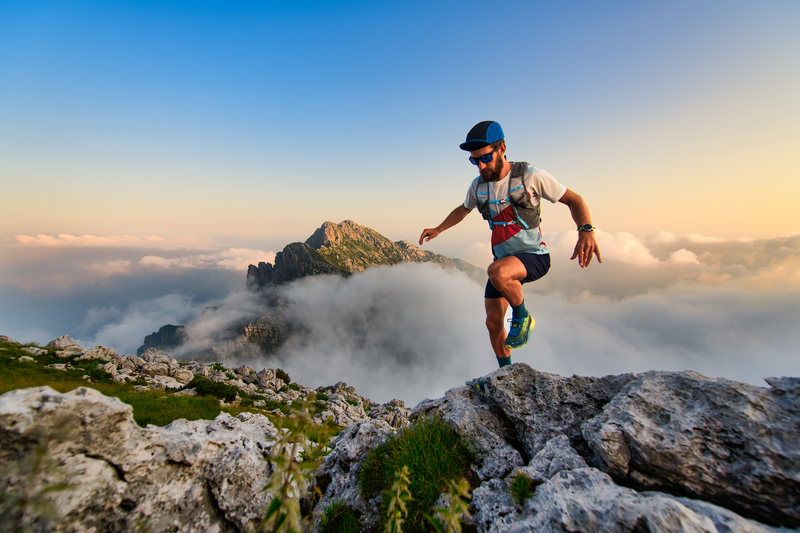
94% of researchers rate our articles as excellent or good
Learn more about the work of our research integrity team to safeguard the quality of each article we publish.
Find out more
ORIGINAL RESEARCH article
Front. Vet. Sci. , 29 May 2024
Sec. Animal Reproduction - Theriogenology
Volume 11 - 2024 | https://doi.org/10.3389/fvets.2024.1399776
This article is part of the Research Topic Exploring 'Omic' Biomarkers in Animal Production and Reproduction View all 8 articles
Poultry broodiness can cause ovarian atresia, which has a detrimental impact on egg production. Non-coding RNAs (ncRNAs) have become one of the most talked-about topics in life sciences because of the increasing evidence of their novel biological roles in regulatory systems. However, the molecular mechanisms of ncRNAs functions and processes in chicken ovarian development remain largely unknown. Whole-transcriptome RNA sequencing of the ovaries of broodiness and laying chickens was thus performed to identify the ncRNA regulatory mechanisms associated with ovarian atresia in chickens. Subsequent analysis revealed that the ovaries of laying chickens and those with broodiness had 40 differentially expressed MicroRNA (miRNAs) (15 up-regulated and 25 down-regulated), 379 differentially expressed Long Noncoding RNA (lncRNAs) (213 up-regulated and 166 down-regulated), and 129 differentially expressed circular RNA (circRNAs) (63 up-regulated and 66 down-regulated). The competing endogenous RNAs (ceRNA) network analysis further revealed the involvement of ECM-receptor interaction, AGE-RAGE signaling pathway, focal adhesion, cytokine-cytokine receptor interaction, inflammatory mediator regulation of TRP channels, renin secretion, gap junction, insulin secretion, serotonergic synapse, and IL-17 signaling pathways in broodiness. Upon further analysis, it became evident that THBS1 and MYLK are significant candidate genes implicated in the regulation of broodiness. The expression of these genes is linked to miR-155-x, miR-211-z, miR-1682-z, gga-miR-155, and gga-miR-1682, as well as to the competitive binding of novel_circ_014674 and MSTRG.3306.4. The findings of this study reveal the existence of a regulatory link between non-coding RNAs and their competing mRNAs, which provide a better comprehension of the ncRNA function and processes in chicken ovarian development.
Broodiness, a natural maternal behavior observed in chickens, turkeys, and geese, is characterized by appetite loss and a halt in egg-laying and incubation activities. This phenomenon poses a challenge to the progress of the modern poultry industry. Generally, broodiness is initiated by a signal that stops egg production (1). The synchronization of hormones secreted by the hypothalamic-pituitary-gonadal (HPG) axis is a prerequisite for the maintenance of broodiness (2). Studies postulate that the ovary is larger in weight and volume during the laying stage than during the broodiness stage. The ovarian weight of the egg-laying chickens was 48.17 g, significantly higher than the 2.67 g weight observed in broody chickens. The upper-grade follicles in the ovary are in active development during the laying stage, while the inner of the ovary is composed of atrophic and atretic follicles during the broodiness stage (3, 4). The eukaryotic genome has a much lower gene density compared to the prokaryotic genome, reflecting an efficient evolutionary adaptation to meet its complex biological demands. Both protein-coding and non-protein-coding RNA play a role in transcriptional regulation. The human genome transcribes over 90% of its content. As a result, the majority of transcribed genes generate noncoding RNAs (ncRNAs), which have primarily function as regulatory elements (5).
Analysis of RNA sequences during DNA transcription reveals that coding RNAs, responsible for protein translation, make up only approximately 4% of total RNA. Conversely, ncRNAs are widely distributed and play diverse roles in gene regulation (6). Both protein-coding and non-protein-coding RNA play a role in transcriptional regulation. Notably, most of the transcriptome comprises ncRNAs (7). Several studies postulate that non-coding RNAs (miRNA, lncRNA, lncRNA, siRNA) play a role in controlling ovarian development and performance. In particular, miR-182 and miR-15a have been reported to modulate granulosa cell proliferation and apoptosis by altering hormone production (8, 9). LncRNAs also serve as regulators in the reproductive process of poultry. Noteworthy, Mao et al. (10) discovered 24,601 lncRNAs linked to egg production in domestic pigeons. Over the last few years, circRNA has become a popular topic in RNA research because of its involvement in the regulation of multiple molecules in organisms through post-transcriptional regulation of classical signaling pathways (11). CircDDX10 is associated with ovarian aging, circTCP11 with pig litter size, and chi_circ_0008219 with goat reproductive rate (12–14). Despite the significance of ncRNA as vital regulators in many biological processes, their molecular regulatory mechanisms during broodiness of Chengkou mountain chicken remain unknown.
Chengkou mountain chicken is a famous local chicken variety in China, mainly reared in Chongqing municipality. However, it has a low production efficiency and high breeding costs because of its strong broodiness ability (around 90%), low egg production, and slow early growth rate. This study aimed to identify and analyze the differentially expressed non-coding RNAs and their expression patterns of Chengkou mountain chicken with a normal laying ovary and ovary with broodiness using whole-transcriptome RNA-seq. The findings of this study provide insights into the molecular processes that control the broodiness of Chengkou mountain chicken, which lay a basis for the utilization of marker-assisted selection and genetic improvement of chicken.
