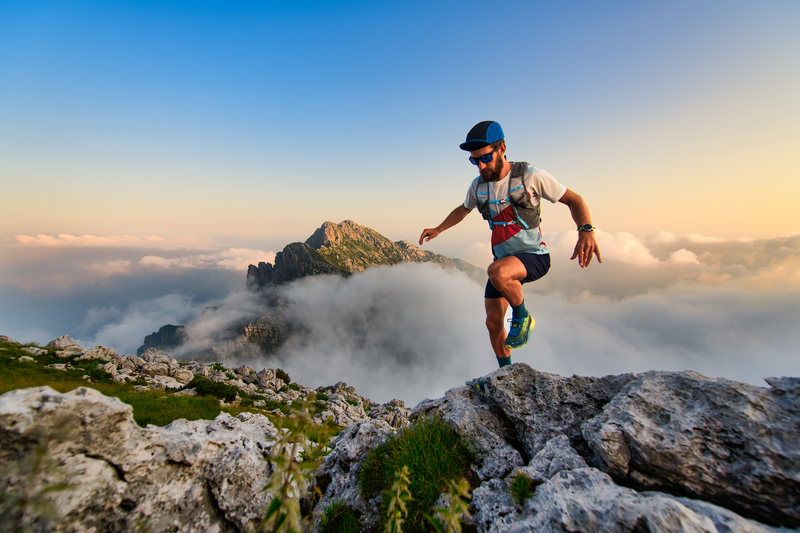
94% of researchers rate our articles as excellent or good
Learn more about the work of our research integrity team to safeguard the quality of each article we publish.
Find out more
ORIGINAL RESEARCH article
Front. Vet. Sci. , 30 October 2024
Sec. Veterinary Epidemiology and Economics
Volume 11 - 2024 | https://doi.org/10.3389/fvets.2024.1410878
Newcastle disease virus (NDV) is a significant poultry pathogen, causing ongoing economic losses in China’s poultry industry. To understand its circulation and risk factors associated with farm infection, we carried out an epidemiological study on 1,065 farms across 18 provinces from 2019 and 2022. We collected a total of 43,696 swabs and tested them for NDV using an RT-PCR that targets the partial F gene. The overall bird-level NDV prevalence in the 4 years (2019, 2020, 2021, and 2022) were 1.49% (95%CI: 1.27, 1.71%), 1.24% (95%CI: 1.04, 1.44%), 0.59% (95%CI: 0.45, 0.74%), and 0.44% (95%CI: 0.31, 0.58%) respectively, while the farm-level prevalence of the 4 years were 11.27% (95%CI: 7.93, 14.60%), 13.85% (95%CI: 10.10, 17.61%), 12.63% (95%CI: 8.00, 17.26%), and 10.71% (95%CI: 6.38, 15.04%), respectively. The result also showed a high degree of variation in farm-level prevalence (ranging from 0 to 90%) across the provinces. A multiple logistic regression analysis revealed that four factors, namely layer farm (OR = 2.282, 95% CI: 1.211, 4.298), broiler farm (OR = 2.339, 95% CI: 1.206, 4.534), using mixed feed (OR = 2.973, 95% CI: 1.962, 4.505), and indoor housing with some free-range access (OR = 1.788, 95%CI: 1.096, 2.917), increased the risk of NDV infection on farms. We isolated 104 NDVs, which were all classified into Class II by the phylogenetic analysis, but from two genotypes: four belonged to genotype I, while the remaining 100 mainly belonged to genotype II, indicating that the circulating NDVs were primarily LaSota-like low pathogenic viruses. We used random forest algorithm to established an occurrence risk model, The accuracy of the model was 90.81%. This large-scale investigation revealed NDV prevalence at bird, farm, and province levels over the 4 years. It also identified risk factors associated with farm NDV infections. The findings provide new insights into the epidemiology of NDV in China and offer references for global NDV control.
Newcastle disease (ND) is a highly contagious viral disease in poultry caused by Newcastle disease virus (NDV). It leads to significant economic losses due to its high morbidity and mortality rates (1). First reported in Java, Indonesia in 1926, the disease has since spread to nearly all poultry production areas worldwide (2, 3). In China, the disease has been reported in almost every province. To combat ND, attenuated Lasota-based vaccines are used on all commercial chicken farms, effectively preventing large-scale outbreaks. However, ND has not been eradicated from China’s poultry populations and remains a significant problem for the poultry industry. Therefore, consistently monitoring of its circulation is critical to the current control program.
