- 1Department of Computer Science, Colgate University, Hamilton, NY, United States
- 2Department of Mathematics, Colgate University, Hamilton, NY, United States
- 3Department of Biology, Colgate University, Hamilton, NY, United States
The composition of the microbiome influences many aspects of physiology and health, and can be altered by environmental factors, including diet and activity. Glucosamine is a dietary supplement often administered to address arthritic symptoms in humans, dogs, and other mammals. To investigate how gut microbial composition varies with glucosamine supplementation, we performed 16S rRNA sequence analysis of fecal samples from 24 Alaskan and Inuit huskies and used mixed effects models to investigate associations with activity, age, and additional factors. Glucosamine ingestion, age, activity, sex, and diet were correlated with differences in alpha-diversity, with diversity decreasing in dogs consuming glucosamine. Beta-diversity analysis revealed clustering of dogs based on glucosamine supplementation status. Glucosamine supplementation and exercise-related activity were associated with greater inter-individual pairwise distances. At the family level, Lactobacillaceae and Anaerovoracaceae relative abundances were lower in supplemented dogs when activity was accounted for. At the genus level, Eubacterium [brachy], Sellimonus, Parvibacter, and an unclassified genus belonging to the same family as Parvibacter (Eggerthellaceae) all were lower in supplemented dogs, but only significantly so post-activity. Our findings suggest that glucosamine supplementation alters microbiome composition in sled dogs, particularly in the context of exercise-related activity.
Introduction
More than 30 trillion microorganisms inhabit the human body (1). A significant portion of these microorganisms reside in the digestive tract, collectively containing more than 3 million genes that comprise the gut microbiome (2), which is the largest and most diverse microbiome in the human body (3). In its coevolution with humans, the functions of the microbiome have become intertwined with human physiology (4, 5). As such, the gut microbiota has been implicated in important physiological functions in the human body, including but not limited to gut barrier stabilization, development of host immune response, endocrine function, and neurological signaling and development (6–9). As a result, the gut microbiome plays an important role in health and disease (10), emerging as an intriguing area of study.
Animal models have proven useful for understanding factors that influence and are influenced by the gut microbiome (11). For example, murine models have been utilized to establish causal links between the gut microbiome and disease (12, 13). Despite this promise, our ability to extrapolate findings from mice and other rodents is limited since only 4% of microbial identity is shared between mice and humans (14). Additionally, evolution has facilitated a notable divide between the biological processes of humans and mice, which further limits the utility of studying the gut microbiota of mice to inform our understanding of human processes (15).
Given the co-evolution between humans and dogs, canine models have pertinent applications for the study of gut microbiota. Dogs share a similar diet to humans, possessing omnivorous metabolism and being able to digest, absorb, and metabolize dietary carbohydrates (16, 17). Moreover, dogs, in contrast to mice, share similar microbiome composition to humans as they have historically shared a similar environment and lifestyle (18). Working sled dogs are frequently used as models for understanding the effects of exercise on physiology and health (19). Sled dogs and other intensely exercising dogs frequently experience exercise-induced gastric disease (EIGD), characterized by gastric lesions and increased intestinal permeability (20–22). Recent work has provided evidence that exercise in racing sled dogs alters the microbiome, resulting in an increase in beneficial microbes and a concomitant decrease in dysbiosis-associated bacteria and supplementation of sled dogs with a targeted symbiotic altered the microbiome and decreased diarrhea, potentially due to a transient increase in Lactobacillaceae and Streptococcaceae and decreases in Clostridiaceae (23, 24). However, the ability of dietary supplements to alter the microbiome is unclear.
In the United States, inflammatory arthritis is a leading cause of disability among adults (25, 26) and dogs (27, 28). Two of the most commonly taken supplements for joint inflammation in humans and dogs are the sulfated carbohydrates chondroitin and glucosamine (29–31). Despite their long history as a treatment for joint pain (32), there is controversy regarding the benefits of glucosamine and chondroitin for joint health, leading some experts to recommend these supplements as an adjunctive treatment (33, 34), and some experts not to recommend supplementation at all (35, 36) based on evidence that glucosamine and chondroitin do not have beneficial effects (37, 38) or may be detrimental (39). Overall, glucosamine and chondroitin exhibit poor absorption in the mammalian intestine (40, 41) and are largely consumed by the gut microflora before they can be absorbed (41). These findings have led to speculation that glucosamine and chondroitin may be indirectly involved in the production of anti-inflammatory compounds through gut bacterial pathways (42, 43). While few studies have examined the exclusive effects of glucosamine and/or chondroitin supplementation on the gut microflora in any mammal, two studies in humans have suggested that glucosamine intake has effects on the relative abundance of specific microbial taxa (44, 45).
Given the intriguing evidence that glucosamine supplementation may affect joint inflammation through the gut microbiome, we sought to examine the effects of glucosamine supplementation on gut microbial composition of sled dogs in this study. We also considered age, sex, breed, diet, health status, and exercise-related activity as potential influences on fecal microbiome composition, since all these factors have previously been suggested to affect the gut microbiome in dogs (46–50). In this retrospective study, we investigate the links between microbiome composition, glucosamine supplementation, and physical activity.
Methods
Data collection and processing
Twenty-four adult sled dogs (11 males; 13 females) aged between 2 and 12 years old were examined in this study. One pre-activity fecal sample was collected from each dog on January 27, 2022, and a post-activity sample was collected on January 31, 2022. Samples were shipped on dry ice and stored frozen at -20°C. Between sample collections, dogs were run 2–3 times per day in teams of four (or six for dogs 10 years old and older) for 3–7 miles per run. We refer to this higher than normal exercise for the sprint sled dogs as “activity.” Variables used in the multivariate and mixed methods analyses were: age in years, sex, breed (pure-bred Inuit husky versus mixed-breed Alaskan husky), weight, diet (Annamaet Plus kibble versus Annamaet Option canned), supplemental diet (eggs, liver, and/or Caribou Creek supplement), history of recent injury or illness, and glucosamine supplementation. For this retrospective study, glucosamine supplementation includes daily intake of either a commercial glucosamine supplement with ascorbic acid or glucosamine as a component of cosequin. Information was also collected on deworming and anti-inflammatory drug intake but not included in analyses as all dogs had recently been treated with Ivermectin and only two dogs received carprofen as an anti-inflammatory.
DNA was extracted using the DNeasy PowerSoil Pro kit (Qiagen Cat# 470141) according to manufacturer’s instructions using up to 250 mg of fecal sample per extraction. Homogenization was performed using two 30-s pulses on a Beadbeater (BioSpec, Inc.) at 4°C. Microbial DNA extraction was confirmed by PCR amplification of the V4 variable region of 16S rDNA using primers 515F-Y (51) and 806R (52) according to Earth Microbiome Project (EMP) protocols (53).2 DNA sequencing of the 16S V4 variable region was performed by the Cornell University Biotechnology Resource Center using Illumina MiSeq and 515F-Y and 806R primers. 16S rDNA concentration after initial amplification and the number of sequencing reads in each sample are provided in Supplementary Table S1. Indexing was performed by diluting each PCR product to 2 ng/ul and using 10 ng per indexing reaction with a 5 uM concentration of i7 and i5 indexing primers (Illumina, Inc. San Diego, CA, United States).
Qiime 2 version 2022.11 was used to process the sequence reads (54). After demultiplexing, Dada2 was used to trim and denoise the sequences (55). We then obtained the feature table and representative sequences. Taxonomy was assigned using the SILVA 16S rRNA database (56). Rarefaction was done with a sampling depth of 58,667 based on the minimum read length.
Alpha diversity analysis
Linear mixed effects models were used to investigate the relationship between selected factors and sample alpha diversity. Age, sex, breed, diet, history of illness or injury, pre- or post-activity, and glucosamine supplementation were selected as variables of interest. Alpha diversity for each sample was calculated using Shannon’s Diversity Index (57) and Faith’s Phylogenetic Diversity (58). The variables of interest were set as independent variables and the alpha diversity metric as the response variable in our models to test for significant associations.
After applying linear mixed effects models on all 48 data points, the samples were split into subgroups depending on each dog’s activity level and glucosamine treatment, and alpha-diversity calculations using Shannon’s Diversity Index and Faith’s Phylogenetic Diversity were repeated to test for subgroup associations. For the pre-activity (Pre-Ac) and post-activity (Post-Ac) subgroups, linear regression models were used. For yes-glucosamine (YG) and no-glucosamine (NG) subgroups, linear mixed effects models for repeated measures were used since each dog had both a Pre-Ac and a Post-Ac sample. For the latter two subgroups diet, breed, and injury variables were eliminated due to lack of variance. Type III F-tests were then performed in all linear models to test for significance.
Beta diversity analysis
Beta diversity was computed using the Bray-Curtis distance metric to investigate across-sample microbial diversity (59). Microbial differences between samples were visualized in principal coordinate analysis (PCoA) using the QIIME 2 plugin Emperor (60). We then applied statistical tests to analyze beta diversity differences. For each dog, the Bray-Curtis distance was computed from its pre-activity to post-activity sample. The Wilcoxon rank sum test (61) was employed to test for differences between these distances based on glucosamine groups.
In addition to variation within the same subject over time, microbial diversity was investigated between subjects. Samples were partitioned into groups based on date of sample collection into pre-activity (Pre-Ac) and post-activity (Post-Ac) groups for which glucosamine was the variable of interest. Samples were also separated into yes-glucosamine (YG) and no-glucosamine (NG) groups. For the YG and NG groups, activity was the variable of interest. Pairwise Bray-Curtis distance was calculated in each group. Based on the type of pairs (pre-post, pre-pre, post-post or YG-NG, YG-YG, NG-NG), the Kruskal-Wallis test (62) and pairwise Wilcoxon rank sum test (61) were applied for comparison.
For both beta diversity analyses, the distances were plotted in box plots grouped by the variable of interest using ggplot2 (63) in R (64).
Differential abundance
Differential abundance testing was carried out on the feature table collapsed on the family and genus levels. Taxonomic bar plots of relative abundances of prevalent families and genera were first generated in QIIME 2 to visualize the microbial compositions and identify important taxa. Samples were then separated by both activity and glucosamine into four subgroups (Pre-Ac/YG; Pre-Ac/NG; Post-Ac/YG; Post-Ac/NG). For each pair of subgroups, Analysis of Compositions of Microbiomes (ANCOM) was applied to identify which taxa are differentially abundant on both the family and genus levels (65). For the identified differential taxa, relative abundance levels in the four subgroups were plotted in box plots using ggplot2 (63) in R (66).
Results
16S rDNA amplification and sequencing
To investigate the presence and relative abundance of microbial taxa present in fecal samples from each sled dog before and after the defined period of activity, we isolated DNA from each sample, amplified the V4 region of rDNA, and performed DNA sequencing using Illumina MiSeq. We obtained 8,408,194 high-quality 16S rRNA V4 region sequences over the 48 samples sequenced, providing an average of 175,170.7 (±19,264.7 S.D.) reads per sample. The minimum trimmed sequence length used in analysis was 251 nucleotides.
Alpha-diversity comparison with demographic factors
To investigate factors influencing gut microbiota diversity in this cohort of sled dogs, we examined the linear mixed effects of age, breed, diet, activity, glucosamine supplementation, injuries, and sex, on bacterial alpha-diversity using Shannon’s diversity index and Faith’s phylogenetic diversity index (Table 1). We found that age (Shannon p = 0.012, Faith p = 0.071) and dietary glucosamine supplementation (Shannon p < 0.001, Faith p = 0.005) correlate with variation in alpha-diversity. None of the other variables tested were associated with changes in alpha-diversity metrics. To examine the pattern of alpha-diversity changes across this cohort of dogs, we plotted the Shannon’s diversity value for each dog against age (Figure 1A) and observed that increased age does not correlate with greater microbial diversity by this measure (p = 0.575, marginal r2 = 0.010). We also examined the variation in alpha-diversity by pooling dogs given daily glucosamine supplements and those not receiving glucosamine supplementation. We observed a significant decrease in diversity in the glucosamine-supplemented group (Figure 1B; p = 0.004). A re-analysis of the data excluding Sami and Kesha, who upon visual analysis appear to be outliers, retained a significant difference in alpha-diversity associated with glucosamine and age (Supplementary Table S2) indicating that the influences of glucosamine and age on alpha-diversity are not simply due to the oversized effects of these two dogs in the small glucosamine-positive dataset.
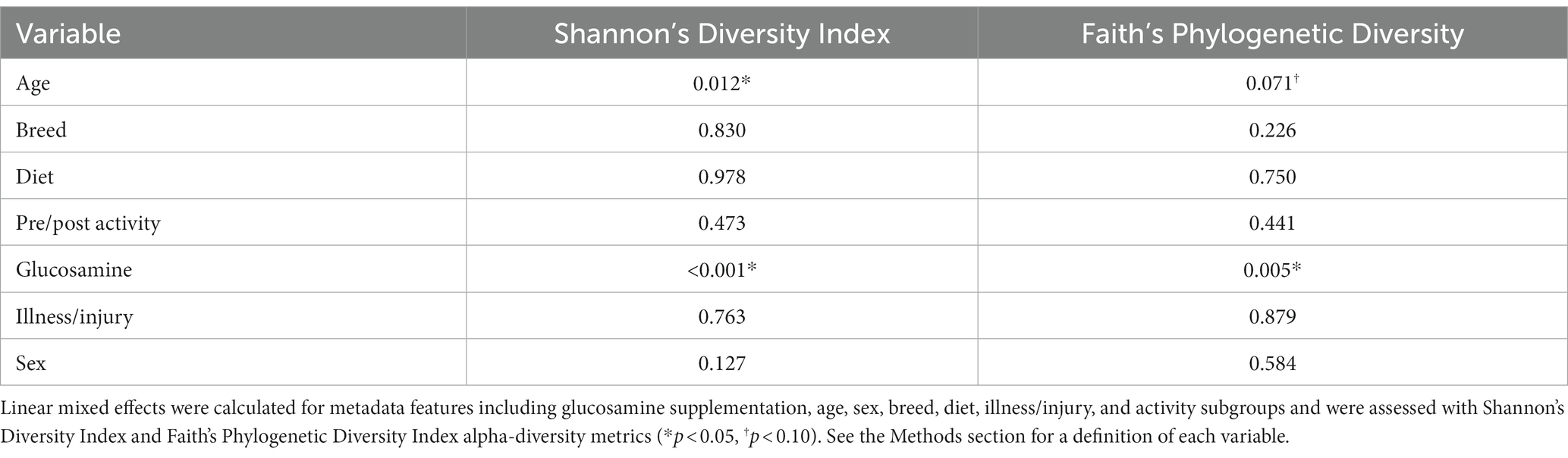
Table 1. Linear mixed effects analysis reveals differences in alpha-diversity associated with glucosamine supplementation and age.
