- 1Shaanxi Key Laboratory of Molecular Biology for Agriculture, College of Animal Science and Technology, Northwest A&F University, Xianyang, China
- 2Institute of Animal Husbandry Quality Standards, Xinjiang Academy of Animal Sciences, Urumqi, China
Understanding the differences in genetic variation between local Chinese dairy goat breeds and imported breeds can help germplasm innovation and molecular breeding. However, the research is limited in this area. In this study, whole-genome resequencing data from 134 individuals of both local and imported dairy goat breeds were analyzed, and their differences in genomic genetic variation, genetic diversity, and population structure were subsequently identified. We also screened candidate genes associated with important traits of dairy goats such as milk production (STK3, GHR, PRELID3B), reproduction (ATP5E), growth and development (CTSZ, GHR), and immune function (CTSZ, NELFCD). Furthermore, we examined allele frequency distributions for the genes of interest and found significant differences between the two populations. This study provides valuable resources for the study of genetic diversity in dairy goats and lays the foundation for the selective breeding of dairy goats in the future.
Introduction
Goats (Capra hircus) were among the first animal species domesticated, providing humans with essential food and living resources including meat, milk, and fur (1–4). As a specialized milk-producing breed, dairy goats are an important part of many countries’ livestock and dairy industries (5–7). China plays a prominent role in global dairy goat breeding and goat milk production, contributing significantly to the global milk supply (8).
The history of breeding dairy goats in China can be traced back to the early 20th century, with a primary focus on improving the Saanen dairy goat and its hybrid offspring. After nearly a century of breeding, the current dairy goat breeds in China mainly consist of Xinong Saanen, Guanzhong, and Laoshan dairy goats (9, 10). As people’s living standards have improved in China in past decades, the demand for dairy products with high quality has increased. To meet such requirements, the breeding of dairy goats has primarily focused on traits associated with high yield and quality (11, 12). Currently, to improve the excellent characteristics of dairy goat breeds in China, most farms focus on improving dairy goat breeds through selective breeding and crossing with imported breeds. The imported breeds in China mainly come from New Zealand and Australia, which comprise Saanen, Alpine, and Toggenburg dairy goats (13, 14). Among them, Saanen is the most commonly imported breed for milk production enhancement, while other breeds are primarily used to improve the milk quality of dairy goats (15, 16). However, the traits remain unclear, which hinders the verification of the breeding process. Meanwhile, dairy goat import primarily relies on phenotype evaluation without genomic level selection, which is not accurate nor very effective.
With the completion of the goat reference genome, significant progress has been made in the study of genetic diversity in dairy goats. These studies not only reveal genetic variations in the goat genome, but also provide important clues for understanding genetic differences and adaptation mechanisms among different breeds (17). However, previous studies have revealed the association between genetic variation and specific traits in the goat genome, research on the genetic variation and trait association of this specific population of dairy goats is still relatively limited (18–20). The existing research mainly focuses on a few major breeds, and the understanding of genetic differences between a wider range of dairy goat breeds is not yet in-depth. Therefore, in-depth exploration of the genetic diversity, population structure, and genetic variations associated with important agronomic traits of dairy goat populations is of great significance for the sustainable development of the dairy goat industry. In this study, we resequenced 50 local dairy goat genomes at 17.01× coverage depth. Then it was combined with the data from another 84 individuals of local and imported dairy goat species for further analysis to identify their differences in genomic genetic variation, genetic diversity, and population structure. Analysis of runs of homozygosity (ROH) islands and selective scanning identified specific regions and genes impacted by selection, associated with various significant morphological and agronomic traits. Additionally, we examined allele frequency distributions for the genes within selective signatures. Our findings contribute to the understanding of the genetic diversity and population genetic structure of dairy goats. Moreover, it revealed candidate genes that are associated with various phenotypes, which is valuable for future importation and breeding selection of dairy goats.
Materials and methods
Sample collection and sequencing
This study collected two populations of Xinong Saanen Dairy Goat (XNG, n = 40) and Guanzhong Dairy Goat (GZG, n = 10) from Shaanxi Province. Each goat had 5 mL of blood collected from the jugular vein, and DNA was extracted using the standard phenol-chloroform method. Samples with determined DNA concentrations were subjected to whole-genome sequencing by Huada Company, with paired-end libraries constructed using the Huada T7 platform having an average insert size of 500 bp per individual and an average read length of 150 bp. In addition, the resequencing data of 84 individuals were downloaded from public databases, including 8 breeds as follows: Australian Alpine Dairy Goat (AAG, n = 2), Australian Saanen Dairy Goat (ASG, n = 6), Guanzhong Dairy Goat (GZG, n = 26), New Zealand Saanen Dairy Goat (NSG, n = 6), New Zealand Alpine Dairy Goat (NAG, n = 4), Laoshan Dairy Goat (LSG, n = 9), Nubian Dairy Goat (NBY, n = 15), Tugenburg Dairy Goat (TGB, n = 16). Resulting in a total of 134 samples in the study.
Read mapping and variant calling
The study utilized Trimmomatic v0.38 (21) for filtering the paired-end sequences. Next, BWA-MEM (v0.7.15-r1140) (22) aligned the clean data with the goat reference genome ARS1.2 (GCF_001704415.2, https://www.ncbi.nlm.nih.gov/datasets/genome/GCF_001704415.2/). Subsequently, samtools (23) was utilized to construct a BAM file index for mapping. The BAM files were then sorted and potential duplicate reads were removed utilizing the Picard1 tool. After mapping, SNP calling was performed using the “Haplotype Caller,” “Genotype GVCFs,” and “Select Variants” modules in the GATK genomic analysis tool package (GATK, version 3.8-1-0-gf15c1c3ef) (24). The initial SNPs were filtered with the “Variant Filtration” module based on the parameters: “QD < 2.0, FS > 60.0, MQ < 40.0, MQRankSum < −12.5, ReadPosRankSum < −8.0, and SOR > 3.0,” and an average sequencing depth of variants within the range “<1/3× and >3×” for all individuals. Lastly, the ANNOVAR (25) software was utilized for functional annotation of the SNPs.
Genetic diversity analysis
After applying linkage disequilibrium (LD) to prune SNPs, the PLINK (26) program is utilized to compute runs of homozygosity (ROH) with specific parameters as follows: (a) 100 consecutive homozygous SNPs, (b) minimum of 50 SNPs per window, (c) 500 kb of contiguous homozygous length, (d) minimum density of 1 SNP per 50 kb, (e) window overlap rate of 0.05, and (f) each window containing 1 heterozygous and 2 missing calls. The subsequent analysis results are categorized into 0.5–1 Mb, 1–2 Mb, and 2–4 Mb for visualization. The heterozygosity of the SNPs is calculated to estimate the inbreeding coefficient (Fhom). VCFtools (27) are used to analyze nucleotide diversity for each breed in non-overlapping 50 kb windows. Next, the PopLDdecay (28) software is used to calculate the decay of LD to assess the physical distance between SNPs within haplotype blocks of different breeds. Finally, the frequencies of runs of homozygosity (ROH) islands for Xinong Saanen dairy goats and Guanzhong dairy goats are calculated, and regions with frequencies above 20% are determined as ROH hotspot islands.
Population genomic analysis
Three methods are used for Variant Call Format (VCF) filtering and population structure estimation: (a) constructing a neighbor-joining tree with MEGA v10.2.6 (29) and visualizing it using iTOL (30); (b) principal component analysis (PCA) with EIGENSOFT v5.0 (31) software; and (c) conducting population structure analysis using ADMIXTURE v1.3.0 (32). Cross-validation is employed to calculate the cross-validation error and determine the optimal K value (assuming the ancestral population K value ranges from 2 to 8).
Detection of selective sweeps
Based on the characteristics and origins of the dairy goat breeds, we categorized 134 dairy goats into native dairy goat (NDG) and imported dairy goat (IDG) breeds. We then compared the genomes of these two dairy goat breeds and estimated the signal scanning regions using a combination of nucleotide diversity (θπ NDG/IDG) and fixation index (FST) with a VCFtools, employing 50 kb sliding windows and 25 kb sliding steps. Additionally, Tajima’s D (33) statistic and cross-population composite likelihood ratio test (XP-CLR) (34) were employed to identify potential regional differences between different breeds. XP-CLR is a likelihood method for detecting selective sweeps by jointly modeling multi-locus allele frequency differences between two groups. The scanned regions detected from the intersection of the two parameters with the highest 5% threshold were annotated to identify candidate genes. Lastly, Bedtools (35) was used to annotate the selected regions for subsequent analysis.