NDV is a member of Avulavirinae within the Paramyxoviridae family (4, 5). NDV has a wide host range among avian species and can infect all domestic poultry. Infected birds are the primary sources of infection, with transmission mainly occurring through respiratory and digestive routes (6, 7). The NDV genome is a single-stranded, negative-sense, non-segmented RNA molecule that encodes seven major viral proteins, including nucleocapsid protein (NP), phosphoprotein (P), matrix protein (M), fusion protein (F), and haemagglutinin-neuraminidase (HN) (8, 9). Based on the F gene’s phylogeny, NDVs are classified into Class I and II, with Class II further divided into at least 20 genotypes (10–14). To date, all NDV genotypes have been identified in China’s poultry populations, with genotype VII being the most prevalent (11, 15).
Recently, epidemiological studies on NDV have primarily focused on the molecular identification of new isolates (11, 16), while the NDV prevalence and risk factors associated with farm infection in China have not been thoroughly examined.
Identifying risk factors is crucial for effective prevention and control of infectious diseases, including ND. Powerful tools like remote sensing (RS), Geographic Information Systems (GIS), and machine learning methods are now used to achieve this goal. Traditional methods of environmental data collection, such as manual gathering, are often challenging. Currently, many researchers use RS technology to monitor disease-related environmental factors—temperature, precipitation, and vegetation coverage—to analyze the primary spatial and temporal characteristics of affected areas (17, 18). GIS is widely used in epidemiological research to display epidemic data on maps, providing a more intuitive understanding of the spatial and temporal distribution of outbreaks. It also helps identify disease patterns and illustrate relationships between environmental factors and diseases. Machine learning methods are extensively employed to analyze data, uncover potential connections between disease occurrence and various factors, and establish risk assessment models (19).
In this study, we conducted an epidemiological study on 1,065 farms across 18 provinces of China from 2019 and 2022. The aim was to estimate the prevalence of NDV, identify the potential risk factors associated with farm NDV infections and assess the risk of disease occurrence under different farming conditions. Our findings offer an updated understanding of NDV circulation status in China, which is useful in formulating control policies.
This epidemiological study was conducted from 2019 to 2022. Samples were collected from 1,065 farms across 18 provinces of China (Figure 1).
Figure 1. Map of China showing locations of sample collection, 2019–2022. The dots mark the farm location.
A total of 43,696 chicken samples, including 15,744 oropharyngeal and 27,952 cloacal swabs. The quantity of samples taken from each farm varied, ranging from eight to 200 (Figure 2). Immediately after collection, each swab was immersed in a viral medium (containing 1 mL sterile phosphate buffered saline, 2000 U/mL penicillin, 2 mg/mL streptomycin, and 10% glycerol) contained in a 2.0 mL EP tube. These samples were then frozen at −20°C for at least 2 h before being transported to our lab in a sample transport container supplied with dry ice. Detailed information about the samples is provided in Table 1.
During sample collection, the investigator administered a questionnaire to collect data about the farm. The information collected mainly included the date of sampling, farm location, farm size, type of feed, feed source, water source, water disinfection methods, excrement management, and treatment of dead animals.
To extract viral RNA from the samples, the swabs were vortexed for 30 s, the cotton swabs were discarded, and the samples were then centrifugated at 3,000 g for 5 min. A 200 μL volume supernatant was used for viral RNA extraction using the EasyPure Viral DNA/RNA Kit (Transgen, China) The partial F gene of NDV was detected using an RT-PCR designed to amplify a 535 bp fragment with a forward primer 5’-ATGGGCYCCAGAYCTTCTAC-3′ and a reverse primer 5’-CTGCCACTGCTAGTTGTGATAATCC-3′. The detection process used the EasyScript One-step RT-PCR SuperMix (Transgen, China) under the following conditions: transcription at 42°C for 45 min, initial PCR activation for 3 min at 95°C, denaturation at 94°C for 30 s, annealing at 55°C for 30 s, extension at 72°C for 45 s with 30 cycles, and a final extension at 72°C for 7 min. The PCR products were tested by agarose gel electrophoresis.
A farm is considered positive for NDV if at least one sample tests positive in the RT-PCR test.