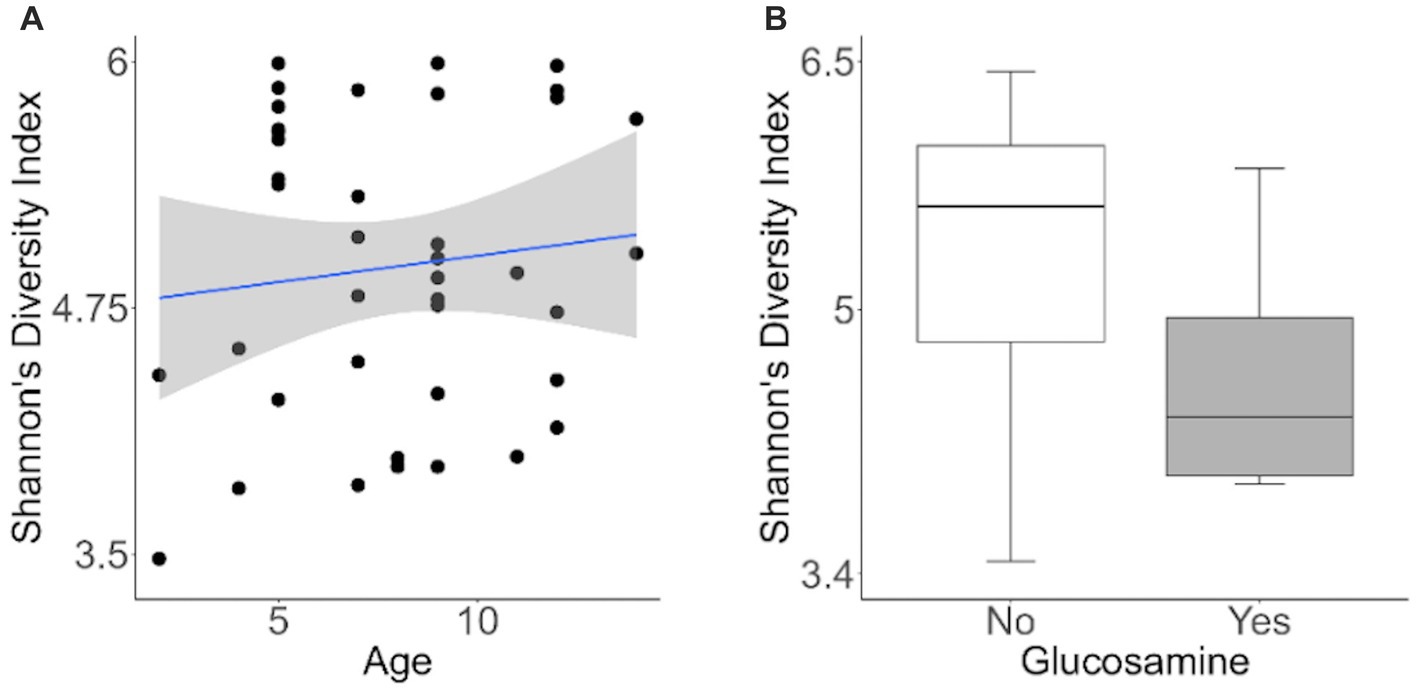
Figure 1. Alpha-diversity decreases with glucosamine supplementation. (A) Alpha-diversity for all dogs was assessed using Shannon’s Diversity index, plotted against age, and significance was calculated by univariate linear regression (p=0.575). The blue line indicates the regression against the mean (r2 = 0.010) and shaded gray area indicates the 95% confidence interval. (B) Shannon’s Diversity index was calculated for dogs not receiving glucosamine supplements (No) or receiving daily glucosamine (Yes) and significance was calculated using the Kruskal Wallis test (p=0.004). Box plots show the median, and first and third quartiles ±1.5 interquartile range (IQR).
To investigate connections between the tested variables, glucosamine supplementation, and gut microbial diversity, we divided the dataset into glucosamine-supplemented versus no glucosamine subgroups and repeated the linear mixed effects analysis of alpha-diversity metrics (Table 2). We observed significant age-related effects on diversity in dogs not supplemented with glucosamine, as well as a slightly significant effect of sex on Shannon’s diversity. In the smaller group of glucosamine-treated dogs (n = 6), we observed significant effects for diet, activity, and sex by at least one measure. To examine these relationships between glucosamine supplementation and microbial diversity more carefully, we plotted alpha-diversity against each variable for cohorts of dogs that were either receiving glucosamine supplements or not glucosamine. We observed a positive relationship between age and alpha diversity in both the absence (p = 0.025, r2 = 0.181; Figure 2A) and presence (p = 0.002, r2 = 0.517; Figure 2B) of glucosamine. In glucosamine-supplemented dogs, we note a trend toward greater alpha-diversity in the gut microbiome in females than males, although this difference was not significant (p = 0.055; Figure 2D). In glucosamine-supplemented dogs we observed an increase in diversity in those dogs on a diet of Annamaet salmon option relative to those consuming Annamaet Extra (p = 0.025; Figure 2E) but not a significant change in alpha-diversity from pre-activity to post-activity fecal sample collections (p = 0.262; Figure 2F).
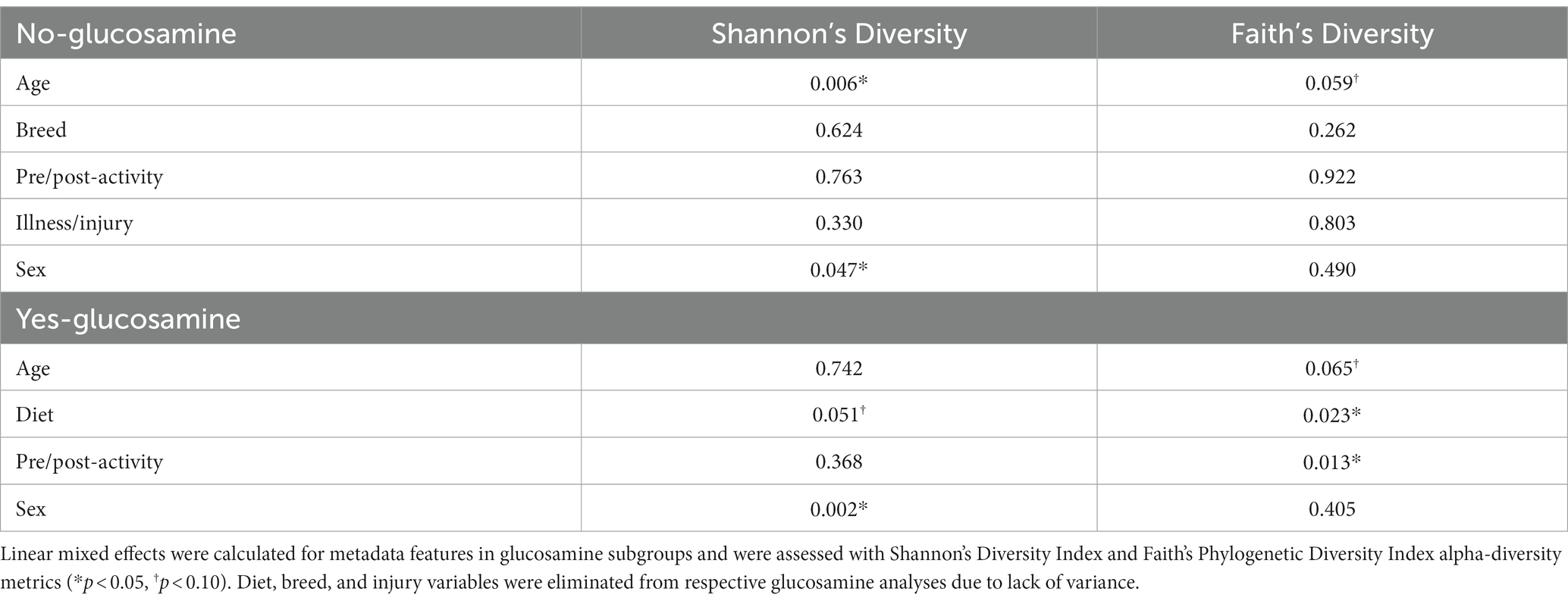
Table 2. Linear mixed effects analyses of dogs lacking or receiving glucosamine supplementation reveal significant differences in alpha-diversity for age, sex, diet, and activity.
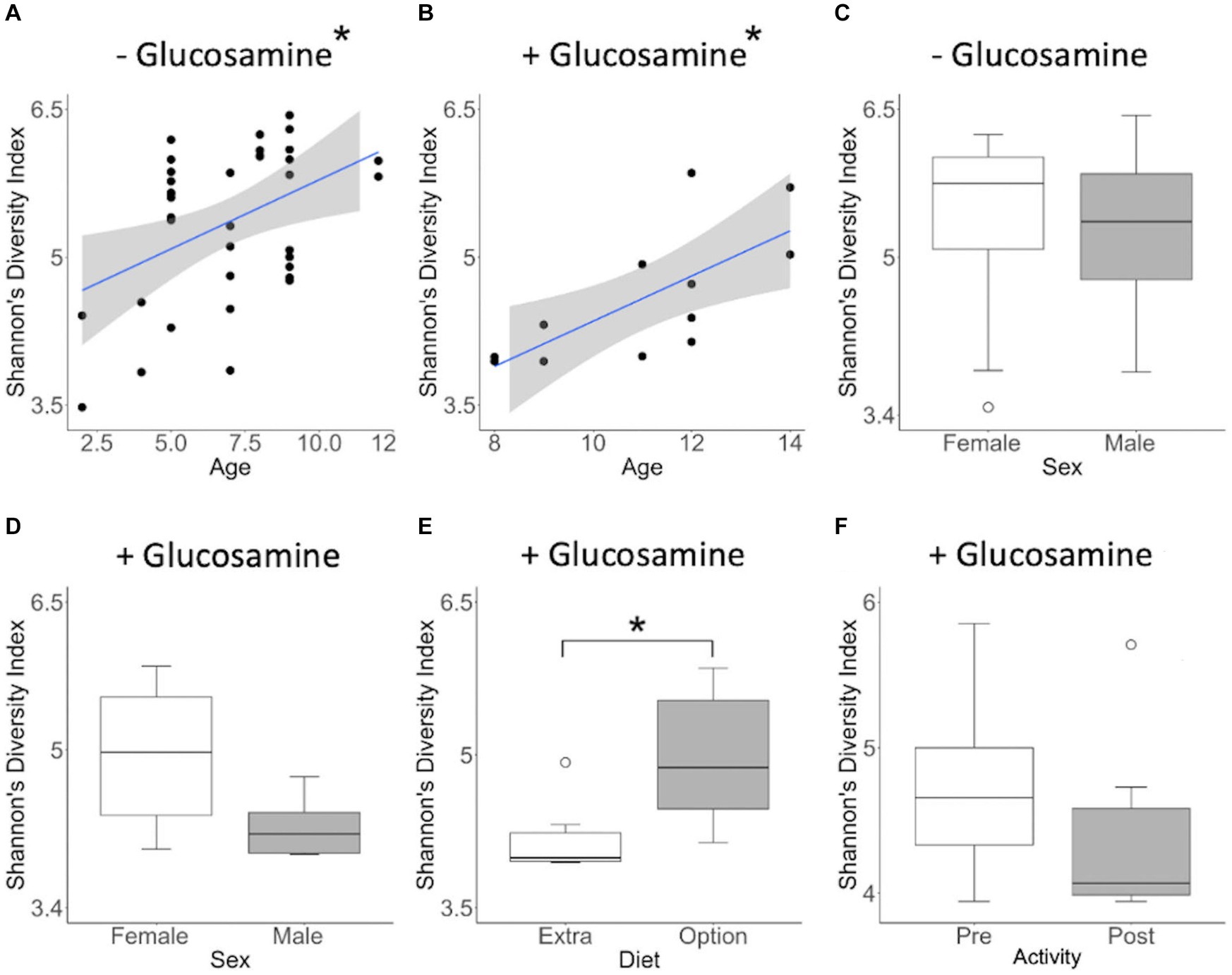
Figure 2. Alpha diversity of gut microbiota varies with age, sex, diet, and activity in the context of glucosamine supplementation. Plots for Shannon’s Diversity index were created for metadata features that were significant in the linear mixed effects analysis for glucosamine subgroups. (A,B) Alpha-diversity plotted against age in dogs not receiving glucosamine (A) and glucosamine supplemented (B). (C,D) Alpha-diversity comparisons by sex in dogs not taking glucosamine (C) or receiving glucosamine supplementation (D). (E) Alpha-diversity of glucosamine-supplemented dogs on Annamaet Extra and Annamaet Option diets. (F) Alpha-diversity pre- and post-activity in dogs receiving glucosamine. Significance was calculated using univariate regression for age and the Kruskal-Wallis test for all other factors (*p < 0.05).
Because of the differences in alpha-diversity observed before and after activity in glucosamine-supplemented dogs, we further examined the relationship between activity, microbiome composition, and the variables examined, again using linear mixed effects analysis (Table 3). We observed that age correlates with alpha-diversity changes in samples collected post-activity and glucosamine supplementation impacts the gut microbiome in both pre- and post-activity samples. We do not observe an increase in Shannon’s index with increasing age in fecal samples taken from dogs post-activity (p = 0.734, r2 = 0.005; Figure 3A), but do detect lower alpha-diversity in glucosamine-supplemented dogs after activity (p = 0.028; Figure 3C).
