- 1Division of Animal Science, West Virginia University, Morgantown, WV, United States
- 2Department of Chemistry, West Virginia University, Morgantown, WV, United States
We utilized plasma proteomics profiling to explore metabolic pathways and key proteins associated with divergent residual body weight gain (RADG) phenotype in crossbred (Angus × Hereford) beef steers. A group of 108 crossbred growing beef steers (average BW = 282.87 ± 30 kg; age = 253 ± 28 days) were fed a high-forage total mixed ration for 49 days in five dry lot pens (20–22 beef steers per pen), each equipped with two GrowSafe8000 intake nodes to determine their RADG phenotype. After RADG identification, blood samples were collected from the beef steers with the highest RADG (most efficient; n = 15; 0.76 kg/d) and lowest RADG (least efficient; n = 15; −0.65 kg/d). Plasma proteomics analysis was conducted on all plasma samples using a nano LC–MS/MS platform. Proteins with FC ≥ 1.2 and false-discovery rate-adjusted p-values (FDR) ≤ 0.05 were considered significantly differentially abundant. The analysis identified 435 proteins, with 59 differentially abundant proteins (DAPs) between positive and negative-RADG beef steers. Plasma abundance of 38 proteins, such as macrophage stimulating 1 and peptidase D was upregulated (FC ≥ 1.2, FDR ≤ 0.05) in positive-RADG beef steers, while 21 proteins, including fibronectin and ALB protein were greater (FC < 1.2, FDR ≤ 0.05) in negative-RADG beef steers. The results of the Gene Ontology (GO) analysis of all the DAPs showed enrichment of pathways such as metabolic processes, biological regulation, and catalytic activity in positive-RADG beef steers. Results of the EuKaryotic Orthologous Groups (KOG) analysis revealed increased abundance of DAPs involved in energy production and conversion, amino acid transport and metabolism, and lipid transport and metabolism in positive-RADG beef steers. The results of this study revealed key metabolic pathways and proteins associated with divergent RADG phenotype in beef cattle which give more insight into the biological basis of feed efficiency in crossbred beef cattle.
Introduction
In animal production, the efficient utilization of feed resources is of paramount concern, mainly due to the high cost of feed (1, 2). To address these challenges, the adoption of phenotypic and genetic selection strategies centered around feed efficiency measurements has become increasingly prevalent. In beef cattle, two widely used indicators of feed efficiency are Residual feed intake (RFI) and Residual body weight gain (RADG). Residual feed intake defined by Koch et al. (2) and Herd et al. (3), quantifies the difference between an animal’s actual and expected dry matter intake (DMI), considering its production and maintenance needs. This involves a regression analysis that considers DMI, body weight (BW), and body weight gain (4). Identification of animals with higher feed efficiency, characterized by negative RFI values, indicates they consume less feed than expected, while positive RFI values signify less efficient animals consuming more feed than expected. Residual body weight gain, following a similar principle as RFI, focuses on the rate of body weight gain, regressed against feed intake and body weight (5). Positive-RADG values denote desirable feed-efficient animals with enhanced body weight gain, while negative values highlight less efficient animals in terms of growth at the same level of DMI (5). Improved RADG can lead to faster growth rates and potentially shorter time to market, which can have significant economic benefits for producers. It is important to note that RADG, like RFI, is influenced by genetic and environmental factors; however, RADG may offer more robust insights in varied environmental conditions by reflecting how growth responds to these conditions.
Over the past decade, high-throughput omics technologies such as proteomics, transcriptomics, and metabolomics have been applied to identify genes, proteins, and biological pathways associated with divergent selection for RFI (6). Proteomics techniques allow for the quantification of protein abundance under different physiological conditions or in response to various stimuli to better understand how protein abundance levels fluctuate during different metabolic states and how animals adapt to changes in their environment or nutritional status (7, 8). Some studies have harnessed proteomic approaches to uncover alterations in protein profiles within blood and skeletal muscle of ruminants (9–11). For instance, Carvalho et al. (10) revealed significant differences in protein abundance within the skeletal muscle of Nellore beef cattle with divergent RFI, suggesting variations in energy expenditure as a contributing factor to observed feed efficiency differences. Additionally, Elolimy et al. (11) indicated that the most feed-efficient beef cattle exhibited a greater abundance of critical proteins involved in cellular protein synthesis. While previous research has focused on critical pathways and proteins influencing RFI, the proteome profiles of beef cattle with divergent RADG phenotype have not been investigated. Our study employed plasma proteomics profiling to investigate metabolic pathways and key proteins associated with divergent RADG phenotype in crossbred beef steers. We hypothesized that beef steers with divergent RADG phenotype would exhibit variations in plasma proteome profiles.
Materials and methods
Animals, feeding, and RADG determination
The research procedures were approved by the Institutional Animal Care and Use Committees of West Virginia (protocol number 2204052569). A group of 108 crossbred (Angus × Hereford) growing beef steers (average BW = 282.87 ± 30 kg; age = 253 ± 28 days) were fed a high-forage total mixed ration (TMR), primarily consisting of corn silage; ground hay; and a ration balancing supplement; CP = 13.2%, NDF = 45.9%, and NEg = 0.93 Mcal/kg; calculated based on NASEM (12). The animals were kept in five dry lot pens (20–22 beef steers per pen) each equipped with two GrowSafe8000 intake nodes (GrowSafe Systems Ltd., Airdrie, Alberta, Canada) for a period of 49 d to determine their RADG phenotype. Details of animal feeding and procedures have been reported in our previous study (13). Briefly, the beef steers were allowed to adapt to the feeding facilities and diet for 15 days. Following this period, individual feed intake was measured for 49 days. Daily BW for each animal, measured using In-Pen Weighing Positions (IPW, Vytelle LLC), was regressed on time using simple linear regression to calculate initial BW, mid-test BW, and average daily gain (ADG). The initial BW, mid-test metabolic BW, and ADG were calculated by regressing the daily BW for each animal using simple linear regression. The ADG of each beef steer was regressed against their daily DMI and mid-test metabolic BW (MMTW = mid-test BW0.75), and the RADG was calculated as the difference between the predicted value of the regression and the actual measured value based on the following equation: Y = β0 + β1X1 + β2X2 + ε, where Y is the ADG (kg/d), β0 is the regression intercept, β1 and β2 are the partial regression coefficients, X1 is the MMTW (kg), X2 is the observed DMI (kg/d) (2, 5). At the end of the feeding trial, the beef steers were ranked based on their RADG coefficients. The most efficient beef steers with the greatest positive-RADG (n = 15) and the least efficient beef steers with the least negative-RADG (n = 15) were identified.
Blood collection and plasma preparation
Upon completion of the feeding trial, blood samples were collected from both positive-RADG beef steers (n = 15) and negative-RADG beef steers (n = 15) via the coccygeal vessel. These samples were collected using 10-mL Vacutainer tubes containing sodium heparin (Vacutainer, Becton Dickinson, Franklin Lakes, NJ, United States). Post-collection, the blood samples were promptly placed on ice and subsequently centrifuged at 2,500 × g for 15 min at 4°C to separate the plasma. The separated plasma samples were then stored at −80°C until further analysis could be conducted.
Protein identification and quantification
The plasma samples underwent a rigorous processing procedure to prepare them for identification and quantification using a nano LC–MS/MS platform (14, 15). The plasma samples were first depleted of high-abundant proteins, such as albumin and immunoglobulin G, using a depletion spin-column procedure (16). The depleted plasma samples were digested with trypsin, and subsequently identified, and quantified by applying the nano LC–MS/MS platform.