The experimental animals were 6 chickens from the same batch obtained from the Chengkou Mountain Chickens Genetic Research Institute (Chongqing, China). The Chengkou mountain chickens exhibits favorable germplasm traits, including robust foraging ability, resistance to coarse feeding, suitability for wild grazing and free range, strong disease resistance, and high adaptability. Their egg production and broody situation were recorded daily. Three individuals exhibiting broody behavior and entering the classic broody phase 30 days earlier were chosen. Their ovarian tissues were harvested in liquid nitrogen for RNA and transcriptome sequencing and then stored at −80°C awaiting RNA extraction.
The histological characteristics of chicken ovaries were evaluated through hematoxylin and eosin staining, following paraffin embedding of the samples and sectioning. The histological ovary micromorphology was visualized using a microscope (Olympus IX53, Japan), followed by image capture using the microphotographic system (Olympus DP71, Japan). The ultrastructure of the chicken ovaries was observed using a transmission electron microscope (TEM Hitachi HT7800/HT7700).
The data quality of RNA Q30 in each sample was more than 92.30%, indicating that the sequencing data quality was good (Supplementary material 4). The total RNA of the ovary tissues was extracted using the Trizol reagent kit (Invitrogen, Carlsbad, CA, United States) following the manufacturer’s instructions. RNA quality was assessed using an Agilent 2100 Bioanalyzer (Agilent Technologies, Palo Alto, CA, United States) and RNase-free agarose gel electrophoresis. After total RNA was extracted, rRNAs were removed to retain mRNAs and ncRNAs. The enriched mRNAs and ncRNAs were fragmented into short fragments by using fragmentation buffer and reverse transcribed into cDNA with random primers. Second-strand cDNAs were synthesized using DNA polymerase I, RNase H, dNTPs (dUTP instead of dTTP), and buffer. The cDNA fragments were then purified using the QiaQuick PCR extraction kit (Qiagen, Venlo, The Netherlands), end-repaired, poly(A) added, and ligated to Illumina sequencing adapters. The second-strand cDNAs were digested using UNG (uracil-N-glycosylase), after which they were size selected by agarose gel electrophoresis, PCR amplified, and sequenced using Illumina HiSeqTM 4000 (Gene Denovo Biotechnology Co, Guangzhou, China). Then the 3′ adapters were added and the 36–48 nt RNAs were enriched. The 5′ adapters were then ligated to the RNAs as well. The ligation products were reverse transcribed through PCR amplification, and the 140–160 bp size PCR products were enriched to generate 6 cDNA libraries, which was sequenced using Illumina HiSeq Xten (Gene Denovo Biotechnology Co, Guangzhou, China).
To refine the raw data, we exclude reads with low quality (Q-value ≤20), reads that include adapters, reads shorter than 18 nt, and reads featuring polyA (15). The clean tags were then aligned with small RNAs in the GeneBank database (Release 209.0) and Rfam database (Release 11.0) to identify and remove rRNA, scRNA, snoRNA, snRNA, and tRNA. All of the clean tags were then searched against miRBase database (Release 22) to identify known (Species studied) miRNAs (exist miRNAs). After tags were annotated as mentioned previously, the annotation results were determined in this priority order: rRNA etc. > exist miRNA > exist miRNA edit > known miRNA > repeat > exon > novel miRNA > intron. The tags that cannot be annotated as any of the above molecules were recorded as unann.
Filtration of the raw data involved removing reads of low quality (Q-value ≤20), reads with adapters, reads composed entirely of A bases, and reads containing more than 10% unknown nucleotides. Following this, alignment of the clean data with chicken rRNA sequences using Bowtie2 facilitated the removal of reads that aligned with rRNA (16). Softwares CNCI (17) (version 2) and CPC (18) (version 0.9-r2)1 and FEELNC (19) (version v0.2)2 were used to assess the protein-coding potential of the novel transcripts using their default parameters. The final prediction for lncRNAs resulted from the intersection of three software programs.
The short reads alignment tool Bowtie2 (16) (version 2.2.8) was thus used to map the reads to the ribosomal RNA (rRNA) database. The rRNA-mapped reads were removed, and the remaining reads were further used for other alignments and analyses. The rRNA-removed reads from each sample were then mapped to the reference genome using HISAT2 (20) (version 2.1.1). Reads that mapped to the reference genomes were discarded, while the unmapped reads were used for circRNA identification. Anchor reads that aligned in the reversed orientation (head-to-tail) indicated circRNA splicing and were subjected to find_circ to identify the circRNAs (21). We identified ncRNAs with fold change ≥2 and FDR (false discovery rate) <0.05 in a comparison as significant DE ncRNAs.
The ceRNA network was constructed based on the ceRNA theory as follows: (1) the target relationship between miRNA and candidate ceRNA and the negative correlation of expression, (2) the positive correlation between the expression levels of candidate ceRNAs, and (3) enrichment degree of candidate ceRNA binding to the same miRNA. miRNA-target gene pairs were first identified because ceRNAs are mutually regulated by miRNAs. The target genes for differential miRNAs were then predicted as the first step in the study of ceRNA regulatory networks. The expression correlation between mRNA-miRNA, lncRNA-miRNA, and circRNA-miRNA was then evaluated using the Spearman rank correlation coefficient (SCC). Pairs with SCC <−0.7 were selected as negatively co-expressed lncRNA-miRNA pairs, mRNA-miRNA pairs, or circRNA-miRNA pairs. The mRNAs, lncRNAs, and circRNAs were miRNA target genes and were all differentially expressed. The expression correlation between lncRNA-mRNA and circRNA-mRNA was evaluated using the Pearson correlation coefficient (PCC). Pairs with PCC >0.9 were selected as co-expressed lncRNA-mRNA pairs or circRNA-mRNA pairs. Both the mRNA and lncRNA or mRNA and circRNA in these pairs were targeted and co-expressed negatively with a common miRNA. A hypergeometric cumulative distribution function test was then done to test whether the common miRNA sponges between the two genes were significant. Only the gene pairs with a p-value less than 0.05 were selected. For each gene pair (A, B), we denoted all their regulator miRNAs as miRNA set C (regulating gene A) and D (regulating gene B). Where x stands for the number of common miRNAs that regulate both genes, U is the number of all the miRNAs in the work, M is the size of miRNA set C, and N is the size of miRNA set D. The lncRNA-miRNA-mRNA network was constructed by assembling all the identified co-expression competing triplets, and was visualized using Cytoscape software (v3.6.0).3
GO functional and KEGG pathway analysis of miRNA, lncRNA, and circRNA target genes was conducted using DAVID and KOBAS software (22, 23). Go terms and KEGG pathways with a p-value <0.05 were considered to be significantly enriched.