To isolate NDV, 428 positive samples were incubated in specific-pathogen-free (SPF) embryonated chicken eggs. In brief, three eggs were inoculated with 0.2 mL of each sample via the allantoic route. The eggs were observed every 12 h and allantoic fluids were collected after 48 h for hemagglutination assay (HA). The HA-positive allantoic fluids were then amplified using the previously described RT-PCR method, and the PCR products were sequenced.
To analyze the phylogeny of the NDVs isolated in this study, we downloaded the F gene sequences of 34 reference NDVs from the GenBank database. We aligned all the sequences using the ClustalW algorithm implemented in MEGA 7 (20). We then built a maximum-likelihood tree with 1,000 bootstrap replicates.
The questionnaire and PCR results were organized and summarized as numbers and percentages to estimate the prevalence of NDV by year and region. Initially, a univariate analysis was performed on the variables. Any variables with a p-value of less than or equal to 0.3 were included in the multivariable regression. A p-value less than 0.05 was considered statistically significant, and less than 0.01 was considered highly significant. All data analyses were conducted using SPSS (Version 19).
An occurrence risk model was established using random forest algorithm in the R package caret.1 The model randomly selected 70% data from 1,065 farms data set for training and the remaining 30% data used to validate the model. The random forest algorithm training multiple decision trees to generated a model and evaluated the model using a 10-fold cross-validation method.
We used an RT-PCR method to test 43,696 swab samples for the presence of NDV, as described in the method section. We found that 0.98% (428/43,696) tested positive for the virus’s F gene. The bird-level prevalence between 2019 and 2022 were 1.49% (95%CI: 1.27, 1.71%), 1.24% (95%CI: 1.04, 1.44%), 0.59% (95%CI: 0.45, 0.74%), and 0.44% (95%CI: 0.31, 0.58%), respectively, as depicted in Figure 3A. The farm-level prevalence for the 4 years were 11.27% (95%CI: 7.93, 14.60%), 13.85% (95%CI: 10.10, 17.61%), 12.63% (95%CI: 8.00, 17.26%), and 10.71% (95%CI: 6.38, 15.04%), respectively, as shown in Figure 3B. These results suggest a gradual decrease in NDV infection in chickens over the four-year period in China. However, infection rates at the farm level remained relatively stable.
Figure 3. Prevalence of NDV in China from 2019 to 2022. (A) Bird-level NDV prevalence. (B) Farm-level NDV prevalence.
We found a high variation in the farm-level prevalence (ranging from 0 to 90%) among provinces (Figure 4A), yet bird-level prevalence remained relatively stable (ranging from 0 to 10%) in the provinces (Figure 4B). This suggests that low-level NDV infection is widespread across many farms in certain provinces.
Figure 4. Prevalence of NDV in different provinces in China. (A) Bird-level NDV prevalence by provinces. (B) Farm-level NDV prevalence by provinces.
The most of positive farms were found in Heilongjiang, Hainan, Hubei and Jiangsu provinces. Some provinces such as Fujian, Guangdong, Henan, Shaanxi, Shanghai, Zhejiang has no NDV positive farm detected for 4 consecutive years (Table 2). This data reveals a diverse NDV circulation status across different regions in China (Figure 5).
We obtained the situation of farms by the questionnaire. Among these farms, 15.95% broiler farms were positive, 18.04% indoor housing with some free-rang access, 23.50% mixed feed, 12.74% drinking well water and 13.73% did not clean water farms were positive. We calculate the p-value of NDV infection in farms under different factors. Following the methods mentioned earlier, we ultimately selected five factors include farm type, housing, feed type, feed source and clean water for subsequent risk factor estimate. Detailed information about the farms is provided in Table 3. Association of farm level risk factors with ND infection
We conducted logistic regression analyses to identify the potential risk factors associated with NDV infection on farms. Initially, we calculated the p-value of all the factors and selected those with an p < 0.3 for the final multiple logistic regression model calculation. The model revealed an increased risk of NDV infection was associated with layer farm (OR = 2.282, 95% CI: 1.211, 4.298), broiler farm (OR = 2.339, 95% CI: 1.206, 4.534), use of mixed feed (OR = 2.973, 95% CI: 1.962, 4.505), indoor housing with some free-range access (OR = 1.788, 95%CI: 1.096, 2.917) (Table 4).