The depletion spin column was equilibrated to room temperature, and 10 μL of the sample was added directly to the resin slurry in the column. After capping the column, it was gently mixed to ensure complete suspension of the resin in the solution and underwent a 30-min incubation at room temperature with gentle end-over-end mixing. Following incubation, the column was centrifuged to remove the resin, leaving behind a filtrate containing the sample with removed albumin and IgG. To further refine the sample, ice acetone was added, and the mixture was incubated overnight at −20°C. After centrifugation, the supernatant was discarded, and the remaining acetone was removed. The samples were then dissolved in 50 mM ammonium bicarbonate and centrifuged. Subsequently, the concentrated samples underwent reduction and alkylation before trypsin digestion. Finally, the extracted peptides were lyophilized and resuspended in 20 μL of 0.1% formic acid before LC–MS/MS analysis (17).
Nano LC–MS/MS analysis
For the nano-liquid chromatography (NLC) phase of the analysis, we employed the Nanoflow UPLC: Ultimate 3000 nano UHPLC system from ThermoFisher Scientific, USA. This system achieves high-resolution separations for subsequent mass spectrometry analysis. The nanocolumn setup included a trapping column (PepMap C18, 100 μm × 2 cm, 5 μm) and an analytical column (PepMap C18, 100 Å, 75 μm × 50 cm, 2 μm). A sample volume of 1 μg was loaded onto the system, and the mobile phase consisted of two components: A, which contained 0.1% formic acid in water, and B, which comprised 0.1% formic acid in 80% acetonitrile. The total flow rate was maintained at 250 nL/min. A full scan was conducted over the m/z range of 300–1,650 at a resolution of 60,000 at 200 m/z. The automatic gain control target for the full scan was set at 3e6, ensuring accurate and reliable mass measurements. Additionally, the MS/MS scan operated in Top 20 mode with settings such as a resolution of 15,000 at 200 m/z, an automatic gain control target of 1e5, a maximum injection time of 19 ms, and a normalized collision energy of 28%.
Statistical and bioinformatics analysis
We processed the thirty raw MS files by searching against the Bos taurus protein database using Maxquant (version 1.6.2.6). Parameters included carbamidomethylation (C) (fixed) and oxidation (M) (variable) for protein modifications, trypsin enzyme specificity, and a maximum of 2 missed cleavages. Precursor ion mass tolerance was set to 10 ppm, with an MS/MS tolerance of 0.5 Da. We visualized proteome differences between positive-RADG and negative-RADG beef steers using partial least squares discriminant analysis (PLS-DA) scores plot and identified DAPs through volcano plot analysis (FC ≥ 1.2, FDR-adjusted p-values ≤0.05). Further analysis included GO enrichment and KOG classification of the identified proteome.
GO enrichment analysis
The proteomic dataset’s gene ontology annotations were sourced from the UniProt-GOA database.1 Protein IDs were initially converted to UniProt IDs and subsequently linked to GO IDs. The proteins were then categorized based on their GO annotations into three groups: biological process, cellular component, and molecular function. Enrichment analysis was conducted using Fisher’s exact test to compare differentially abundant proteins to all identified proteins within each category. GO terms with a false discovery rate (FDR) ≤ 0.05 were deemed statistically significant.
Results
The results of the growth performance of the beef steers with divergent RADG phenotype are shown in Table 1. The average RADG values of positive-RADG and negative-RADG beef steers were 0.76 and −0.65 kg/d, respectively. The initial BW and DMI were similar (p > 0.05) for the two groups; however, final body weight and ADG were greater (p = 0.01) in beef steers with positive-RADG (1.25 kg/d) than those with negative-RADG (0.94 kg/d).
A total of 435 proteins were detected and identified (Supplementary Table 1). The PLS-DA score plot showed a clear separation between the two groups of beef steers using the first two principal components with 9.6 and 14% of explained variance (Figure 1), indicating differences in the plasma proteome profile between the two groups. A total of 59 differentially abundant proteins were detected between negative-RADG beef steers and positive-RADG beef steers (Figure 2). Plasma abundance of 38 proteins, including macrophage stimulating 1, peptidase D, plasma serine protease inhibitor, transthyretin, and adiponectin B were greater (FC ≥ 1.2, FDR ≤ 0.05) in positive-RADG beef steers while 21 proteins, including fibronectin, apolipoprotein F, MSH3 protein, and ALB protein, were greater (FC < 1.2, FDR ≤ 0.05) in negative-RADG beef steers.
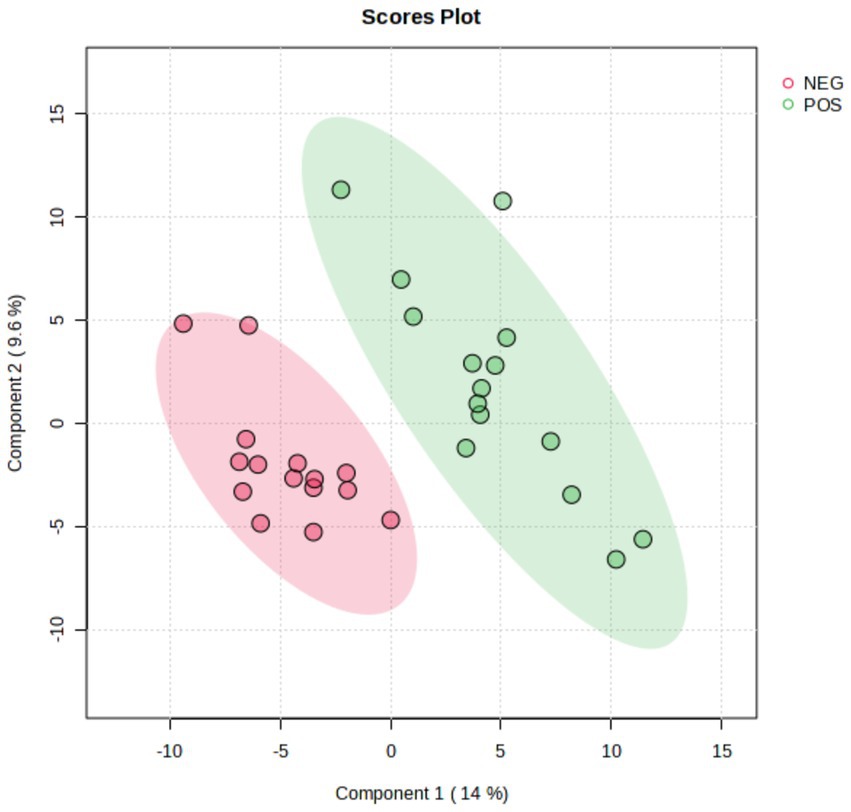
Figure 1. PLS-DA scores plot of the plasma proteome of beef steers with divergent residual body weight gain (RADG) phenotype.