Real-time quantitative PCR (RT-qPCR) was performed to validate the expression levels of DE circRNAs, DE lncRNAs, and DE miRNAs. Four lncRNAs, four miRNAs, and four circRNAs associated with significantly differentially expressed genes in the ovary were randomly selected for the RT-qPCR validation tests. The methods of RNA reverse transcription and real-time fluorescence quantitative PCR were conducted as per previously published protocols (24). The relevant primers were designed using Primer Premier. As stated earlier, U6 should be referred to as the steward gene sequence of miRNA (23). The methods of RNA reverse transcription and real-time fluorescence quantitative PCR were conducted as per previously published protocols (24). While GAPDH was used as the housekeeping gene for lncRNA and circRNA. The relative miRNA and mRNA expression were calculated using the 2−∆∆Ct method (25), and data were expressed as mean ± standard deviation of the mean. The data was displayed as mean ± standard deviation, with variations analyzed via the independent-sample t-test, via SPSS 20.0 (SPSS Inc., United States).
The ovarian volume diminished as the laying stage progressed to the broodiness stage (Figures 1A,B). During the laying stage, the ovaries contain varying developmental and atretic follicles. However, follicle development stops, and different levels of atretic follicles are observed when approaching the broodiness stage. Noteworthy, the egg-laying hens had a higher ovary weight and volume and a heftier stroma weight, than the broody chicken (Table 1). Moreover, the broody chicken did not have large yellow follicles. The hen only had a few yellow follicles, with the majority being white.
Figure 1. Anatomical morphological observation of ovarian tissue in poultry was conducted by examining two hematoxylin and eosin stained sections. (A) Normal ovarian tissue that continuously lays eggs. (B) Atrophy of ovaries for 30 days of broodiness. (C) Histological characteristics in egg-laying hens (staining at 200 μm). (D) Histological characteristics in egg-laying hens (staining at 50 μm). (E) Histological characteristics in broody hens (staining at 200 μm). (F) Histological characteristics in broody hens (staining at 50 μm). (G) The mitochondrial ultrastructure of the chicken egg-laying ovaries were analyzed by TEM. (H) The mitochondrial ultrastructure of the chicken broody ovaries were analyzed by TEM.
Table 1. A comparison of the ovarian morphological characteristics between egg-laying and broody hens (n = 6).
The ovaries of chicken in the broodiness group had fewer follicles, a thicker granular layer, sparse cell density, and indistinct inner and outer layers compared to the ovaries of hens in the normal laying group (Figures 1C–F). Moreover, the ultrastructure of the ovary of chicken in the broodiness group revealed a lack of nucleus and a pattern of mitochondrial aggregation (Figures 1G,H).
Transcriptome sequencing yielded 38,460,846 and 37,304,337 total raw reads from the broodiness and egg-laying groups, respectively. Subsequent filtering of the raw reads was done to acquire clean tags with more than 97% accuracy. As Figures 2A,B shown, 96.8% of miRNAs were between 20 and 24 nt in length (Supplementary material 1). In Figures 2C,D, plots are shown comparing the tag abundances of non-coding RNA in GenBank and Rfam for each sample. The proportion of existing miRNA is the highest. A miRNA expression heat map was subsequently generated for each sample to study the miRNA patterns in the samples (Figure 2E). As shown in Supplementary Figure S4, PCA principal component analysis showed intersample repeatability of AO (atrophic ovaries) and NO (normal ovaries). We also provide a violin diagram in Supplementary Figure S6, showing better correlation between samples. Moreover, 40 differential expression (DE) miRNAs were identified in comparing the broodiness and laying groups. A scatterplot analysis was performed based on the significantly different miRNAs to demonstrate the differences between the comparison groups (Figure 2F). GO and KEGG analysis of the 40 DE miRNA genes was then done to investigate the role of miRNA in the laying and broodiness groups. A total of 104 GO terms were enriched. Figures 3A,B shows the top 20 terms of biological process (BP), cellular component (CC), and molecular function (MF). GO enrichment analysis revealed that the DE miRNAs were mainly enriched in membrane-bounded organelle cytoplasm and some intracellular-related items. Additionally, the target genes were found to be involved in various metabolic pathways, including cysteine and methionine metabolism, SNARE interactions in vesicular transport, protein processing in endoplasmic reticulum, pertussis, viral protein interaction with cytokine and cytokine receptors, ferroptosis, and peroxisome pathways (Figure 3C).
Figure 2. Overview of small RNA sequencing in the chicken ovary. (A) The length distribution of small RNA was AO1, AO2 and AO3, respectively. (B) The length distribution of small RNA was NO1, NO2 and NO3. (C) Statistical analysis of miRNA sequence abundance across various categories in each sample. (D) Statistical graph depicting the distribution of miRNA sequence species across various sample categories. exist_mirna (The miRNA of this species has been included in miRbase). known_miRNA (The identified miRNAs were compared with the known animal miRNAs in miRbase). novel_mirna (Combined with the reference sequence, the issuer structure was predicted and the miRNA was identified.) unann (The tags that cannot be annotated as any of the above molecules were recorded as unann). (E) Heatmap of differentially expressed miRNAs. (F) Compare the group NO –vs. -AO scatter plots.
Figure 3. GO and KEGG analysis of DE miRNAs. (A) The GO enrichment classification histogram of DEmiRNA is divided into three levels: BP, MF, and CC. (B) Top 20 significantly changed GOs of DEmiRNAs in biological processes. (C) The top 20 pathways significantly associated with differentially expressed miRNA transcripts.
RNA-seq yielded 292,328,750 and 268,349,060 total raw reads from the broodiness and laying groups, respectively. Thus, to get high quality clean reads, reads were further filtered by fastp (26) (version 0.18.0). Filtering was then done to obtain a clean data set with more than 95% accuracy. The intersection of the three software results, which is depicted in the Venn diagram, was taken as the final lncRNA prediction result (Figure 4A). Noteworthy, 9,640 intergenic lncRNA, 465 bidirectional lncRNA, 83 intronic lncRNA, 327 antisense lncRNA and 334 sense overlapping lncRNA were identified based on the position of the new lncRNA relative to the protein-coding gene (Figure 4B). We also identified 2,588 new lncRNA for subsequent analysis. The expression of lncRNA and transcripts in different samples were visualized using expression distribution and violin maps (Figures 4C,D). By comparing the laying group and the broodiness group had 379 DE lncRNA (213 up-regulated and 166 down-regulated). The expression patterns are depicted in the volcanic maps and heat maps (Figures 4E,F). Our study revealed that ENSGALT00000105586 is the top DElncRNAs (Figure 4E).