Enrichment analyses and visualization
The enrichment module in KOBAS (36) was utilized to identify pathways and Gene Ontology (GO) terms that showed statistically significant associations (at a significance level of p ≤ 0.05). Subsequently, the results were visualized through the OmicShare tool.2
Results
Sequencing and variation calling
To identify the genomic difference between local (XNG, GZG, LSG) and imported breeds of dairy goats (AAG, ASG, NSG, NAG, TGB, NBY), we compared 134 sets of whole-genome resequencing data, including 40 genomes of XNG and 10 genomes of GZG, combined with 84 published genome data that includes both local and imported breeds (GZG, LSG, AAG, ASG, NSG, NAG, TGB, NBY) (Figure 1). The data was aligned to the goat reference genome ARS1.2 (GCF_001704415), achieving an average alignment rate of 97.74% and a mean sequencing depth of 11.3×. After the removal of low-quality sequences, the average clean reads for each sample were 373,568,004 bp, with GC content ranging from 41.82 to 42.33%. The average alignment rate for the studied populations (XNG, GZG) is 97.5%, accompanied by an average sequencing depth of 17.01×. The imported breed populations (AAG, ASG, NSG, NAG, TGB) exhibited an average alignment rate of 97.04% and an average sequencing depth of 22.51× (Supplementary Table S1).
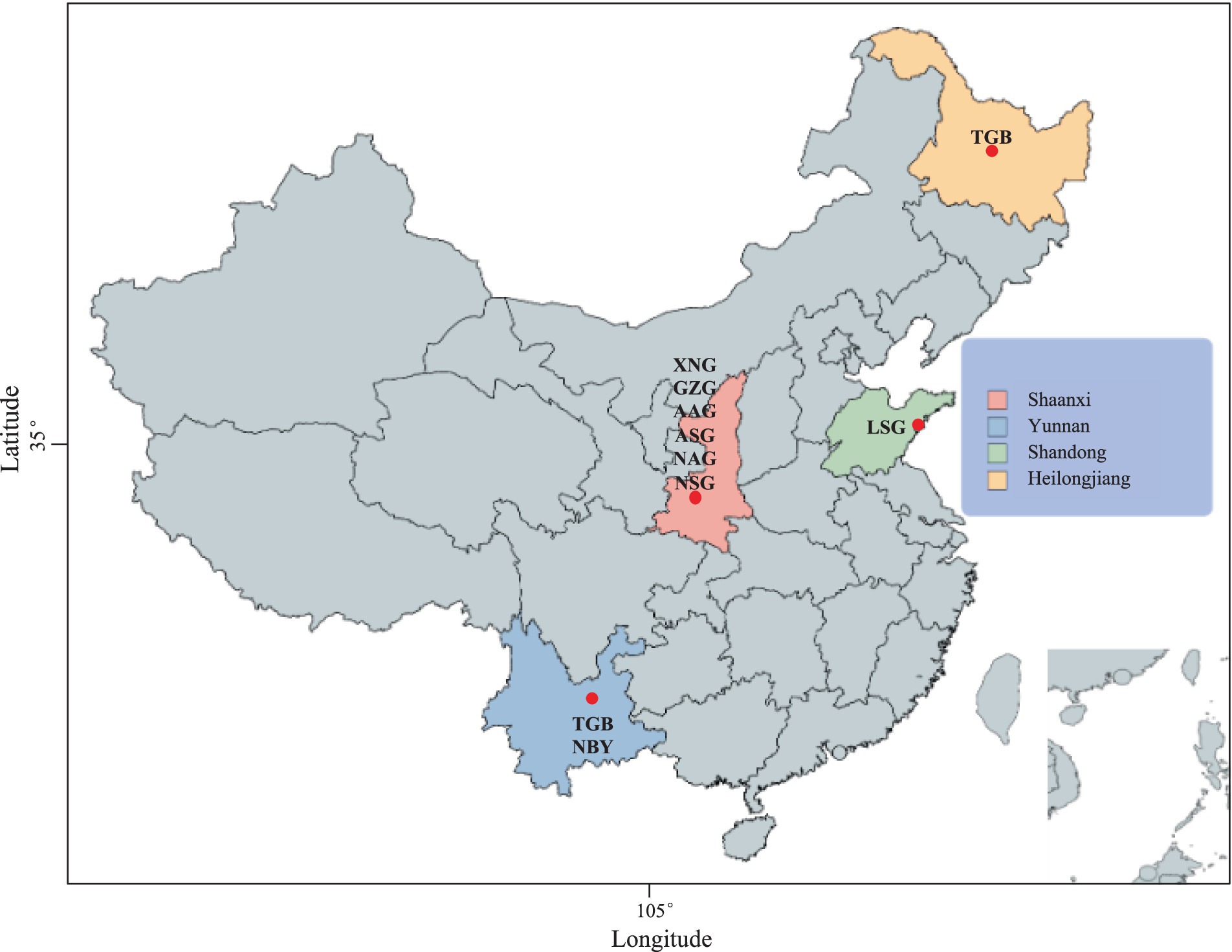
Figure 1. The geographic distribution of native dairy goat (NDG) and imported dairy goat (IDG) breeds. Xinong Saanen dairy goat (XNG, n = 40) and Guanzhong dairy goat (GZG, n = 10), Australian Alpine dairy goat (AAG, n = 2), Australian Saanen dairy goat (ASG, n = 6), Guanzhong dairy goat (GZG, n = 26), New Zealand Saanen dairy goat (NSG, n = 6), New Zealand Alpine dairy goat (NAG, n = 4), Laoshan dairy goat (LSG, n = 9), Nubian dairy goat (NBY, n = 15), Tugenburg dairy goat (TGB, n = 16).
After SNP variation detection and statistical analysis of the obtained 134 dairy goat genome data sets, a total of 33,011,806 SNPs were detected. Among them, the proportion of intronic SNPs was the highest (32,705,301), accounting for 55.04% of the total; the proportion of exonic SNPs was approximately 1.142%. There were 283,685 synonymous SNPs and 227,235 missense SNPs. The transition/transversion ratio (Ti/Tv) was computed and analyzed, revealing a ratio of 2.344, consistent with the general value for goat populations. These findings indicate a high quality of sequencing, thus the obtained data meet the requirements for subsequent analysis (Supplementary Table S2).
Genetic diversity analysis
The LD analysis shows that GZG and XNG exhibit the fastest decay rate (Figure 2A), suggesting lower domestication levels and higher genetic diversity. Nucleotide diversity analysis (Figure 2B) reveals higher diversity in local breeds compared to imported ones. ROH is an indicator of kinship proximity and inbreeding history, and the analysis reveals the genomic patterns of recent demographic history. Short ROH indicates that ancient inbreeding is much more prevalent in the XNG and GZG compared to other imported breeds. In addition, XNG exhibits a higher value of ROH, indicating a longer breeding history (Figure 2C). Meanwhile, the coefficient of inbreeding due to recent inbreeding is highest for each individual genome in TGB, with a range from 0.1 to 0.51 (Figure 2D).
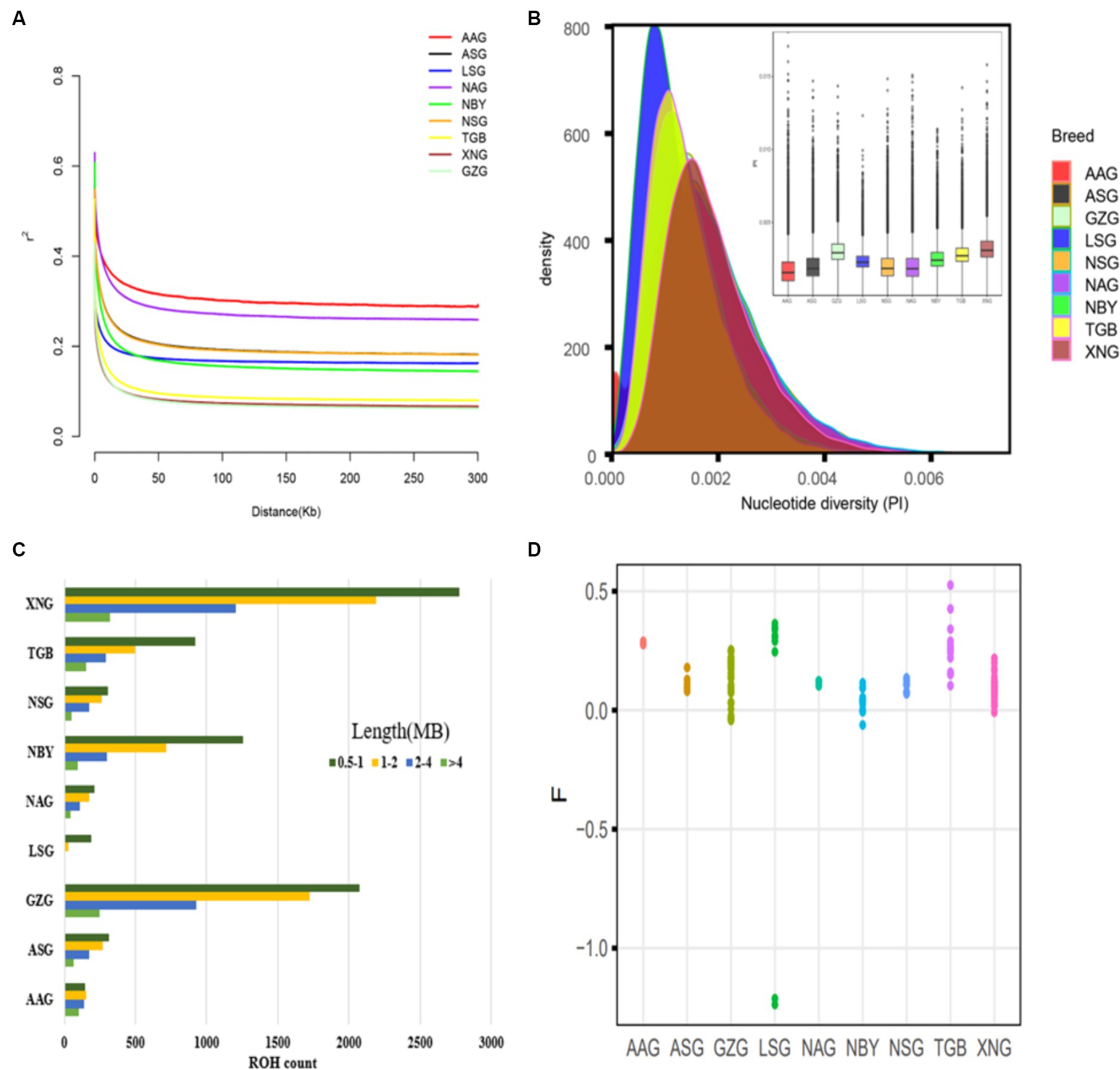
Figure 2. Genetic diversity among 134 samples from 10 populations. (A) Genome-wide average LD decay estimated from each group. (B) Density plots and Box plots of the nucleotide diversity for each group. (C) Estimation of the total number of ROH for each group. (D) Inbreeding coefficient for each individual.