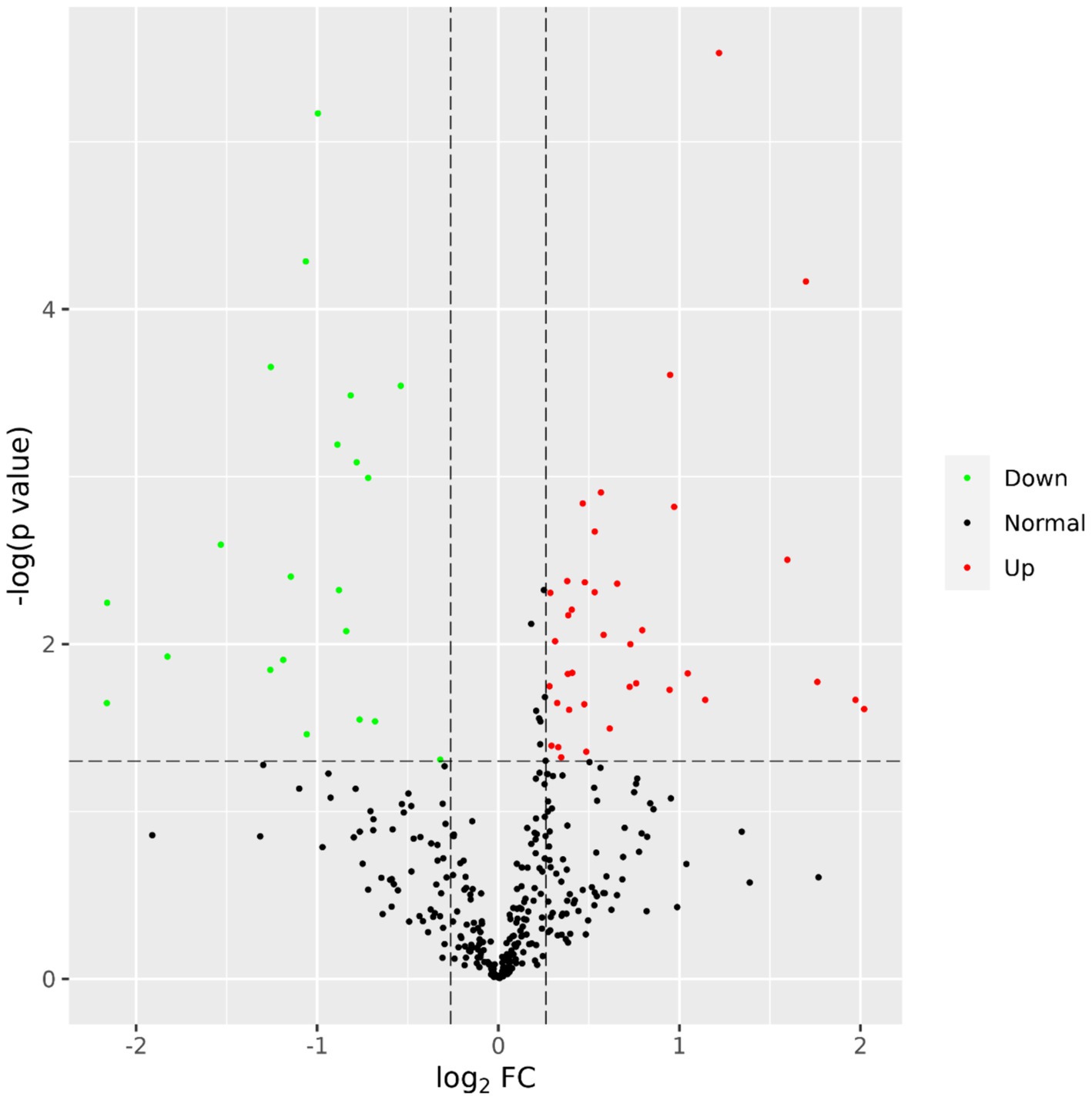
Figure 2. Volcano plot showing the differentially abundant proteins. Proteins with false discovery ratio ≤ 0.05 (red or green) are differentially increased (red dots) or reduced (green dots) in positive- residual body weight gain beef steers, relative to negative- residual body weight gain beef steers.
GO analysis of differentially abundant proteins
The relative abundance of DAPs associated with metabolic processes, single-organism processes, biological regulation, multi-organism processes, biogenesis, and localization, catalytic activity and molecular function regulation were greater in positive-RADG beef steers (Figure 3). However, DAPs associated with processes such as signaling, developmental process, cellular process, organelle, and binding were lower in positive-RADG beef steers, compared to negative-RADG beef steers (Table 2).
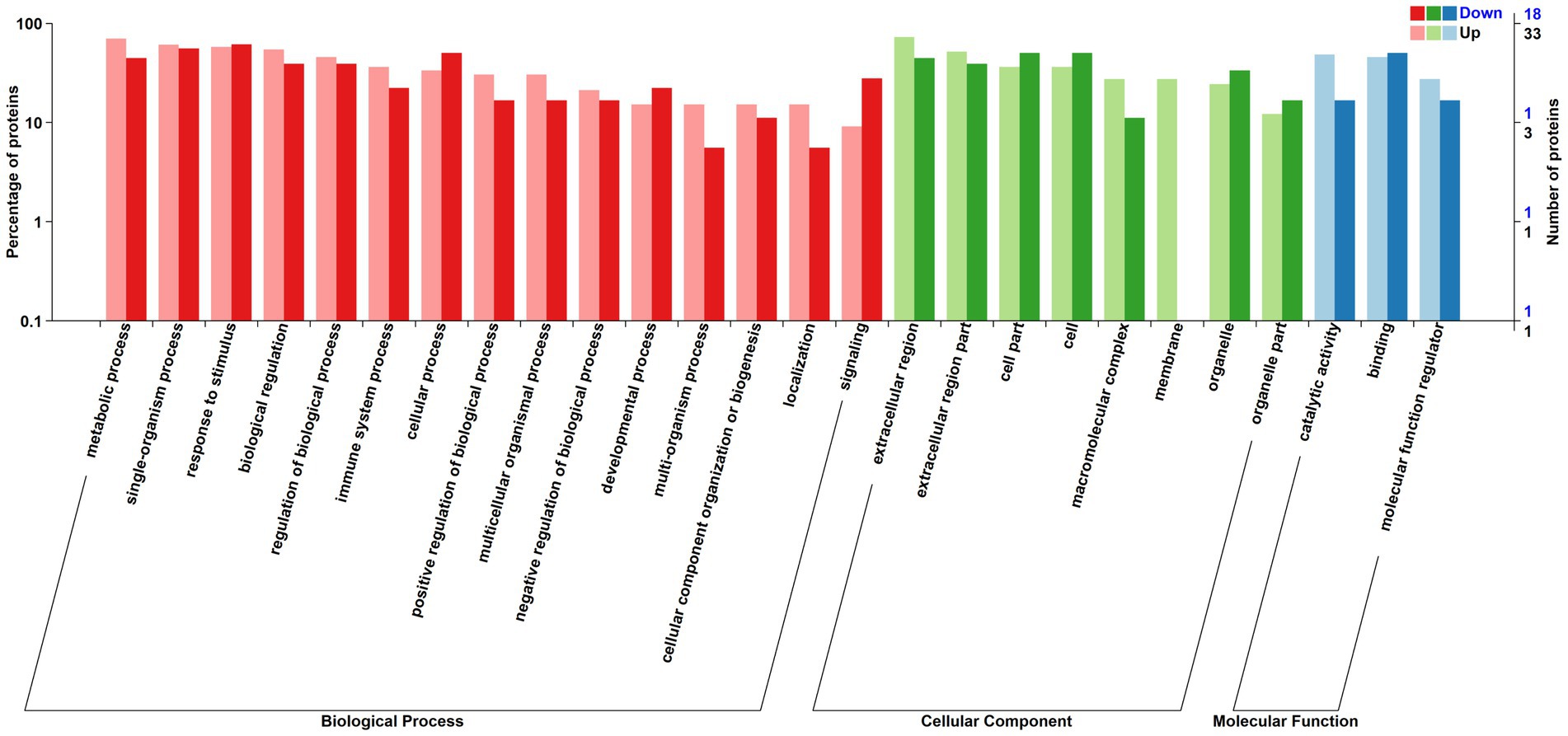
Figure 3. Distribution of differentially abundant proteins annotated in Gene Ontology level. x-axis displays Gene Ontology term; y-axis displays protein count. Up; Differentially abundant proteins associated with biological processes, cellular component, and molecular function greater in positive- residual body weight gain; Down; Differentially abundant proteins associated with biological processes, cellular component, and molecular function greater in negative- residual body weight gain beef steers.
