- 1Herbivore Laboratory, College of Animal Science, South China Agricultural University, Guangzhou, China
- 2Guangdong Gene Bank of Livestock and Poultry, College of Animal Science, South China Agricultural University, Guangzhou, China
- 3Guangdong Key Laboratory of Agricultural Animal Genomics and Molecular Breeding, South China Agricultural University, Guangzhou, China
The reproductive performance of goats significantly influences breeding efficiency and economic returns, with litter size serving as a comprehensive indicator. Despite this, research on the genetic control of litter size remains limited. Therefore, we aimed to explore the candidate genes affecting fecundity and compared the whole-genome sequences (WGS) of 15 high-litter (HL) and 15 low-litter (LL) size in Chuanzhong black goats. Then genetic diversity and genomic variation patterns were analyzed by phylogenetic, principal component and population genetic structure analysis, it was found that HL and LL subpopulations diverged. Population evolutionary selection elimination analysis was performed by Fst and θπ resulted in 506 genes were annotated in HL and 528 genes in LL. These genes were mainly related to Hippo signaling pathway, G protein-coupled signaling pathway, G protein-coupled receptor activity, cell surface receptor signaling pathway, gonadal and reproductive structure development. According to the significantly selected genomic regions and important pathways, we found that the g.89172108T > G variant locus in the exon of the AMH gene was significantly associated with litter size (P < 0.05), which could be used as an auxiliary selection gene for the high fertility of Chuanzhong black Goat.
1 Introduction
Goats, as highly versatile domestic animals, boast widespread distribution and adaptability globally. They exhibit commendable production capabilities, offering a diverse array of products including meat, milk, wool, and more to meet human needs. Notably, goat milk and its derivatives hold superior nutritional value compared to those of other mammals (1–3). Despite these advantages, the current domestic landscape reveals a significant shortfall in the supply of goat meat, milk, and related products, largely driven by escalating living standards. This imbalance necessitates substantial reliance on imports to satisfy domestic demand (4). Therefore, addressing the efficiency of goat production is paramount to rectify this supply-demand disparity and advance the goat industry.
Reproductive performance in goats, a critical determinant of production efficiency, is a multifaceted trait influenced by a complex interplay of genetic and environmental factors (5). Among these, litter size serves as a pivotal metric in assessing reproductive efficiency, directly impacting breeding efficacy and economic outcomes (6). The identification of fertility-related genes and genetic variations is paramount for deploying targeted breeding strategies to improve reproductive efficiency. High-throughput sequencing technology has been a powerful tool in recent years to study the relationship between traits and genes (7, 8). For example, Bi et al. found that CNV3 and CNV5 of the BMPR1B gene and c.71A > G loci of PRNT gene, especially the AA genotype, have a significant impact on the litter size, which can be used as DNA markers to participate in molecular marker-assisted selective breeding of Shaanbei white cashmere goats (9, 10). These genetic markers hold promise for molecular maker-assisted selective breeding. Despite the progress in identifying candidate genes associated with reproductive traits in goats, the discovery of dominant genes influencing litter size, akin to the FecB gene in sheep, remains elusive (11). This gap highlights the necessity for further comprehensive research to uncover such genes in goats, which would significantly contribute to the refinement of breeding programs.
The Chuanzhong black goat, a breed recently introduced to South China, demonstrates rapid growth, superior meat production, and broad adaptability (12). However, its reproductive performance displays considerable variability, with some individuals achieving a lambing rate and kidding rate exceeding 2.5, while some goats fall below 2.0. This variability presents both a challenge and an opportunity for genetic improvement. Accordingly, this study endeavors to explore candidate genes related to reproductive performance and their regulatory networks. We conducted WGS to investigate the population genetics of high and low-litter size within the Chuanzhong black goat and endeavored to identify genomic regions and genes associated with reproduction. Our findings are intended to provide a scientific foundation for the selection and improvement of this breed, thereby contributing to the theoretical and practical optimization of the goat breeding industry in South China and potentially informing global breeding practices.
2 Materials and methods
2.1 Sample collection and whole genome sequencing
At Wens Liangdong Goat Farm, we conducted a screening process on 30 black goats with homogeneous genetic backgrounds, robust physical conditions, comparable ages, uniform breeding environments, and identical parity, yet varying litter sizes. This selection comprised 15 high-litter (HL) goats, exhibiting an average litter size exceeding 2.6 in the 2nd, 3rd, and 4th births, alongside 15 low-litter (LL) goats, manifesting an average litter size below 1.7. After the DNA extraction of the selected goat ear samples using the TIANamp Genomic DNA Kit (TIANGEN, CHINA), their purity and integrity were evaluated. Subsequently, the qualified DNA samples underwent library construction and sequencing at Novogene Co., Ltd. (13). The sequencing libraries were prepared with the NEB Next® Ultra™ DNA Library Prep Kit for Illumina (NEB, USA), following the manufacturer's protocols, with index codes assigned to each sample. Index-coded samples were clustered using the Illumina PE Cluster Kit (Illumina, USA) on a cBot Cluster Generation System. Following cluster generation, sequencing of the DNA libraries was conducted on the Illumina platform, resulting in the generation of 150 bp paired-end reads (14).
2.2 Data quality control, mapping, and annotation
Before proceeding with the analysis of sequencing data, it is imperative to conduct quality control measures to ensure the precision and reliability of the data. This involves filtering the raw data to ensure that the sequencing error rate at each base position is maintained below 1%. Then valid sequencing data was mapped to the reference genome (https://ftp.ncbi.nlm.nih.gov/genomes/all/GCF/001/704/415/GCF_001704415.1_ARS1) by Burrows-Wheeler Aligner (BWA) software (Version: 0.7.8) with parameter as mem -t 4 -k 32 -M to get the original mapping results (15).
The variation detection in this study primarily focused on single nucleotide polymorphism sites (SNPs), which are primarily focused on SAMtools (version 1.2) with the parameters as Dp4-miss0.1-maf0.05 (16). To reduce the error rate of SNP detection, The depth of the variate position < 4 and mapping quality (MQ) < 20 were filtered out, and the filtered high-quality SNPs were functionally annotated with ANNOVAR software (Version: 2013-05-20) (17).