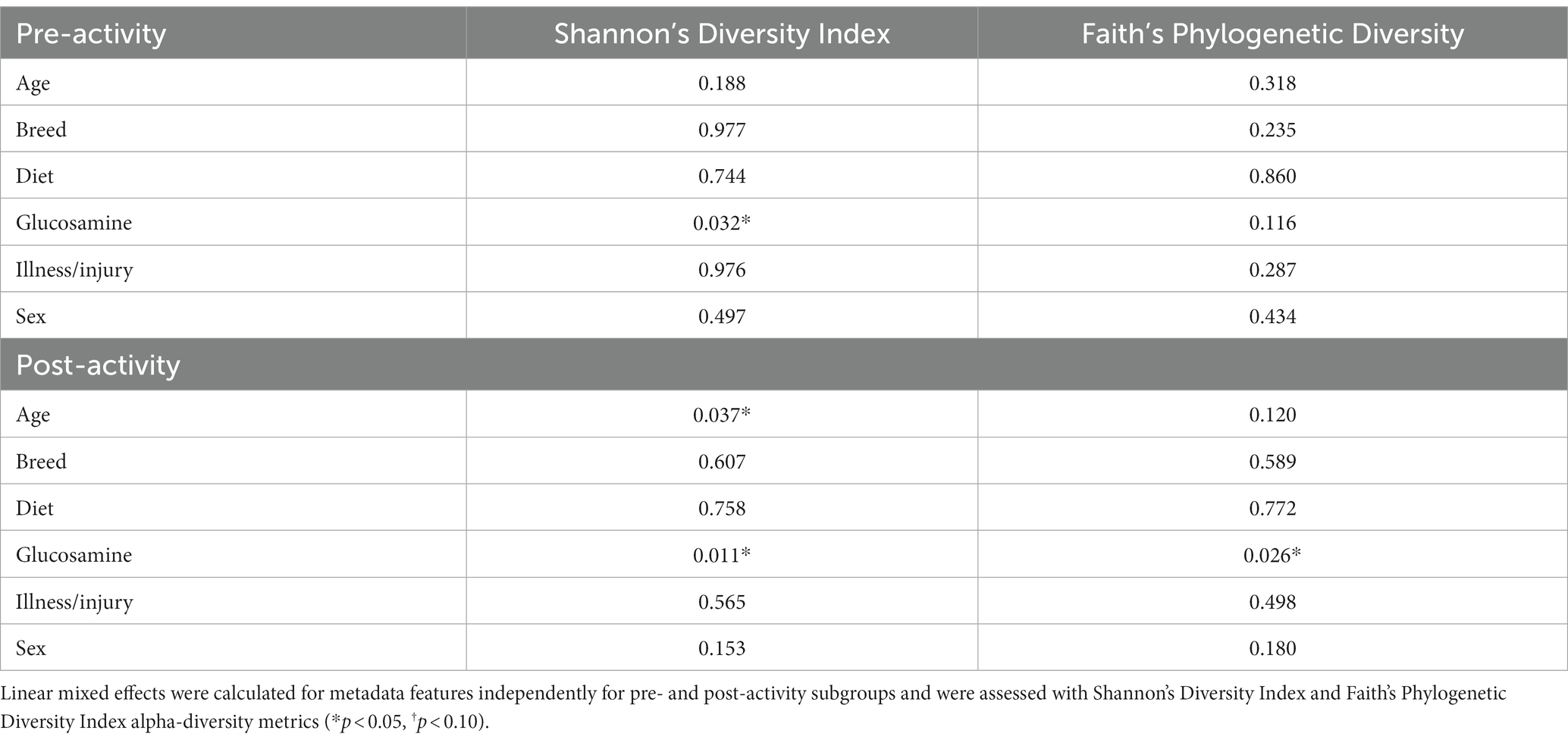
Table 3. Glucosamine supplementation is a significant contributor to alpha-diversity both before and after activity.
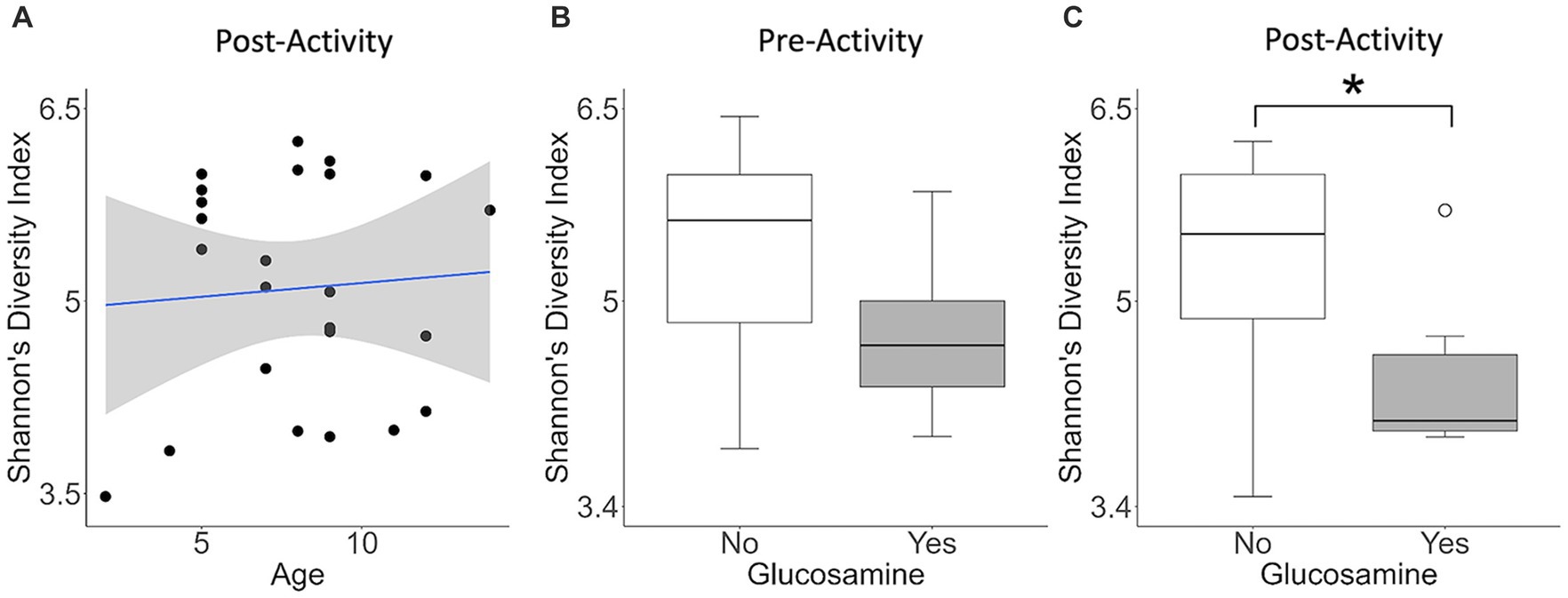
Figure 3. Alpha diversity is lower in glucosamine-supplemented dogs post-activity. Plots for Shannon’s Diversity index were created for metadata features that were significant in the linear mixed effects analysis for activity subgroups (Table 3). Significance was calculated using univariate linear regression for age and the Kruskal-Wallis test for glucosamine supplementation. (A) Shannon’s Diversity versus age in dogs post-activity. (B,C) Median Shannon’s Diversity comparing dogs receiving glucosamine (Yes) and non-supplemented dogs (No) for pre-activity (B) and post-activity (C) samples (*p < 0.05).
Beta-diversity comparison in glucosamine and activity subgroups
To further investigate which variables correlate with variation in the overall composition of the gut microbiome in this cohort of dogs, we used principal coordinates analysis (PCoA) to visualize differences in Bray-Curtis beta-diversity distance among pre- and post-activity dogs (Figure 4). We observed clustering of the glucosamine-supplemented dogs in both the pre-activity and post-activity analyses. We observed no variation when we compared self-pairwise beta-diversity distances before and after activity between dogs based on glucosamine supplementation status (p = 0.255; Figure 5). Because Sami (11 y.o.) and Kesha (12 y.o.) appeared to be potential outliers in both pre- and post-activity PCoAs (Figure 4), we also performed pairwise analyses without the inclusion of these dogs. We again observed no variation in self-pairwise distance between glucosamine treated or untreated dogs when Sami and Kesha were not included in the analysis (p = 0.993; Supplementary Figure S1).
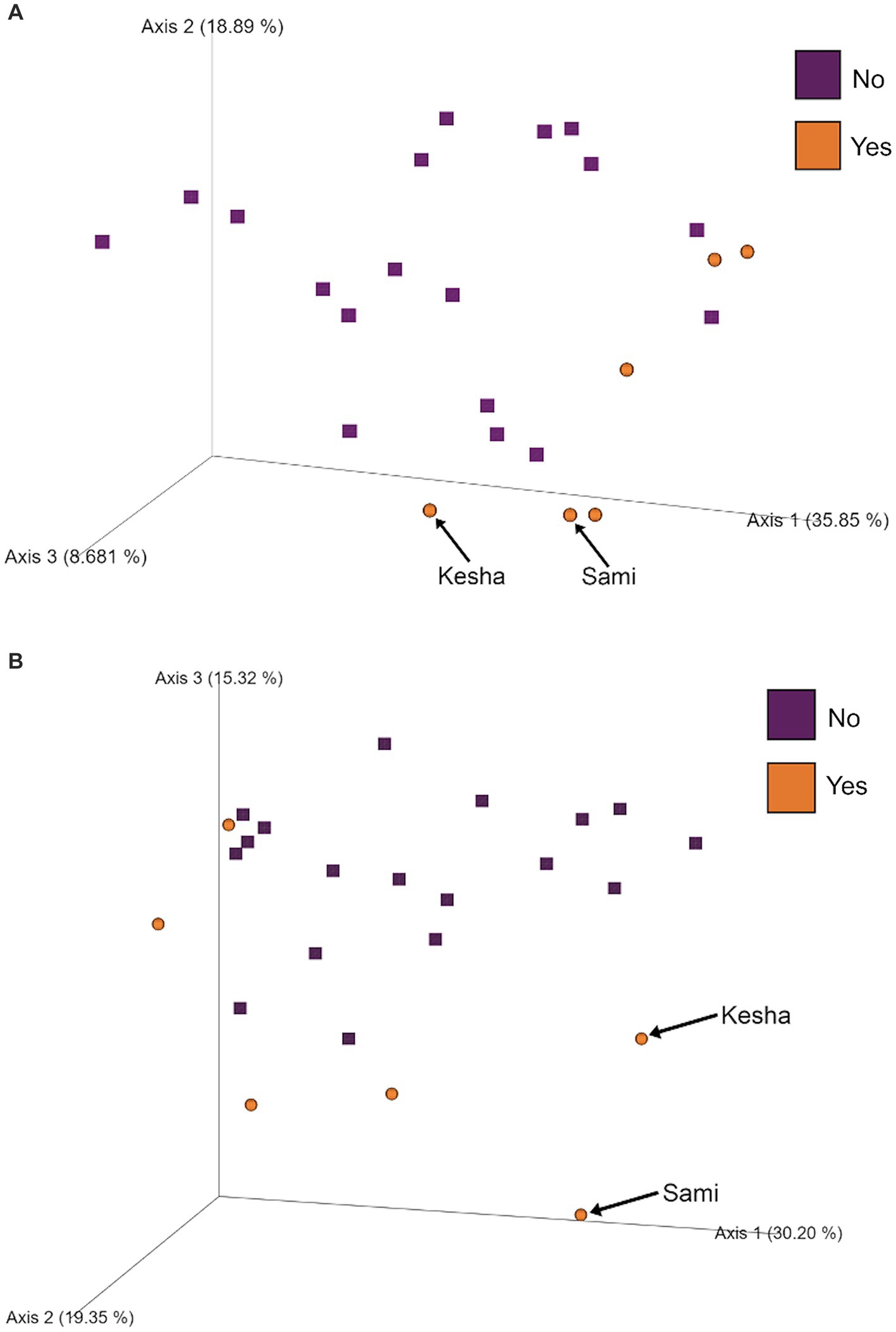
Figure 4. PCoA plots show clustering of dogs based on glucosamine supplementation in pre- and post-activity groups. PCoA plots were created to visualize beta-diversity differences between dogs who did (Yes) and did not (No) receive glucosamine supplementation in (A) pre-activity and (B) post-activity groups. Arrows indicate dogs Sami and Kesha.
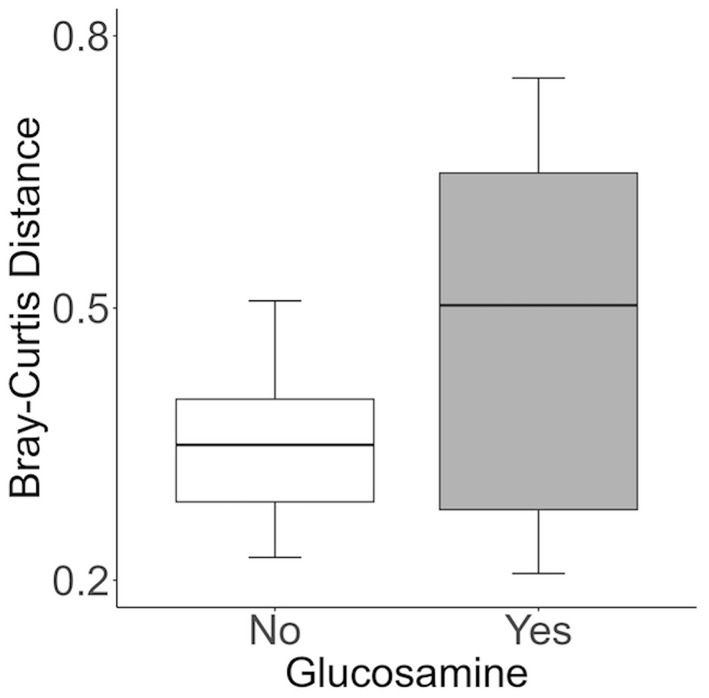
Figure 5. Self-pairwise biodiversity distances between no- and yes-glucosamine groups are not significantly different. Self-pairwise distances were calculated using Bray-Curtis for each dog (n = 24) and then a Kruskal Wallis test was performed between distance metrics and glucosamine supplementation status (Yes n = 6, No n = 18) (p = 0.255).