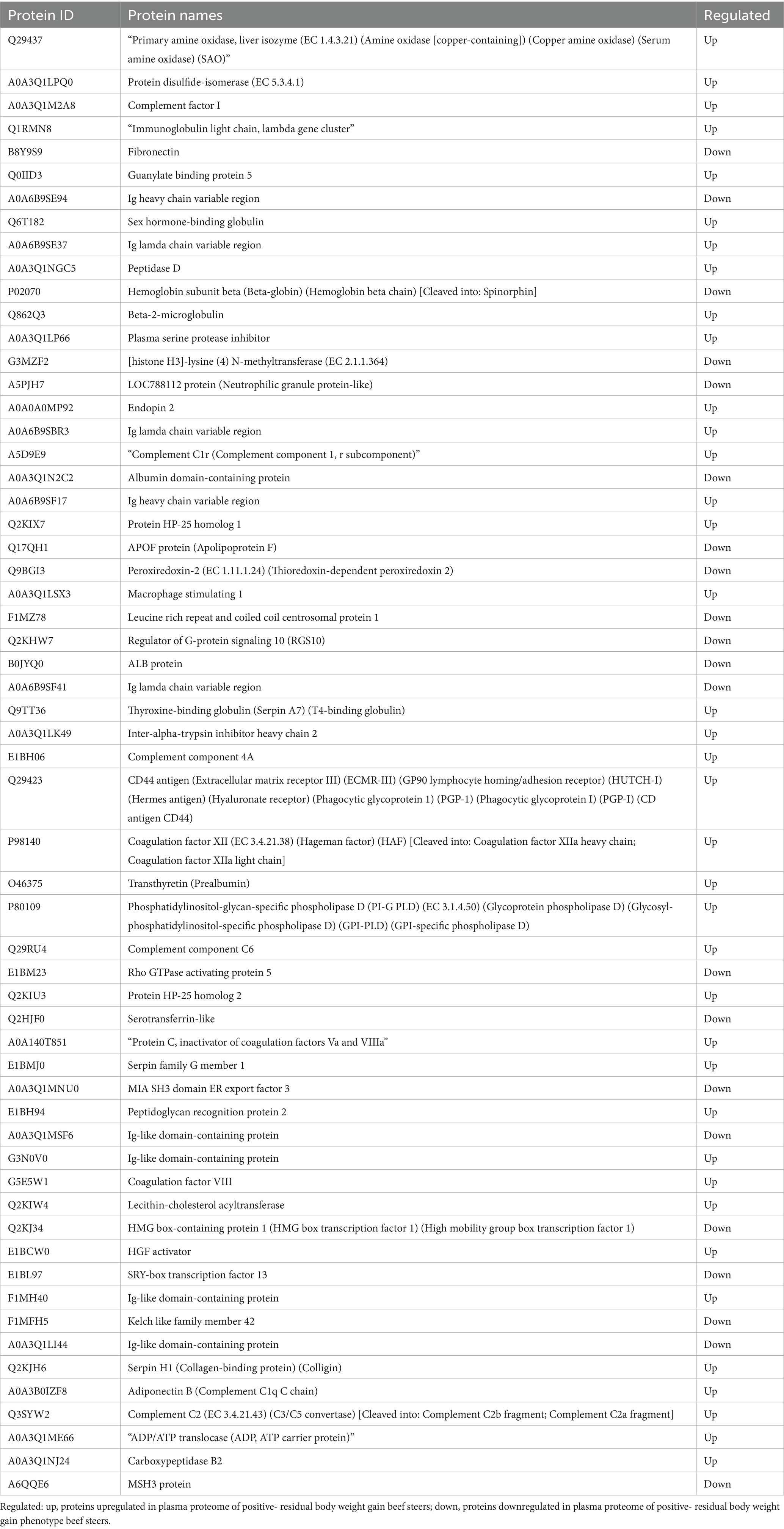
Table 2. List of differentially abundant proteins in the plasma of beef steers with divergent residual body weight gain phenotype.
KOG analysis of differential abundant proteins
The results of the KOG function classifications revealed that a total of 28 DAPs are annotated or classified in KOG and are associated with energy production and conversion, amino acid transport and metabolism, lipid transport and metabolism, transcription, replication recombination and repair, posttranslational modification, protein turnover, secondary metabolites biosynthesis, signal transduction mechanisms, defense mechanisms, and extracellular structures (Figure 4). Out of the 28 DAPs, 24 were greater in positive-RADG beef steers, compared to the negative-RADG beef steers (Supplementary Table 2).
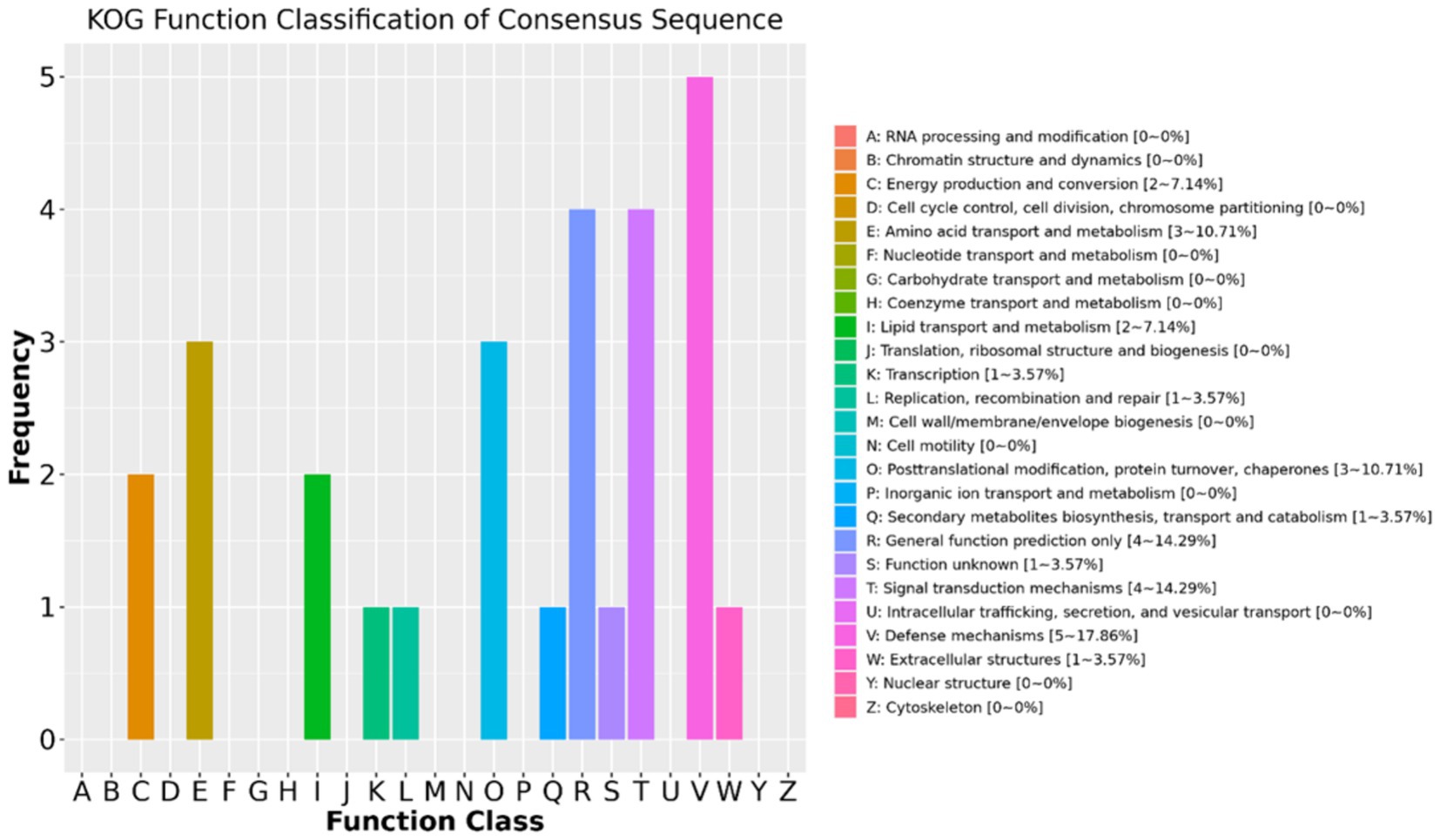
Figure 4. EuKaryotic Orthologous Group classification of the differentially abundant proteins. x-axis displays EuKaryotic Orthologous Group terms; y-axis displays protein count. Only 28 DAPs are annotated (24 of which are greater in positive-RADG beef steers) (see Supplementary Table 2).