2.3 Population structure
Population genetic structure can reflect the relationships and evolutionary process between groups or within populations. Population structure analysis was performed using PLINK 1.9 (18). The input file-Ped file of PLINK 1.9 was first created to describe individual genotype data and kinship, then use the ADMIXTURE V 1.3.0 software (19) to construct the population genetic structure and population lineage information. In this study, three methods of population genetic structure analysis were used: phylogenetic tree, PCA, and Structure (20).
2.4 Population evolution selection elimination analysis
According to the filtered SNPs, population genome scanning of HL and LL groups of Chuanzhong black goats were performed using PopGenome software (21) with sliding window algorithm. The size of the selection and elimination window was determined according to the density of SNPs at the genome level. The calculation of windows with different lengths showed that starting from 100 kb, the number of SNPs in the window began to stabilize, so a 100 kb window was chosen for selection elimination analysis. Based on the Fixation indices (F-statistics) (22) of population differentiation, Fst values were calculated for each window using vcftools_v0.1.14 software (23) with a sliding window of 100 kb interval and 50% overlap as a step to assess the population genetic structure among subpopulations. Based on nucleotide diversity (θπ) (24), it involves sliding a window of a certain θπ size across the genome and analyzing the differences in population genetic information (SNPs) within the sliding window. In this study, vcftools_v0.1.14 was used with 100 kb intervals as sliding windows, 50% overlap as step size, to compute the population θπ value. We identified and retrieved genes annotated within the overlapping regions representing the top 5% selections by both θπ and Fst (25).
2.5 Gene function enrichment
For gene functional enrichment analysis, we selected the top 5% regions identified by θπ and the top 5% regions identified by Fst, then we extracted overlapping regions and annotated the genes within those regions. All candidate genes were first mapped to each term in the GO database, the number of target genes mapped to each term was calculated, then the GO entries that were significantly enriched in the candidate target genes were identified using the hypergeometric test. The major biological functions of the candidate target genes can be determined by GO functional significance enrichment analysis (26). KEGG pathway analysis was performed on candidate genes using the KOBAS website and pathways with corrected p-value ≤ 0.05 were selected as significantly enriched in the candidate genes (27). The pathway significant enrichment analysis can identify the most important biochemical metabolic pathways and signal transduction pathways in which the candidate genes are involved.
2.6 Re-sample collection and AMH gene polymorphism detection
AMH is the highly prioritized gene within the HL group. To authenticate the selected SNPs, we procured an additional 161 ear samples from healthy female Chuanzhong black goats at Guangdong Wen's Liangdong Goat Farm. These samples were sourced from animals with documented litter sizes for their first three births, and devoid of any instances of abortion, dystocia, or stillbirths. Subsequently, ear DNA extraction was performed. Next, specific primers were designed for two SNP sites on the AMH gene exon (g.89172108T > G F: AGCGAAGGTGGTCAAGTCAC g.89172183G > A R: AGGAGATAGGGACTGCCCTG). PCR amplification was performed using these primers, and the qualified PCR products were sent to BGI Genomics Co., Ltd. for Sanger sequencing. Then, use SeqMan Pro (https://www.dnastar.com/software/lasergene/seqman-ultra/) to examine the sequencing chromatograms and determine the genotypes of the 2 SNP mutation sites in each goat sample. Then, the sequencing results were compared with the gene fragments to find out the SNP variation sites, and the gene frequency was analyzed using SPSS 25 (https://www.ibm.com/support/pages/downloading-ibm-spss-statistics-25) to determine the impact of the mutation sites on the litter size.
3 Results
3.1 Gene sequencing and general features of samples
Per the local standard DB51T 2355-2017 of Sichuan Province, the reproductive performance of female Chuanzhong black goats (Figure 1) is categorized into four classes (Table 1). Specifically, a litter size exceeding 2.5 is designated as super class, over 2.4 as first class, over 2.2 as second class, and over 2.0 as third class. This study focuses on extreme populations with litter size above super class (≥2.6) or below third class ( ≤ 1.7). WGS was performed on 15 HL and 15 LL Chuanzhong black goats using Illumina platform. We obtained 851.26GB (Q20 ≥ 96.74%, Q30 ≥ 91.53%) clean data with 43.03% average GC content. As show in Supplementary Table S1. Sequencing depth and coverage statistics are shown in Supplementary Table S2.
A total of 9,890,068 SNPs were obtained after the data filtered by SAMTOOLS software. Among them, 44,408 (0.45%) were in the 1 Kb region upstream of the gene and 54,284 (0.55%) were in the 1 Kb region downstream of the gene. There were 61,544 exon SNPs (0.62%), most of them were synonymous mutations (0.37%) and non-synonymous mutations (0.25%); a small part was acquired stop codon mutations (0.002 %), and loss of stop codon variation (0.25%). In addition, there were 132 (0.0013%) SNPs located in the splice site. The SNP mutation types of goats were mainly conversions of T/C and G/A (Supplementary Figure S1B). The software annotation revealed that the number of chromosomal SNPs in the HL and LL groups showed an overall decreasing trend starting from chromosome 1 (Supplementary Figure S1A), with the highest number of chromosome 1 SNPs and the lowest number of chromosome 25 SNPs.
3.2 Population structure and characterization of HL and LL Chuanzhong black goats
To investigate the relationship among experimental individuals, phylogenetic tree and PCA were conducted on all individuals. The results of the phylogenetic tree constructed by Treebest showed that although the two groups of high and low litters came from the similar genetic background, there was obvious genetic differentiation between the two groups (Figure 2A). The PCA plot also demonstrated clear separation between the HL and LL groups, classifying them into two distinct populations (Figure 2B).