This study conducted ROH island analysis on XNG and GZG. In the XNG samples, 16 ROH islands with a frequency of 20% were identified on chromosomes 12, 13, and 19 (Figure 3A), annotating to 31 genes. Similarly, on chromosomes 1, 7, 12, 13, and 18 of the GZG, 15 ROH islands with a frequency of 20% were identified (Figure 3B), annotating 25 genes. The Venn diagram (Supplementary Figure S1) illustrates 4 common genes between the two breeds (MPHOSPH8, LOC108637296, PSPC1, NBEA). In addition, the GO and KEGG enrichment analyses (Supplementary Figure S2) indicate enrichment of those identified genes in 23 biological processes (BP), 9 molecular functions (MF), and 2 cellular components (CC). The enriched pathways primarily include biological regulation, metabolic processes, immune system processes, and transcriptional regulation. Furthermore, the KEGG analysis reveals enrichment in valine, leucine, and isoleucine degradation, apoptosis, PI3K-Akt signaling pathway, and Rap1 signaling pathway.
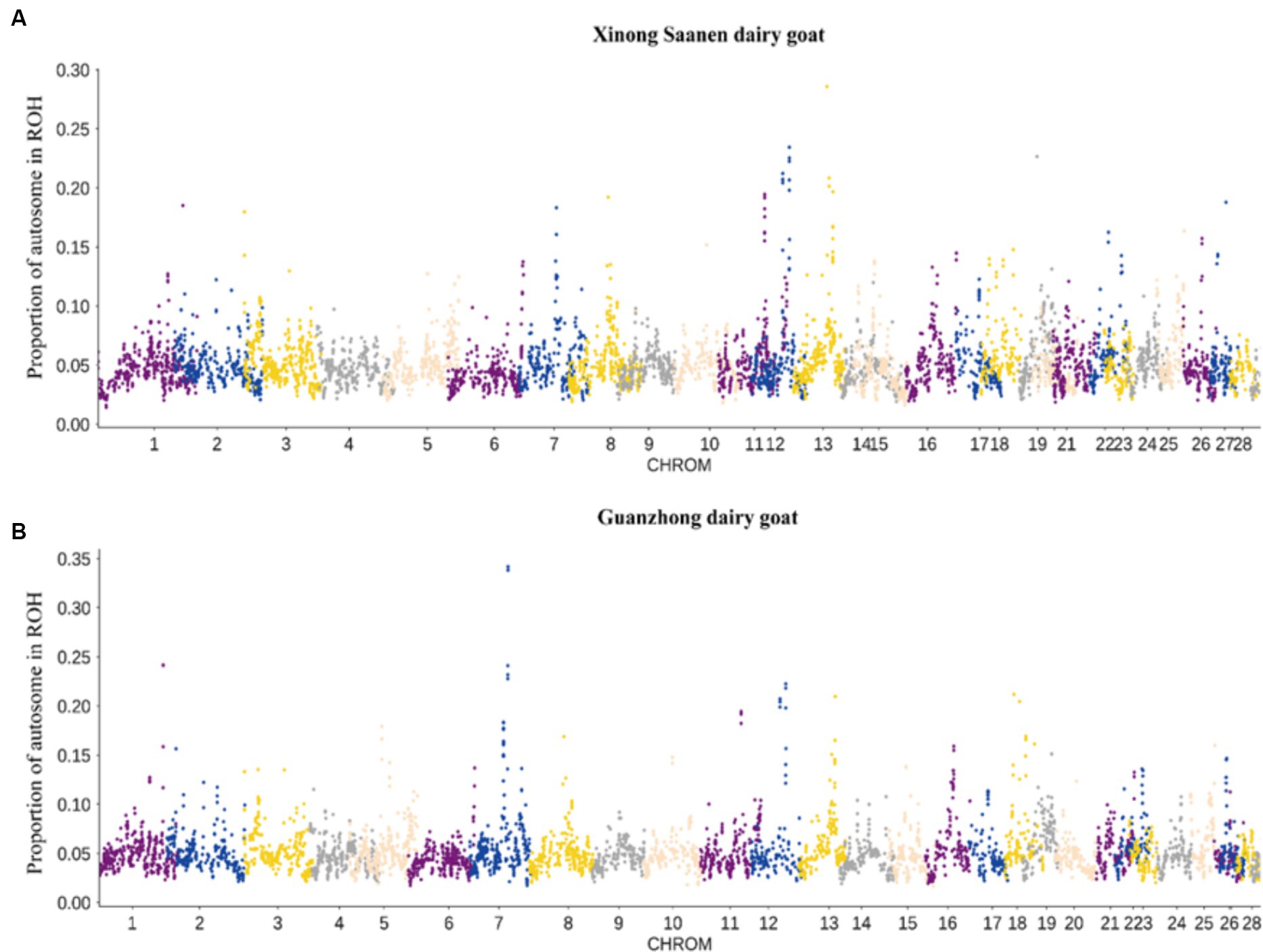
Figure 3. Manhattan plots of ROH frequencies. (A) Manhattan plots of ROH frequencies in Xinong Saanen dairy goat (XNG). (B) Manhattan plots of ROH frequencies in Guanzhong dairy goat (GZG).
Phylogeny and population genetic structure
To comprehend the population structure of local dairy goats in China, we looked into the SNP results derived from the resequencing data. Employing imported breeds as outgroups, we constructed SNP differentiation clustering based on various populations, the results of which can be used to mutually verify with other clustering methods. The percentages of PC1, PC2, and PC3 are 7.91, 5.61, and 3.60%, respectively, (Figure 4A; Supplementary Figure S3). There are three major clusters that AAG, ASG, LSG, NAG, NSG, and TGB are closely clustered, XNG and GZG form another cluster, while NBY is away from both of them. The result indicates that local breeds can be differentiated from imported breeds, and there are differences in clustering patterns among the 9 populations. The genetic data of the populations were utilized to calculate the degree of kinship between individuals, construct a genetic distance matrix, and build a phylogenetic tree utilizing the distance matrix. Although GZG and XNG are partially overlapped in the phylogenetic tree, the XNG group is clustered on its own, and imported breeds can be differentiated from local breeds, which is consistent with the PCA results (Figure 4B). In addition, for the 9 population, the number of subgroups (value of K) was preset to 2–9 for clustering, and the clustering results were cross-validated. The optimal number of subgroups was identified based on the minimum cross-validation error rate. K = 4 was identified as the optimal number of subgroups, and there were significant genetic differences among the XNG, NBY, NAG, and TGB populations. Regardless of the value of K, it consistently displayed genetic differentiation between local and imported breeds, indicating different genetic compositions among the populations, and relatively consistent genetic backgrounds among the different 9 populations (Figure 4C).