Discussion
Determining the metabolic mechanisms associated with feed efficiency, especially using accessible and non-invasive samples like blood, is crucial for the future of livestock production in terms of both profitability and animal welfare. This study aimed to identify metabolic pathways and key proteins associated with divergent RADG classification in beef steers. Using a plasma proteomics profiling approach, we identified and quantified 59 DAPs between the two groups of beef steers.
The number of DAPs involved in metabolic processes, single-organism processes, biological regulation, multi-organism processes, biogenesis, and localization were greater in positive-RADG beef steers. Livestock metabolic processes are fundamental for growth and overall health. These processes involve various biochemical pathways, hormonal regulation, and nutrient utilization. These processes contribute to the efficient breakdown and utilization of nutrients from the feed, ensuring that energy resources are effectively harnessed for growth and production. Upregulation of single-organism and multi-organism processes tailor physiological functions to maximize individual efficiency (18). Biogenesis processes contribute to the efficient formation of cellular structures and organelles. This ensures the proper functioning of cells, tissues, and organs, supporting overall health and performance (19). Biological regulation and localization ensure precise control over various physiological and metabolic pathways, ensuring the targeted delivery of nutrients to specific cellular compartments (20, 21). These processes also contribute to adaptive responses to environmental and nutritional challenges (22). Upregulation of these aforementioned processes in positive-RADG beef steers suggests that energy wastage is reduced, and resources are allocated to processes that positively impact feed efficiency (23).
In the molecular function category, DAPs associated with catalytic activity and molecular function regulation were notably higher in positive-RADG compared to negative-RADG beef steers. Catalytic activity plays a pivotal role in the digestion and absorption of nutrients, facilitating the efficient breakdown of complex molecules in the digestive system to allow for optimal processing of nutrients (24). Molecular function regulation involves the control of gene expression (25) and includes the control of hormonal signaling pathways (26). A balance in signaling could contribute to the coordinated regulation of physiological processes such as nutrient metabolism and energy utilization, which probably explain the increased feed efficiency of positive-RADG beef steers.
The DAPs involved in signaling, developmental processes, cellular processes, and binding were downregulated in positive-RADG beef steers compared to negative-RADG beef steers. Several studies have reported the association of genes related to immune signaling pathways with feed efficiency status in animals. For instance, (27) identified biological processes such as the immune signaling as potentially associated with RFI divergence in beef cattle. Similar findings were reported in several other studies (28–30). Signaling pathways play a crucial role in regulating various physiological processes related to immune function, feed intake, digestion, metabolism, and nutrient utilization in cattle, ultimately influencing feed efficiency (31, 32). However, upregulated signaling pathways and cellular processes often require additional energy for their activation and maintenance (33). Developmental processes such as growth and tissue remodeling require substantial energy resources (34); likewise, cellular processes often require higher energy expenditure for activities such as cell growth, maintenance, and repair (35). When these processes are upregulated, less energy is diverted toward supporting growth and development (34, 36). This increased energy demand could divert resources away from productive functions such as growth and production, ultimately reducing feed efficiency.
Using KOG analysis, DAPs associated with several pathways such as energy production and conversion, amino acid transport and metabolism, lipid transport and metabolism, transcription, replication recombination and repair, protein turnover, and defense mechanisms were greater in positive-RADG beef steers. Energy production and conversion plays a pivotal role in feed efficiency of animals, influencing both economic and environmental aspects of livestock production. This pathway plays a crucial role in converting dietary energy into usable forms that support various physiological functions, including growth, maintenance, and reproduction (37, 38). Previous studies have demonstrated that feed-efficient beef cattle show increased activity in energy production and conversion pathways, including glycolysis, the tricarboxylic acid (TCA) cycle, oxidative phosphorylation, and beta-oxidation (18, 39). Glycolysis is essential in glucose metabolism, converting glucose into pyruvate while generating ATP and NADH (40). Recent research by Xie et al. (41) and Elolimy et al. (42) further highlights the importance of glycolysis in feed efficiency, with both studies reporting elevated levels of glycolytic pathways in feed-efficient cattle. Additionally, the TCA cycle, which oxidizes acetyl-CoA from various nutrients to produce ATP, NADH, and FADH2, plays a crucial role in energy production (43). Previous studies reported an upregulation of the TCA cycle in more feed-efficient beef cattle, indicating their ability to efficiently extract energy from nutrients (42, 44).
The productivity of farm animals is significantly influenced by amino acid metabolism, as it plays integral roles in various biochemical and metabolic processes within animal cells. These processes, including growth, production, and reproduction, underscore the crucial contribution of amino acid metabolism to the overall efficiency and performance of farm animals (45, 46). Research by Elolimy et al. (42) observed that feed-efficient cattle exhibit upregulated metabolic pathways associated with both energy and amino acid metabolism in the rumen and skeletal muscle.
Lipids, including fatty acids, sterols, phospholipids, and triglycerides, serve as essential energy storage molecules in the body (47). Lipid metabolism involves the breakdown and synthesis of fatty acids, triglycerides, cholesterol, and ketone bodies, which play crucial roles in energy production, cell membrane structure, and hormone synthesis (47, 48). A recent study by Taiwo et al. (49) found that feed efficient beef steers exhibit upregulated molecular mechanisms related to fatty acid transport, fatty acid β-oxidation, and mitochondrial ATP synthesis. This suggests an enhanced metabolic capacity, enabling these beef cattle to maximize energy and nutrient utilization from their feed. In feed-efficient cattle, there is an upregulation of lipid metabolism in the liver, resulting in increased synthesis of lipoproteins. These lipoproteins play a crucial role in transporting lipids to various tissues, ensuring an effective delivery of lipids for energy production and other metabolic functions (50). The coordinated upregulation of lipid transport and metabolism pathways in feed-efficient cattle signifies a multifaceted approach to optimize energy utilization, contributing to their overall efficiency in nutrient utilization.
The upregulation of transcription, replication, recombination, and repair, along with posttranslational modification pathways in feed-efficient cattle, signifies intricate molecular adaptations contributing to increased metabolic efficiency and overall performance (51). Upregulated transcription ensures the streamlined synthesis of mRNA, thereby increasing the expression of genes potentially involved in nutrient metabolism and energy utilization. According to Tizioto et al. (52), this increased transcription of key genes associated with feed efficiency optimizes the processing of dietary nutrients and metabolic pathways.