Figure 4. Overview of lncRNA sequencing in the chicken ovary. (A) CPC2, CNCI, and Feelnc were used to evaluate the encoding capabilities of all transcripts. The intersection of transcripts without coding potential is a reliable prediction result. (B) Total lncRNA type statistics. (C) lncRNA expression abundance distribution map. (D) Violin diagram of lncRNA expression. (E) Comparison of group NO-vs. -AO volcano maps. (F) Compare group NO-vs. -AO heat maps.
GO analysis revealed that the lncRNA target genes were significantly enriched in processes related to extracellular activity, multicellular organismal processes, collagen fibril organization, and tissue development (Supplementary Figures S1A,B). The three most significant signaling pathways in the KEGG analysis of the lncRNA genes were ECM-receptor interaction, protein digestion and absorption, and focal adhesion (Supplementary Figure S1C).
We obtained 55,838,523 clean reads from 6 samples after strict filtering (Supplementary material 3). A total of 18,666 circRNAs were subsequently identified from the clean reads in both the broodiness and laying groups (Supplementary Table S3). Notably, these circRNAs were distributed on almost all chromosomes, with the highest concentration on chromosome 1 (Figure 5A). A majority (83.82%) of circRNAs had a length of less than 3,000 bp, amongst which 30.55% were less than 500 bp and 47.42% were between 500–2,000 bp, with an average length of 3,156 bp (Figure 5B). The most abundant circRNA type was annot_exons (12,869; Figure 5C). A comparison between the expression levels of circRNA in the broodiness group and the laying group yielded 129 DE circRNAs (p < 0.05; Figure 5D). These 129 DE circRNAs were further analyzed using a volcanic map, we has been determined in our investigation that novel_circ_018025 stands out as a top DEcircRNA (Figure 5E). A heat map analysis of the differential circRNA expression patterns was also conducted (Figure 5F).
Figure 5. Overview of circRNA sequencing in the chicken ovary. (A) All the circular RNA chromosome statistics. (B) All the circular RNA length distribution statistics. (C) Statistical map of circular RNA type distribution. (D) CircRNA statistical map of differences. (E) Comparison of group NO-vs. -AO volcano maps. (F) Differential circRNA clustering heat map.
GO enrichment analysis of the DE circRNAs revealed their involvement in histone demethylase, phosphoprotein phosphatase, and metalloendopeptidase activities (Supplementary Figure S2B). KEGG enrichment analysis further revealed that DE circRNAs were linked to adherens junction, lysine degradation, cell adhesion molecules, thiamine metabolism, and PI3K-Ark signaling pathway (Supplementary Figure S2C).
An analysis of the differential expression of miRNAs in the ovarian tissue revealed 258 lncRNA-miRNA-mRNA and 70 circRNA-miRNA-mRNA interaction pairs, encompassing 291 lncRNAs, 79 circRNAs, 40 miRNAs, and 346 mRNAs (Figures 6A,B). GO and KEGG enrichment analyses (Supplementary Figures S5A,B) revealed that these transcriptional interactions were associated with ECM-receptor interaction, AGE-RAGE signaling pathway, focal adhesion, cytokine–cytokine receptor interaction, inflammatory mediator regulation of TRP channels, renin secretion, gap junction, insulin secretion, serotonergic synapse, and IL-17 signaling pathway. Through KEGG and GO analysis, we identified differentially expressed genes within relevant pathways. Furthermore, 25 genes potentially associated with broodiness were identified (Table 2).
Figure 6. CeRNA network constructed by DEmRNAs, DElncRNAs, DEcircRNAs, and DEmiRNAs. (A) mRNA-miRNA-circRNA network. (B) mRNA-miRNA-lncRNA network. The shades of the colors indicate the upregulated (red) and downregulated (green) level. The characters of the figures correspond to different RNA species, round -mRNA, diamond-miRNA, v-circRNA, triangle-lncRNA.
Table 2. Twenty-five candidate genes associated with the regulation of broodiness and their binding pathways, and differentially expressed miRNA, lncRNA, and circRNA.
A lncRNA/circRNA-miRNA-mRNA regulatory network was subsequently created by constructing ceRNA networks of candidate genes (Figure 7). In the network, miRNAs were at its center and was associated with 4 circRNAs, 18 lncRNAs, 5 miRNAs, and 2 mRNAs. The ceRNA network analysis identified MYLK and THBS1 genes as potential targets of 2 and 3 differentially expressed miRNAs, and 8 and 10 lncRNAs, respectively. Moreover, four circRNAs had a regulatory effect on MYLK and THBS1 genes. The novel_circ_014674 and MSTRG.3306.4 potentially regulated MYLK and THBS1 genes via different miRNAs, which was consistent with the ceRNA regulatory hypothesis (27).
Figure 7. lncRNA/circRNA-miRNA-mRNA co-regulatory network. The characters of the figures correspond to different RNA species, round -mRNA, diamond -miRNA, to v-circRNA, triangle -lncRNA.
Seven transcripts were randomly selected from DE circRNA, DE lncRNA, and DE miRNA and subjected to RT-qPCR to verify the accuracy of the RNA sequencing data. Subsequent RT-qPCR validation demonstrated extremely significant differences (p < 0.01) in gga-miR-215-5p, gga-miR-489-3p, novel_circ_017769, and MSTRG.18953.2. Additionally, novel_circ_000405, MSTRG.18017.1, and MSTRG.10467.1 exhibited significant differences (p < 0.05), as depicted in Figure 8A. The log2FC values of DE miRNA, DE lncRNA, and DE circRNA obtained from the RT-qPCR results revealed that the seven genes exhibited a consistent expression trend with those of RNA-Seq data, thus confirming the accuracy of the sequencing results in Figure 8B.
Poultry broodiness is a complex characteristic with low heritability, it is a result of a combination of genetic influences, the endocrine system, and environmental factors (28). Broody behavior frequently results in follicular atresia and ovarian atrophy (29). In addition, the adoption of artificial incubation technology has eliminated the need for broody behavior in chicken production. Consequently, identifying potential targets that impact broody behavior serves as a method to enhance egg production in native chicken populations. The ovaries and fallopian tubes of broody chickens undergo degeneration, which impedes the growth of follicles and reduces the number of granulosa and thecal cells within them, thus hindering egg production (4, 30). During the brooding stage, Magang geese have very few large yellow follicles and small yellow follicles, yet the amount of large white follicles remains unchanged (31). In this study, histological examination revealed that the ovaries of broody chickens had atrophied, with atretic follicles, which is consistent with the results of other studies (32, 33). Transmission electron microscope (TEM) images of the ovarian ultrastructure suggested that the ovarian granulosa cells of polycystic ovarian syndrome (PCOS) model rats exhibited an accumulation of mitochondria, destruction of crests, and breaking up as opposed to those of normal rats (34). Granulosa cells with dysfunctional mitochondria can lead to a decrease in steroid production and oocyte maturation rate, resulting in reduced fertility. Conversely, functional mitochondria increase the steroid production of granulosa cells (35).