In order to further investigate the correlation between activity, glucosamine supplementation, and variation in the gut microbiome, we performed inter-individual pairwise analyses of beta-diversity distances based on glucosamine supplementation (NG vs. YG) and activity (Pre vs. Post) subgroup data (Figure 6). Analysis of fecal samples collected prior to activity (Figure 6A) indicate significantly greater differences in Bray-Curtis distance between dogs supplemented with glucosamine and those not supplemented (NG-YG) relative to comparisons of non-supplemented dogs to each other (NG-NG; p = 0.00082) or supplemented to each other (YG-YG; p = 0.019), providing further evidence that glucosamine influences microbiome composition. Post-activity, the NG-YG interindividual distance is again greater than the NG-NG comparisons (Figure 6B; p = 8.9E-11). However, the NG-YG distance trends lower than YG-YG distance (p = 0.074).
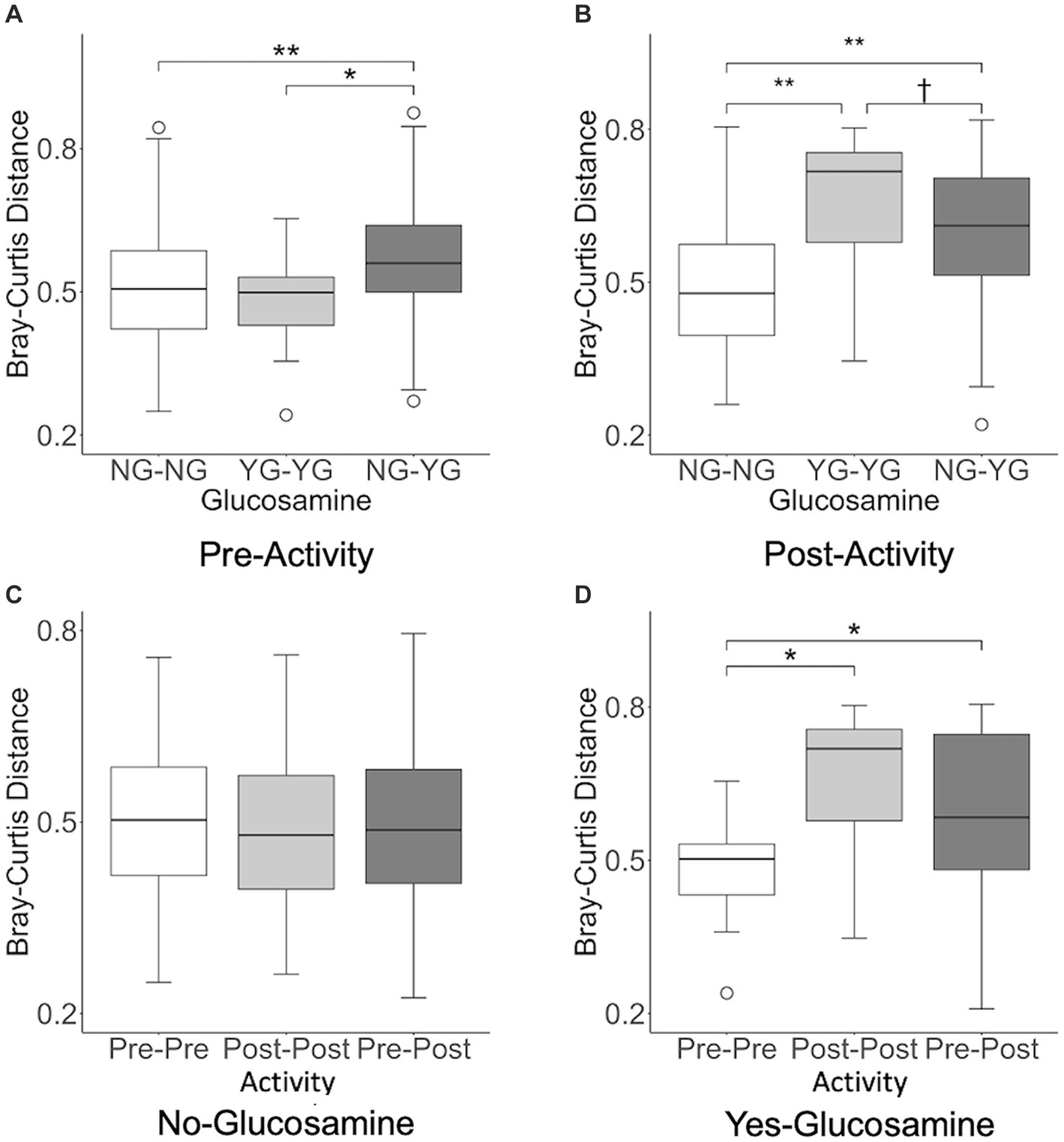
Figure 6. Interindividual pairwise distance analysis reveals variation in beta-diversity distances in pre-activity, post-activity, and glucosamine supplementation groups. Every pairwise Bray-Curtis beta-diversity distance was calculated in (A) pre-activity samples, (B) post-activity samples, (C) no glucosamine samples, and (D) glucosamine supplementation samples (†p < 0.10, *p < 0.05, **p < 0.001 as determined by the Wilcoxon rank-sum test with Benjamini-Hochberg p-value correction). NG, no glucosamine; YG, yes glucosamine; Pre, pre-activity; Post, post-activity.
We next examined variation in the gut microbiome pre- and post-activity in the context of glucosamine supplementation. For dogs not taking glucosamine supplementation, pairwise distances between different exercise-related activity subgroups did not differ (Figure 6C). However, for dogs taking a glucosamine supplement, the Pre-Pre distances were shorter than both the Post-Post distances (Figure 6D; p = 0.0081) and Pre-Post distances (Figure 6D; p = 0.045), again providing evidence for increased microbiome variability with glucosamine supplementation, especially in the context of activity.
We repeated this interindividual pairwise analysis on the dataset excluding Sam and Kesha (Supplementary Figure S2). Before activity, glucosamine supplementation was still associated with greater interindividual pairwise distance between NG-NG and YG-YG (p = 0.0018) and between NG-NG and NG-YG (p = 0.031). However, in the post-activity group only the NG-NG versus NG-YG differences remained significant (p = 0.0015; NG-NG vs. YG-YG: p = 0.82). While significant activity-related effects on distance were not observed in glucosamine-treated dogs in the absence of Sami and Kesha, the same general trends in Bray-Curtis distances were retained (Supplementary Figures S2C,D).
Taxonomic differential abundance analysis
As overall beta-diversity comparisons revealed variation associated with glucosamine supplementation and activity, we sought to determine if specific bacterial taxa were driving these differences. To this end, we first generated taxa bar plots showing relative abundances of the 8 most abundant phyla and found Firmicutes to be the most profuse taxa, followed by Bacteroidota, and Fusobacteria (Figure 7A). Investigating more deeply, we then generated bar plots for each dog’s 16 most abundant families in pre-activity and post-activity samples (Figure 7B). Grouping of these taxa by order reveals that bacteria from the Eubacteriales, Lactobacillales, Clostridiales, Bacteriodales, Fusobacteriales, and Erysipelotrichales orders were consistently most abundant. There was considerable variability in microbiome composition across all dogs. Visual inspection of changes in relative abundance of families pre- and post-activity suggests an increase in Streptococcaceae and a decrease in Fusobacteriaceae, although not consistently across all dogs. Two older dogs, Sami and Kesha exhibit a dramatic increase in Lactobacillaceae abundance after activity, but this increase is not consistent among most other dogs.
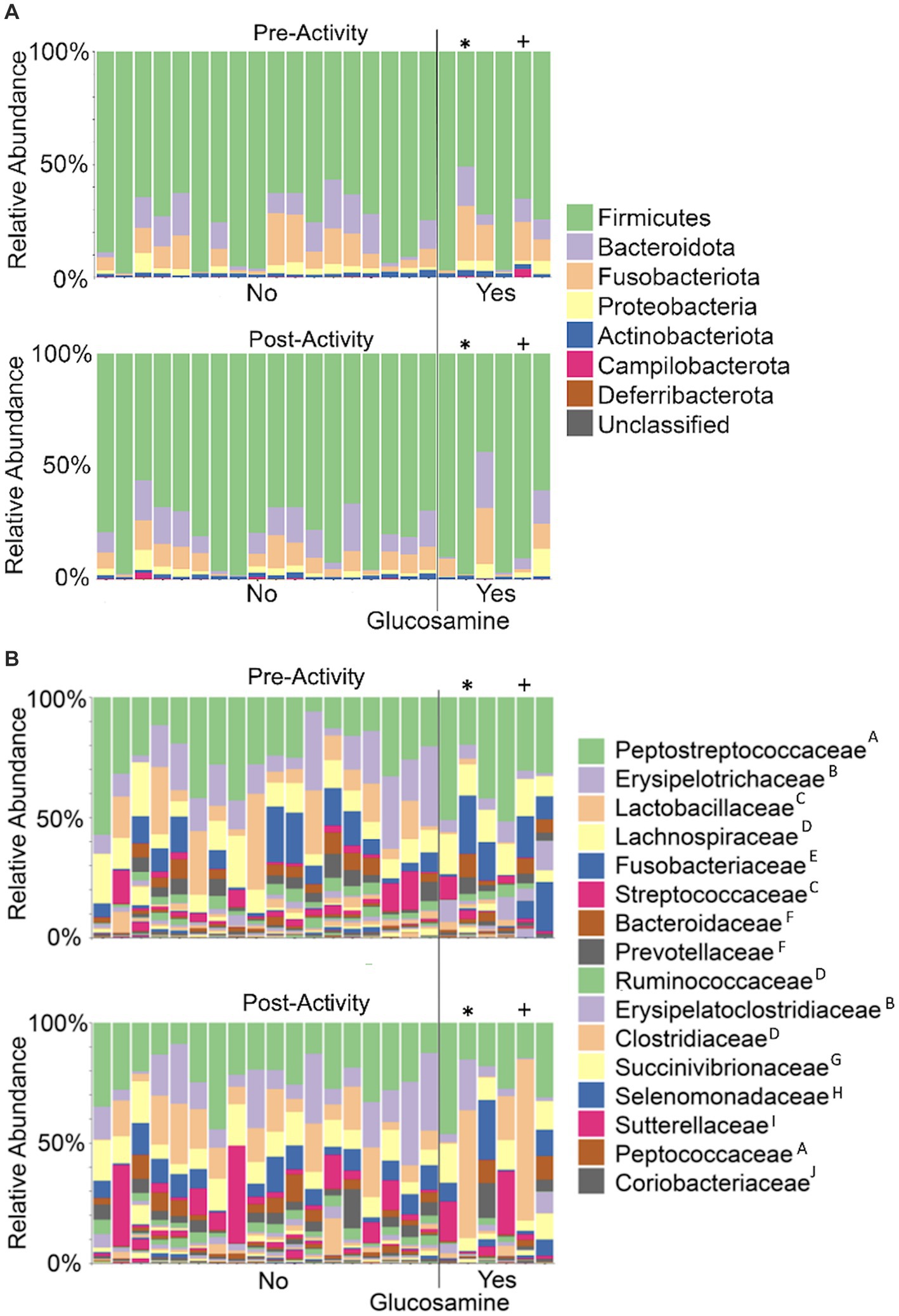
Figure 7. Taxonomic composition of the gut microbiome varies among dogs and within individual dogs pre- and post-activity. Microbial taxa were plotted for relative abundance by phylum (A) or family (B) for each dog pre-activity (top plot of each panel) or post-activity. Dogs to the left of the vertical line did not receive glucosamine (No) and those to the right received glucosamine supplementation (Yes). (* = Sami; + = Kesha) Superscripts indicate taxonomic orders: AEubacteriales, BErysipelotrichales, CLactobacillales, DClostridiales, EFusobacteriales, FBacteroidales, GAeromonadales, HSelenomonadales, IBurkholderiales, JCoriobacteriales.
To more closely examine which bacterial taxa exhibit variability among sled dogs, we performed an Analysis of Composition of Microbiomes (ANCOM) (65). ANCOM revealed that Lactobacillaceae and Anaerovoccaceae families exhibited the greatest variation in relative abundance among sled dogs (Figure 8). To more carefully examine the relationship between activity, glucosamine supplementation, and the variation in Lactobacillaceae and Anaerovoracaceae, we observed the relative change in abundance of each taxa under conditions of pre- and post-activity in dogs grouped by glucosamine supplementation. Lactobacillaceae was decreased in glucosamine-supplemented dogs before activity relative to the abundance levels in dogs not receiving glucosamine before and after activity (Figure 9A). Much greater Lactobacillaceae variability was observed in glucosamine-supplemented dogs post-activity, although the observed differences from dogs not taking glucosamine were not statistically significant. Given the increased relative abundance of Lactobacillaceae in Sami and Kesha after activity (see Figure 7), we repeated the analysis of relative abundance on the cohort minus these dogs (Supplementary Figure S3A). In the absence of data from Sami and Kesha, we still observe significantly lower Lactobacillaceae in glucosamine-supplemented dogs pre-activity relative to dogs not receiving glucosamine, and also see a trend toward lower Lactobacillaceae post-activity in glucosamine-treated dogs. However, this number is not statistically significant.
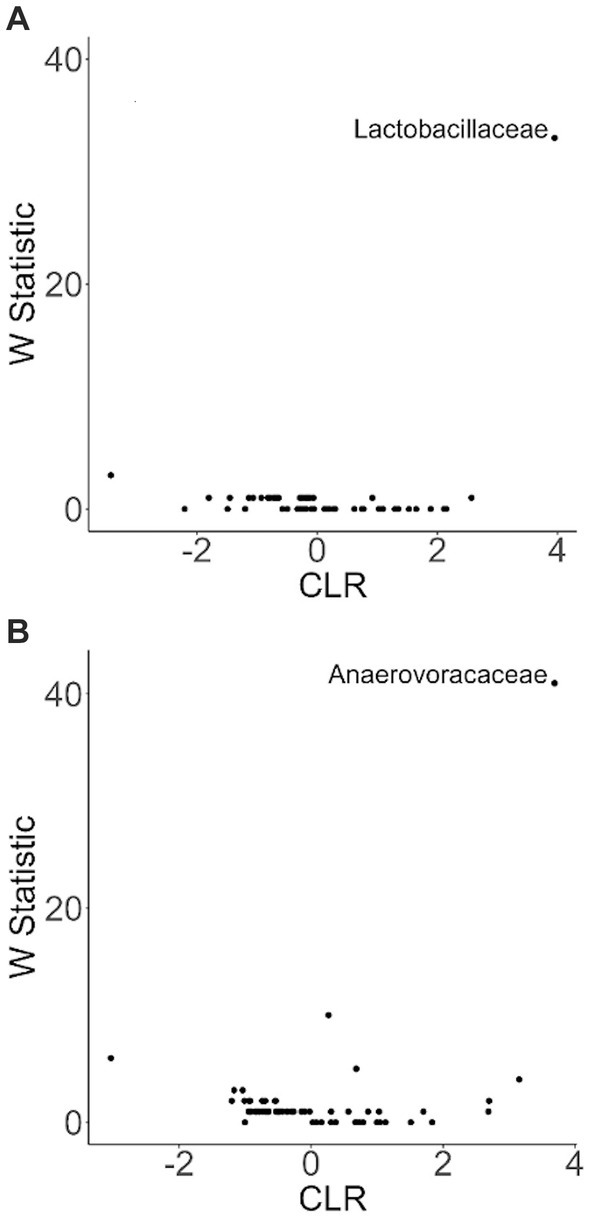
Figure 8. ANCOM plot at the genus level reveals relative abundance variability between glucosamine-treated and untreated dogs for Lactobacillaceae and Anaerovoccaceae families. ANCOM plots were created and visualized at the family level for the (A) pre-activity and (B) post-activity subgroups, using glucosamine as the comparison variable.