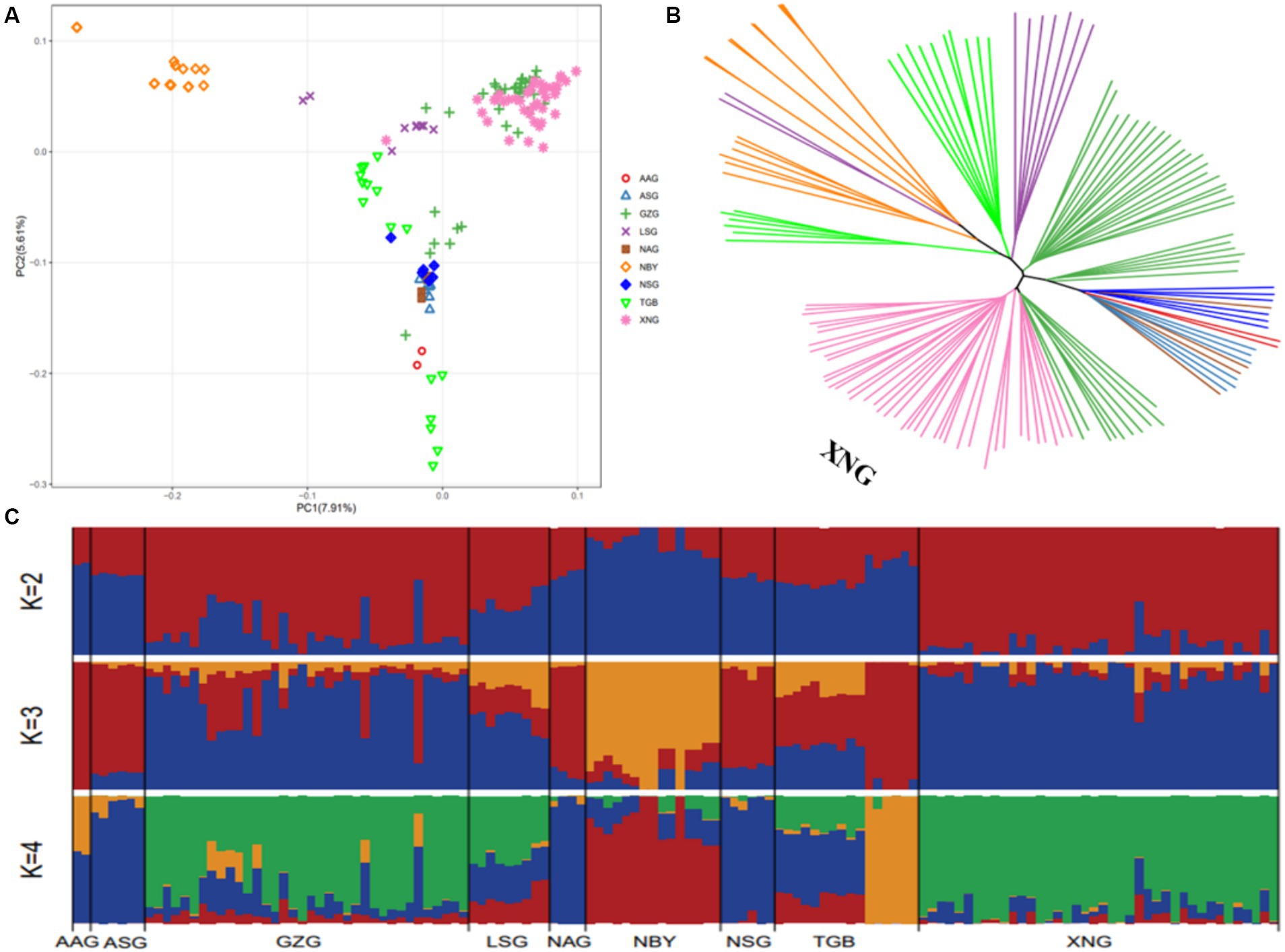
Figure 4. The structures of 134 samples from 10 populations. (A) PCA. Principal components 1 (7.91%) and 2 (5.61%) for the 134 dairy goats. (B) Phylogenetic tree. Phylogenetic relationships were estimated using the neighbor-joining method. (C) Genetic structure of cattle using ADMIXTURE when K ranged from 2 to 4.
Genome-wide selective sweep test
To identify the regions of selection among the native dairy goat breeds and imported dairy goat breeds, we further conducted a comparative analysis using the resequencing data. Next, using the top 5% of both FST (Figure 5A) and θπ ratio (Figure 5B) cutoffs (Figure 5C), we observed that the native dairy goat breeds exhibited a total of 41.25 Mb across 607 selectively scanned regions. These regions encompassed 549 genes (Figure 5D) and showed significant enrichment in pathways such as the calcium signaling pathway, MAPK signaling pathway, fatty acid metabolism, and the PI3K-Akt signaling pathway (Figure 5E). On the other hand, imported dairy goat breeds displayed 25.05 Mb in 385 selective scanning regions, which encompassed 387 genes (Figure 5D). Pathway enrichment analysis revealed that those genes are involved in oocyte meiosis, cell senescence, calcium signaling pathway, GnRH signaling pathway, and the synthesis and secretion of growth hormone pathway (Figure 5F). These findings emphasize the genetic functional differences between local and imported breeds (Supplementary Figure S4).
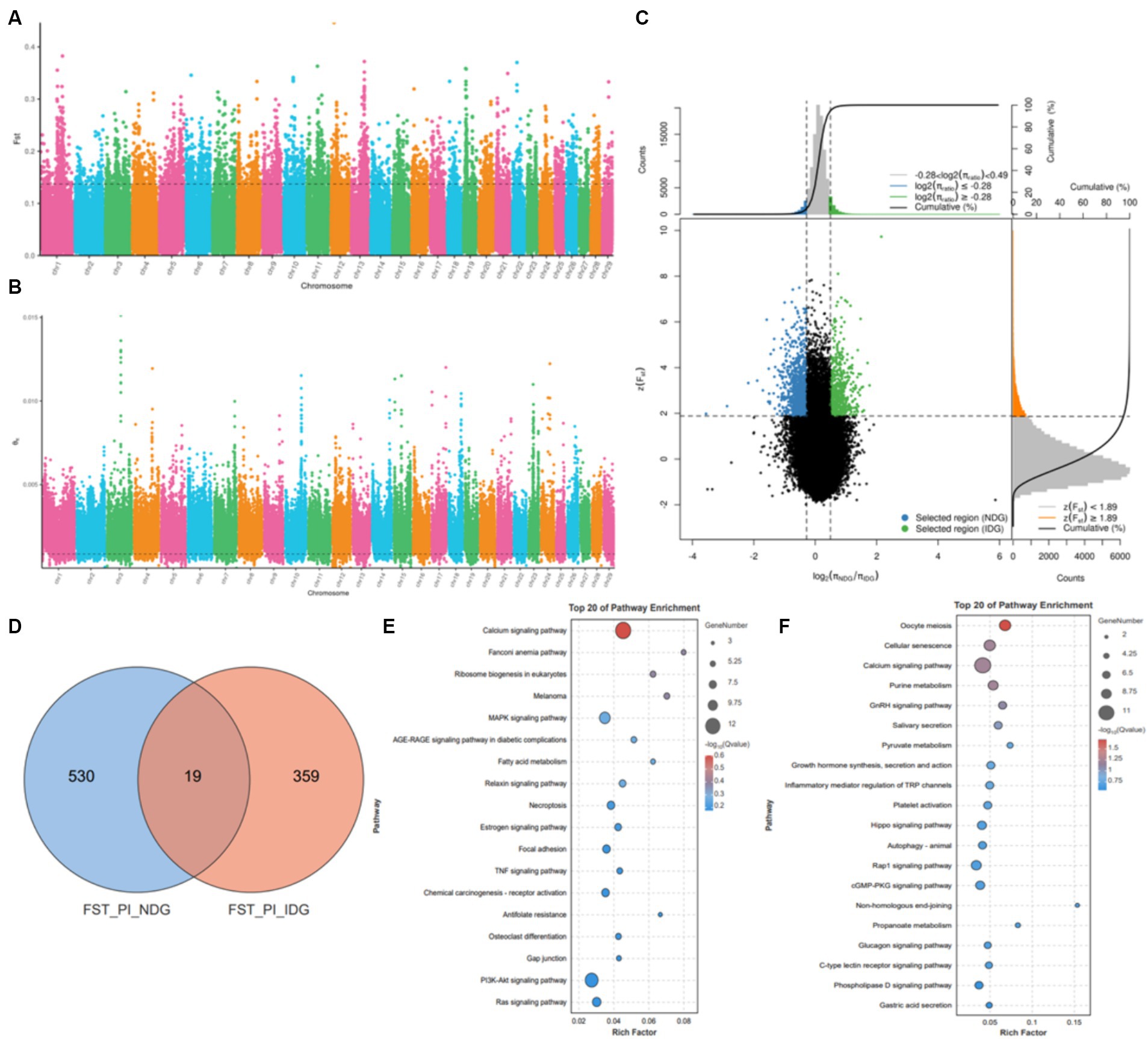
Figure 5. Selective sweep analysis of NDG and IDG. (A) Manhattan plot showing the FST. (B) Manhattan plot showing the θπ. (C) Distribution of log2 (θπ ratios) and FST values calculated in 50 kb sliding windows between NDG and IDG. (D) Venn diagram showing the gene overlaps among FST_PI_NDG and FST_PI_IDG. (E) KEGG pathway enrichment analysis based on genes across significant selective regions from NDG. (F) KEGG pathway enrichment analysis based on genes across significant selective regions from IDG.