For efficient feed utilization, a dynamic and responsive cellular environment is essential, necessitating the upregulation of DNA replication, recombination, and repair pathways (53). Feed-efficient cattle also exhibit increased activity in posttranslational modification pathways, including phosphorylation, acetylation, and ubiquitination. This heightened activity, as noted by Hunter (54), allows for swift and precise responses to changing nutritional conditions, further contributing to the adaptive capacity of feed-efficient cattle.
Protein turnover, encompassing both synthesis and degradation of proteins within cells (55), was upregulated in feed-efficient cattle. This increased turnover ensures the effective breakdown and synthesis of proteins, directing dietary amino acids toward essential proteins crucial for growth, metabolism, and overall physiological functions (56). Secondary metabolites, organic natural products synthesized by animals through enzymatic cascades, play diverse roles in biological functions such as defense, signaling, and adaptation (57). The upregulation of secondary metabolite biosynthesis in feed-efficient cattle contributes to improved nutrient absorption in the gastrointestinal tract, directly enhancing the utilization of dietary nutrients and positively influencing overall feed efficiency (58). Moreover, specific secondary metabolites, including alkaloids and flavonoids, known for their immunomodulatory properties, further enhance immune function in feed-efficient cattle (59).
Signal transduction mechanisms involve how cells receive and respond to signals from the extracellular environment or other cells (60). The upregulation of these pathways enhances the cattle’s ability to detect changes in nutrient availability, facilitating rapid and precise adjustments in physiological processes. This optimization of dietary nutrient utilization contributes to improved feed efficiency (61). Notably, research suggests that genes involved in neuronal signal transduction potentially influence feed efficiency by modulating feed intake in pigs (62). Extracellular structures, including the extracellular matrix, cell wall, or extracellular vesicles, surround or support cells (63). Upregulated extracellular structures play a role in maintaining the structural integrity of tissues and organs (64). This maintenance could enhance the overall health and well-being of feed-efficient cattle, allowing them to sustain their efficient conversion of feed resources.
Defense mechanisms safeguard cells from harmful agents or stimuli, such as pathogens, toxins, or stress (63). Therefore, the upregulation of defense mechanisms, particularly in the immune system, contributes to improved health in feed-efficient cattle. A robust immune response minimizes energy diversion toward combating infections, redirecting more resources to growth and production, thus positively impacting feed efficiency (65, 66).
Conclusion
The results of this study revealed key metabolic pathways associated with divergent RADG phenotype in beef cattle. Our study provides valuable insights into the molecular underpinnings of feed efficiency in crossbred beef steers, offering a foundation for future research and practical applications in livestock production.
Data availability statement
The original contributions presented in the study are publicly available. This data can be found here: https://massive.ucsd.edu, accession MassIVE MSV000095325.
Ethics statement
The animal study was approved by the Institutional Animal Care and Use Committees of West Virginia. The study was conducted in accordance with the local legislation and institutional requirements.
Author contributions
MI: Writing – review & editing, Writing – original draft, Visualization, Validation, Software, Methodology, Investigation, Formal analysis, Data curation, Conceptualization. GT: Writing – review & editing, Validation, Methodology, Investigation, Data curation. TS: Writing – review & editing, Visualization, Validation, Methodology, Investigation. AA: Writing – review & editing, Validation, Software, Methodology, Investigation. IO: Writing – review & editing, Visualization, Validation, Supervision, Software, Resources, Project administration, Methodology, Investigation, Funding acquisition, Formal analysis, Data curation, Conceptualization.
Funding
The author(s) declare that financial support was received for the research, authorship, and/or publication of this article. This study was funded by West Virginia University Experimental Station in support of U.S. department of Agriculture hatch multi-state regional project W-3010.
Conflict of interest
The authors declare that the research was conducted in the absence of any commercial or financial relationships that could be construed as a potential conflict of interest.
Publisher’s note
All claims expressed in this article are solely those of the authors and do not necessarily represent those of their affiliated organizations, or those of the publisher, the editors and the reviewers. Any product that may be evaluated in this article, or claim that may be made by its manufacturer, is not guaranteed or endorsed by the publisher.
Supplementary material
The Supplementary material for this article can be found online at: https://www.frontiersin.org/articles/10.3389/fvets.2024.1415594/full#supplementary-material
SUPPLEMENTARY TABLE 1 | List of differentially abundant proteins detected and identified between negative-RADG and positive-RADG beef steers.
Footnotes
References
1. Herd, RM, Archer, JA, and Arthur, PF. Reducing the cost of beef production through genetic improvement in residual feed intake: opportunity and challenges to application. J Anim Sci. (2003) 81:9–17. doi: 10.2527/2003.8113_suppl_1E9x
2. Koch, RM, Swiger, LA, Chambers, D, and Gregory, KE. Efficiency of feed use in beef cattle. J Anim Sci. (1963) 22:486–94. doi: 10.2527/jas1963.222486x
3. Herd, RM, Oddy, VH, and Richardson, EC. Biological basis for variation in residual feed intake in beef cattle. 1. Review of potential mechanisms. Aust J Exp Agric. (2004) 44:423–30. doi: 10.1071/EA02220
4. Berry, DP, and Crowley, JJ. Residual intake and body weight gain: a new measure of efficiency in growing cattle. J Anim Sci. (2012) 90:109–15. doi: 10.2527/jas.2011-4245
5. Crowley, JJ, McGee, M, Kenny, DA, Crews, DH, Evans, RD, and Berry, DP. Phenotypic and genetic parameters for different measures of feed efficiency in different breeds of Irish performance-tested beef bulls. J Anim Sci. (2010) 88:885–94. doi: 10.2527/jas.2009-1852
6. Vincent, A, Louveau, I, Gondret, F, Tréfeu, C, Gilbert, H, and Lefaucheur, L. Divergent selection for residual feed intake affects the transcriptomic and proteomic profiles of pig skeletal muscle12. J Anim Sci. (2015) 93:2745–58. doi: 10.2527/jas.2015-8928
7. Kuhla, B, and Metges, CC. Proteomic tools help understanding the metabolic adaptation to negative energy balance in dairy cows In: JW Oltjen, E Kebreab, and H Lapierre, editors. Energy and protein metabolism and nutrition is sustainable animal protection. Wageningen: Wageningen Academic Publishers (2013). 213–21.