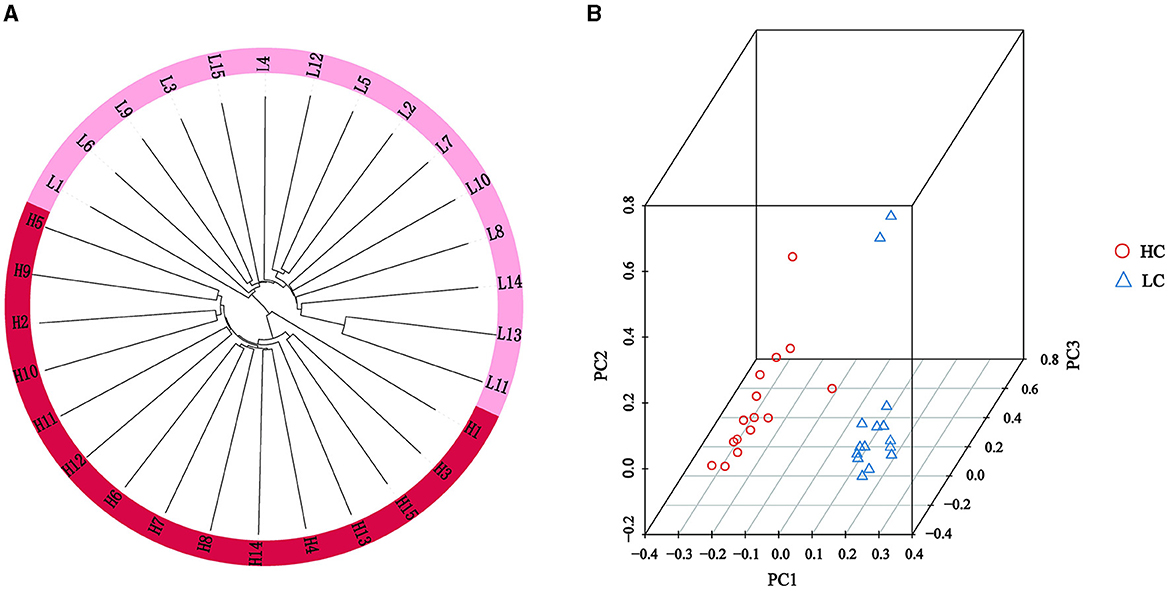
Figure 2. Population structure and characterization of high and low litter sizes in Chuanzhong black goat. (A) Phylogenetic tree of HL and LL groups; (B) PCA of HL and LL groups.
3.3 Mining the genetic of high and low litter size
To identify genetic variants or loci associated with the differences in reproductive performance between HL and LL groups, we performed a population evolutionary selection elimination analysis to calculate the Fst values between two experimental groups, a total of 59,067 windows were obtained (Figure 3A). We conducted the Fst & θπ joint analysis by scanning the genomes of the HL and LL groups, which aimed to screen out stronger candidate genes related to the target traits, this involved selecting windows that simultaneously reached the top 5% for both Fst values and θπ values. The results of selected regions were shown in Figure 3B. It can be observed that the green region represents the top 5% selected regions in the HL group [log2 θπ ratio (θπLL/θπHL) < −0.22, Fst > 0.03], with annotations for a total of 506 genes. Among the genes annotated by SNPs with maximum Fst values were SKAP1, CBX1, and SNX11, in addition to AMH, HESX1, WNT2, PRDM1, and TAF4. The blue region represents the top 5% selected regions in the LL group [log2 θπ ratio (θπLL/θπHL) < −0.24, Fst > 0.03], with annotations for a total of 528 genes. Include ARRDC1, GMDS, MRPL40, RAPSN, and SLC39A13. In addition, except for the selected genes specific to the HL and LL groups, there were 8 common selected genes in the selected regions of both groups, namely CEP350, EYA1, GMDS, KIAA2012, MTCL1, NRG3, PRDM1, and RABGAP1L.
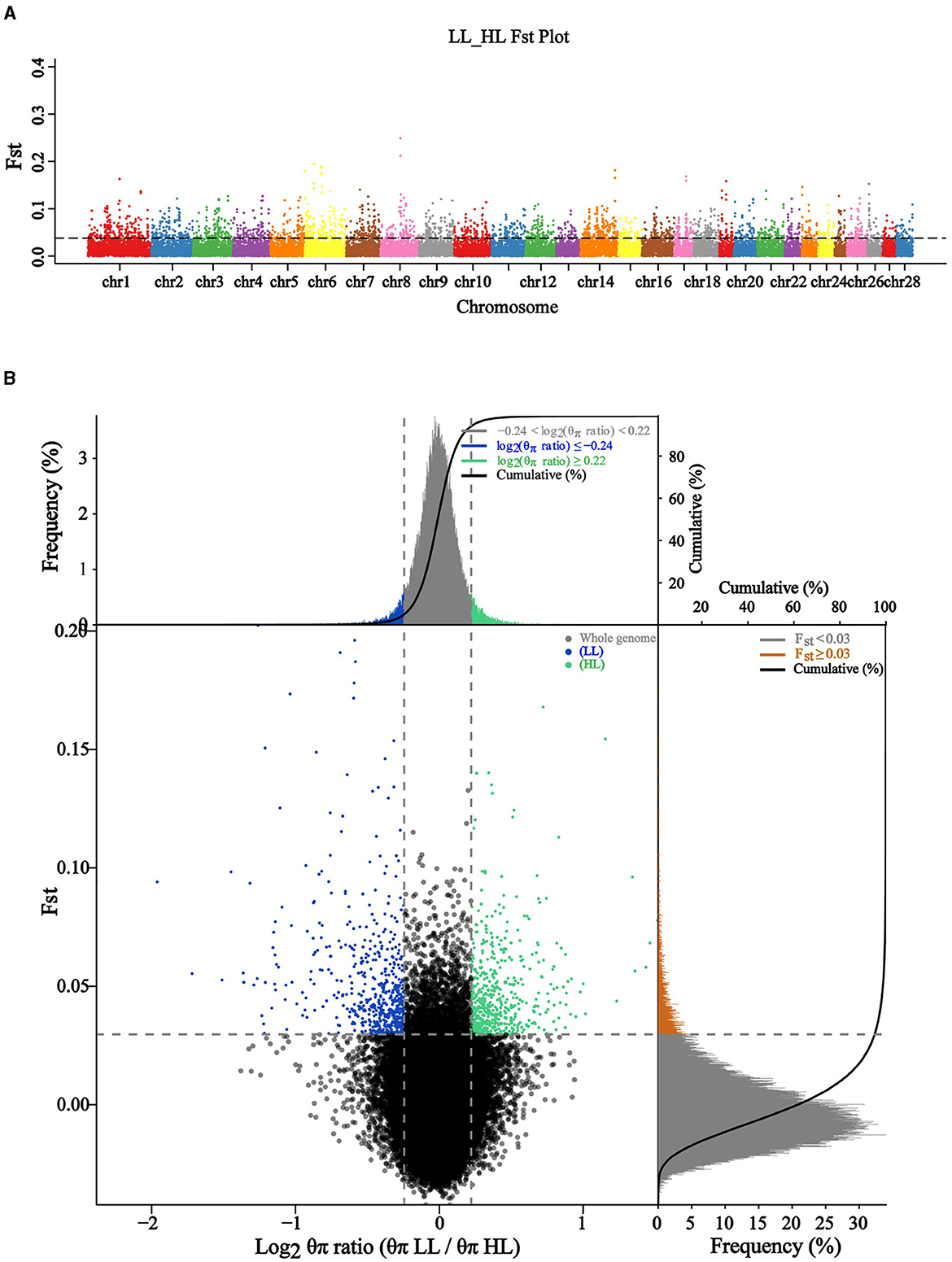
Figure 3. Genetic excavating on high and low litter size in Chuanzhong black goat. (A) Distribution of autosomal Fst in HL and LL groups; (B) Fst & θπ selection elimination analysis for HL and LL groups.