Selective signatures for traits
To further explore the traits corresponding to selection signals, we performed FST, PI, and XP-CLR assays to discover positive selection in dairy goats. Meanwhile, for a more comprehensive annotation of genes We integrated and annotated the QTLdb database and a previously published genome-wide association analysis on important traits of dairy goats into our selection signaling results (Figure 6A). We found a large number of genes associated with milk production traits on chromosomes 5, 13, and 19 (Figure 6A), which were mainly involved in the monoacylglycerol biosynthetic process (GO:0006640), the long-chain fatty-acyl-CoA metabolic process (GO:00353336), monoacylglycerol metabolic process (GO:0046462), and fatty acid homeostasis (GO:0055089) (Figure 6B). In addition, these genes were also significantly enriched in the PI3K-Akt signaling pathway, glycerolipid metabolism, Fat digestion and absorption, and Regulation of lipolysis in adipocyte signaling pathways (Supplementary Figure S5). Integrative analysis (Fst, PI, XP-CLR) revealed two overlapping genes (Supplementary Figure S6), ASIP and STK3, respectively. Notably, integrative analysis (Fst, PI, XP-CLR, ROH_island) of the genes obtained from the above analytical methods revealed seven overlapping genes, including PRELID3B, ATP5E, CTSZ, NELFCD, LOC108637417, TRNAF-GAA, and TRNAN-GUU (Figure 6C). We then analyzed the allele frequency distribution of selected regions for STK3, GHR, and PRELID3B since many methods have detected these three genes, which showed that the gene frequencies of the selected genes differed between the local and imported breeds (Figure 6D; Supplementary Figure S7). Through the selection feature, we found that some regions were strongly selected. Some candidate genes were associated with milk production traits, growth and development, and immune traits.
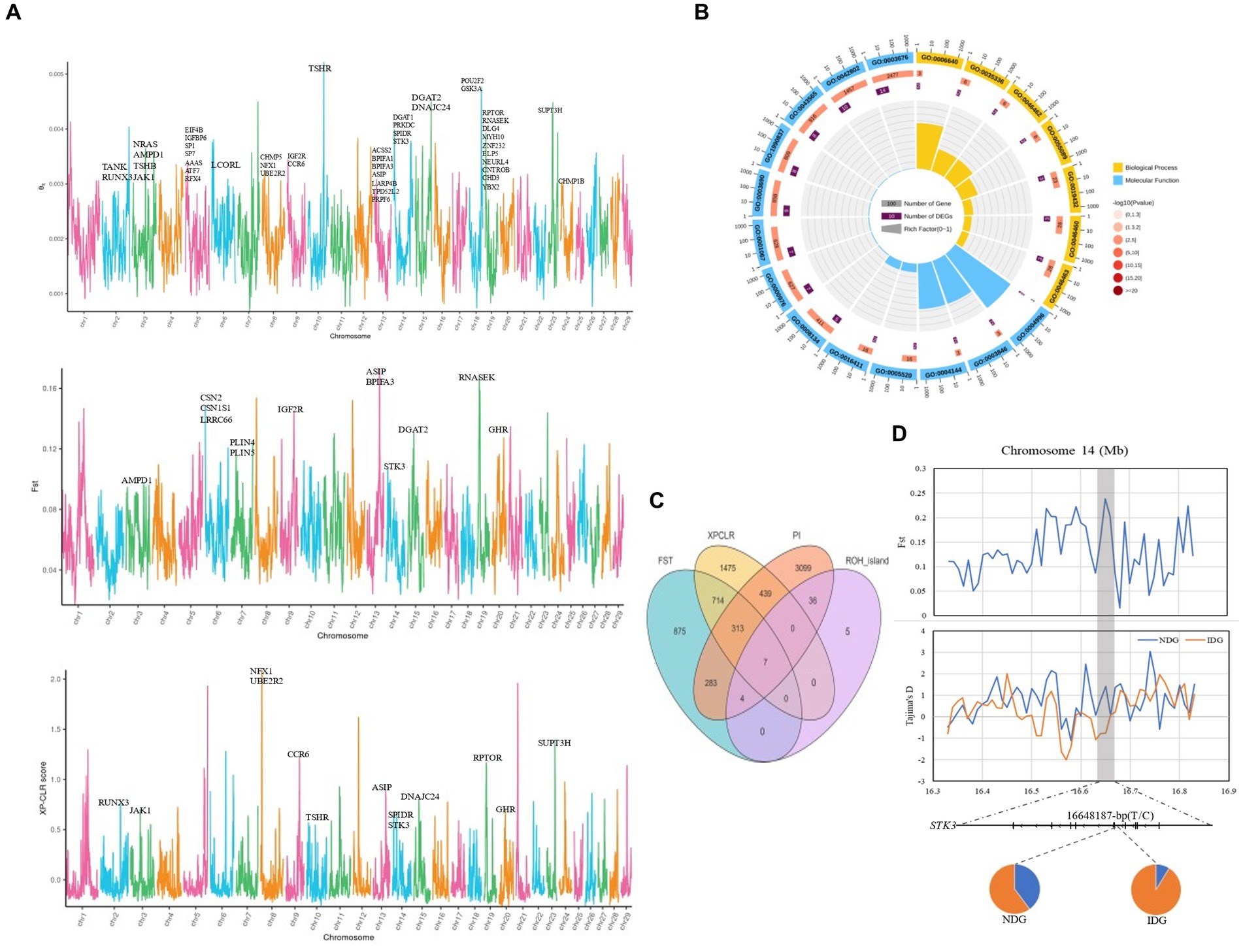
Figure 6. Genome-wide annotations during NDG and IDG. (A) Manhattan plot showing the θπ, FST and XP-CLR. (B) GO pathway enrichment analysis based on genes across significant selective regions. (C) Venn diagram showing the gene overlaps among FST, XP-CLR, PI and ROH_island. (D) FST value and Tajima’s D values around the STK3 locus. Allele frequencies of SNPs within the STK3 gene across the NDG and IDG, respectively.
Discussion
Detecting recent positive selection signatures in domesticated animals that have gone through both artificial and natural selection can provide information on genomic sites that can contribute to the identification of beneficial mutations and underlying biological pathways for economically important traits. In this study, we analyzed the whole genome of 134 dairy goats from both native and imported breeds. In addition, the samples were obtained from populations that were also widely distributed in China, a feature that is absent in previous studies, which provides a more reliable background for the study. The detection of genetic variation, genetic diversity, and population structure showed that local dairy goat breeds are more genetically diverse than imported breeds. Previous studies in cattle (37, 38) and sheep (17) have shown similar results. This phenomenon suggests the local breeds have undergone less intensive selection, which may explain why their milk production performance is not outstanding. Therefore, crossing with imported breeds plus selection could be a promising way to improve the local breeds. However, detailed genetic information is needed to guide such a breeding process.
Milk quality traits are a complex biological issue that involves the interaction of multiple genes and biological pathways. Fatty acids, as one of the main components of milk fat, have a significant impact on the quality and characteristics of milk (39). By analyzing the selection signals of local and imported breeds for positive selection. we identified four genes that are involved in fatty acid metabolism (HSD17B12, ACOX3, CPT1B, and CBR4) in the selection signals of local dairy goats. The HSD17B12 gene is involved in the synthesis of unsaturated fatty acids (40) and is also associated with reproductive performance (41). The ACOX3 gene is associated with milk fat traits (42) and is a novel candidate for milk production traits (43). The CPT1B gene regulates long-chain saturated fatty acids and is involved in lipolysis (44, 45). The synthesis of fatty acids may involve CBR4 (46). Notably, different pathway enrichments were observed in the imported breeds. ACADS, AGPAT4, PTDSS2, and SMOX are mainly involved in lipid metabolism and amino acid metabolism pathways. ACADS is involved in the fatty acid metabolism pathway (47), AGPAT4 promotes triacylglycerol synthesis and fatty acid composition (48), PTDSS2 is involved in the glycerophospholipid metabolism pathway (49), and SMOX is a candidate gene for growth, carcass composition traits, and milk production traits (50, 51). In addition, previously reported traits associated with immunity (BPIFA3, LRRC66) (52, 53), coat color (ASIP) (54), milk composition (CSN1S1, DGAT2, PLIN4, PLIN5, IGF2R) (55), and milk production (AMPD1) (56) were also found in imported breeds. Together, the results, suggest that the traits under selection were mainly associated with milk production, reproduction, and immunity for both the local and the imported breeds of dairy goats despite the difference in genes.