We use a random forest algorithm to establish an occurrence risk model that includes 8 risk factors. There were 1,065 records in the model and 130 of them were positive, the mtry and ntree values were 6 and 300, respectively. The model adjusted mtry and ntree values to maximize the accuracy of the model. The accuracy of the model was 90.81%, indicating that the model can effectively predict the risk of NDV infection. The model predicted several farming situations with a probability of infection exceeding 80% (Table 5).
To determine the genotypes of the circulating NDVs in chicken populations, we isolated NDV from 428 RT-PCR-positive samples in SPF eggs. We obtained 104 NDVs and sequenced their F genes. We submitted the sequences to the GenBank (Accession No: PP238275-PP238378). A phylogenetic analysis, based on the F gene nucleotide sequences of these viruses and 34 reference strains from the GenBank database, revealed that all the newly isolated viruses belonged to Class II but were divided into two genotypes. Four isolates from Hubei Province were assigned to genotype I, while the remaining 100 isolates from Shandong Province (9) and Jiangsu Province (91) were assigned to genotype II, which also included the low pathogenic NDV strain LaSota (Figure 6). This suggests that the dominant circulating NDVs are of low pathogenicity to chickens.
Figure 6. The maximum-likelihood phylogenetic tree based on the partial F gene sequences of NDVs isolated in this study and 34 reference strains. Red branches and dots mark the viruses isolated in this study.
Vaccines are widely used on chicken farms in China to prevent NDV infection. However, ND remains a major issue causing significant losses in the poultry industry. Therefore, close monitoring of NDV’s circulation is crucial for adapting disease control policies. Our four-year investigation showed that NDV is relatively well controlled in China. The overall low bird- and farm-level prevalence were about 1 and 10% respectively, which are lower than in countries like Egypt and Vietnam, and similar to India and Africa (21–23). We observed a decrease in bird-level prevalence, suggesting improved farm management. However, there were significant differences at the provincial level, with farm-level prevalences ranging from 0% to approximately 90%. This suggests that NDV circulation remains severe in certain regions. Positive farms were mainly concentrated in Hainan, Heilongjiang, Hubei, and Jiangsu provinces, this may be closely related to the aquaculture situation in these provinces. These four provinces have a large number of livestock and poultry products (data sources from https://www.stats.gov.cn/sj/ndsj/) and the breeding situation was complex. Consequently, NDV control should primarily focus on reducing farm-level infections in these extensively affected regions.
Although the overall prevalence of NDV at bird- and farm-level remained stable, it varied at the provincial level. A logistic regression analysis was conducted to identify potential risk factors associated with NDV infections on farms. The analysis revealed that the prevalence of NDV in layer and broiler farms was significantly higher (p < 0.05) than in breeding farms, which is likely the result of good biosecurity practices in breeding farms. Farms where chickens were raised indoors but had some free-range outdoor access were found to have 1.788 times higher odds of NDV infection (p = 0.02) than intensive farms. This suggests that outdoor access may increase the chance of effective contact between poultry and carriers of the pathogen, such as infected poultry and wild birds, or contaminated personal and other materials (22). Farms that used mixed feed observed a 2.973 times higher rate of NDV infection (p < 0.01) than those using formula feed. Compared to mixed feed, commercial complete formula feeds contain complete nutritional components, which may be more effective in improving poultry’s physical fitness, thereby increasing their resistance to viral infections. To reduce the prevalence of NDV infection in provinces with high farm-level prevalence, these identified risk factors could be targeted for control policy improvement.
The random forest algorithm has been widely used in the field of biology and it has been successfully applied to predict the risk of various diseases occurring (19, 24, 25). The accuracy of the model prediction in this study was 90.81%, indicating that the model can effectively predict the occurrence of NDV infection in different farms. As monitoring continues, the supplemented dataset can continuously optimize model parameters and improve model accuracy. Model prediction results will provide support for disease warning and reference for disease prevention and control.
In this study, we learned about the situation of the farm through the questionnaire, but did not consider the environmental factor data, which may lead us to ignore some risk factors related to the spread of NDV. If we obtained the data of environmental factors through RS and use the GIS spatial analysis tool, it is possible to grasp the spatial and temporal distribution characteristics of diseases and more accurately explain the relationship between NDV and risk factors (26). It is also possible to optimize the occurrence risk model to provide more accurate early warning information.