Non-coding RNAs are important in the biological processes of living organisms. The ceRNA hypothesis enables the exploration of potential regulatory mechanisms by combining multiple RNA information (36). In this study, we identified 40 differentially expressed miRNAs (DEmiRNAs), 379 differentially expressed long non-coding RNAs (DElncRNAs), and 129 differentially expressed circular RNAs (DEcircRNAs) in the ovaries of laying chickens and those with broodiness. Subsequent enrichment analysis enabled the construction of a hierarchical ceRNA network associated with ovarian development. The network identified miRNAs, such as miR-9-x, miR-4324-z, miR-155-x, gga-miR-143-5p, and miR-211-z, miR-155, miR-9, and miR-4324 are involved in the development of the ovary. miR-155 has an essential effect on the glycolysis of granule cells in PCOS sufferers, thereby controlling follicular dysplasia (37). miR-9 is highly expressed in the follicular fluid of PCOS patients, and its interaction with the vitamin D receptor modulates the proliferation and apoptosis of ovarian granulosa cells, thereby regulating follicle growth (38–40). Moreover, miR-9 increases during the ovarian development of tilapia, thereby affecting sex determination and differentiation by regulating dmrt1 expression (41). Notably, there are differences in the development process of miR-9 in different regions of the chick’s brain (42). miR-4324 is associated with cell proliferation and migration. Its expression is thus significantly reduced in ovarian cancer tissues. miR-4324 can be targeted and combined with FEN1 to impede cell proliferation in ovarian cancer (43, 44). Recent studies postulate that miR-143 has a regulatory effect on glucose metabolism and mitochondrial function in the placenta (45). Yang et al. (46) reported that miR-143-5p combines with CRIM1 to regulate endometrial receptivity in goats. In the same line, studies postulate that the regulatory axis of lncRNA PROX1-AS1, miR-211-5p, and caspase-9 is connected to preeclampsia and affects the presence of trophoblast cells (47).
Herein, miRNA, lncRNA, and circRNA source genes were significantly enriched in the ECM-receptor interaction pathway. ECM receptors are associated with the ripening of follicles and the reduction of the ovaries (34, 48). Research has demonstrated that extracellular matrix (ECM) components furnish tissue-specific frameworks that impact key cellular processes, including proliferation, differentiation, and apoptosis. The extensive alterations in ECM throughout follicular development necessitate a sequence of proteolytic events crucial for the regular progression, function, regression, and degeneration of follicles (49). Recent studies have indicated that matrix proteins play a significant role in regulating ECM turnover during follicular development or atresia. Apart from specifically degrading ECM components and activating other matrix metalloproteinases, these proteins also influence various signaling pathways by releasing numerous signaling proteins. This regulation impacts cell biology during both normal physiological processes and pathological conditions, involving growth factors, cytokines, cadherin E, Fas ligands, and tumor necrosis factors (50). Simultaneously, we observed potential regulatory interactions between miR-9-x and the genes FN1, TNC, COL3A1, PDGFD, KCNMB1, ACTA2, and GNAQ through the ceRNA network. Additionally, previous studies have indicated that FN1 is a principal constituent of the extracellular matrix (ECM) (51). Furthermore, an examination of high and low egg production in white Muscovy ducks identified potential candidate genes in both hypothalamic and ovarian tissue for the high-yield mechanism in this breed. Notably, the FN1 gene displayed significant expression levels in ovarian tissue and is implicated in this mechanism (52). Focal adhesion was also a significant pathway in the ceRNA network in this study. Previous studies have demonstrated its involvement in cell proliferation (53). Moreover, studies postulate that focal adhesion is a factor in the expression of the IGF-I gene and results in a difference in the gene’s expression in Muscovy ducks. IGF-I is responsible for controlling the expression of lncRNA through the focal adhesion pathway, thereby promoting cell proliferation and regulating the reproduction of Muscovy ducks (54). ECM-receptor interaction and focal adhesion are involved in the early development of geese and ducks ovaries (48). lncRNAs regulate the functioning of chicken ovaries through their interaction with ECM-receptor and focal adhesion pathways (55). In this study, focal adhesion was found to be an enrichment pathway associated with higher and lesser egg production, which is in line with other research findings (56). Through additional analysis of the ceRNA network, we have pinpointed two crucial genes: THBS1 and MYLK. However, there has been limited research on poultry involving THBS1 and MYLK. Increased THBS1 expression in rat follicles has been demonstrated to notably diminish angiogenesis while enhancing follicular atresia (57). In another study on ovarian cancer, it was observed that the inhibition of circRNA_MYLK led to a decrease in the proliferation of ovarian cancer cells (58). We hypothesized that ncRNAs modulate the broodiness behavior of poultry by regulating THBS1 and MYLK gene expression. The findings of this study revealed the existence of a regulatory connection between ncRNAs and their competing mRNAs, which provides a better understanding of the ceRNA function and processes in chicken ovarian development.
This study reveals 40 DE miRNAs, 379 DE lncRNAs, and 129 DE circRNAs from the ovaries of laying chickens and hens with broodiness. THBS1 and MYLK are candidate genes in the regulation of broody behavior, potentially influenced by miR-155-x, miR-211-z, miR-1682-z, gga-miR-155, and gga-miR-1682. Additionally, they may be subject to competitive binding by novo-el_circ_014674 and MSTRG.3306.4. The GO and KEGG enrichment analyses revealed a connection between the ECM-receptor interaction pathway and focal adhesion in the regulation of broody behavior, offering a novel avenue for investigating the regulatory network of Chengkou mountain chicken’s broody behavior further.
The raw data has been furnished to the National Center for Biotechnology Information (NCBI) Sequence Read Archive (SRA) database, assigned the accession number PRJNA1074948.
The animal study was approved by Experimental Animal Ethics Review Committee of Southwest University. The study was conducted in accordance with the local legislation and institutional requirements.