Milk production traits are the most important economic traits of dairy goats. This study includes milk production traits-associated gene markers from the QTLdb database for selection signal analysis. A large number of selected genes on chromosomes 13, and 19 were identified, which is similar to the results of a previous Genome-wide association study in dairy goats (15, 57). Through Fst, PI, and XP-CLR analysis, two genes with the highest selection signals showed up including ASIP and STK3. ASIP is responsible for regulating pigmentation and is associated with fat deposition and fatty acid composition in sheep (58, 59). The gene was identified as strongly selected due to the coat color difference between Alpine dairy goats (black) and native dairy goats (white). Whereas the STK3 gene was identified as a new candidate gene for milk production traits (60), it also showed a strong selection signal in this study. We incorporated the results of the ROH_island analysis into the selective signaling analysis and identified seven genes that overlapped, including PRELID3B, ATP5E, CTSZ, NELFCD, LOC108637417, TRNAF-GAA, and TRNAN-GUU. PRELID3B has an important role in excessive melanin deposition (61) and regulates lipid accumulation in mitochondria (62). In lamb MII oocytes, down-regulation of ATP5E and up-regulation of CUL1, MARCH7, and TRIM17 might cause low competence of lamb embryos (63). Furthermore, it has been reported that ATP5E is a promising candidate biomarker for oocyte viability after IVM (64), so the gene might be associated with reproductive traits in female animals. Many studies have demonstrated that CTSZ is linked to various traits, including immunity (65), pregnancy (66), regulation of thermogenesis in brown adipocytes (67), growth, carcass, and production (68). NELFCD may serve as a novel candidate gene for early immunomodulation (69) and it is strongly linked to CTSZ (70). TRNAF-GAA and TRNAN-GUU are tRNA-related genes, which might associated with immune (71) and reproductive traits (72, 73). The function of the gene LOC108637417, however, remains unclear. It is worth noting that the GHR is not only strongly selected, and also associated with various economic traits [milk production (74), growth (75), and milk quality traits (76)]. In summary, the identified genes in this study could be new candidate genes of milk production, reproduction, and immune traits in dairy goats.
To further investigate the differences of the genes associated with the selected regions in different breeds, the key genes STK3, GHR, and PRELID3B were subjected to allele frequency analysis. it was found that the genotypic frequencies of the strongly selected genes differed significantly among different populations. Similar results were found in chicken (77) and sheep (17) genomic research. By comparing the genetic differences between different varieties, we can better understand the genetic mechanisms behind these differences, providing guidance for future variety improvement and genetic resource protection.
Conclusion
This study analyzed the genetic diversity, population structure, selection signals, and allele frequencies of local and imported dairy goats in China. It was found that there were significant genomic differences between the two populations. Moreover, candidate genes related to milk production (STK3, GHR, PRELID3B), reproduction (ATP5E), growth and development (CTSZ, GHR), and immune (CTSZ, NELFCD) traits were identified. It provides valuable insights into the genetic diversity of dairy goats and thus lays, the data support for future dairy goat breeding and selection in China.
Data availability statement
The data that support the findings of this study are openly available in NCBI Sequence Read Archive at https://www.ncbi.nlm.nih.gov/, Accession number: PRJNA1127047.
Ethics statement
The animal studies were approved by the Institutional Animal Care and Use Committee of Northwest A&F University. The studies were conducted in accordance with the local legislation and institutional requirements. Written informed consent was obtained from the owners for the participation of their animals in this study.
Author contributions
JZ: Visualization, Writing – review & editing, Writing – original draft, Formal analysis. YM: Writing – review & editing, Data curation. PG: Writing – review & editing, Resources. BL: Writing – review & editing, Supervision. FZ: Software, Writing – review & editing, Methodology. LZ: Writing – review & editing, Methodology. CS: Writing – review & editing, Methodology. XL: Writing – review & editing, Resources. JL: Project administration, Writing – original draft, Writing – review & editing, Supervision, Conceptualization.
Funding
The author(s) declare financial support was received for the research, authorship, and/or publication of this article. This work was supported by Shaanxi Livestock and Poultry Breeding Double-chain Fusion Key Project of China (2022GD-TSLD-46-0201), Hohhot City Science and Technology Plan Project (Major Science and Technology Special Project) in China (2023150103000025).
Acknowledgments
This work received support from the high-performance computing (HPC) resources at Northwest A&F University (NWAFU).
Conflict of interest
The authors declare that the research was conducted in the absence of any commercial or financial relationships that could be construed as a potential conflict of interest.
Publisher’s note
All claims expressed in this article are solely those of the authors and do not necessarily represent those of their affiliated organizations, or those of the publisher, the editors and the reviewers. Any product that may be evaluated in this article, or claim that may be made by its manufacturer, is not guaranteed or endorsed by the publisher.
Supplementary material
The Supplementary material for this article can be found online at: https://www.frontiersin.org/articles/10.3389/fvets.2024.1409282/full#supplementary-material
Footnotes
References
1. Zeder, MA. Domestication and early agriculture in the Mediterranean basin: origins, diffusion, and impact. Proc Natl Acad Sci USA. (2008) 105:11597–604. doi: 10.1073/pnas.0801317105
2. Zhang, B, Chang, L, Lan, X, Asif, N, Guan, F, Fu, D, et al. Genome-wide definition of selective sweeps reveals molecular evidence of trait-driven domestication among elite goat (Capra species) breeds for the production of dairy, cashmere, and meat. GigaScience. (2018) 7:giy105. doi: 10.1093/gigascience/giy105
3. Pereira, F, and Amorim, A. (2010). Origin and spread of goat pastoralism. eLS. Available at: https://www.researchgate.net/profile/Filipe-Pereira-17/publication/222094707_Origin_and_Spread_of_Goat_Pastoralism/links/5a0485b5a6fdcceda02baefa/Origin-and-Spread-of-Goat-Pastoralism.pdf. (Accessed December 9, 2023)
4. Cao, Y, Feng, T, Wu, Y, Xu, Y, Du, L, Wang, T, et al. The multi-kingdom microbiome of the goat gastrointestinal tract. Microbiome. (2023) 11:219. doi: 10.1186/s40168-023-01651-6
5. Pulina, G, Milán, MJ, Lavín, MP, Theodoridis, A, Morin, E, Capote, J, et al. Invited review: current production trends, farm structures, and economics of the dairy sheep and goat sectors. J Dairy Sci. (2018) 101:6715–29. doi: 10.3168/jds.2017-14015
6. Monica, K. Influence of dairy Farming practices on milk production. A critical literature review. Anim Health J. (2022) 3:1–15. doi: 10.47941/ahj.771
7. Nayik, GA, Jagdale, YD, Gaikwad, SA, Devkatte, AN, Dar, AH, and Ansari, MJ. Nutritional profile, processing and potential products: a comparative review of goat milk. Dairy. (2022) 3:622–47. doi: 10.3390/dairy3030044
8. Xiaopa, G. Based on the milk goats industry construction projects of Feihe dairy, study the development of Fuping County Shaanxi Province milk goats industry. Feed Rev. (2015) Available at: https://api.semanticscholar.org/CorpusID:114375686
9. Korma, SA, Li, L, Wei, W, Liu, P, Zhang, X, Bakry, IA, et al. A comparative study of Milk fat extracted from the Milk of different goat breeds in China: fatty acids, triacylglycerols and thermal and spectroscopic characterization. Biomolecules. (2022) 12:730. doi: 10.3390/biom12050730
10. Zhang, L. Genetic diversity of five goat breeds in China based on microsatellite markers. Afr J Biotechnol. (2012) 11:54. doi: 10.5897/AJB12.539
11. Fan, L, Shen, J, Li, X, Li, H, Shao, Y, Lu, CD, et al. Analysis of temporal changes of microbiota diversity and environmental interactions in Saanen dairy goats. J Appl Anim Res. (2023) 51:749–63. doi: 10.1080/09712119.2023.2273945
12. Idamokoro, EM. The significance of goat milk in enhancing nutrition security: a scientiometric evaluation of research studies from 1966 to 2020. Agric Food Secur. (2023) 12:34. doi: 10.1186/s40066-023-00441-5
13. Rout, PK, and Behera, BK. Goat and sheep farming In: PK Rout and BK Behera, editors. Sustainability in ruminant livestock: management and marketing. Singapore: Springer (2021). 33–76.
14. Harper, K, Tait, A, Li, X, Sullivan, M, Gaughan, J, Poppi, D, et al. Livestock industries in Australia: production systems and management (2021). 79–139.