8. Reinhardt, TA, Lippolis, JD, Nonnecke, BJ, and Sacco, RE. Bovine milk exosome proteome. J Proteome. (2012) 75:1486–92. doi: 10.1016/j.jprot.2011.11.017
9. Baldassini, WA, Bonilha, SFM, Branco, RH, Vieira, JCS, Padilha, PM, and Lanna, DPD. Proteomic investigation of liver from beef cattle (Bos indicus) divergently ranked on residual feed intake. Mol Biol Rep. (2018) 45:2765–73. doi: 10.1007/s11033-018-4341-2
10. Carvalho, EB, Gionbelli, MP, De Souza Rodrigues, RT, Bonilha, SFM, Newbold, CJ, Guimarães, SEF, et al. Differentially expressed mRNAs, proteins and miRNAs associated to energy metabolism in skeletal muscle of beef cattle identified for low and high residual feed intake. BMC Genomics. (2019) 20:501. doi: 10.1186/s12864-019-5890-z
11. Elolimy, AA, Abdel-Hamied, E, Hu, L, McCann, JC, Shike, DW, and Loor, JJ. RAPID COMMUNICATION: residual feed intake in beef cattle is associated with differences in protein turnover and nutrient transporters in ruminal epithelium. J Anim Sci. (2019) 97:2181–7. doi: 10.1093/jas/skz080
12. [NASEM] National academies of sciences, engineering, and medicine (2016). Nutrient requirements of beef cattle, eighth revised edition. Washington, DC: The national academies press. doi: 10.17226/19014
13. Taiwo, G, Idowu, M, Collins, S, Sidney, T, Wilson, M, Pech-Cervantes, A, et al. Chemical group-based metabolome analysis identifies candidate plasma biomarkers associated with residual feed intake in beef steers. Front Anim Sci. (2022) 2:783314. doi: 10.3389/fanim.2021.783314
14. Lu, Q, Zheng, X, McIntosh, T, Davis, H, Nemeth, JF, Pendley, C, et al. Development of different analysis platforms with LC− MS for pharmacokinetic studies of protein drugs. Anal Chem. (2009) 81:8715–23. doi: 10.1021/ac901991x
15. Wang, X, Shen, S, Rasam, SS, and Qu, J. MS1 ion current-based quantitative proteomics: a promising solution for reliable analysis of large biological cohorts. Mass Spectrom Rev. (2019) 38:461–82. doi: 10.1002/mas.21595
16. Cao, X, Sandberg, A, Araújo, JE, Cvetkovski, F, Berglund, E, Eriksson, LE, et al. Evaluation of spin columns for human plasma depletion to facilitate MS-based proteomics analysis of plasma. J Proteome Res. (2021) 20:4610–20. doi: 10.1021/acs.jproteome.1c00378
17. Bian, Y, Zheng, R, Bayer, FP, Wong, C, Chang, YC, Meng, C, et al. Robust, reproducible and quantitative analysis of thousands of proteomes by micro-flow LC–MS/MS. Nat Commun. (2020) 11:157. doi: 10.1038/s41467-019-13973-x
18. Shabat, S, Sasson, G, Doron-Faigenboim, A, Durman, T, Yaacoby, S, Miller, M, et al. Specific microbiome-dependent mechanisms underlie the energy harvest efficiency of ruminants. ISME J. (2016) 10:2958–72. doi: 10.1038/ismej.2016.62
20. Antonescu, CN, McGraw, TE, and Klip, A. Reciprocal regulation of endocytosis and metabolism. Cold Spring Harb Perspect Biol. (2014) 6:a016964. doi: 10.1101/cshperspect.a016964
21. Toby, G, and Golemis, E. Targeting proteins to specific cellular compartments to optimize physiological activity. Methods Enzymol. (2001) 332:77–87. doi: 10.1016/S0076-6879(01)32193-6
22. Towle, H. Chapter 10 nutritional regulation of hepatic gene expression. Cell Mol Res Stress. (2001) 2:129–43. doi: 10.1016/S1568-1254(01)80012-7
23. Kohn, K, and Aladjem, M. Circuit diagrams for biological networks. Mol Syst Biol. (2006) 2:2006.0002. doi: 10.1038/msb4100044
24. Goodman, BE. Insights into digestion and absorption of major nutrients in humans. Adv Physiol Educ. (2010) 34:44–53. doi: 10.1152/advan.00094.2009
25. Wilkie, GS, Dickson, KS, and Gray, NK. Regulation of mRNA translation by 5′-and 3′-UTR-binding factors. Trends Biochem Sci. (2003) 28:182–8. doi: 10.1016/S0968-0004(03)00051-3
26. Dehkhoda, F, Lee, C, Medina, J, and Brooks, A. The growth hormone receptor: mechanism of receptor activation, cell signaling, and physiological aspects. Front Endocrinol. (2018) 9:35. doi: 10.3389/fendo.2018.00035
27. Higgins, MG, Kenny, DA, Fitzsimons, C, Blackshields, G, Coyle, S, McKenna, C, et al. The effect of breed and diet type on the global transcriptome of hepatic tissue in beef cattle divergent for feed efficiency. BMC Genomics. (2019) 20:1–11. doi: 10.1186/s12864-019-5906-8
28. Paradis, F, Yue, S, Grant, J, Stothard, P, Basarab, J, and Fitzsimmons, C. Transcriptomic analysis by RNA sequencing reveals that hepatic interferoninduced genes may be associated with feed efficiency in beef heifers. J Anim Sci. (2015) 93:3331–41. doi: 10.2527/jas.2015-8975
29. Zarek, CM, Lindholm-Perry, AK, Kuehn, LA, and Freetly, HC. Differential expression of genes related to gain and intake in the liver of beef cattle. BMC Res Notes. (2017) 10:1. doi: 10.1186/s13104-016-2345-3
30. Salleh, MS, Mazzoni, G, Höglund, D, Olijhoek, W, Lund, P, and Løvendahl, P. RNA-seq transcriptomics and pathway analyses reveal potential regulatory genes and molecular mechanisms in high and low residual feed intake in Nordic dairy cattle. BMC Genomics. (2017) 18:258. doi: 10.10s12864-017-3622-9
31. Taniguchi, C, Emanuelli, B, and Kahn, C. Critical nodes in signalling pathways: insights into insulin action. Nat Rev Mol Cell Biol. (2006) 7:85–96. doi: 10.1038/nrm1837
32. Xi, Y, Yang, Z, Wu, F, Han, Z, and Wang, G. Gene expression profiling of hormonal regulation related to the residual feed intake of Holstein cattle. Biochem Biophys Res Commun. (2015) 465:19–25. doi: 10.1016/j.bbrc.2015.07.092
33. Hotamisligil, GS, and Davis, RJ. Cell signaling and stress responses. Cold Spring Harb Perspect Biol. (2016) 8:a006072. doi: 10.1101/cshperspect.a006072
34. Pinet, K, and McLaughlin, KA. Mechanisms of physiological tissue remodeling in animals: manipulating tissue, organ, and organism morphology. Dev Biol. (2019) 451:134–45. doi: 10.1016/j.ydbio.2019.04.001
35. Erecínska, M, and Wilson, D. Regulation of cellular energy metabolism. J Membr Biol. (2005) 70:1–14. doi: 10.1007/BF01871584
36. Ghosh, S, Koerte, A, Serafini, G, Yadav, V, and Rodenfels, J. Developmental energetics: energy expenditure, budgets and metabolism during animal embryogenesis In: Seminars in cell and developmental biology, vol. 138: Academic Press (2023). 83–93.
38. Perl, A. Review: metabolic control of immune system activation in rheumatic diseases. Arthritis Rheumatol. (2017) 69:2259–70. doi: 10.1002/art.40223
39. Kong, R, Liang, G, Chen, Y, Stothard, P, and Guan, L. Transcriptome profiling of the rumen epithelium of beef cattle differing in residual feed intake. BMC Genomics. (2016) 17:592. doi: 10.1186/s12864-016-2935-4
40. Ryan, J, and Archer, S. Emerging concepts in the molecular basis of pulmonary arterial hypertension: part I metabolic plasticity and mitochondrial dynamics in the pulmonary circulation and right ventricle in pulmonary arterial hypertension. Circulation. (2015) 131:1691–702. doi: 10.1161/CIRCULATIONAHA.114.006979
41. Xie, Y, Sun, H, Xue, M, and Liu, J. Metagenomics reveals differences in microbial composition and metabolic functions in the rumen of dairy cows with different residual feed intake. Anim Microbiome. (2022) 4:19. doi: 10.1186/s42523-022-00170-3
42. Elolimy,, Ahmed, A, Mohamed Zeineldin,, Mohamed Abdelmegeid,, Alzahraa, A, Abdelatty,, et al. Abdulrahman S. Alharthi, Mohammed H. Bakr, Mona MMY Elghandour, Abdelfattah ZM Salem, and Juan J. Loor. “Metabolomics and Proteomics Signatures in Feed-Efficient Beef and Dairy Cattle.” Sustainable Agriculture Reviews 54: Animal Biotechnology for Livestock Production 1 (2021). 153–65.