HX: Data curation, Software, Validation, Writing – original draft. WL: Formal analysis, Validation, Writing – review & editing. LW: Formal analysis, Writing – review & editing. XW: Data curation, Writing – review & editing. BT: Conceptualization, Formal analysis, Supervision, Writing – review & editing. ZC: Conceptualization, Software, Writing – review & editing. LL: Funding acquisition, Project administration, Resources, Writing – original draft, Writing – review & editing.
The author(s) declare that financial support was received for the research, authorship, and/or publication of this article. This work was financially supported by Special Key Project of Chongqing Technology Innovation and Application Development (cstc2021jscx-gksbX0004 and CSTB2023TIAD-LUX0003), Fundamental Research Funds for the Central Universities (SWU-KT22010), Chongqing Modern Agricultural Industry Technology System (Poultry) (CQMAITS202314), the Science and Technology Research Program of Chongqing Municipal Education Commission (KJQN202100220 and KJQN202200201), the Undergraduate Innovation and Entrepreneurship Training Program of Southwest University (202210635058).
The authors declare that the research was conducted in the absence of any commercial or financial relationships that could be construed as a potential conflict of interest.
All claims expressed in this article are solely those of the authors and do not necessarily represent those of their affiliated organizations, or those of the publisher, the editors and the reviewers. Any product that may be evaluated in this article, or claim that may be made by its manufacturer, is not guaranteed or endorsed by the publisher.
The Supplementary material for this article can be found online at: https://www.frontiersin.org/articles/10.3389/fvets.2024.1399776/full#supplementary-material
1. Yu, J, Lou, YP, and Zhao, A. Transcriptome analysis of follicles reveals the importance of autophagy and hormones in regulating broodiness of Zhedong white goose. Sci Rep. (2016) 6:36877. doi: 10.1038/srep36877
2. Kuo, YM, Shiue, YL, Chen, CF, Tang, PC, and Lee, YP. Proteomic analysis of hypothalamic proteins of high and low egg production strains of chickens. Theriogenology. (2005) 64:1490–502. doi: 10.1016/j.theriogenology.2005.03.020
3. Shen, X, Bai, X, Xu, J, Zhou, M, Xu, HP, Nie, QH, et al. Transcriptome sequencing reveals genetic mechanisms underlying the transition between the laying and brooding phases and gene expression changes associated with divergent reproductive phenotypes in chickens. Mol Biol Rep. (2016) 43:977–89. doi: 10.1007/s11033-016-4033-8
4. Liu, LB, Xiao, QH, Gilbert, ER, Cui, ZF, Zhao, XL, Wang, Y, et al. Whole-transcriptome analysis of atrophic ovaries in broody chickens reveals regulatory pathways associated with proliferation and apoptosis. Sci Rep. (2018) 8:7231:7231. doi: 10.1038/s41598-018-25103-6
5. Rincon-Riveros, A, Morales, D, Rodriguez, JA, Villegas, VE, and Lopez-Kleine, L. Bioinformatic tools for the analysis and prediction of ncRNA interactions. Int J Mol Sci. (2021) 22:11397. doi: 10.3390/ijms222111397
6. Park, YB, and Kim, JM. Identification of long non-coding RNA-mRNA interactions and genome-wide lncRNA annotation in animal transcriptome profiling. J Anim Sci Technol. (2023) 65:293–310. doi: 10.5187/jast.2023.e17
7. Djebali, S, Davis, CA, Merkel, A, Dobin, A, Lassmann, T, Mortazavi, A, et al. Landscape of transcription in human cells. Nature. (2012) 489:101–8. doi: 10.1038/nature11233
8. Sirotkin, AV, Kisova, G, Brenaut, P, Ovcharenko, D, Grossmann, R, and Mlyncek, M. Involvement of microRNA Mir15a in control of human ovarian granulosa cell proliferation, apoptosis, steroidogenesis, and response to FSH. Microrna. (2014) 3:29–36. doi: 10.2174/2211536603666140227232824
9. Hossain, MM, Cao, M, Wang, Q, Kim, JY, Schellander, K, Tesfaye, D, et al. Altered expression of miRNAs in a dihydrotestosterone-induced rat PCOS model. J Ovarian Res. (2013) 6:36. doi: 10.1186/1757-2215-6-36
10. Mao, H, Xu, X, Cao, H, Dong, X, Zou, X, Xu, N, et al. Comparative transcriptome profiling of mRNA and lncRNA of ovaries in high and low egg production performance in domestic pigeons (Columba livia). Front Genet. (2021) 12:571325. doi: 10.3389/fgene.2021.571325
11. Yu, T, Wang, Y, Fan, Y, Fang, N, Wang, T, Xu, T, et al. CircRNAs in cancer metabolism: a review. J Hematol Oncol. (2019) 12:90. doi: 10.1186/s13045-019-0776-8
12. Cai, HC, Li, YM, Li, HM, Niringiyumukiza, JD, Zhang, MD, Chen, L, et al. Identification and characterization of human ovary-derived circular RNAs and their potential roles in ovarian aging. Aging. (2018) 10:2511–34. doi: 10.18632/aging.101565
13. Xu, GX, Zhang, HF, Li, X, Hu, JH, Yang, GS, and Sun, SD. Genome-wide differential expression profiling of ovarian circRNAs associated with litter size in pigs. Front Genet. (2019) 10:1010. doi: 10.3389/fgene.2019.01010
14. Tao, H, Xiong, Q, Zhang, F, Zhang, N, Liu, Y, Suo, XJ, et al. Circular RNA profiling reveals chi_circ_0008219 function as microRNA sponges in pre-ovulatory ovarian follicles of goats (Capra hircus). Genomics. (2018) 110:257–66. doi: 10.1016/j.ygeno.2017.10.005
15. Chen, L, Zhang, T, Zhang, S, Huang, J, Zhang, G, Xie, K, et al. Identification of long non-coding RNA-associated competing endogenous RNA network in the differentiation of chicken preadipocytes. Genes. (2019) 10:795. doi: 10.3390/genes10100795
16. Langmead, B, and Salzberg, SL. Fast gapped-read alignment with Bowtie 2. Nat Methods. (2012) 9:357–9. doi: 10.1038/Nmeth.1923