Based on the F gene’s phylogeny, NDVs have been divided into at least 20 subtypes. Previous studies found that all NDV genotypes have been identified in China, with most recently circulating NDVs primarily from genotype VII (11, 27). In contrast, our study revealed geographical differences among NDV genotypes. Of these 104 NDVs isolated in this study, four belonged to genotype I, and the rest were predominantly from genotype II. These were genetically closely related to the low pathogenic strain LaSota, which differs from other studies findings. This discrepancy may be because our samples were primarily from clinically healthy chickens, unlike other studies aimed at isolating NDVs from diseased chickens, which increases the chance of obtaining virulent strains (28, 29). In recent years, virulent NDVs from genotype VI, VII VIII, IX, and XII have been sporadically reported, but there are few reports on the isolation of genotype I viruses in China (15, 16, 22, 30, 31). Phylogenetic analysis of NDV has important guiding significance for understanding virus mutations and vaccine development. Therefore, long-term monitoring is necessary to prevent outbreaks of diseases.
From 2019 to 2022, a prevalence of NDV at farm-level was high. This means that ND continues to be endemic in different provinces of China and pose a threat to the flock. Farms infection with NDV could be attributed to these factors: layer farm, broiler farm, using mixed feed, and indoor housing with some free-range access. These results provide a reference for ND prevention and control. Hence, continuous pathogen surveillance in poultry flocks should be conducted to prevent the spread of low-pathogenicity strains, and preventive measures should be improved based on risk factors.
The data that support the findings of this study are available on request from the corresponding author (d2FuZ2ppbmdmZWlAY2Fhcy5jbg==), upon reasonable request.
The animal study was approved by the Animal Experimentation Ethics Committee of Harbin Veterinary Research Institute. The study was conducted in accordance with the local legislation and institutional requirements.
SW: Formal analysis, Investigation, Methodology, Visualization, Writing – original draft. LW: Conceptualization, Data curation, Formal analysis, Project administration, Writing – original draft. JW: Conceptualization, Data curation, Funding acquisition, Project administration, Resources, Supervision, Writing – original draft, Writing – review & editing. ZZ: Conceptualization, Project administration, Supervision, Validation, Writing – original draft, Writing – review & editing.
The author(s) declare that financial support was received for the research, authorship, and/or publication of this article. This work was supported by the Central Public-interest Scientific Institution Basal Research Fund (Y2023LM07).
The authors declare that the research was conducted in the absence of any commercial or financial relationships that could be construed as a potential conflict of interest.
The author(s) declared that they were an editorial board member of Frontiers, at the time of submission. This had no impact on the peer review process and the final decision.
All claims expressed in this article are solely those of the authors and do not necessarily represent those of their affiliated organizations, or those of the publisher, the editors and the reviewers. Any product that may be evaluated in this article, or claim that may be made by its manufacturer, is not guaranteed or endorsed by the publisher.
1. Alexander, DJ. Newcastle disease and other avian paramyxoviruses. Rev Sci Tech. (2000) 19:443–62. doi: 10.20506/rst.19.2.1231
2. Abozaid, KGA, and Abdel-Moneim, AS. Epidemiological surveillance of Newcastle disease virus in Egypt - a 6-year cohort study. Trop Anim Health Prod. (2022) 54:243. doi: 10.1007/s11250-022-03234-3
3. Wang, J, Yu, X, Zheng, D, Zhao, Y, Lv, Y, Shu, B, et al. Continuous surveillance revealing a wide distribution of class I Newcastle disease viruses in China from 2011 to 2020. PLoS One. (2022) 17:e0264936. doi: 10.1371/journal.pone.0264936
4. Amarasinghe, GK, Ayllón, MA, Bào, Y, Basler, CF, Bavari, S, Blasdell, KR, et al. Taxonomy of the order Mononegavirales: update 2019. Arch Virol. (2019) 164:1967–80. doi: 10.1007/s00705-019-04247-4
5. Murphy, F. A., Fauquet, C. M., Bishop, D. H. L., Ghabrial, S. A., and Virology, M. D. S. J. A. O. (1995). Virus toxonomy classification and nomenclature of viruses sixth report of the international committee on taxonomy of viruses. Archives Suppl. 10:275–288.