Next, the GO and KEGG functional enrichment were performed on the strongly selected genes obtained by the Fst & θπ analysis in the HL and LL group, Terms significantly enriched among the candidate genes were selected based on an overrepresented p-value threshold of ≤ 0.05. In the HL group, a total of 121 GO function items were significantly enriched, mainly including cell surface receptor signaling pathway, G-protein coupled receptor activity, cellular response to stimulus, signal transduction, single organism signaling, reproductive system development, ATPase activity, coupled to transmembrane movement of ions, rotational mechanism, etc. (Figure 4A). There were 7 KEGG items significantly enriched, namely olfactory transduction, proteasome, amino sugar and nucleotide sugar metabolism, hippo signaling pathway, neuroactive ligand-receptor interaction, RNA transport and arachidonic acid metabolism (Figure 4C). This showed that the HL group had a more active biological response, a more sensitive sense of smell, etc. While in the LL group, 132 GO items were significantly enriched, including cellular catabolic process, cytoskeleton organization, negative regulation of WNT receptor signaling pathway, negative regulation of signal transduction, ligase activity, etc. (Figure 4B). And 14 KEGG items were significantly enriched. They were hypertrophic cardiomyopathy, dilated cardiomyopathy, glycosaminoglycan degradation, hepatitis C, RNA degradation, retrograde endocannabinoid signaling, RIG-I-like receptor signaling pathway, etc. (Figure 4D). Apparently, more disease-related pathways were enriched in the LL group, which was very detrimental to reproductive ability.
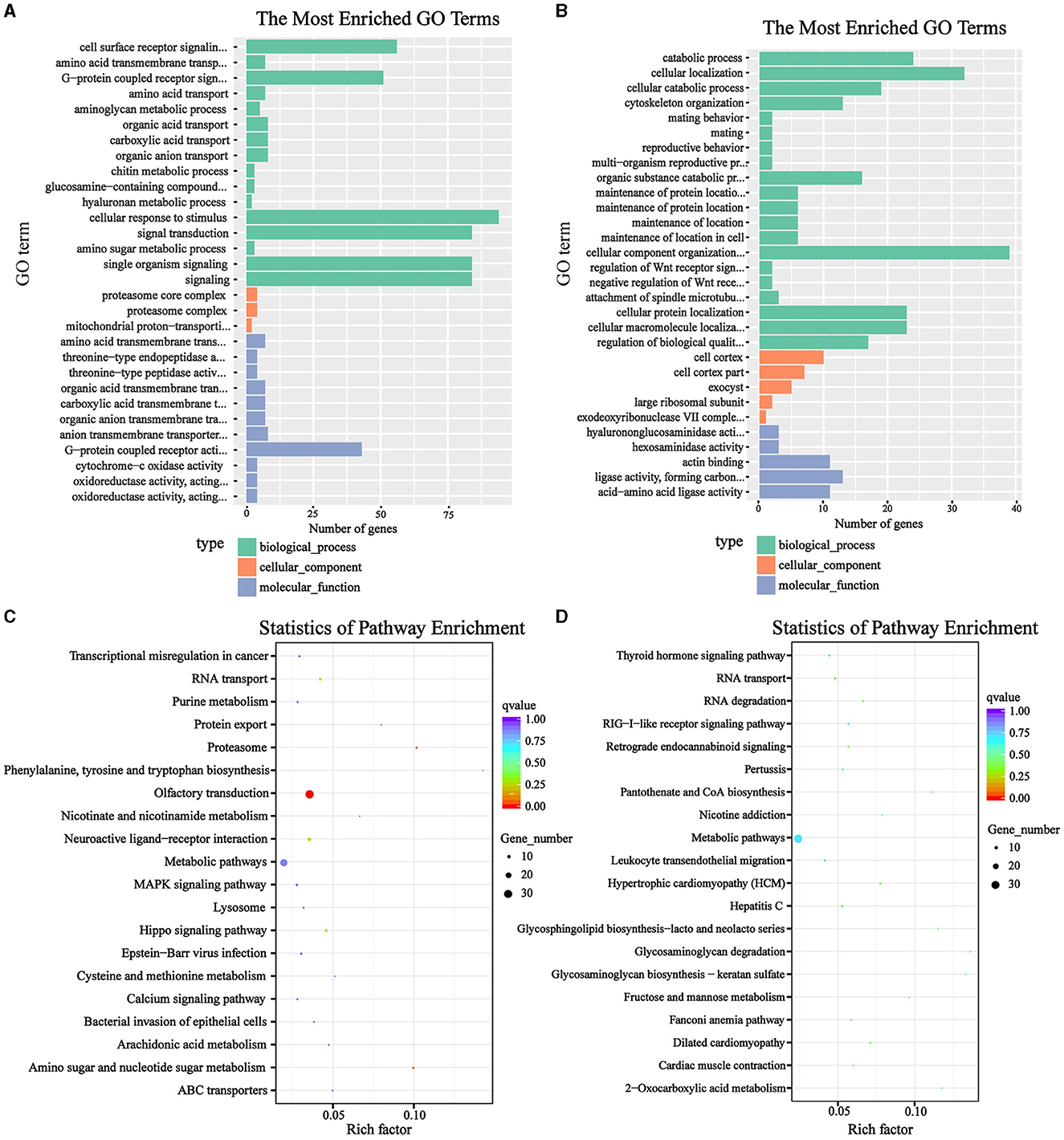
Figure 4. Functional enrichment of genes related to litter size. (A, B) GO enrichment analysis of HL and LL group; (C, D) KEGG enrichment analysis of HL and LL group.