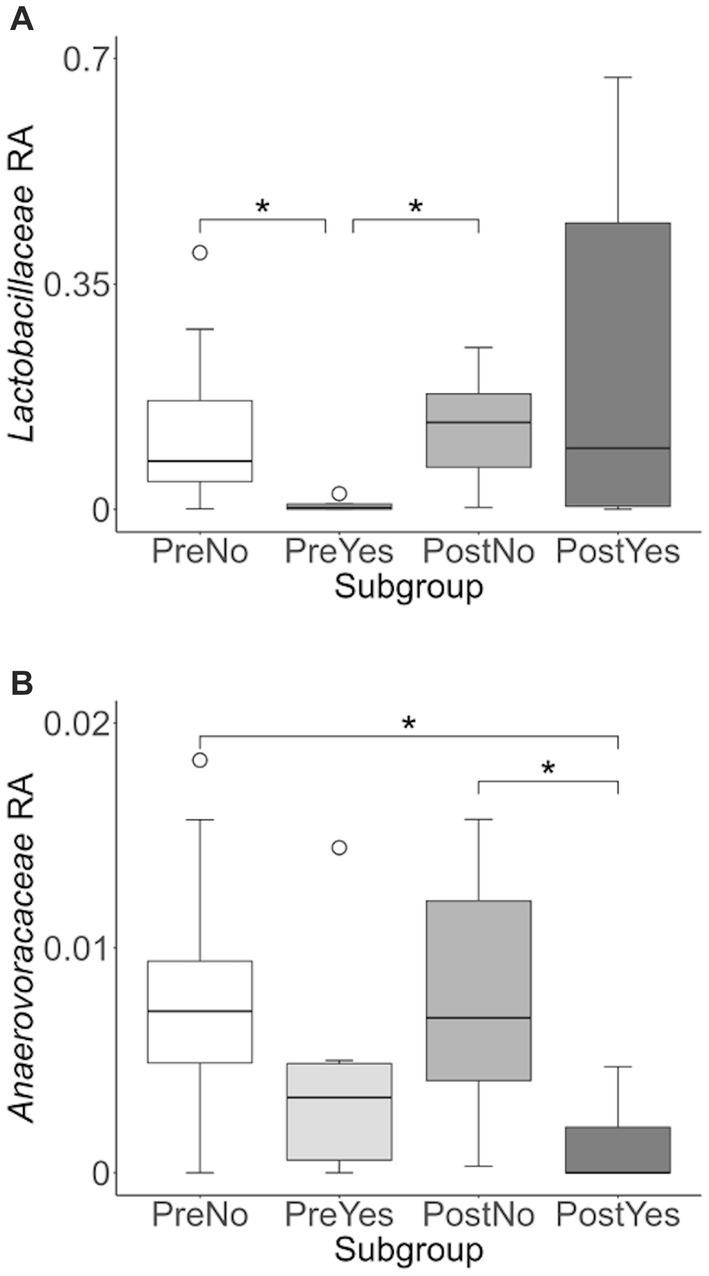
Figure 9. Lactobacillaceae and Anaerovoracaceae taxa abundance plots reveals differences in relative abundance by population subgroups. Relative abundance analysis was performed in (A) Lactobacillaceae and (B) Anaerovoracaceae families for pre-activity no-glucosamine (PreNo), pre-activity yes-glucosamine (PreYes), post-activity no-glucosamine (PostNo), and post-activity and yes-glucosamine (PostYes) population subgroups. (*p < 0.05 as determined by the Wilcoxon rank-sum test with Benjamini-Hochberg p-value correction).
Anaerovoracaceae abundance was decreased in glucosamine-supplemented dogs after activity, compared to dogs not receiving glucosamine, both before and after activity (Figure 9B). The same pattern of significant differences in Anaerovoracaceae were retained even when Sami and Kesha were excluded from analysis (Supplementary Figure S3B).
To investigate variation in the microbiome at a finer taxonomic level, ANCOM analysis was repeated using genus as the taxonomic criteria. At the genus level, our ANCOM plot revealed that Eubacterium [brachy], Sellimonas, Parvibacter, and an uncultured genus belonging to the same Eggerthellaceae family as Parvibacter clearly exhibited the greatest abundance variability among dogs, relative to other taxa (Figure 10). We again investigated the importance of activity and glucosamine supplementation on the varying abundances of these genera (Figure 11). Eubacterium [brachy] and Sellimonas abundances were lower in glucosamine-supplemented dogs post-activity relative to the abundances of pre- and post-activity dogs that did not receive glucosamine (Figures 11A,B). Parvibacter abundance was significantly lower after activity in glucosamine-supplemented dogs, compared to after activity in dogs that did not receive glucosamine and trended toward lower before activity, as well (Figure 11C). The uncultured genus belonging to the same Eggerthellaceae family as Parvibacter exhibited lower abundance in glucosamine-supplemented dogs after activity, compared to abundance in dogs that did not receive glucosamine, in both pre- and post-activity groups (Figure 11D). In genus-level analysis without Sami and Kesha the same trends were observed for Eubacterium [brachy], Sellimonus, and the uncultured genus, but not for Parvibacter (Supplementary Figure S4).
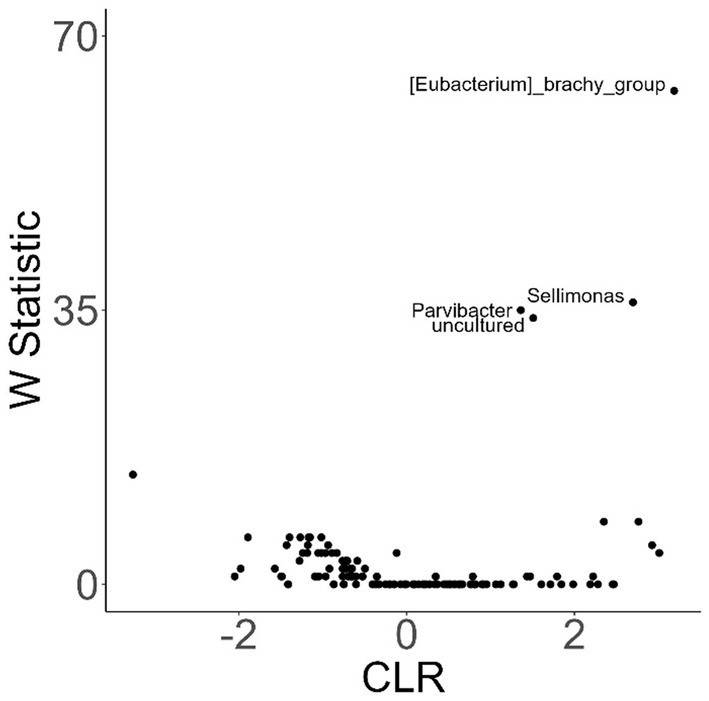
Figure 10. ANCOM plot at the genus level reveals relative abundance variability between glucosamine-treated and untreated dogs for Eubacterium [brachy], Sellimonas, Parvibacter, and an uncultured genus belonging to the same Eggerthellaceae family. ANCOM plots were created and visualized at the genus level for dogs post-activity, using glucosamine supplementation as the comparison variable.
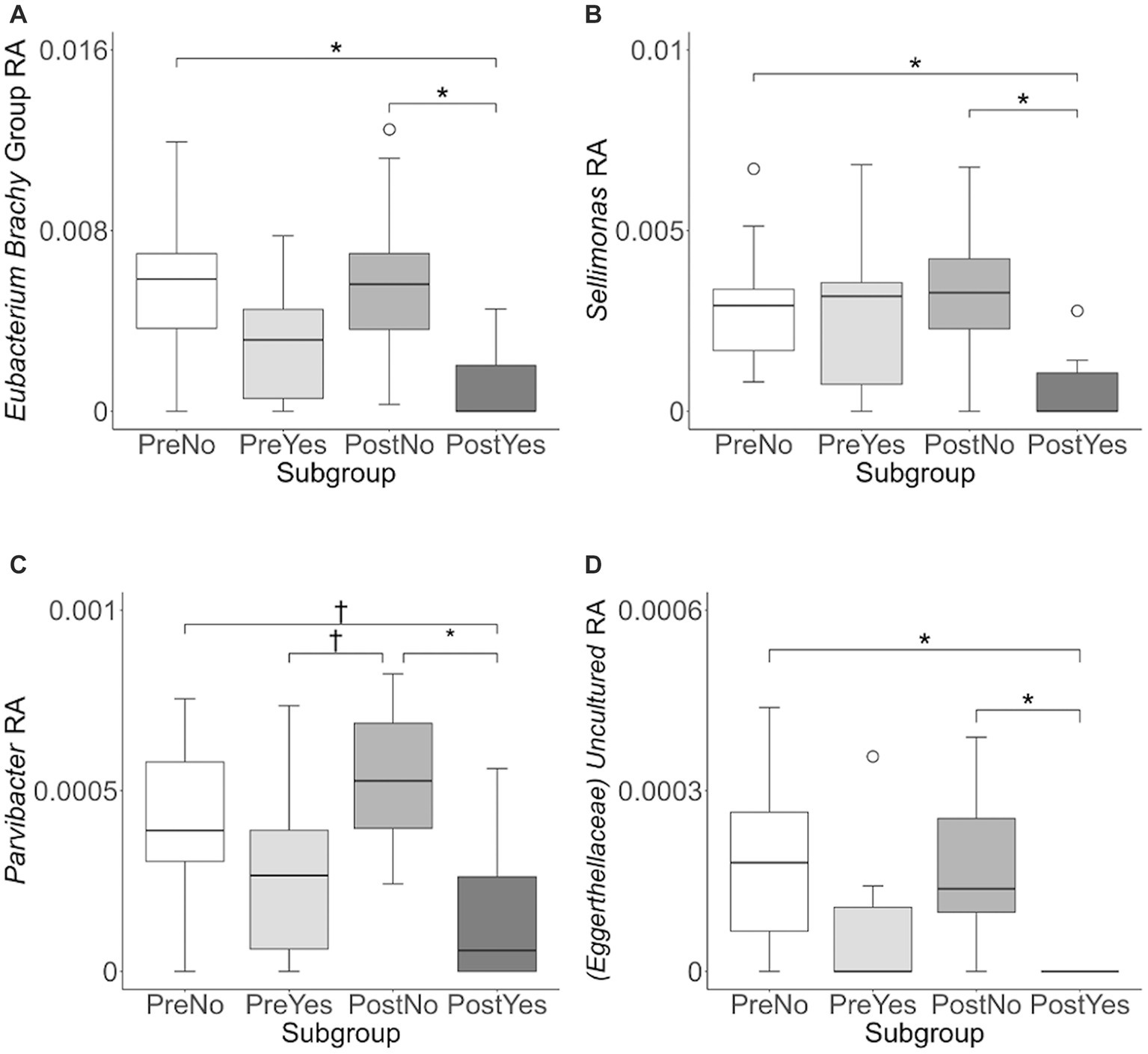
Figure 11. Eubacterium [brachy], Sellimonus, Parvibacter, and uncultured genera taxa abundance plots reveals differences in relative abundance by population subgroups. Relative abundance analysis was performed in (A) Eubacterium [brachy] (B) Sellimonas (C) Parvibacter, and (D) Uncultured genera from the same family as Parvibacter (Eggerthellaceae) for exercise and glucosamine supplementation subgroups. Eubacterium [brachy] includes the genus (Eubacterium), and the analytical tool also gave the species (brachy) when this analysis was performed at the genus level. (PreNo = pre-activity no-glucosamine; PreYes = pre-activity yes-glucosamine; PostNo = post-activity no-glucosamine; PostYes = post-activity and yes-glucosamine subgroups. *p < 0.05 and †p < 0.10 as determined by the Wilcoxon rank-sum test with Benjamini-Hochberg p-value correction).
Discussion
In this study, we sought to identify factors that correlate with variation in the fecal microbiome of a cohort of active sled dogs. We observe that younger age and dietary glucosamine supplementation are associated with reduced alpha-diversity. Variations in sex and activity also correlate with differences in alpha-diversity, specifically among those dogs receiving glucosamine supplementation. Beta-diversity analysis using PCoA reveals clustering among glucosamine supplemented dogs and inter-individual pairwise distances are longest between dogs of different glucosamine ingestion statuses. Taxonomic analysis at the family level reveals that sled dogs taking glucosamine have decreased Lactobacillaceae and Anaerovoracaceae. At the genus level, Eubacterium brachy, Sellimonas, Parvibacter, and an unclassified genus belonging to the same family as Parvibacter exhibited reduced abundances in glucosamine-supplemented dogs, particularly after activity. Our data provide evidence that glucosamine supplementation and exercise-related activity alter the gut microbiome in active sled dogs.
Alpha-diversity
The majority of significant variables in our alpha-diversity analysis were identified exclusively by Shannon’s Diversity metric. This metric considers both species richness and relative abundance, while Faith’s phylogenetic diversity, our other metric, reflects the number of phylogenetic units in a sample. Therefore, these findings suggest that most of these factors contribute to differences in relative abundances amid a relatively stable environment of microbial species richness. We observed variations in alpha-diversity for dogs that received glucosamine supplementation in the overall dataset and in the pre- and post-activity subgroups. Our results agree with previous observations that dogs supplemented with glucosamine or similar sulfated carbohydrates exhibit a significant decrease in alpha-diversity (44, 45, 67). Here, we provide evidence that other lifestyle factors, including activity and age, contribute to these changes.