6. Jindal, N, Chander, Y, Chockalingam, AK, De Abin, M, Redig, PT, and Goyal, SM. Phylogenetic analysis of Newcastle disease viruses isolated from waterfowl in the upper Midwest region of the United States. Virol J. (2009) 6:191. doi: 10.1186/1743-422X-6-191
7. Snoeck, CJ, Adeyanju, AT, Owoade, AA, Couacy-Hymann, E, Alkali, BR, Ottosson, U, et al. Genetic diversity of Newcastle disease virus in wild birds and pigeons in West Africa. Appl Environ Microbiol. (2013) 79:7867–74. doi: 10.1128/AEM.02716-13
8. Millar, NS, and Emmerson, PT. Molecular cloning and nucleotide sequencing of Newcastle disease virus. Newcastle disease. New York: Springer. (1988). 79–97.
9. Steward, M, Vipond, IB, Millar, NS, and Emmerson, PT. Rna editing in Newcastle disease virus. J Gen Virol. (1993) 74:2539–47. doi: 10.1099/0022-1317-74-12-2539
10. Abd Elfatah, KS, Elabasy, MA, El-Khyate, F, Elmahallawy, EK, Mosad, SM, El-Gohary, FA, et al. Molecular characterization of Velogenic Newcastle disease virus (sub-genotype vii.1.1) from wild birds, with assessment of its pathogenicity in susceptible chickens. Animals. (2021) 11:505. doi: 10.3390/ani11020505
11. Chen, L, Song, J, Liu, H, Cai, J, Lin, Q, Xu, C, et al. Phylodynamic analyses of class I Newcastle disease virus isolated in China. Transbound Emerg Dis. (2021) 68:1294–304. doi: 10.1111/tbed.13785
12. Dimitrov, KM, Abolnik, C, Afonso, CL, Albina, E, Bahl, J, Berg, M, et al. Updated unified phylogenetic classification system and revised nomenclature for Newcastle disease virus. Infect Genet Evol. (2019) 74:103917. doi: 10.1016/j.meegid.2019.103917
13. Lomniczi, B, Wehmann, E, Herczeg, J, Ballagi-Pordány, A, Kaleta, EF, Werner, O, et al. Newcastle disease outbreaks in recent years in western Europe were caused by an old (vi) and a novel genotype (vii). Arch Virol. (1998) 143:49–64. doi: 10.1007/s007050050267
14. Maminiaina, OF, Gil, P, Briand, FX, Albina, E, Keita, D, Andriamanivo, HR, et al. Newcastle disease virus in Madagascar: identification of an original genotype possibly deriving from a died out ancestor of genotype iv. PLoS One. (2010) 5:e13987. doi: 10.1371/journal.pone.0013987
15. Xiang, B, Chen, L, Cai, J, Liang, J, Lin, Q, Xu, C, et al. Insights into genomic epidemiology, evolution, and transmission dynamics of genotype vii of class ii Newcastle disease virus in China. Pathogens. (2020) 9:837. doi: 10.3390/pathogens9100837
16. Yu, X, Luo, Y, Wang, J, Shu, B, Jiang, W, Liu, S, et al. A molecular, epidemiological and pathogenicity analysis of pigeon paramyxovirus type 1 viruses isolated from live bird markets in China in 2014-2021. Virus Res. (2022) 318:198846. doi: 10.1016/j.virusres.2022.198846
17. Carella, E, Orusa, T, Viani, A, Meloni, D, Borgogno-Mondino, E, and Orusa, R. An integrated, tentative remote-sensing approach based on NDVI entropy to model canine distemper virus in wildlife and to prompt science-based management policies. Animals. (2022) 12:1049. doi: 10.3390/ani12081049
18. Orusa, T, Orusa, R, Viani, A, Carella, E, and Borgogno Mondino, E. Geomatics and Eo data to support wildlife diseases assessment at landscape level: a pilot experience to map infectious Keratoconjunctivitis in chamois and Phenological trends in Aosta Valley (Nw Italy). Remote Sens. (2020) 12:3542. doi: 10.3390/rs12213542
19. Shi, Z, Wei, L, Wang, P, Wang, S, Liu, Z, Jiang, Y, et al. Spatio-temporal spread and evolution of influenza a (H7N9) viruses. Front Microbiol. (2022) 13:1002522. doi: 10.3389/fmicb.2022.1002522
20. Kumar, S, Stecher, G, and Tamura, K. Mega7: molecular evolutionary genetics analysis version 7.0 for bigger datasets. Mol Biol Evol. (2016) 33:1870–4. doi: 10.1093/molbev/msw054
21. Joshi, VG, Chaudhary, D, Bansal, N, Singh, R, Maan, S, Mahajan, NK, et al. Prevalence of Newcastle disease virus in commercial and backyard poultry in Haryana, India. Front Vet Sci. (2021) 8:725232. doi: 10.3389/fvets.2021.725232