3.4 Association analysis of AMH gene polymorphism and litter size performance
The analysis of Fst and θπ revealed a highly selected gene, AMH, within the HL group, hypothesized to exert an influence on goat litter size in conjunction with existing literature. Therefore, specific primers were designed targeting two SNP mutation sites in the AMH gene exon. PCR amplification was performed using DNA templates from 161 Chuanzhong black goats (Supplementary Table S3). The results revealed polymorphisms at both SNP mutation sites in the AMH gene exon, and the base types of the mutation sites were consistent with the results of whole-genome resequencing. Among them, g.89172108T > G exhibited moderate polymorphism (0.25 < PIC < 0.5), while g.89172183G > A showed low polymorphism (PIC < 0.25). Chi-square tests showed that the P-values for g.89172108T > G and g.89172183G > A were both >0.05, indicating that they were in Hardy-Weinberg equilibrium.
Subsequently, SPSS 25 was used to analyze the association between different genotypes of AMH gene and different litters of Chuanzhong black goat and the average number of lambs produced in the first three litters. As shown in Table 2, the AMH g.89172108T > G variant locus was significantly (P < 0.05) associated with the average number of lambs produced in the first three litters, and the TT genotype was significantly higher than the GG genotype, and it was also observed that the number of lambs produced by the TT genotype was higher than that of the TG and GG genotypes in the first, second and third litter. However, the g.89172108T > G variant site showed no significant association with the overall litter size of Chuanzhong black goats (P > 0.05).
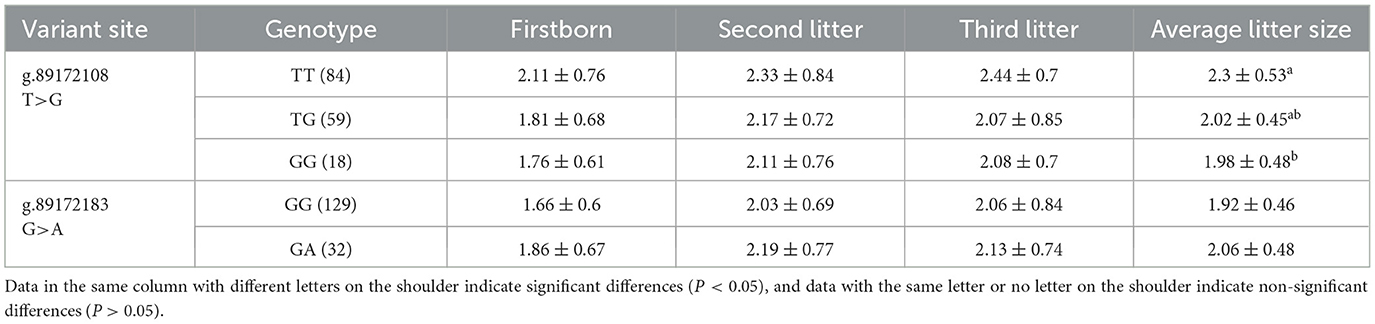
Table 2. Association analysis between SNP variant site of AMH and litter size in Chuanzhong black goat.
4 Discussion
Optimizing litter size is crucial for enhancing livestock productivity and farm profitability, as it directly correlates with reproductive efficiency. To better understand the genetic underpinnings of high fecundity, this study employed WGS on 15 high and 15 low litter size Chuanzhong black goats. The goal was to elucidate gene expression profiles linked to reproductive performance and identify potential marker genes for selective breeding.
The selected sample consisted of 30 goats from the same genetic background and farm, ensuring uniformity in environmental conditions. Population structure analysis and principal component analysis both revealed significant genetic differentiation between the high and low litter size groups, underscoring the distinct genetic bases of reproductive traits. These findings are consistent with prior research by Kang et al. (28), who reported global differences in DNA methylation between high and low litter size goat ovaries. Similarly, Zhang et al. (29) identified significant genomic copy number variations (CNVs) associated with litter size differences, further supporting the notion of genetic divergence in reproductive traits. The current study builds on these findings by highlighting differences in gene expression, not only within goats (30) but also in other species like pigs (31) and mice (32). Interestingly, the observed genetic convergence in prolificacy between goats and sheep (33) suggests that similar genetic trends may govern reproductive traits across different species.
Goat reproductive traits are governed by polygenic inheritance and are influenced by a myriad of internal and external factors, making them complex quantitative traits. These traits involve various physiological processes such as ovarian follicle development, oocyte maturation, and embryo development. Understanding these traits has both theoretical and practical implications for animal breeding. However, the heritability of these traits is relatively low, and environmental factors often slow breeding progress (34). Selective elimination remains a pivotal tool in livestock management, as it enhances productivity, health, and economic viability by culling undesirable traits or genotypes. This study's selective elimination analysis identified candidate genes and variant sites related to reproduction, which could improve reproductive performance and enhance production efficiency. Through combined Fst and θπ analysis, a total of 506 genes were selected in the high litter size group. Notably, the genes SKAP1, CBX1, and SNX11 were among those annotated with the SNPs having the highest Fst values. SKAP1, an immune cell adapter, plays a role in T cell immune responses (35), which are vital for ovarian function (36). CBX1, a member of the heterochromatin protein family, is involved in chromatin condensation and transcriptional regulation, processes that significantly influence both sperm development and cognitive function (37, 38).
In addition, we found AMH related to reproduction in the genes selected and enriched signaling pathways by the HL group. AMH belongs to the transforming growth factor-beta (TGF-β) superfamily, which is produced by granulosa cells of early-growing follicles in the ovary and is associated with the antral follicle number. It is most strongly expressed in the granulosa cells of preantral and small antral follicles, whereas its expression decreases after dominant follicle selection and is absent in atretic follicles (39). This dynamic pattern has been confirmed in various animals, including rats (40), humans (41), cattle (42), sheep (43), and goats (44), suggesting that AMH is a major regulator of early follicular growth. Furthermore, Han et al. (45) found a significant association between a non-synonymous SNP (g.89172108A > C) in the AMH and litter size in the second parity of Dazu black goats (46). In this study, we discovered two non-synonymous SNP variants in the AMH of Chuanzhong Black goats: g.89172108T > G and g.89172183G > A. It was validated that the g.89172108T > G variant was significantly associated with the average litter size of the first three parities (P < 0.05). The TT genotype showed significantly higher litter size than the GG genotype, and across the data of the first, second and third litter size, the TT genotype was higher than that of the TG and GG genotypes. It is speculated that Chuanzhong black goats with the TT genotype may possess more ovarian reserves, leading to an increase in litter size. This genotype could be a potential molecular marker for selecting individuals with high reproductive performance in goats.