Activity was associated with reduced alpha-diversity in the subgroup of dogs receiving glucosamine, according to Faith’s phylogenetic diversity. Previously, Tysnes et al. (24) found that alpha-diversity in non-supplemented sled dogs did not differ pre- and post-race. Similar to our findings above, it was also found that age had an important role in changes in gut composition after exercise (24). Interestingly, the removal of our two oldest dogs from the glucosamine subgroup eliminated the significant effect of activity. For this reason, it is unclear how much of this association is due to glucosamine and how much is due to age-related effects. Moreover, human studies have yielded conflicting findings but suggest that the effects of exercise are also highly dependent on the duration and intensity of exercise (68). Since activity was not uniform among dogs, this may also have a confounding role in our findings, although it was previously shown that training duration did not affect alpha-diversity in hunting dogs (69).
Our mixed effects analyses indicate that sled dogs in our cohort have a significant variation in alpha-diversity correlating with age, both overall and within the post-activity dogs and dogs not taking glucosamine. Age was not associated with alpha-diversity in the yes-glucosamine subgroup, possibly suggesting that glucosamine affects microbial richness in ways that obscure age-related effects. Plotting alpha-diversity with age revealed a trend toward an increase in Shannon’s Diversity index as dogs age, and this trend was retained in dogs taking glucosamine. Interestingly, previous studies in dogs have observed a decrease in gut microbiome alpha-diversity with age (70, 71) or no overall trend in diversity (72). However, these inconsistencies could be explained by the sample population, as the age range and health condition of sampled dogs strongly influence overall findings. Multiple studies find correlation between a decrease in alpha-diversity and advancing age in humans (73–76) see Martino et al. (77). However, more recent analyses suggest that the relationship between aging and microbiome complexity may be more complicated. Sato et al. (78) observe that centenarians actually have increased alpha-diversity compared to a young control population as calculated by Shannon index. Studies taking into account health status across a broad age range suggest that there is little difference in microbiome complexity between healthy young and old groups (79), or observe stability or increases in Shannon diversity in very old participants (80, 81). Health status and environmental factors are likely better predictors of microbiome composition than age (82, 83), or at least must be taken into account when considering the complex interactions between aging and gut microbiota (84, 85). The dogs in our study may retain high alpha-diversity at an older age as a result of some lifestyle factor such as exercise, communal living, diet, or health intervention.
Beta-diversity
PCoA analysis examining beta-diversity revealed clustering of the glucosamine supplemented cohort of dogs. This was reinforced by our inter-individual pairwise distance analysis, as Bray-Curtis distances are greatest when comparing glucosamine-treated dogs with untreated prior to activity. These findings suggest that glucosamine had clear effects on beta-diversity. These findings are supported in humans by Navarro et al. (67) who supplemented individuals with both glucosamine and chondroitin, a polymeric sulfated glycosaminoglycan. In a small study on humans, Moon et al. (45) supplemented individuals with glucosamine for 3 weeks at 3,000 mg and did not find significant differences in beta-diversity in supplemented individuals, despite changes in individual taxa. Interestingly, we found a greater Bray-Curtis distance after activity between dogs who received glucosamine compared to those without supplementation, which is contrary to the notion that glucosamine fosters a specific gut microbial environment conducive to anti-inflammatory symptoms. However, this difference largely disappeared upon removal of two older, glucosamine-supplemented dogs (Sami and Kesha) from our pairwise analysis. While our data reveal significant differences in both alpha and beta-diversity correlating with glucosamine supplementation, further studies will help clarify the mechanism of these alterations and their connections to activity and other life history factors.
Differential abundance of specific taxa
As part of our analysis, we examined changes in abundance across all taxa of bacteria and archaea detected through sequencing of the V4 variable region of 16S rRNA from glucosamine-supplemented and non-supplemented dogs. Glucosamine, a sulfated monosaccharide, can thus serve as a substrate for sulfate-reducing bacteria (43). One model for potential beneficial effects of glucosamine treatment is that the H2S produced by sulfate-reducing bacteria may have anti-inflammatory and cytoprotective effects (86–88), and may be involved in the resolution of tissue injury in the gut (88, 89). However, our data analysis did not reveal increased abundance of sulfate-reducing bacteria among taxa in glucosamine-treated dogs.
While we did not observe increases in sulfate-reducing taxa, we did observe the differential abundance of taxa implicated in inflammatory and anti-inflammatory processes at the family and genus levels. At the family level, we observed the reduction of Lactobacillaceae in glucosamine-treated dogs prior to activity and a significant variation in abundance post- activity. Lactobacillaceae rhamnosus has been shown to exert important anti-inflammatory effects in the gut (90), and is used as a therapeutic probiotic strain (91). Lactobacillus abundance has been associated with both inflammatory bowel diseases and chronic rheumatic diseases (92), and Lactobacillaceae has emerged as a potential biomarker for systemic inflammation (93). Indeed, recent research in Alaskan sled dogs found that dogs exhibited intestinal erosions following exercise. However, there was no difference in pro-inflammatory cytokines in these sled dogs (22). Therefore, the increase in pro-inflammatory taxa that we observe after activity in supplemented dogs may represent a more efficient response to inflammation, not exerting its effect by preventing inflammation in the first place.
We also observed differences in Anaerovoccaceae between glucosamine-treated and untreated dogs. While Anaerovoccaceae abundance did not significantly differ with or without glucosamine in the pre-activity group, it trended lower. And the abundance of this taxa was significantly reduced post-activity in glucosamine-supplemented dogs. Previously, physical activity has been positively associated with Anaerovoccaceae and negatively associated with propionate abundance (94). Propionate is a short-chain fatty acid (SCFA) known to have anti-inflammatory effects (95). Therefore, it seems possible that Anaerovoccaceae is a pro-inflammatory bacterial family, involved in suppressing inflammatory response.
At the genus level, we also observed the reduction of Parvibacter, Eubacterium [brachy], and Sellimonus after activity in glucosomine-treated dogs. Parvibacter abundance is also inversely associated with propionate abundance (96), so it may also be suppressed by Lactobacillaceae and/or sulfate-reducing bacteria. Eubacterium brachy was initially isolated from subgingival samples of patients with moderate and severe periodontitis (97), it has recently been found to have a potential role in metabolic syndrome in the microflora (98). Eubacterium brachy, among other species belonging to the Eubacteria genus, may secrete virulence factors that are associated with the degradation of host tissue as it localizes to infected sites (99). Eubacterium brachy could also be suppressed by Lactobacillaceae or sulfate-reducing bacteria following activity, which would mediate decreases in inflammatory response.
Sellimonus abundance has previously been associated with several inflammatory disorders including rheumatoid arthritis, ankylosing spondylitis, liver cirrhosis, and systemic-onset juvenile arthritis (100–102). Recent examination of Sellimonas has revealed that this genus may be a biomarker for gut homeostasis in diseased patients (103). S. intestinalis was sequenced and found to include genes involved in SCFA production (103), which is involved in gut barrier integrity, metabolism, immune system regulation, and regulation of inflammatory response (104). Sellimonus belongs to the Lachnospiraceae family, which contains many taxa involved in SCFA production and taxa that have been implicated in intra- and extra-intestinal diseases (105). Previously, it has been shown that acids produced by Lactobacillaceae species inhibit the growth of Lachnospiraceae taxa (106). While the mechanism of this interaction has not yet been delineated (106), this regulatory effect may demonstrate a decreased need for this reactionary anti-inflammatory response since there are more Lactobacillaceae taxa present to mitigate the more immediate inflammatory effects of exercise.
Limitations
Our findings must be considered in light of the following limitations. First, this study is a retrospective study utilizing a small dataset of 24 dogs, which may limit the generalizability of our findings. Those dogs receiving glucosamine supplementation ingested it in different forms (glucosamine and cosequin tablets and chews, some of which may contain ascorbic acid and/or methylsufonylmethane) and in different quantities. In addition, dogs with a wide variation in age were included in the study and those on glucosamine supplementation skewed older than those not taking glucosamine. Activity was also not equivalent for all dogs, as some pulled tour groups over a three-day span while others ran independently of sleds. However, all dogs engaged in physical activity over this span, potentially providing the effects that accompany endurance activity (107). With a larger sample size in the future, we might better be able to analyze the influence of sex and age on microbiome composition, and also include whether dogs are intact, as spayed or neutered dogs may have variations in hormone levels that could influence microbial composition (50, 108). Additionally, inter-individual genetic variation between dogs that could have contributed to variation in gut microbial composition and influenced our results. While insights suggest that Inuit dogs have retained genetic markers unique to their indigenous history, these breeds have occupied overlapping geographical regions as sled dogs since the European colonization of the Arctic (109, 110). Multiple studies have shown that gut microbial composition depends on environment (18, 111), which may contribute to similarities in microbiota among dogs in this study. Careful longitudinal analysis of multiple samples from before and after intense activity and with and without glucosamine supplementation will likely provide further insights into the influence of these variables on microbiome composition.
Conclusion
Our findings suggest that glucosamine supplementation impacts gut microbiome composition in this population of sled dogs, especially in the context of periods of greater activity. Sex, diet, and age also correlate with changes in gut microbiota, with an increasing complexity of microbes with increasing age. These results suggest that further study is important for understanding fully the effects of glucosamine supplementation in active dogs.
Data availability statement
The datasets presented in this study can be found in online repositories. The names of the repository/repositories and accession number(s) are available at the following link: http://www.ncbi.nlm.nih.gov/bioproject/1060330.
Ethics statement
The animal study was approved by Colgate University Institutional Animal Care and Use Committee. The study was conducted in accordance with the local legislation and institutional requirements.
Author contributions
DW: Data curation, Formal analysis, Investigation, Methodology, Visualization, Writing – review & editing. WR: Investigation, Visualization, Writing – original draft. KM: Data curation, Formal analysis, Investigation, Methodology, Visualization, Writing – review & editing. VD: Conceptualization, Investigation, Writing – review & editing. AA: Conceptualization, Formal analysis, Methodology, Project administration, Resources, Supervision, Visualization, Writing – review & editing. KDB: Conceptualization, Funding acquisition, Investigation, Project administration, Resources, Supervision, Writing – review & editing.
Funding
The author(s) declare financial support was received for the research, authorship, and/or publication of this article. This study was funded by Colgate University through the Stuart Updike Fund for Undergraduate Research, Faculty Research Council, and Russell Colgate Distinguished Chair to KDB. Summer support from Colgate University was provided for KM and DW through the Michael J. Wolk '60 Heart Foundation and for WAR through the Division of Natural Sciences and Mathematics. Support for open access publication comes from the Colgate University Faculty Research Council.
Acknowledgments
The authors would like to thank Deborah (DJ) Erb for sample collection, shipping, and helpful suggestions, Ana Jimenez for intellectual input, logistical guidance, and critical reading of the manuscript, Heather Huson for discussion and insights, Peter Schweitzer and Linda Cote for sequencing guidance and technical support, and lab members Aaron Jaynes, Elle Winter, Megan Herbruck, Isabela Cunio, Jeff Day, Precious Odiase, and Julia Kasperkowicz for valuable discussions. We extend special thanks to Kathleen Anderson at Wintermoon Adventures for her input into this study and for the participation of her dogs.
Conflict of interest
The authors declare that the research was conducted in the absence of any commercial or financial relationships that could be construed as a potential conflict of interest.
Publisher’s note
All claims expressed in this article are solely those of the authors and do not necessarily represent those of their affiliated organizations, or those of the publisher, the editors and the reviewers. Any product that may be evaluated in this article, or claim that may be made by its manufacturer, is not guaranteed or endorsed by the publisher.