43. Sonnewald, U. Glutamate synthesis has to be matched by its degradation – where do all the carbons go? J Neurochem. (2014) 131:399–406. doi: 10.1111/jnc.12812
44. Elolimy, AA, Abdelmegeid, M, McCann, JC, Shike, DW, and Loor, JJ. Residual feed intake in beef cattle and its association with carcass traits, ruminal solid-fraction bacteria, and epithelium gene expression. J Anim Sci Biotechnol. (2018) 9:67. doi: 10.1186/s40104-018-0283-8
45. Floc’h, N, Wessels, A, Corrent, E, Wu, G, and Bosi, P. The relevance of functional amino acids to support the health of growing pigs. Anim Feed Sci Technol. (2018) 245:104–16. doi: 10.1016/J.ANIFEEDSCI.2018.09.007
46. Taiwo, G, Idowu, M, Denvir, J, Pech-Cervantes, AA, and Ogunade, IM. Identification of key pathways associated with residual feed intake of beef cattle based on whole blood transcriptome data analyzed using gene set enrichment analysis. Front Vet Sci. (2022) 9:848027. doi: 10.3389/fvets.2022.848027
47. Ertunc, M, and Hotamışlıgil, G. Lipid signaling and lipotoxicity in metaflammation: indications for metabolic disease pathogenesis and treatment. J Lipid Res. (2016) 57:2099–114. doi: 10.1194/jlr.R066514
48. Rui, L. Energy metabolism in the liver. Compr Physiol. (2014) 4:177–97. doi: 10.1002/cphy.c130024
49. Taiwo, GA, Idowu, M, Wilson, ME, Pech-Cervantes, AA, Estrada-Reyes, ZM, and Ogunade, IM. Residual feed intake in beef cattle is associated with differences in hepatic mRNA expression of fatty acid, amino acid, and mitochondrial energy metabolism genes. Front Anim Sci. (2022) 3:828591. doi: 10.3389/fanim.2022.828591
50. Artegoitia, V, Foote, A, Lewis, R, and Freetly, H. Metabolomics profile and targeted Lipidomics in multiple tissues associated with feed efficiency in beef steers. ACS Omega. (2019) 4:3973–82. doi: 10.1021/acsomega.8b02494
51. Smith, E, and Shilatifard, A. The chromatin signaling pathway: diverse mechanisms of recruitment of histone-modifying enzymes and varied biological outcomes. Mol Cell. (2010) 40:689–701. doi: 10.1016/j.molcel.2010.11.031
52. Tizioto, P, Coutinho, L, Oliveira, P, Cesar, A, Diniz, W, Lima, A, et al. Gene expression differences in longissimus muscle of Nelore steers genetically divergent for residual feed intake. Sci Rep. (2016) 6:39493. doi: 10.1038/srep39493
53. West, S. Molecular views of recombination proteins and their control. Nat Rev Mol Cell Biol. (2003) 4:435–45. doi: 10.1038/nrm1127
54. Hunter, T. The age of crosstalk: phosphorylation, ubiquitination, and beyond. Mol Cell. (2007) 28:730–8. doi: 10.1016/J.MOLCEL.2007.11.019
55. Abukhalaf, M, Proksch, C, Thieme, D, Ziegler, J, and Hoehenwarter, W. Changing turn-over rates regulate abundance of tryptophan, GS biosynthesis, IAA transport and photosynthesis proteins in Arabidopsis growth defense transitions. BMC Biol. (2023) 21:249. doi: 10.1186/s12915-023-01739-3
56. Boisclair, Y, Bell, A, Dunshea, F, Harkins, M, and Bauman, D. Evaluation of the arteriovenous difference technique to simultaneously estimate protein synthesis and degradation in the hindlimb of fed and chronically underfed steers. J Nutr. (1993) 123:1076–88. doi: 10.1093/JN/123.6.1076
57. Hossain, R, Quispe, C, Saikat, ASM, Jain, D, Habib, A, Janmeda, P, et al. Biosynthesis of secondary metabolites based on the regulation of MicroRNAs. Biomed Res Int. (2022) 2022:1–20. doi: 10.1155/2022/9349897
58. Bibb, M. Regulation of secondary metabolism in streptomycetes. Curr Opin Microbiol. (2005) 8:208–15. doi: 10.1016/J.MIB.2005.02.016
59. Tallei, TE, Fatimawali, F, Yelnetty, A, Niode, NJ, Kusumawaty, D, Idroes, R, et al. Unveiling the immunomodulatory mechanisms of pineapple metabolites: a multi-modal computational analysis using network pharmacology, molecular docking, and molecular dynamics simulation. J Adv Biotechnol Exp Ther. (2024) 7:1. doi: 10.5455/jabet.2024.d01
60. Cashin, P, Goldsack, L, Hall, D, and O’Toole, R. Contrasting signal transduction mechanisms in bacterial and eukaryotic gene transcription. FEMS Microbiol Lett. (2006) 261:155–64. doi: 10.1111/j.1574-6968.2006.00295.x
61. Cohen, G, Ren, R, and Baltimore, D. Modular binding domains in signal transduction proteins. Cell. (1995) 80:237–48. doi: 10.1016/0092-8674(95)90406-9
62. Hou, Y, Hu, M, Zhou, H, Li, C, Li, X, Liu, X, et al. Neuronal signal transduction-involved genes in pig hypothalamus affect feed efficiency as revealed by transcriptome analysis. Biomed Res Int. (2018) 2018:1–10. doi: 10.1155/2018/5862571
63. Zeng, Y, Qiu, Y, Jiang, W, Shen, J, Yao, X, He, X, et al. Biological features of extracellular vesicles and challenges. Front Cell Dev Biol. (2022) 10:816698. doi: 10.3389/fcell.2022.816698
64. Rosso, F, Giordano, A, Barbarisi, M, and Barbarisi, A. From cell–ECM interactions to tissue engineering. J Cell Physiol. (2004) 199:174–80. doi: 10.1002/jcp.10471
65. Burvenich, C, Bannerman, D, Lippolis, J, Peelman, L, Nonnecke, B, Kehrli, M, et al. Cumulative physiological events influence the inflammatory response of the bovine udder to Escherichia coli infections during the transition period. J Dairy Sci. (2007) 90:E39–54. doi: 10.3168/JDS.2006-696
Keywords: feed efficiency, proteome, metabolic process, cattle, immune signaling
Citation: Idowu M, Taiwo G, Sidney T, Adewoye A and Ogunade IM (2024) Plasma proteomic analysis reveals key pathways associated with divergent residual body weight gain phenotype in beef steers. Front. Vet. Sci. 11:1415594. doi: 10.3389/fvets.2024.1415594
Edited by:
Luciana Regitano, Brazilian Agricultural Research Corporation (EMBRAPA), BrazilReviewed by:
Kate Keogh, Teagasc Grange Animal and Bioscience Research Department (ABRD), IrelandAline Morais, Embrapa Southeast Livestock, Brazil
Copyright © 2024 Idowu, Taiwo, Sidney, Adewoye and Ogunade. This is an open-access article distributed under the terms of the Creative Commons Attribution License (CC BY). The use, distribution or reproduction in other forums is permitted, provided the original author(s) and the copyright owner(s) are credited and that the original publication in this journal is cited, in accordance with accepted academic practice. No use, distribution or reproduction is permitted which does not comply with these terms.
*Correspondence: Ibukun M. Ogunade, SWJ1a3VuLm9ndW5hZGVAbWFpbC53dnUuZWR1