The functional enrichment of selected genes provides valuable insights for identifying candidate genes related to reproductive traits. Several significantly enriched pathways closely associated with reproduction were identified, including the Hippo signaling pathway in the KEGG database and G-protein coupled receptor signaling, G-protein coupled receptor activity, cell surface receptor signaling, gonad development, reproductive organ development, and reproductive system development in the GO database. The Hippo signaling pathway is an evolutionarily conserved pathway that regulates organ size by controlling cell proliferation, differentiation, apoptosis, and cell fate determination (47). The Hippo pathway and its effector, YAP1, are crucial for fine-tuning ovarian physiology, including cell fate determination, follicle activation, growth and differentiation, and steroidogenesis (48, 49). Dysregulation of this pathway can lead to excessive activation of YAP1 in granulosa cells, resulting in the disruption of tissue homeostasis and decreased fertility. G protein-coupled receptors (GPCRs) are the largest transmembrane receptor superfamily found in the genomes of humans, mice, nematodes, fruit flies, and other organisms. They play crucial roles in regulating various physiological functions, including external and internal secretion, smooth muscle and cardiac contraction, fluid homeostasis, and immune responses (50, 51). Research has revealed that GPCRs can activate or inhibit the Hippo-YAP pathway, with the Hippo pathway established as a critical signaling branch downstream of GPCRs (52). This indicates that the selected genes of the high and low litter size groups of Chuanzhong black goats in this study may be related to the reproductive process by participating in the regulation of Hippo signaling pathway, G protein-coupled receptor activity, G protein-coupled signaling pathway and reproductive system development. Future research should focus on validating these findings in other goat breeds and livestock species, as well as exploring the environmental interactions with these genetic markers to optimize breeding strategies.
5 Conclusion
Our study showed that based on WGS data, there was a divergence between the high and low litter size subpopulations of Chuanzhong black goats. The evolutionary selection for high-yield traits may implicate pathways such as the Hippo signaling pathway, G protein-coupled signaling pathway, G protein-coupled receptor activity, cell surface receptor signaling pathway, gonadal development, reproductive structure development. Furthermore, we identified a significant association between the mutation locus g.89172108T > G in the exon of the AMH gene and the average number of lambs within the first three litters (P < 0.05), suggesting its potential utility as a molecular marker for assisted selection.
Data availability statement
The datasets presented in this study can be found in online repositories. The names of the repository/repositories and accession number(s) can be found in the article/Supplementary material.
Ethics statement
The study was conducted following the ARRIVE guidelines, and no anesthetics or euthanasia drugs were used in our study. All experimental animal procedures complied with the relevant guidelines and regulations for the management and welfare of experimental animals approved by the Ethics Committee of South China Agricultural University, Guangdong Province (license number: SYXK-2018-0123). The studies were conducted in accordance with the local legislation and institutional requirements. Written informed consent was obtained from the owners for the participation of their animals in this study.
Author contributions
CG: Methodology, Writing – original draft, Writing – review & editing. JY: Investigation, Supervision, Writing – original draft. JL: Conceptualization, Data curation, Writing – original draft. ZL: Formal analysis, Writing – original draft. MD: Data curation, Formal analysis, Writing – review & editing. YG: Resources, Writing – review & editing. GL: Resources, Supervision, Writing – review & editing. BS: Funding acquisition, Writing – review & editing. YL: Funding acquisition, Methodology, Writing – review & editing. DL: Funding acquisition, Project administration, Writing – review & editing.
Funding
The author(s) declare financial support was received for the research, authorship, and/or publication of this article. This work was supported by Agricultural and Rural Department of Guangdong Province (2022XPY00009) and the Department of Science and Technology of Guangdong Province (KTP20210344).
Conflict of interest
The authors declare that the research was conducted in the absence of any commercial or financial relationships that could be construed as a potential conflict of interest.
Publisher's note
All claims expressed in this article are solely those of the authors and do not necessarily represent those of their affiliated organizations, or those of the publisher, the editors and the reviewers. Any product that may be evaluated in this article, or claim that may be made by its manufacturer, is not guaranteed or endorsed by the publisher.
Supplementary material
The Supplementary Material for this article can be found online at: https://www.frontiersin.org/articles/10.3389/fvets.2024.1420164/full#supplementary-material
References
1. Clark S, Mora García MB. A 100-year review: advances in goat milk research. J Dairy Sci. (2017) 100:10026–44. doi: 10.3168/jds.2017-13287
2. Li N, Xie Q, Chen Q, Evivie SE, Liu D, Dong J, et al. Cow, goat, and mare milk diets differentially modulated the immune system and gut microbiota of mice colonized by healthy infant feces. J Agric Food Chem. (2020) 68:15345–57. doi: 10.1021/acs.jafc.0c06039
3. Amills M, Capote J, Tosser-Klopp G. Goat domestication and breeding: a jigsaw of historical, biological and molecular data with missing pieces. Anim Genet. (2017) 48:631–44. doi: 10.1111/age.12598
4. Vacca GM, Stocco G, Dettori ML, Pira E, Bittante G, Pazzola M. Milk yield, quality, and coagulation properties of 6 breeds of goats: environmental and individual variability. J Dairy Sci. (2018) 101:7236–47. doi: 10.3168/jds.2017-14111