15. Massender, E, Brito, LF, Maignel, L, Oliveira, HR, Jafarikia, M, Baes, CF, et al. Single-step genomic evaluation of milk production traits in Canadian Alpine and Saanen dairy goats. J Dairy Sci. (2022) 105:2393–407. doi: 10.3168/jds.2021-20558
16. Lima, ARC, Silveira, RMF, Castro, MSM, De Vecchi, LB, da Rocha Fernandes, MHM, and de Resende, KT. Relationship between thermal environment, thermoregulatory responses and energy metabolism in goats: a comprehensive review. J Therm Biol. (2022) 109:103324. doi: 10.1016/j.jtherbio.2022.103324
17. Li, X, Yang, J, Shen, M, Xie, X-L, Liu, G-J, Xu, Y-X, et al. Whole-genome resequencing of wild and domestic sheep identifies genes associated with morphological and agronomic traits. Nat Commun. (2020) 11:2815. doi: 10.1038/s41467-020-16485-1
18. Li, X, Liu, Q, Fu, C, Li, M, Li, C, Li, X, et al. Characterizing of structural variants based on graph-genotyping provides insights into pig domestication and local adaption. J Genet Genomics. (2023) S1673-8527:00241–2. doi: 10.1016/j.jgg.2023.11.005
19. Xia, X, Zhang, F, Li, S, Luo, X, Peng, L, Dong, Z, et al. Structural variation and introgression from wild populations in East Asian cattle genomes confer adaptation to local environment. Genome Biol. (2023) 24:211. doi: 10.1186/s13059-023-03052-2
20. Chen, N, Xia, X, Hanif, Q, Zhang, F, Dang, R, Huang, B, et al. Global genetic diversity, introgression, and evolutionary adaptation of indicine cattle revealed by whole genome sequencing. Nat Commun. (2023) 14:7803. doi: 10.1038/s41467-023-43626-z
21. Bolger, AM, Lohse, M, and Usadel, B. Trimmomatic: a flexible trimmer for Illumina sequence data. Bioinformatics. (2014) 30:2114–20. doi: 10.1093/bioinformatics/btu170
22. Li, H, and Durbin, R. Fast and accurate short read alignment with Burrows–Wheeler transform. Bioinformatics. (2009) 25:1754–60. doi: 10.1093/bioinformatics/btp324
23. Li, H, Handsaker, B, Wysoker, A, Fennell, T, Ruan, J, Homer, N, et al. The sequence alignment/map format and SAMtools. Bioinformatics. (2009) 25:2078–9. doi: 10.1093/bioinformatics/btp352
24. McKenna, A, Hanna, M, Banks, E, Sivachenko, A, Cibulskis, K, Kernytsky, A, et al. The genome analysis toolkit: a MapReduce framework for analyzing next-generation DNA sequencing data. Genome Res. (2010) 20:1297–303. doi: 10.1101/gr.107524.110
25. Wang, K, Li, M, and Hakonarson, H. ANNOVAR: functional annotation of genetic variants from high-throughput sequencing data. Nucleic Acids Res. (2010) 38:e164. doi: 10.1093/nar/gkq603
26. Purcell, S, Neale, B, Todd-Brown, K, Thomas, L, Ferreira, MAR, Bender, D, et al. PLINK: a tool set for whole-genome association and population-based linkage analyses. Am J Hum Genet. (2007) 81:559–75. doi: 10.1086/519795
27. Danecek, P, Auton, A, Abecasis, G, Albers, CA, Banks, E, DePristo, MA, et al. The variant call format and VCFtools. Bioinformatics. (2011) 27:2156–8. doi: 10.1093/bioinformatics/btr330
28. Zhang, C, Dong, S-S, Xu, J-Y, He, W-M, and Yang, T-L. PopLDdecay: a fast and effective tool for linkage disequilibrium decay analysis based on variant call format files. Bioinformatics. (2019) 35:1786–8. doi: 10.1093/bioinformatics/bty875
29. Kumar, S, Stecher, G, Li, M, Knyaz, C, and Tamura, K. MEGA X: molecular evolutionary genetics analysis across computing platforms. Mol Biol Evol. (2018) 35:1547–9. doi: 10.1093/molbev/msy096
30. Letunic, I, and Bork, P. Interactive Tree Of Life (iTOL) v5: an online tool for phylogenetic tree display and annotation. Nucleic Acids Res. (2021) 49:W293–6. doi: 10.1093/nar/gkab301
31. Patterson, N, Price, AL, and Reich, D. Population structure and eigenanalysis. PLoS Genet. (2006) 2:e190. doi: 10.1371/journal.pgen.0020190
32. Alexander, DH, and Lange, K. Enhancements to the ADMIXTURE algorithm for individual ancestry estimation. BMC Bioinformatics. (2011) 12:246. doi: 10.1186/1471-2105-12-246
33. Korneliussen, TS, Moltke, I, Albrechtsen, A, and Nielsen, R. Calculation of Tajima’s D and other neutrality test statistics from low depth next-generation sequencing data. BMC Bioinformatics. (2013) 14:289. doi: 10.1186/1471-2105-14-289
34. Chen, H, Patterson, N, and Reich, D. Population differentiation as a test for selective sweeps. Genome Res. (2010) 20:393–402. doi: 10.1101/gr.100545.109
35. Quinlan, AR, and Hall, IM. BEDTools: a flexible suite of utilities for comparing genomic features. Bioinformatics. (2010) 26:841–2. doi: 10.1093/bioinformatics/btq033
36. Bu, D, Luo, H, Huo, P, Wang, Z, Zhang, S, He, Z, et al. KOBAS-i: intelligent prioritization and exploratory visualization of biological functions for gene enrichment analysis. Nucleic Acids Res. (2021) 49:W317–25. doi: 10.1093/nar/gkab447
37. Jin, L, Qu, K, Hanif, Q, Zhang, J, Liu, J, Chen, N, et al. Whole-genome sequencing of endangered Dengchuan cattle reveals its genomic diversity and selection signatures. Front Genet. (2022) 13:833475. doi: 10.3389/fgene.2022.833475
38. Jin, L, Zhang, B, Luo, J, Li, J, Liang, J, Wu, W, et al. Genomics, origin and selection signals of Loudi cattle in Central Hunan. Biology. (2022) 11:1775. doi: 10.3390/biology11121775
39. Palombo, V, Milanesi, M, Sgorlon, S, Capomaccio, S, Mele, M, Nicolazzi, E, et al. Genome-wide association study of milk fatty acid composition in Italian Simmental and Italian Holstein cows using single nucleotide polymorphism arrays. J Dairy Sci. (2018) 101:11004–19. doi: 10.3168/jds.2018-14413
40. Liu, T, Feng, H, Yousuf, S, Xie, L, and Miao, X. Differential regulation of mRNAs and lnc RNAs related to lipid metabolism in Duolang and Small Tail Han sheep. Sci Rep. (2022) 12:11157. doi: 10.1038/s41598-022-15318-z
41. Juengel, JL, Mosaad, EMO, Mitchell, MD, Phyn, CVC, French, MC, Meenken, ED, et al. Relationships between prostaglandin concentrations, a single nucleotide polymorphism in HSD17B12, and reproductive performance in dairy cows. J Dairy Sci. (2022) 105:4643–52. doi: 10.3168/jds.2021-21298
42. Ariyarathne, HBPC, Correa-Luna, M, Blair, HT, Garrick, DJ, and Lopez-Villalobos, N. Identification of genomic regions associated with concentrations of milk fat, protein, urea and efficiency of crude protein utilization in grazing dairy cows. Genes. (2021) 12:456. doi: 10.3390/genes12030456
43. Ibeagha-Awemu, EM, Peters, SO, Akwanji, KA, Imumorin, IG, and Zhao, X. High density genome wide genotyping-by-sequencing and association identifies common and low frequency SNPs, and novel candidate genes influencing cow milk traits. Sci Rep. (2016) 6:31109. doi: 10.1038/srep31109
44. Dervishi, E, Joy, M, Sanz, A, Alvarez-Rodriguez, J, Molino, F, and Calvo, JH. Forage preservation (grazing vs. hay) fed to ewes affects the fatty acid profile of milk and CPT1B gene expression in the sheep mammary gland. BMC Vet Res. (2012) 8:106. doi: 10.1186/1746-6148-8-106
45. Fang, X, Zhao, Z, Jiang, P, Yu, H, Xiao, H, and Yang, R. Identification of the bovine HSL gene expression profiles and its association with fatty acid composition and fat deposition traits. Meat Sci. (2017) 131:107–18. doi: 10.1016/j.meatsci.2017.05.003
46. Cai, C, Li, M, Zhang, Y, Meng, S, Yang, Y, Gao, P, et al. Comparative transcriptome analyses of Longissimus thoracis between pig breeds differing in muscle characteristics. Front Genet. (2020) 11:526309. doi: 10.3389/fgene.2020.526309
47. Alaedin, M, Ghaffari, MH, Sadri, H, Meyer, J, Dänicke, S, Frahm, J, et al. Effects of dietary l-carnitine supplementation on the response to an inflammatory challenge in mid-lactating dairy cows: hepatic mRNA abundance of genes involved in fatty acid metabolism. J Dairy Sci. (2021) 104:11193–209. doi: 10.3168/jds.2021-20226
48. Li, Z, Li, R, Ren, H, Qin, C, Su, J, Song, X, et al. Role of different members of the AGPAT gene family in milk fat synthesis in Bubalus bubalis. Genes. (2023) 14:2072. doi: 10.3390/genes14112072
