributed under the terms of the Creative Commons Attribution License (CC BY). The use, distribution or reproduction in other forums is permitted, provided the original author(s) and the copyright owner(s) are credited and that the original publication in this journal is cited, in accordance with accepted academic practice. No use, distribution or reproduction is permitted which does not comply with these terms.
*Correspondence: Ludovica Chiavaccini, lchiavaccini@ufl.edu