5. Halsey AH. Genetic and environmental factors in human ability: a review. Eugen Rev. (1967) 59:49–51.
6. Wang J-J, Zhang T, Chen Q-M, Zhang R-Q, Li L, Cheng S-F, et al. Genomic signatures of selection associated with litter size trait in jining gray goat. Front Genet. (2020) 11:286. doi: 10.3389/fgene.2020.00286
7. Liu L-L, Meng J, Ma H-Y, Cao H, Liu W-J. Candidate genes for litter size in Xinjiang sheep identified by Specific Locus Amplified Fragment (SLAF) sequencing. Anim Biotechnol. (2023) 34:3053–62. doi: 10.1080/10495398.2022.2131561
8. Tian Q, He J-P, Zhu C, Zhu Q-Y, Li Y-G, Liu J-L. Revisiting the transcriptome landscape of pig embryo implantation site at single-cell resolution. Front Cell Dev Biol. (2022) 10:796358. doi: 10.3389/fcell.2022.796358
9. Bi Y, Feng W, Kang Y, Wang K, Yang Y, Qu L, et al. Detection of mRNA expression and copy number variations within the goat FecB gene associated with litter size. Front Veter Sci. (2021) 8:758705. doi: 10.3389/fvets.2021.758705
10. Bi Y, Zhang S, Li J, He L, Kang Y, Chen H, et al. The mRNA expression profile of the goat prion protein testis-specific (PRNT) gene and its associations with litter size. Theriogenology. (2021) 165:69–75. doi: 10.1016/j.theriogenology.2021.02.013
11. Gootwine E, Reicher S, Rozov A. Prolificacy and lamb survival at birth in Awassi and Assaf sheep carrying the FecB (Booroola) mutation. Anim Reprod Sci. (2008) 108:402–11. doi: 10.1016/j.anireprosci.2007.09.009
12. Lu T, Zou X, Liu G, Deng M, Sun B, Guo Y, et al. Preliminary study on the characteristics of microRNAs in ovarian stroma and follicles of chuanzhong black goat during estrus. Genes. (2020) 11:970. doi: 10.3390/genes11090970
13. Yang J, Li W-R, Lv F-H, He S-G, Tian S-L, Peng W-F, et al. Whole-genome sequencing of native sheep provides insights into rapid adaptations to extreme environments. Mol Biol Evol. (2016) 33:2576–92. doi: 10.1093/molbev/msw129
14. Shi H, Li T, Su M, Wang H, Li Q, Lang X, et al. Whole genome sequencing revealed genetic diversity, population structure, and selective signature of Panou Tibetan sheep. BMC Genomics. (2023) 24:50. doi: 10.1186/s12864-023-09146-2
15. Li H, Durbin R. Fast and accurate short read alignment with Burrows–Wheeler transform. Bioinformatics. (2009) 25:1754–60. doi: 10.1093/bioinformatics/btp324
16. Li H, Handsaker B, Wysoker A, Fennell T, Ruan J, Homer N, et al. The sequence alignment/map format and SAMtools. Bioinformatics. (2009) 25:2078–9. doi: 10.1093/bioinformatics/btp352
17. Wang K, Li M, Hakonarson H. ANNOVAR functional annotation of genetic variants from high-throughput sequencing data. Nucleic Acids Res. (2010) 38:e164. doi: 10.1093/nar/gkq603
18. Purcell S, Neale B, Todd-Brown K, Thomas L, Ferreira MAR, Bender D, et al. PLINK: a tool set for whole-genome association and population-based linkage analyses. Am J Hum Genet. (2007) 81:559–75. doi: 10.1086/519795
19. Tang H, Peng J, Wang P, Risch NJ. Estimation of individual admixture: analytical and study design considerations. Genet Epidemiol. (2005) 28:289–301. doi: 10.1002/gepi.20064
20. Paradis E, Claude J, Strimmer K. APE analyses of phylogenetics and evolution in R language. Bioinformatics. (2004) 20:289–90. doi: 10.1093/bioinformatics/btg412
21. Pfeifer B, Wittelsbürger U, Ramos-Onsins SE, Lercher MJ. PopGenome: an efficient Swiss army knife for population genomic analyses in R. Mol Biol Evol. (2014) 31:1929–36. doi: 10.1093/molbev/msu136
22. Weir BS, Cockerham CC. Estimating F-statistics for the analysis of population structure. Evolution. (1984) 38:1358–70. doi: 10.1111/j.1558-5646.1984.tb05657.x
23. Danecek P, Auton A, Abecasis G, Albers CA, Banks E, DePristo MA, et al. The variant call format and VCFtools. Bioinformatics. (2011) 27:2156–8. doi: 10.1093/bioinformatics/btr330
24. Tajima F. Statistical method for testing the neutral mutation hypothesis by DNA polymorphism. Genetics. (1989) 123:585–95. doi: 10.1093/genetics/123.3.585
25. Lin T, Zhu G, Zhang J, Xu X, Yu Q, Zheng Z, et al. Genomic analyses provide insights into the history of tomato breeding. Nat Genet. (2014) 46:1220–6. doi: 10.1038/ng.3117
26. Gene Ontology Consortium. The Gene Ontology resource: enriching a GOld mine. Nucleic Acids Res. (2021) 49:D325–D334. doi: 10.1093/nar/gkaa1113
27. Kanehisa M, Furumichi M, Sato Y, Kawashima M, Ishiguro-Watanabe M. KEGG for taxonomy-based analysis of pathways and genomes. Nucleic Acids Res. (2023) 51:D587–92. doi: 10.1093/nar/gkac963
28. Kang B, Wang J, Zhang H, Shen W, El-Mahdy Othman O, Zhao Y, et al. Genome-wide profile in DNA methylation in goat ovaries of two different litter size populations. J Anim Physiol Anim Nutr. (2022) 106:239–49. doi: 10.1111/jpn.13600
29. Zhang R-Q, Wang J-J, Zhang T, Zhai H-L, Shen W. Copy-number variation in goat genome sequence: a comparative analysis of the different litter size trait groups. Gene. (2019) 696:40–6. doi: 10.1016/j.gene.2019.02.027
30. Zhao YX, Gao GX, Zhou Y, Guo CX Li B, El-Ashram S, Li ZL. Genome-wide association studies uncover genes associated with litter traits in the pig. Animal. (2022) 16:100672. doi: 10.1016/j.animal.2022.100672
31. Zhang X, Huang L, Wu T, Feng Y, Ding Y, Ye P, et al. Transcriptomic analysis of ovaries from pigs with high and low litter size. PLoS ONE. (2015) 10:e0139514. doi: 10.1371/journal.pone.0139514
32. Langhammer M, Wytrwat E, Michaelis M, Schön J, Tuchscherer A, Reinsch N, et al. Two mouse lines selected for large litter size display different lifetime fecundities. Reproduction. (2021) 161:721–30. doi: 10.1530/REP-20-0563