49. Lin, X, Wei, Y, Li, Y, Xiong, Y, Fang, B, Li, C, et al. Tormentic acid ameliorates hepatic fibrosis in vivo by inhibiting glycerophospholipids metabolism and PI3K/Akt/mTOR and NF-κB pathways: based on transcriptomics and metabolomics. Front Pharmacol. (2022) 13:801982. doi: 10.3389/fphar.2022.801982
50. Yilmaz, O, Kizilaslan, M, Arzik, Y, Behrem, S, Ata, N, Karaca, O, et al. Genome-wide association studies of preweaning growth and in vivo carcass composition traits in Esme sheep. J Anim Breed Genet. (2022) 139:26–39. doi: 10.1111/jbg.12640
51. Li, X, Yuan, L, Wang, W, Zhang, D, Zhao, Y, Chen, J, et al. Whole genome re-sequencing reveals artificial and natural selection for milk traits in east Friesian sheep. Front Vet Sci. (2022) 9:1034211. doi: 10.3389/fvets.2022.1034211
52. Guan, D, Landi, V, Luigi-Sierra, MG, Delgado, JV, Such, X, Castelló, A, et al. Analyzing the genomic and transcriptomic architecture of milk traits in Murciano-Granadina goats. J Anim Sci Biotechnol. (2020) 11:35. doi: 10.1186/s40104-020-00435-4
53. Lien, Y-C, Wang, PZ, Lu, XM, and Simmons, RA. Altered transcription factor binding and gene bivalency in islets of intrauterine growth retarded rats. Cells. (2020) 9:1435. doi: 10.3390/cells9061435
54. Liu, M, Zhou, Y, Rosen, BD, Van Tassell, CP, Stella, A, Tosser-Klopp, G, et al. Diversity of copy number variation in the worldwide goat population. Heredity. (2019) 122:636–46. doi: 10.1038/s41437-018-0150-6
55. Xiong, J, Bao, J, Hu, W, Shang, M, and Zhang, L. Whole-genome resequencing reveals genetic diversity and selection characteristics of dairy goat. Front Genet. (2023) 13:1044017. doi: 10.3389/fgene.2022.1044017
56. Wei, CB, He, F, Tang, JC, Yang, YH, Deng, XL, and Liu, FX. DNA sequence polymorphism within the bovine adenosine monophosphate deaminase 1 (AMPD1) is associated with production traits in Chinese cattle. Genet Mol Res. (2015) 14:1025–33. doi: 10.4238/2015.February.6.6
57. Scholtens, M, Jiang, A, Smith, A, Littlejohn, M, Lehnert, K, Snell, R, et al. Genome-wide association studies of lactation yields of milk, fat, protein and somatic cell score in New Zealand dairy goats. J Anim Sci Biotechnol. (2020) 11:55. doi: 10.1186/s40104-020-00453-2
58. Norris, BJ, and Whan, VA. A gene duplication affecting expression of the ovine ASIP gene is responsible for white and black sheep. Genome Res. (2008) 18:1282–93. doi: 10.1101/gr.072090.107
59. Rochus, CM, Westberg Sunesson, K, Jonas, E, Mikko, S, and Johansson, AM. Mutations in ASIP and MC1R: dominant black and recessive black alleles segregate in native Swedish sheep populations. Anim Genet. (2019) 50:712–7. doi: 10.1111/age.12837
60. Teng, J, Wang, D, Zhao, C, Zhang, X, Chen, Z, Liu, J, et al. Longitudinal genome-wide association studies of milk production traits in Holstein cattle using whole-genome sequence data imputed from medium-density chip data. J Dairy Sci. (2023) 106:2535–50. doi: 10.3168/jds.2022-22277
61. Li, D, Sun, G, Zhang, M, Cao, Y, Zhang, C, Fu, Y, et al. Breeding history and candidate genes responsible for black skin of Xichuan black-bone chicken. BMC Genomics. (2020) 21:511. doi: 10.1186/s12864-020-06900-8
62. Miliara, X, Tatsuta, T, Berry, J-L, Rouse, SL, Solak, K, Chorev, DS, et al. Structural determinants of lipid specificity within ups/PRELI lipid transfer proteins. Nat Commun. (2019) 10:1130. doi: 10.1038/s41467-019-09089-x
63. Ying, C, Yangsheng, W, Jiapeng, L, Liqin, W, Xiaolin, L, Mingjun, L, et al. Transcriptome profiles of pre-pubertal and adult in vitro matured ovine oocytes obtained from FSH-stimulated animals. Reprod Domest Anim. (2021) 56:1085–94. doi: 10.1111/rda.13951
64. Gao, L, Jia, G, Li, A, Ma, H, Huang, Z, Zhu, S, et al. RNA-Seq transcriptome profiling of mouse oocytes after in vitro maturation and/or vitrification. Sci Rep. (2017) 7:13245. doi: 10.1038/s41598-017-13381-5
65. Hu, Y, Yu, L, Fan, H, Huang, G, Wu, Q, Nie, Y, et al. Genomic signatures of coevolution between nonmodel mammals and parasitic roundworms. Mol Biol Evol. (2021) 38:531–44. doi: 10.1093/molbev/msaa243
66. Song, G, Spencer, TE, and Bazer, FW. Cathepsins in the ovine uterus: regulation by pregnancy, progesterone, and interferon tau. Endocrinology. (2005) 146:4825–33. doi: 10.1210/en.2005-0768
67. Shen, Y, Su, Y, Silva, FJ, Weller, AH, Sostre-Colón, J, Titchenell, PM, et al. Shared PPARα/γ target genes regulate brown adipocyte thermogenic function. Cell Rep. (2020) 30:3079–3091.e5. doi: 10.1016/j.celrep.2020.02.032
68. Russo, V, Fontanesi, L, Scotti, E, Beretti, F, Davoli, R, Nanni Costa, L, et al. Single nucleotide polymorphisms in several porcine cathepsin genes are associated with growth, carcass, and production traits in Italian large white pigs. J Anim Sci. (2008) 86:3300–14. doi: 10.2527/jas.2008-0920
69. van Bilsen, JHM, Dulos, R, van Stee, MF, Meima, MY, Rouhani Rankouhi, T, Neergaard Jacobsen, L, et al. Seeking windows of opportunity to shape lifelong immune health: a network-based strategy to predict and prioritize markers of early life immune modulation. Front Immunol. (2020) 11:644. doi: 10.3389/fimmu.2020.00644
70. Nishida, N, Aiba, Y, Hitomi, Y, Kawashima, M, Kojima, K, Kawai, Y, et al. NELFCD and CTSZ loci are associated with jaundice-stage progression in primary biliary cholangitis in the Japanese population. Sci Rep. (2018) 8:8071. doi: 10.1038/s41598-018-26369-6
71. Mailu, BM, Li, L, Arthur, J, Nelson, TM, Ramasamy, G, Fritz-Wolf, K, et al. Plasmodium apicoplast Gln-tRNAGln biosynthesis utilizes a unique GatAB amidotransferase essential for erythrocytic stage parasites. J Biol Chem. (2015) 290:29629–41. doi: 10.1074/jbc.M115.655100
72. Wang, D, Ning, C, Xiang, H, Zheng, X, Kong, M, Yin, T, et al. Polymorphism of mitochondrial tRNA genes associated with the number of pigs born alive. J Anim Sci Biotechnol. (2018) 9:86. doi: 10.1186/s40104-018-0299-0
73. Zhang, Y, Labrecque, R, Tremblay, P, Plessis, C, Dufour, P, Martin, H, et al. Sperm-borne tsRNAs and miRNAs analysis in relation to dairy cattle fertility. Theriogenology. (2023) 215:241–8. doi: 10.1016/j.theriogenology.2023.11.029
74. Dettori, ML, Pazzola, M, Paschino, P, Amills, M, and Vacca, GM. Association between the GHR, GHRHR, and IGF1 gene polymorphisms and milk yield and quality traits in Sarda sheep. J Dairy Sci. (2018) 101:9978–86. doi: 10.3168/jds.2018-14914
75. Wu, M, Zhao, H, Tang, X, Li, Q, Yi, X, Liu, S, et al. Novel InDels of GHR, GHRH, GHRHR and their association with growth traits in seven Chinese sheep breeds. Animals. (2020) 10:1883. doi: 10.3390/ani10101883
76. Pazzola, M, Vacca, GM, Paschino, P, Bittante, G, and Dettori, ML. Novel genes associated with dairy traits in Sarda sheep. Animals. (2021) 11:2207. doi: 10.3390/ani11082207
Keywords: dairy goat, whole-genome sequence, genetic diversity, selective signal, production traits
Citation: Zhao J, Mu Y, Gong P, Liu B, Zhang F, Zhu L, Shi C, Lv X and Luo J (2024) Whole-genome resequencing of native and imported dairy goat identifies genes associated with productivity and immunity. Front. Vet. Sci. 11:1409282. doi: 10.3389/fvets.2024.1409282
Edited by:
Shijie Lyu, Henan Academy of Agricultural Sciences (HNAAS), ChinaReviewed by:
Gan Shangquan, Xinjiang Academy of Agricultural and Reclamation Sciences (XAARS), ChinaMarina Mortati Dias Barbero, Federal Rural University of Rio de Janeiro, Brazil
Copyright © 2024 Zhao, Mu, Gong, Liu, Zhang, Zhu, Shi, Lv and Luo. This is an open-access article distributed under the terms of the Creative Commons Attribution License (CC BY). The use, distribution or reproduction in other forums is permitted, provided the original author(s) and the copyright owner(s) are credited and that the original publication in this journal is cited, in accordance with accepted academic practice. No use, distribution or reproduction is permitted which does not comply with these terms.
*Correspondence: Jun Luo, luojun@nwafu.edu.cn