Supplementary material
The Supplementary material for this article can be found online at: https://www.frontiersin.org/articles/10.3389/fvets.2024.1272711/full#supplementary-material
Footnotes
1. ^https://www.qiagen.com/us/products/discovery-and-translational-research/dna-rna-purification/dna-purification/microbial-dna/dneasy-powersoil-pro-kit
References
1. Sender, R, Fuchs, S, and Milo, R. Revised estimates for the number of human and bacteria cells in the body. PLoS Biol. (2016) 14:e1002533. doi: 10.1371/journal.pbio.1002533
2. Qin, J, Li, R, Raes, J, Arumugam, M, Burgdorf, KS, Manichanh, C, et al. A human gut microbial gene catalogue established by metagenomic sequencing. Nature. (2010) 464:59–65. doi: 10.1038/nature08821
3. Carroll, IM, Threadgill, DW, and Threadgill, DS. The gastrointestinal microbiome: a malleable, third genome of mammals. Mamm Genome. (2009) 20:395–403. doi: 10.1007/s00335-009-9204-7
4. Alessandri, G, Milani, C, Mancabelli, L, Mangifesta, M, Lugli, GA, Viappiani, A, et al. Metagenomic dissection of the canine gut microbiota: insights into taxonomic, metabolic and nutritional features. Environ Microbiol. (2019) 21:1331–43. doi: 10.1111/1462-2920.14540
5. Ley, RE, Peterson, DA, and Gordon, JI. Ecological and evolutionary forces shaping microbial diversity in the human intestine. Cell. (2006) 124:837–48. doi: 10.1016/j.cell.2006.02.017
6. Dash, S, Syed, YA, and Khan, MR. Understanding the role of the gut microbiome in brain development and its association with neurodevelopmental psychiatric disorders. Front Cell Dev Biol. (2022) 10:880544. doi: 10.3389/fcell.2022.880544
7. Fulde, M, and Hornef, MW. Maturation of the enteric mucosal innate immune system during the postnatal period. Immunol Rev. (2014) 260:21–34. doi: 10.1111/imr.12190
8. Hiippala, K, Jouhten, H, Ronkainen, A, Hartikainen, A, Kainulainen, V, Jalanka, J, et al. The potential of gut commensals in reinforcing intestinal barrier function and alleviating inflammation. Nutrients. (2018) 10:988. doi: 10.3390/nu10080988
9. Neuman, H, Debelius, JW, Knight, R, and Koren, O. Microbial endocrinology: the interplay between the microbiota and the endocrine system. FEMS Microbiol Rev. (2015) 39:509–21. doi: 10.1093/femsre/fuu010
10. Giles, EM, and Couper, J. Microbiome in health and disease. J Paediatr Child Health. (2020) 56:1735–8. doi: 10.1111/jpc.14939
11. Turner, PV. The role of the gut microbiota on animal model reproducibility. Anim Model Exp Med. (2018) 1:109–15. doi: 10.1002/ame2.12022
12. Huang, R, Ju, Z, and Zhou, PK. A gut dysbiotic microbiota-based hypothesis of human-to-human transmission of non-communicable diseases. Sci Total Environ. (2020) 745:141030. doi: 10.1016/j.scitotenv.2020.141030
13. West, CE, Renz, H, Jenmalm, MC, Kozyrskyj, AL, Allen, KJ, Vuillermin, P, et al. The gut microbiota and inflammatory noncommunicable diseases: associations and potentials for gut microbiota therapies. J Allergy Clin Immunol. (2015) 135:3–13. doi: 10.1016/j.jaci.2014.11.012
14. Hugenholtz, F, and de Vos, WM. Mouse models for human intestinal microbiota research: a critical evaluation. Cell Mol Life Sci. (2018) 75:149–60. doi: 10.1007/s00018-017-2693-8
15. Yue, F, Cheng, Y, Breschi, A, Vierstra, J, Wu, W, Ryba, T, et al. A comparative encyclopedia of DNA elements in the mouse genome. Nature. (2014) 515:355–64. doi: 10.1038/nature13992
16. Axelsson, E, Ratnakumar, A, Arendt, ML, Maqbool, K, Webster, MT, Perloski, M, et al. The genomic signature of dog domestication reveals adaptation to a starch-rich diet. Nature. (2013) 495:360–4. doi: 10.1038/nature11837
17. Botigué, LR, Song, S, Scheu, A, Gopalan, S, Pendleton, AL, Oetjens, M, et al. Ancient European dog genomes reveal continuity since the early Neolithic. Nat Commun. (2017) 8:16082. doi: 10.1038/ncomms16082
18. Song, SJ, Lauber, C, Costello, EK, Lozupone, CA, Humphrey, G, Berg-Lyons, D, et al. Cohabiting family members share microbiota with one another and with their dogs. elife. (2013) 2:e00458. doi: 10.7554/eLife.00458
19. Fleyshman, DI, Wakshlag, JJ, Huson, HJ, Loftus, JP, Olby, NJ, Brodsky, L, et al. Development of infrastructure for a systemic multidisciplinary approach to study aging in retired sled dogs. Aging (Albany NY). (2021) 13:21814–37. doi: 10.18632/aging.203600
20. Davis, MS, Willard, MD, Nelson, SL, Mandsager, RE, McKiernan, BS, Mansell, JK, et al. Prevalence of gastric lesions in racing Alaskan sled dogs. J Vet Intern Med. (2003) 17:311–4. doi: 10.1111/j.1939-1676.2003.tb02453.x
21. Davis, MS, Willard, MD, Williamson, KK, Steiner, JM, and Williams, DA. Sustained strenuous exercise increases intestinal permeability in racing Alaskan sled dogs. J Vet Intern Med. (2005) 19:34–9. doi: 10.1111/j.1939-1676.2005.tb02655.x
22. Mabry, K, Davis, MS, Gould, E, Gogal, RM, Steiner, JM, Tolbert, MK, et al. Assessment of gastrointestinal health in racing Alaskan sled dogs using capsule endoscopy and inflammatory cytokines. J Small Anim Pract. (2023) 64:574–80. doi: 10.1111/jsap.13627
23. Gagné, JW, Wakshlag, JJ, Simpson, KW, Dowd, SE, Latchman, S, Brown, DA, et al. Effects of a synbiotic on fecal quality, short-chain fatty acid concentrations, and the microbiome of healthy sled dogs. BMC Vet Res. (2013) 9:246. doi: 10.1186/1746-6148-9-246
24. Tysnes, KR, Angell, IL, Fjellanger, I, Larsen, SD, Søfteland, SR, Robertson, LJ, et al. Pre- and post-race intestinal microbiota in long-distance sled dogs and associations with performance. Animals (Basel). (2020) 10:204. doi: 10.3390/ani10020204
25. Lo, J, Chan, L, and Flynn, S. A systematic review of the incidence, prevalence, costs, and activity and work limitations of amputation, osteoarthritis, rheumatoid arthritis, Back pain, multiple sclerosis, spinal cord injury, stroke, and traumatic brain injury in the United States: a 2019 update. Arch Phys Med Rehabil. (2021) 102:115–31. doi: 10.1016/j.apmr.2020.04.001
26. Eakin, GS, Amodeo, KL, and Kahlon, RS. Arthritis and its public health burden. Dela J Public Health. (2017) 3:36–44. doi: 10.32481/djph.2017.03.006
28. Johnston, SA. Osteoarthritis. Joint anatomy, physiology, and pathobiology. Vet Clin North Am Small Anim Pract. (1997) 27:699–723. doi: 10.1016/S0195-5616(97)50076-3
29. Henrotin, Y, Mobasheri, A, and Marty, M. Is there any scientific evidence for the use of glucosamine in the management of human osteoarthritis? Arthritis Res Ther. (2012) 14:201. doi: 10.1186/ar3657
30. Qato, DM, Alexander, GC, Conti, RM, Johnson, M, Schumm, P, and Lindau, ST. Use of prescription and over-the-counter medications and dietary supplements among older adults in the United States. JAMA. (2008) 300:2867–78. doi: 10.1001/jama.2008.892
31. Sandersoln, RO, Beata, C, Flipo, RM, Genevois, JP, Macias, C, Tacke, S, et al. Systematic review of the management of canine osteoarthritis. Vet Rec. (2009) 164:418–24. doi: 10.1136/vr.164.14.418
32. Clegg, DO, Reda, DJ, Harris, CL, Klein, MA, O'Dell, JR, Hooper, MM, et al. Glucosamine, chondroitin sulfate, and the two in combination for painful knee osteoarthritis. N Engl J Med. (2006) 354:795–808. doi: 10.1056/NEJMoa052771
33. Bernetti, A, Mangone, M, Villani, C, Alviti, F, Valeo, M, Grassi, MC, et al. Appropriateness of clinical criteria for the use of SYmptomatic slow-acting drug for OsteoArthritis (SYSADOA). A Delphi method consensus initiative among experts in Italy. Eur J Phys Rehabil Med. (2019) 55:658–64. doi: 10.23736/S1973-9087.19.05633-8
34. Sellam, J, Courties, A, Eymard, F, Ferrero, S, Latourte, A, Ornetti, P, et al. Recommendations of the French Society of Rheumatology on pharmacological treatment of knee osteoarthritis. Joint Bone Spine. (2020) 87:548–55. doi: 10.1016/j.jbspin.2020.09.004
35. Bannuru, RR, Osani, MC, Vaysbrot, EE, Arden, NK, Bennell, K, Bierma-Zeinstra, SMA, et al. OARSI guidelines for the non-surgical management of knee, hip, and polyarticular osteoarthritis. Osteoarthr Cartil. (2019) 27:1578–89. doi: 10.1016/j.joca.2019.06.011
36. Kolasinski, SL, Neogi, T, Hochberg, MC, Oatis, C, Guyatt, G, Block, J, et al. 2019 American College of Rheumatology/Arthritis Foundation guideline for the Management of Osteoarthritis of the hand, hip, and knee. Arthritis Care Res (Hoboken). (2020) 72:149–62. doi: 10.1002/acr.24131
37. Zhu, X, Sang, L, Wu, D, Rong, J, and Jiang, L. Effectiveness and safety of glucosamine and chondroitin for the treatment of osteoarthritis: a meta-analysis of randomized controlled trials. J Orthop Surg Res. (2018) 13:170. doi: 10.1186/s13018-018-0871-5
38. Kampa, N, Kaenkangploo, D, Jitpean, S, Srithunyarat, T, Seesupa, S, Hoisang, S, et al. Study of the effectiveness of glucosamine and chondroitin sulfate, marine based fatty acid compounds (PCSO-524 and EAB-277), and carprofen for the treatment of dogs with hip osteoarthritis: a prospective, block-randomized, double-blinded, placebo-controlled clinical trial. Front Vet Sci. (2023) 10:10. doi: 10.3389/fvets.2023.1033188
39. Roman-Blas, J, Castañeda, S, Sánchez-Pernaute, O, Largo, R, and Herrero-Beaumont, GCS/GS Combined Therapy Study Group. Chondroitin sulfate plus glucosamine sulfate shows no superiority over placebo in a randomized, double-blind, placebo-controlled clinical trial in patients with knee osteoarthritis. Arthritis Rheumatol (Hoboken, NJ). (2016) 69:77–85. doi: 10.1002/art.39819
40. Adebowale, A, du, J, Liang, Z, Leslie, JL, and Eddington, ND. The bioavailability and pharmacokinetics of glucosamine hydrochloride and low molecular weight chondroitin sulfate after single and multiple doses to beagle dogs. Biopharm Drug Dispos. (2002) 23:217–25. doi: 10.1002/bdd.315
41. Ibrahim, A, Gilzad-kohan, MH, Aghazadeh-Habashi, A, and Jamali, F. Absorption and bioavailability of glucosamine in the rat. J Pharm Sci. (2012) 101:2574–83. doi: 10.1002/jps.23145
42. Shmagel, A, Demmer, R, Knights, D, Butler, M, Langsetmo, L, Lane, N, et al. The effects of glucosamine and chondroitin sulfate on gut microbial composition: a systematic review of evidence from animal and human studies. Nutrients. (2019) 11:988. doi: 10.3390/nu11020294
43. Benjdia, A, and Berteau, O. Sulfatases and radical SAM enzymes: emerging themes in glycosaminoglycan metabolism and the human microbiota. Biochem Soc Trans. (2016) 44:109–15. doi: 10.1042/BST20150191
44. Coulson, S, Butt, H, Vecchio, P, Gramotnev, H, and Vitetta, L. Green-lipped mussel extract (Perna canaliculus) and glucosamine sulphate in patients with knee osteoarthritis: therapeutic efficacy and effects on gastrointestinal microbiota profiles. Inflammopharmacology. (2013) 21:79–90. doi: 10.1007/s10787-012-0146-4
45. Moon, JM, Finnegan, P, Stecker, RA, Lee, H, Ratliff, KM, Jäger, R, et al. Impact of glucosamine supplementation on gut health. Nutrients. (2021) 13:2180. doi: 10.3390/nu13072180
46. Craig, JM. Atopic dermatitis and the intestinal microbiota in humans and dogs. Vet Med Sci. (2016) 2:95–105. doi: 10.1002/vms3.24
47. Kieler, IN, Shamzir Kamal, S, Vitger, AD, Nielsen, DS, Lauridsen, C, and Bjornvad, CR. Gut microbiota composition may relate to weight loss rate in obese pet dogs. Vet Med Sci. (2017) 3:252–62. doi: 10.1002/vms3.80
48. Masuoka, H, Shimada, K, kiyosue-Yasuda, T, Kiyosue, M, Oishi, Y, Kimura, S, et al. Transition of the intestinal microbiota of dogs with age. Biosci Microbiota Food Health. (2017) 36:27–31. doi: 10.12938/bmfh.BMFH-2016-021
49. Reddy, KE, Kim, HR, Jeong, JY, So, KM, Lee, S, Ji, SY, et al. Impact of breed on the fecal microbiome of dogs under the same dietary condition. J Microbiol Biotechnol. (2019) 29:1947–56. doi: 10.4014/jmb.1906.06048
50. Scarsella, E, Stefanon, B, Cintio, M, Licastro, D, Sgorlon, S, Dal Monego, S, et al. Learning machine approach reveals microbial signatures of diet and sex in dog. PLoS One. (2020) 15:e0237874. doi: 10.1371/journal.pone.0237874
51. Parada, AE, Needham, DM, and Fuhrman, JA. Every base matters: assessing small subunit rRNA primers for marine microbiomes with mock communities, time series and global field samples. Environ Microbiol. (2016) 18:1403–14. doi: 10.1111/1462-2920.13023
52. Apprill, A, McNally, S, Parsons, R, and Weber, L. Minor revision to V4 region SSU rRNA 806R gene primer greatly increases detection of SAR11 bacterioplankton. Aquat Microb Ecol. (2015) 75:129–37. doi: 10.3354/ame01753
53. Caporaso, JG, Lauber, CL, Walters, WA, Berg-Lyons, D, Huntley, J, Fierer, N, et al. Ultra-high-throughput microbial community analysis on the Illumina HiSeq and MiSeq platforms. ISME J. (2012) 6:1621–4. doi: 10.1038/ismej.2012.8
54. Bolyen, E, Rideout, JR, Dillon, MR, Bokulich, NA, Abnet, CC, al-Ghalith, GA, et al. Reproducible, interactive, scalable and extensible microbiome data science using QIIME 2. Nat Biotechnol. (2019) 37:852–7. doi: 10.1038/s41587-019-0209-9
55. Callahan, BJ, McMurdie, PJ, Rosen, MJ, Han, AW, Johnson, AJA, and Holmes, SP. DADA2: high-resolution sample inference from Illumina amplicon data. Nat Methods. (2016) 13:581–3. doi: 10.1038/nmeth.3869
56. Quast, C, Pruesse, E, Yilmaz, P, Gerken, J, Schweer, T, Yarza, P, et al. The SILVA ribosomal RNA gene database project: improved data processing and web-based tools. Nucleic Acids Res. (2013) 41:D590–6. doi: 10.1093/nar/gks1219
57. Shannon, CE. A mathematical theory of communication. Bell Syst Tech J. (1948) 27:379–423. doi: 10.1002/j.1538-7305.1948.tb01338.x
58. Faith, DP. Conservation evaluation and phylogenetic diversity. Biol Conserv. (1992) 61:1–10. doi: 10.1016/0006-3207(92)91201-3
59. Bray, JR, and Curtis, JT. An ordination of the upland Forest communities of southern Wisconsin. Ecol Monogr. (1957) 27:325–49. doi: 10.2307/1942268
60. Vázquez-Baeza, Y, Pirrung, M, Gonzalez, A, and Knight, R. EMPeror: a tool for visualizing high-throughput microbial community data. Gigascience. (2013) 2:16. doi: 10.1186/2047-217X-2-16
61. Wilcoxon, F. Individual comparisons by ranking methods. Biom Bull. (1945) 1:80–3. doi: 10.2307/3001968
62. Kruskal, WH, and Wallis, WA. Use of ranks in one-criterion variance analysis. J Am Stat Assoc. (1952) 47:583–621. doi: 10.1080/01621459.1952.10483441
65. Mandal, S, Van Treuren, W, White, RA, Eggesbø, M, Knight, R, and Peddada, SD. Analysis of composition of microbiomes: a novel method for studying microbial composition. Microb Ecol Health Dis. (2015) 26:27663. doi: 10.3402/mehd.v26.27663
66. R Core Team. R: A language and environment for statistical computing. Vienna: R Foundation for Statistical Computing (2021).