33. Tao L, He X, Jiang Y, Liu Y, Ouyang Y, Shen Y, et al. Genome-wide analyses reveal genetic convergence of prolificacy between goats and sheep. Genes. (2021) 12:480. doi: 10.3390/genes12040480
34. Zhang W-Y, Yuan Y, Zhang H-Y, He Y-M, Liu C-L, Xu L, et al. Genetic basis investigation of wattle phenotype in goat using genome-wide sequence data. Animal Genet. (2022) 53:700–5. doi: 10.1111/age.13235
35. Smith X, Taylor A, Rudd CE. T-cell immune adaptor SKAP1 regulates the induction of collagen-induced arthritis in mice. Immunol Lett. (2016) 176:122–7. doi: 10.1016/j.imlet.2016.04.007
36. Jiao X, Zhang X, Li N, Zhang D, Zhao S, Dang Y, et al. Treg deficiency-mediated TH1 response causes human premature ovarian insufficiency through apoptosis and steroidogenesis dysfunction of granulosa cells. Clin Transl Med. (2021) 11:e448. doi: 10.1002/ctm2.448
37. Charaka V, Tiwari A, Pandita RK, Hunt CR, Pandita TK. Role of HP1β during spermatogenesis and DNA replication. Chromosoma. (2020) 129:215–26. doi: 10.1007/s00412-020-00739-4
38. Kuroda Y, Iwata-Otsubo A, Dias K-R, Temple SEL, Nagao K, De Hayr L, et al. Dominant-negative variants in CBX1 cause a neurodevelopmental disorder. Genetics in Medicine. (2023) 25:100861. doi: 10.1016/j.gim.2023.100861
39. di Clemente N, Racine C, Pierre A, Taieb J. Anti-müllerian hormone in female reproduction. Endocr Rev. (2021) 42:753–82. doi: 10.1210/endrev/bnab012
40. Baarends WM, Uilenbroek JT, Kramer P, Hoogerbrugge JW, van Leeuwen EC, Themmen AP, et al. Anti-müllerian hormone and anti-müllerian hormone type II receptor messenger ribonucleic acid expression in rat ovaries during postnatal development, the estrous cycle, and gonadotropin-induced follicle growth. Endocrinology. (1995) 136:4951–62. doi: 10.1210/endo.136.11.7588229
41. Weenen C, Laven JSE, von Bergh ARM, Cranfield M, Groome NP, Visser JA, et al. Anti-Müllerian hormone expression pattern in the human ovary: potential implications for initial and cyclic follicle recruitment. Mol Hum Reprod. (2004) 10:77–83. doi: 10.1093/molehr/gah015
42. Monniaux D, Barbey S, Rico C, Fabre S, Gallard Y, Larroque H. Anti-Mllerian hormone: a predictive marker of embryo production in cattle. Reprod Fertil Dev. (2010) 22:1083–91. doi: 10.1071/RD09279
43. Veiga-Lopez A, Ye W, Padmanabhan V. Developmental programming: prenatal testosterone excess disrupts anti-Müllerian hormone expression in preantral and antral follicles. Fertil Steril. (2012) 97:748–56. doi: 10.1016/j.fertnstert.2011.12.028
44. Rocha R, Lima L, Carvalho A, Chaves R, Bernuci M, Rosa-e-Silva A, et al. Immunolocalization of the anti-müllerian hormone (AMH) in caprine follicles and the effects of AMH on in vitro culture of caprine pre-antral follicles enclosed in ovarian tissue reproduction in domestic. Animals. (2016) 51:212–9. doi: 10.1111/rda.12668
45. Han Y-G, Zeng Y, Huang Y-F, Huang D-L, Peng P, Na R-S, et al. A nonsynonymous SNP within the AMH gene is associated with litter size in Dazu black goats. Anim Biotechnol. (2022) 33:992–6. doi: 10.1080/10495398.2020.1842750
46. Han M, Liang C, Liu Y, He X, Chu M. Integrated transcriptome analysis reveals the crucial mRNAs and miRNAs related to fecundity in the hypothalamus of yunshang black goats during the luteal phase. Animals. (2022) 12:3397. doi: 10.3390/ani12233397
47. Wu Z, Guan K-L. Hippo signaling in embryogenesis and development. Trends Biochem Sci. (2021) 46:51–63. doi: 10.1016/j.tibs.2020.08.008
48. Clark KL, George JW, Przygrodzka E, Plewes MR, Hua G, Wang C, et al. Hippo signaling in the ovary: emerging roles in development, fertility, and disease. Endocr Rev. (2022) 43:1074–96. doi: 10.1210/endrev/bnac013
49. Piccolo S, Dupont S, Cordenonsi M. The biology of YAP/TAZ: hippo signaling and beyond. Physiol Rev. (2014) 94:1287–312. doi: 10.1152/physrev.00005.2014
50. Pavlos NJ, Friedman PA. GPCR signaling and trafficking: the long and short of it. Trends Endocrinol Metab. (2017) 28:213–26. doi: 10.1016/j.tem.2016.10.007
51. Allen JA, Roth BL. Strategies to discover unexpected targets for drugs active at G protein–coupled receptors. Annu Rev Pharmacol Toxicol. (2011) 51:117–44. doi: 10.1146/annurev-pharmtox-010510-100553
Keywords: litter size, whole genome sequencing, Chuanzhong black goats, AMH, reproduction
Citation: Guo C, Ye J, Liu J, Li Z, Deng M, Guo Y, Liu G, Sun B, Li Y and Liu D (2024) Whole-genome sequencing identified candidate genes associated with high and low litter size in Chuanzhong black goats. Front. Vet. Sci. 11:1420164. doi: 10.3389/fvets.2024.1420164
Received: 19 April 2024; Accepted: 09 September 2024;
Published: 20 September 2024.
Edited by:
Kirsten E. Scoggin, University of Kentucky, United StatesReviewed by:
Mahak Singh, ICAR Research Complex for NEH Region, IndiaZhiying Wang, Inner Mongolia Agricultural University, China
Copyright © 2024 Guo, Ye, Liu, Li, Deng, Guo, Liu, Sun, Li and Liu. This is an open-access article distributed under the terms of the Creative Commons Attribution License (CC BY). The use, distribution or reproduction in other forums is permitted, provided the original author(s) and the copyright owner(s) are credited and that the original publication in this journal is cited, in accordance with accepted academic practice. No use, distribution or reproduction is permitted which does not comply with these terms.
*Correspondence: Yaokun Li, eWtsaUBzY2F1LmVkdS5jbg==; Dewu Liu, ZHdsaXVAc2NhdS5lZHUuY24=