22. Pham, HM, and Do, TT. Detection and assessment of risk factors associated with Newcastle disease virus infection in birds in backyard poultry in Laichau province of Vietnam. Avian Pathol. (2023) 52:144–52. doi: 10.1080/03079457.2022.2160697
23. Sahoo, N, Bhuyan, K, Panda, B, Behura, NC, Biswal, S, Samal, L, et al. Prevalence of Newcastle disease and associated risk factors in domestic chickens in the Indian state of Odisha. PLoS One. (2022) 17:e0264028. doi: 10.1371/journal.pone.0264028
24. Goodswen, SJ, Barratt, JLN, Kennedy, PJ, Kaufer, A, Calarco, L, and Ellis, JT. Machine learning and applications in microbiology. FEMS Microbiol Rev. (2021) 45:fuab015. doi: 10.1093/femsre/fuab015
25. Gussow, AB, Auslander, N, Wolf, YI, and Koonin, EV. Prediction of the incubation period for Covid-19 and future virus disease outbreaks. BMC Biol. (2020) 18:186. doi: 10.1186/s12915-020-00919-9
26. Orusa, T, Viani, A, Cammareri, D, and Borgogno Mondino, E. A Google earth engine algorithm to map Phenological metrics in mountain areas worldwide with Landsat collection and Sentinel-2. Geomatics. (2023) 3:221–38. doi: 10.3390/geomatics3010012
27. Chang, Z, Dong, X, Guan, Z, Lu, K, Chen, X, Wei, X, et al. Antigenic variation in hemagglutinin-neuraminidase of Newcastle disease virus isolated from Tibet, China. Vet Microbiol. (2023) 285:109872. doi: 10.1016/j.vetmic.2023.109872
28. Naguib, MM, Höper, D, Elkady, MF, Afifi, MA, Erfan, A, Abozeid, HH, et al. Comparison of genomic and antigenic properties of Newcastle disease virus genotypes ii, xxi and vii from Egypt do not point to antigenic drift as selection marker. Transbound Emerg Dis. (2022) 69:849–63. doi: 10.1111/tbed.14121
29. Zhang, S, Wang, X, Zhao, C, Liu, D, Hu, Y, Zhao, J, et al. Phylogenetic and pathotypical analysis of two virulent Newcastle disease viruses isolated from domestic ducks in China. PLoS One. (2011) 6:e25000. doi: 10.1371/journal.pone.0025000
30. Duan, X, Zhang, P, Ma, J, Chen, S, Hao, H, Liu, H, et al. Characterization of genotype ix Newcastle disease virus strains isolated from wild birds in the northern Qinling Mountains, China. Virus Genes. (2014) 48:48–55. doi: 10.1007/s11262-013-0987-y
Keywords: Newcastle disease virus, prevalence, risk factor, phylogenetic analysis, random forest, China
Citation: Wang S, Wei L, Wang J and Zhang Z (2024) Epidemiological study of Newcastle disease in chicken farms in China, 2019–2022. Front. Vet. Sci. 11:1410878. doi: 10.3389/fvets.2024.1410878
Received: 02 April 2024; Accepted: 30 September 2024;
Published: 30 October 2024.
Edited by:
Dirk Werling, Royal Veterinary College (RVC), United KingdomCopyright © 2024 Wang, Wei, Wang and Zhang. This is an open-access article distributed under the terms of the Creative Commons Attribution License (CC BY). The use, distribution or reproduction in other forums is permitted, provided the original author(s) and the copyright owner(s) are credited and that the original publication in this journal is cited, in accordance with accepted academic practice. No use, distribution or reproduction is permitted which does not comply with these terms.
*Correspondence: Jingfei Wang, d2FuZ2ppbmdmZWlAY2Fhcy5jbg==; Zhigang Zhang, emhhbmd6aGlnYW5nQG5lYXUuZWR1LmNu
Disclaimer: All claims expressed in this article are solely those of the authors and do not necessarily represent those of their affiliated organizations, or those of the publisher, the editors and the reviewers. Any product that may be evaluated in this article or claim that may be made by its manufacturer is not guaranteed or endorsed by the publisher.
Research integrity at Frontiers
Learn more about the work of our research integrity team to safeguard the quality of each article we publish.