17. Sun, L, Luo, H, Bu, D, Zhao, G, Yu, K, Zhang, C, et al. Utilizing sequence intrinsic composition to classify protein-coding and long non-coding transcripts. Nucleic Acids Res. (2013) 41:e166. doi: 10.1093/nar/gkt646
18. Kong, L, Zhang, Y, Ye, ZQ, Liu, XQ, Zhao, SQ, Wei, L, et al. CPC: assess the protein-coding potential of transcripts using sequence features and support vector machine. Nucleic Acids Res. (2007) 35:W345–9. doi: 10.1093/nar/gkm391
19. Wucher, V, Legeai, F, Hedan, B, Rizk, G, Lagoutte, L, Leeb, T, et al. FEELnc: a tool for long non-coding RNA annotation and its application to the dog transcriptome. Nucleic Acids Res. (2017) 45:e57. doi: 10.1093/nar/gkw1306
20. Kim, D, Landmead, B, and Salzberg, SL. HISAT: a fast spliced aligner with low memory requirements. Nat Methods. (2015) 12:357–60. doi: 10.1038/Nmeth.3317
21. Memczak, S, Jens, M, Elefsinioti, A, Torti, F, Krueger, J, Rybak, A, et al. Circular RNAs are a large class of animal RNAs with regulatory potency. Nature. (2013) 495:333–8. doi: 10.1038/nature11928
22. Liu, LB, Yi, JQ, Ray, WK, Vu, LT, Helm, RF, Siegel, PB, et al. Fasting differentially alters the hypothalamic proteome of chickens from lines with the propensity to be anorexic or obese. Nutr Diabetes. (2019) 9:13. doi: 10.1038/s41387-019-0081-1
23. Shi, JA, Li, WD, Liu, AF, Ren, LT, Zhang, PS, Jiang, T, et al. MiRNA sequencing of embryonic myogenesis in Chengkou mountain chicken. BMC Genomics. (2022) 23:571. doi: 10.1186/s12864-022-08795-z
24. Ren, L, Liu, A, Wang, Q, Wang, H, Dong, D, and Liu, L. Transcriptome analysis of embryonic muscle development in Chengkou mountain chicken. BMC Genomics. (2021) 22:431. doi: 10.1186/s12864-021-07740-w
25. Livak, KJ, and Schmittgen, TD. Analysis of relative gene expression data using real-time quantitative PCR and the 2−ΔΔCT method. Methods. (2001) 25:402–8. doi: 10.1006/meth.2001.1262
26. Chen, SF, Zhou, YQ, Chen, YR, and Gu, J. Fastp: an ultra-fast all-in-one FASTQ preprocessor. Bioinformatics. (2018) 34:i884–90. doi: 10.1093/bioinformatics/bty560
27. Braga, EA, Fridman, MV, Moscovtsev, AA, Filippova, EA, Dmitriev, AA, and Kushlinskii, NE. LncRNAs in ovarian cancer progression, metastasis, and main pathways: ceRNA and alternative mechanisms. Int J Mol Sci. (2020) 21:8855. doi: 10.3390/ijms21228855
28. Wei, QY, Li, J, He, HR, Cao, YC, Li, DM, Amevor, FK, et al. miR-23b-3p inhibits chicken granulosa cell proliferation and steroid hormone synthesis via targeting GDF9. Theriogenology. (2022) 177:84–93. doi: 10.1016/j.theriogenology.2021.10.011
29. Sharp, PJ, Dawson, A, and Lea, RW. Control of luteinizing hormone and prolactin secretion in birds. Comp Biochem Physiol C Pharmacol Toxicol Endocrinol. (1998) 119:275–82. doi: 10.1016/s0742-8413(98)00016-4
30. Shimmura, T, Maruyama, Y, Fujino, S, Kamimura, E, Uetake, K, and Tanaka, T. Persistent effect of broody hens on behaviour of chickens. Anim Sci J. (2015) 86:214–20. doi: 10.1111/asj.12253
31. Huang, YM, Shi, ZD, Liu, Z, Liu, Y, and Li, XW. Endocrine regulations of reproductive seasonality, follicular development and incubation in Magang geese. Anim Reprod Sci. (2008) 104:344–58. doi: 10.1016/j.anireprosci.2007.02.005
32. He, HR, Li, DM, Tian, YT, Wei, QY, Amevor, FK, Sun, CJ, et al. miRNA sequencing analysis of healthy and atretic follicles of chickens revealed that miR-30a-5p inhibits granulosa cell death via targeting Beclin1. J Anim Sci Biotechnol. (2022) 13:55. doi: 10.1186/s40104-022-00697-0
33. Liu, HH, Wang, JW, Li, L, Han, CC, He, H, and Xu, HY. Transcriptome analysis revealed the possible regulatory pathways initiating female geese broodiness within the hypothalamic-pituitary-gonadal axis. PLoS One. (2018) 13:e0191213. doi: 10.1371/journal.pone.0191213
34. Shuyuan, Y, Meimei, W, Fenghua, L, Huishan, Z, Min, C, Hongchu, B, et al. hUMSC transplantation restores follicle development in ovary damaged mice via re-establish extracellular matrix (ECM) components. J Ovarian Res. (2023) 16:172. doi: 10.1186/s13048-023-01217-y
35. Sreerangaraja Urs, DB, Wu, WH, Komrskova, K, Postlerova, P, Lin, YF, Tzeng, CR, et al. Mitochondrial function in modulating human granulosa cell steroidogenesis and female fertility. Int J Mol Sci. (2020) 21:3592. doi: 10.3390/ijms21103592
36. Salmena, L, Poliseno, L, Tay, Y, Kats, L, and Pandolfi, PP. A ceRNA hypothesis: the Rosetta Stone of a hidden RNA language? Cell. (2011) 146:353–8. doi: 10.1016/j.cell.2011.07.014
37. Cao, JP, Huo, P, Cui, KQ, Wei, HM, Cao, JN, Wang, JY, et al. Correction: follicular fluid-derived exosomal miR-143-3p/miR-155-5p regulate follicular dysplasia by modulating glycolysis in granulosa cells in polycystic ovary syndrome. Cell Commun Signal. (2022) 20:61. doi: 10.1186/s12964-022-00938-9
38. Roth, LW, McCallie, B, Alvero, R, Schoolcraft, WB, Minjarez, D, and Katz-Jaffe, MG. Altered microRNA and gene expression in the follicular fluid of women with polycystic ovary syndrome. J Assist Reprod Genet. (2014) 31:355–62. doi: 10.1007/s10815-013-0161-4
39. Stubbs, SA, Stark, J, Dilworth, SM, Franks, S, and Hardy, K. Abnormal preantral folliculogenesis in polycystic ovaries is associated with increased granulosa cell division. J Clin Endocrinol Metab. (2007) 92:4418–26. doi: 10.1210/jc.2007-0729
40. Kong, FJ, Du, CX, and Wang, Y. MicroRNA-9 affects isolated ovarian granulosa cells proliferation and apoptosis via targeting vitamin D receptor. Mol Cell Endocrinol. (2019) 486:18–24. doi: 10.1016/j.mce.2019.02.012