67. Navarro, SL, Levy, L, Curtis, KR, Lampe, JW, and Hullar, MAJ. Modulation of gut microbiota by glucosamine and chondroitin in a randomized, double-blind pilot trial in humans. Microorganisms. (2019) 7:610. doi: 10.3390/microorganisms7120610
68. Ortiz-Alvarez, L, Xu, H, and Martinez-Tellez, B. Influence of exercise on the human gut microbiota of healthy adults: a systematic review. Clin Transl Gastroenterol. (2020) 11:e00126. doi: 10.14309/ctg.0000000000000126
69. Zannoni, A, Pietra, M, Gaspardo, A, Accorsi, PA, Barone, M, Turroni, S, et al. Non-invasive assessment of fecal stress biomarkers in hunting dogs during exercise and at rest. Front Vet Sci. (2020) 7:126. doi: 10.3389/fvets.2020.00126
70. Mizukami, K, Uchiyama, J, Igarashi, H, Murakami, H, Osumi, T, Shima, A, et al. Age-related analysis of the gut microbiome in a purebred dog colony. FEMS Microbiol Lett. (2019) 366:fnz095. doi: 10.1093/femsle/fnz095
71. OMATSU, T, OMURA, M, KATAYAMA, Y, KIMURA, T, OKUMURA, M, OKUMURA, A, et al. Molecular diversity of the faecal microbiota of toy poodles in Japan. J Vet Med Sci. (2018) 80:749–54. doi: 10.1292/jvms.17-0582
72. Jha, AR, Shmalberg, J, Tanprasertsuk, J, Perry, LA, Massey, D, and Honaker, RW. Characterization of gut microbiomes of household pets in the United States using a direct-to-consumer approach. PLoS One. (2020) 15:e0227289. doi: 10.1371/journal.pone.0227289
73. Biragyn, A, and Ferrucci, L. Gut dysbiosis: a potential link between increased cancer risk in ageing and inflammaging. Lancet Oncol. (2018) 19:e295–304. doi: 10.1016/S1470-2045(18)30095-0
74. Kumar, M, Babaei, P, Ji, B, and Nielsen, J. Human gut microbiota and healthy aging: recent developments and future prospective. Nutr Healthy Aging. (2016) 4:3–16. doi: 10.3233/NHA-150002
75. O'Toole, PW, and Jeffery, IB. Gut microbiota and aging. Science. (2015) 350:1214–5. doi: 10.1126/science.aac8469
76. Saraswati, S, and Sitaraman, R. Aging and the human gut microbiota-from correlation to causality. Front Microbiol. (2014) 5:764. doi: 10.3389/fmicb.2014.00764
77. Martino, C, Dilmore, AH, Burcham, ZM, Metcalf, JL, Jeste, D, and Knight, R. Microbiota succession throughout life from the cradle to the grave. Nat Rev Microbiol. (2022) 20:707–20. doi: 10.1038/s41579-022-00768-z
78. Sato, Y, Atarashi, K, Plichta, DR, Arai, Y, Sasajima, S, Kearney, SM, et al. Novel bile acid biosynthetic pathways are enriched in the microbiome of centenarians. Nature. (2021) 599:458–64. doi: 10.1038/s41586-021-03832-5
79. Bian, G, Gloor, GB, Gong, A, Jia, C, Zhang, W, Hu, J, et al. The gut microbiota of healthy aged Chinese is similar to that of the healthy young. mSphere. (2017) 2:e00327-17. doi: 10.1128/mSphere.00327-17
80. Pang, S, Chen, X, Lu, Z, Meng, L, Huang, Y, Yu, X, et al. Longevity of centenarians is reflected by the gut microbiome with youth-associated signatures. Natu Aging. (2023) 3:436–49. doi: 10.1038/s43587-023-00389-y
81. Zhou, X, Wang, B, Demkowicz, PC, Johnson, JS, Chen, Y, Spakowicz, DJ, et al. Exploratory studies of oral and fecal microbiome in healthy human aging. Front Aging. (2022) 3:1002405. doi: 10.3389/fragi.2022.1002405
82. Si, J, Vázquez-Castellanos, JF, Gregory, AC, Decommer, L, Rymenans, L, Proost, S, et al. Long-term life history predicts current gut microbiome in a population-based cohort study. Nat Aging. (2022) 2:885–95. doi: 10.1038/s43587-022-00286-w
83. Claesson, MJ, Jeffery, IB, Conde, S, Power, SE, O’Connor, EM, Cusack, S, et al. Gut microbiota composition correlates with diet and health in the elderly. Nature. (2012) 488:178–84. doi: 10.1038/nature11319
84. Ghosh, TS, das, M, Jeffery, IB, and O'Toole, PW. Adjusting for age improves identification of gut microbiome alterations in multiple diseases. elife. (2020) 9:9. doi: 10.7554/eLife.50240
85. Wilmanski, T, Diener, C, Rappaport, N, Patwardhan, S, Wiedrick, J, Lapidus, J, et al. Gut microbiome pattern reflects healthy ageing and predicts survival in humans. Nat Metab. (2021) 3:274–86. doi: 10.1038/s42255-021-00348-0
86. Gambari, L, Grigolo, B, and Grassi, F. Hydrogen sulfide in bone tissue regeneration and repair: state of the art and new perspectives. Int J Mol Sci. (2019) 20:5231. doi: 10.3390/ijms20205231
87. Saini, V, Chinta, KC, Reddy, VP, Glasgow, JN, Stein, A, Lamprecht, DA, et al. Hydrogen sulfide stimulates Mycobacterium tuberculosis respiration, growth and pathogenesis. Nat Commun. (2020) 11:557. doi: 10.1038/s41467-019-14132-y
88. Wallace, JL, and Wang, R. Hydrogen sulfide-based therapeutics: exploiting a unique but ubiquitous gasotransmitter. Nat Rev Drug Discov. (2015) 14:329–45. doi: 10.1038/nrd4433
89. Papapetropoulos, A, Pyriochou, A, Altaany, Z, Yang, G, Marazioti, A, Zhou, Z, et al. Hydrogen sulfide is an endogenous stimulator of angiogenesis. Proc Natl Acad Sci U S A. (2009) 106:21972–7. doi: 10.1073/pnas.0908047106
90. Segers, ME, and Lebeer, S. Towards a better understanding of Lactobacillus rhamnosus GG--host interactions. Microb Cell Factories. (2014) 13:S7. doi: 10.1186/1475-2859-13-S1-S7
91. Doron, S, Snydman, DR, and Gorbach, SL. Lactobacillus GG: bacteriology and clinical applications. Gastroenterol Clin N Am. (2005) 34:483–98, ix. doi: 10.1016/j.gtc.2005.05.011
92. Salem, F, Kindt, N, Marchesi, JR, Netter, P, Lopez, A, Kokten, T, et al. Gut microbiome in chronic rheumatic and inflammatory bowel diseases: similarities and differences. United European Gastroenterol J. (2019) 7:1008–32. doi: 10.1177/2050640619867555
93. Asgharian, M, Gholizadeh, P, Samadi Kafil, H, Ghojazadeh, M, Samadi, A, Soleymani, J, et al. Correlation of inflammatory biomarkers with the diversity of Bacteroidaceae, Bifidobacteriaceae, Prevotellaceae and Lactobacillaceae families in the intestinal microbiota of patients with end stage renal disease. Adv Med Sci. (2022) 67:304–10. doi: 10.1016/j.advms.2022.07.004
94. Magzal, F, Shochat, T, Haimov, I, Tamir, S, Asraf, K, Tuchner-Arieli, M, et al. Increased physical activity improves gut microbiota composition and reduces short-chain fatty acid concentrations in older adults with insomnia. Sci Rep. (2022) 12:2265. doi: 10.1038/s41598-022-05099-w
95. Tedelind, S, Westberg, F, Kjerrulf, M, and Vidal, A. Anti-inflammatory properties of the short-chain fatty acids acetate and propionate: a study with relevance to inflammatory bowel disease. World J Gastroenterol. (2007) 13:2826–32. doi: 10.3748/wjg.v13.i20.2826
96. Li, Q, Li, L, Li, Q, Wang, J, Nie, S, and Xie, M. Influence of natural polysaccharides on intestinal microbiota in inflammatory bowel diseases: an overview. Foods. (2022) 11:1084. doi: 10.3390/foods11081084
97. Holdeman, LV, Cato, EP, Burmeister, J, and Moore, W. Descriptions of Eubacterium timidum sp. nov., Eubacterium brachy sp. nov., and Eubacterium nodatum sp. nov. isolated from human periodontitis. Int J Syst Evol Microbiol. (1980) 30:163–9.
98. Chen, Q, Wang, B, Wang, S, Qian, X, Li, X, Zhao, J, et al. Modulation of the gut microbiota structure with probiotics and Isoflavone alleviates metabolic disorder in Ovariectomized mice. Nutrients. (2021) 13:1793. doi: 10.3390/nu13061793
99. Wade, WG. The role of Eubacterium species in periodontal disease and other Oral infections. Microb Ecol Health Dis. (1996) 9:367–70. doi: 10.3109/08910609609166480
100. Nayfach, S, Shi, ZJ, Seshadri, R, Pollard, KS, and Kyrpides, NC. New insights from uncultivated genomes of the global human gut microbiome. Nature. (2019) 568:505–10. doi: 10.1038/s41586-019-1058-x
101. Sun, Y, Chen, Q, Lin, P, Xu, R, He, D, Ji, W, et al. Characteristics of gut microbiota in patients with rheumatoid arthritis in Shanghai, China. Front Cell Infect Microbiol. (2019) 9:369. doi: 10.3389/fcimb.2019.00369
102. Dong, YQ, Wang, W, Li, J, Ma, MS, Zhong, LQ, Wei, QJ, et al. Characterization of microbiota in systemic-onset juvenile idiopathic arthritis with different disease severities. World J Clin Cases. (2019) 7:2734–45. doi: 10.12998/wjcc.v7.i18.2734
103. Muñoz, M, Guerrero-Araya, E, Cortés-Tapia, C, Plaza-Garrido, A, Lawley, TD, and Paredes-Sabja, D. Comprehensive genome analyses of Sellimonas intestinalis, a potential biomarker of homeostasis gut recovery. Microb Genom. (2020) 6:mgen000476. doi: 10.1099/mgen.0.000476
104. Nogal, A, Valdes, AM, and Menni, C. The role of short-chain fatty acids in the interplay between gut microbiota and diet in cardio-metabolic health. Gut Microbes. (2021) 13:1–24. doi: 10.1080/19490976.2021.1897212
105. Vacca, M, Celano, G, Calabrese, FM, Portincasa, P, Gobbetti, M, and de Angelis, M. The controversial role of human gut Lachnospiraceae. Microorganisms. (2020) 8:573. doi: 10.3390/microorganisms8040573
106. Brownlie, EJE, Chaharlangi, D, Wong, EOY, Kim, D, and Navarre, WW. Acids produced by lactobacilli inhibit the growth of commensal Lachnospiraceae and S24-7 bacteria. Gut Microbes. (2022) 14:2046452. doi: 10.1080/19490976.2022.2046452
107. Bonomini-Gnutzmann, R, Plaza-Díaz, J, Jorquera-Aguilera, C, Rodríguez-Rodríguez, A, and Rodríguez-Rodríguez, F. Effect of intensity and duration of exercise on gut microbiota in humans: a systematic review. Int J Environ Res Public Health. (2022) 19:9518. doi: 10.3390/ijerph19159518
108. Coelho, LP, Kultima, JR, Costea, PI, Fournier, C, Pan, Y, Czarnecki-Maulden, G, et al. Similarity of the dog and human gut microbiomes in gene content and response to diet. Microbiome. (2018) 6:72. doi: 10.1186/s40168-018-0450-3
109. Brown, SK, Darwent, CM, Wictum, EJ, and Sacks, BN. Using multiple markers to elucidate the ancient, historical and modern relationships among north American Arctic dog breeds. Heredity (Edinb). (2015) 115:488–95. doi: 10.1038/hdy.2015.49
110. Moon, KL, Huson, HJ, Morrill, K, Wang, MS, Li, X, Srikanth, K, et al. Comparative genomics of Balto, a famous historic dog, captures lost diversity of 1920s sled dogs. Science. (2023) 380:eabn5887. doi: 10.1126/science.abn5887
Keywords: microbiome, glucosamine, cosequin, canine health, gut health, microbial diversity
Citation: Wang D, Russel WA, Macdonald KM, De Leon VM, Ay A and Belanger KD (2024) Analysis of the gut microbiome in sled dogs reveals glucosamine- and activity-related effects on gut microbial composition. Front. Vet. Sci. 11:1272711. doi: 10.3389/fvets.2024.1272711
Edited by:
Monica Isabella Cutrignelli, University of Naples Federico II, ItalyReviewed by:
Dennis E. Jewell, Kansas State University, United StatesGeorge Golovko, University of Texas Medical Branch at Galveston, United States
Bruno Stefanon, University of Udine, Italy
Copyright © 2024 Wang, Russel, Macdonald, De Leon, Ay and Belanger. This is an open-access article distributed under the terms of the Creative Commons Attribution License (CC BY). The use, distribution or reproduction in other forums is permitted, provided the original author(s) and the copyright owner(s) are credited and that the original publication in this journal is cited, in accordance with accepted academic practice. No use, distribution or reproduction is permitted which does not comply with these terms.
*Correspondence: Ahmet Ay, YWF5QGNvbGdhdGUuZWR1; Kenneth D. Belanger, a2JlbGFuZ2VyQGNvbGdhdGUuZWR1
†These authors have contributed equally to this work