41. Anand, AAP, Huber, C, Mary, JA, Gallus, N, Leucht, C, Klafke, R, et al. Expression and function of microRNA-9 in the mid-hindbrain area of embryonic chick. BMC Dev Biol. (2018) 18:3. doi: 10.1186/s12861-017-0159-8
42. Tao, WJ, Sun, LN, Shi, HJ, Cheng, YY, Jiang, DN, Fu, BD, et al. Integrated analysis of miRNA and mRNA expression profiles in tilapia gonads at an early stage of sex differentiation. BMC Genomics. (2016) 17:328. doi: 10.1186/s12864-016-2636-z
43. Li, H, Zhu, G, Xing, Y, Zhu, Y, and Piao, D. RETRACTION NOTICE: miR-4324 functions as a tumor suppressor in colorectal cancer by targeting HOXB2. J Int Med Res. (2021) 49:03000605211041196. doi: 10.1177/03000605211041196
44. Wu, HX, Yan, YL, Yuan, JL, Luo, MZ, and Wang, YJ. miR-4324 inhibits ovarian cancer progression by targeting FEN1. J Ovarian Res. (2022) 15:32. doi: 10.1186/s13048-022-00959-5
45. Muralimanoharan, S, Maloyan, A, and Myatt, L. Mitochondrial function and glucose metabolism in the placenta with gestational diabetes mellitus: role of miR-143. Clin Sci. (2016) 130:931–41. doi: 10.1042/Cs20160076
46. Yang, DQ, Liu, AI, Zhang, YY, Nan, S, Yin, RL, Lei, QH, et al. Essential role of CRIM1 on endometrial receptivity in goat. Int J Mol Sci. (2021) 22:5323. doi: 10.3390/ijms22105323
47. Tang, D, Geng, L, and Ma, J. lncRNA PROX1-AS1 mediates the migration and invasion of placental trophoblast cells via the miR-211-5p/caspase-9 axis. Bioengineered. (2021) 12:4100–10. doi: 10.1080/21655979.2021.1953213
48. Ran, MX, Ouyang, QY, Li, XJ, Hu, SQ, Hu, B, Hu, JW, et al. Exploring right ovary degeneration in duck and goose embryos by histology and transcriptome dynamics analysis. BMC Genomics. (2023) 24:389. doi: 10.1186/s12864-023-09493-0
49. Ny, T, Wahlberg, P, and Brandstrom, IJ. Matrix remodeling in the ovary: regulation and functional role of the plasminogen activator and matrix metalloproteinase systems. Mol Cell Endocrinol. (2002) 187:29–38. doi: 10.1016/s0303-7207(01)00711-0
50. Hrabia, A, Socha, JK, and Sechman, A. Involvement of matrix metalloproteinases (MMP-2, -7, -9) and their tissue inhibitors (TIMP-2, -3) in the regression of chicken postovulatory follicles. Gen Comp Endocrinol. (2018) 260:32–40. doi: 10.1016/j.ygcen.2018.02.008
51. Lemanska-Perek, A, and Adamik, B. Fibronectin and its soluble EDA-FN isoform as biomarkers for inflammation and sepsis. Adv Clin Exp Med. (2019) 28:1561–7. doi: 10.17219/acem/104531
52. Bello, SF, Xu, H, Guo, L, Li, K, Zheng, M, Xu, Y, et al. Hypothalamic and ovarian transcriptome profiling reveals potential candidate genes in low and high egg production of white Muscovy ducks (Cairina moschata). Poult Sci. (2021) 100:101310. doi: 10.1016/j.psj.2021.101310
53. Petit, V, and Thiery, JP. Focal adhesions: structure and dynamics. Biol Cell. (2000) 92:477–94. doi: 10.1016/s0248-4900(00)01101-1
54. Wu, X, Jiang, L, Xu, F, Cao, S, Chen, Y, Zhang, Y, et al. Long noncoding RNAs profiling in ovary during laying and nesting in Muscovy ducks (Cairina moschata). Anim Reprod Sci. (2021) 230:106762. doi: 10.1016/j.anireprosci.2021.106762
55. Li, Q, Li, J, Li, C, Wu, X, Si, SJ, Yang, PK, et al. Transcriptome identification and characterization of long non-coding RNAs in the ovary of hens at four stages. Anim Biotechnol. (2023) 34:1342–53. doi: 10.1080/10495398.2021.2024217
56. Zhang, T, Chen, L, Han, KP, Zhang, XQ, Zhang, GX, Dai, GJ, et al. Transcriptome analysis of ovary in relatively greater and lesser egg producing Jinghai yellow chicken. Anim Reprod Sci. (2019) 208:106114. doi: 10.1016/j.anireprosci.2019.106114
57. Garside, SA, Henkin, J, Morris, KD, Norvell, SM, Thomas, FH, and Fraser, HM. A thrombospondin-mimetic peptide, ABT-898, suppresses angiogenesis and promotes follicular atresia in pre- and early-antral follicles in vivo. Endocrinology. (2010) 151:5905–15. doi: 10.1210/en.2010-0283
Keywords: broodiness, ovarian development, whole transcriptome analysis, ncRNAs, ceRNA network
Citation: Xiong H, Li W, Wang L, Wang X, Tang B, Cui Z and Liu L (2024) Whole transcriptome analysis revealed the regulatory network and related pathways of non-coding RNA regulating ovarian atrophy in broody hens. Front. Vet. Sci. 11:1399776. doi: 10.3389/fvets.2024.1399776
Received: 12 March 2024; Accepted: 08 May 2024;
Published: 29 May 2024.
Edited by:
Miguel A. Gutiérrez Reinoso, Universidad Técnica de Cotopaxi, EcuadorReviewed by:
Zhuanjian Li, Henan Agricultural University, ChinaCopyright © 2024 Xiong, Li, Wang, Wang, Tang, Cui and Liu. This is an open-access article distributed under the terms of the Creative Commons Attribution License (CC BY). The use, distribution or reproduction in other forums is permitted, provided the original author(s) and the copyright owner(s) are credited and that the original publication in this journal is cited, in accordance with accepted academic practice. No use, distribution or reproduction is permitted which does not comply with these terms.
*Correspondence: Lingbin Liu, bGl1bGI1MTVAMTYzLmNvbQ==
Disclaimer: All claims expressed in this article are solely those of the authors and do not necessarily represent those of their affiliated organizations, or those of the publisher, the editors and the reviewers. Any product that may be evaluated in this article or claim that may be made by its manufacturer is not guaranteed or endorsed by the publisher.
Research integrity at Frontiers
Learn more about the work of our research integrity team to safeguard the quality of each article we publish.