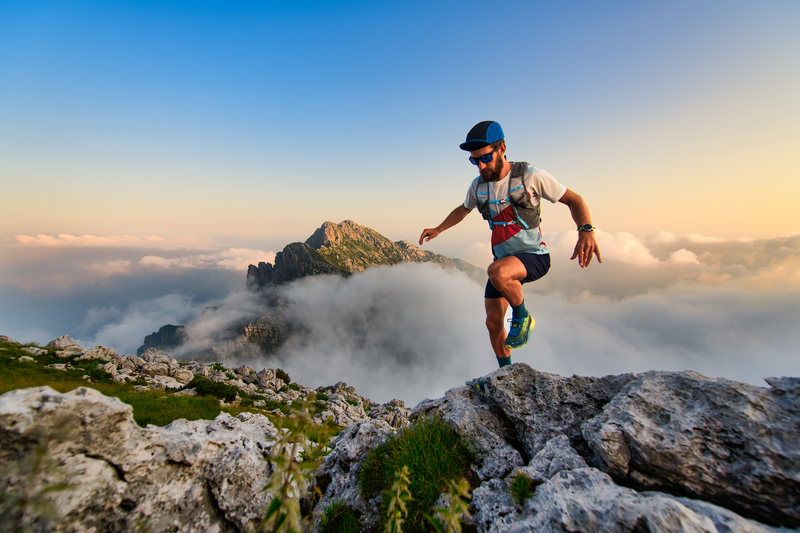
95% of researchers rate our articles as excellent or good
Learn more about the work of our research integrity team to safeguard the quality of each article we publish.
Find out more
ORIGINAL RESEARCH article
Front. Vet. Sci. , 23 October 2023
Sec. Veterinary Infectious Diseases
Volume 10 - 2023 | https://doi.org/10.3389/fvets.2023.1238634
Introduction: Brucella, a type of intracellular Gram-negative bacterium, has unique features and acts as a zoonotic pathogen. It can lead to abortion and infertility in animals. Eliminating brucellosis becomes very challenging once it spreads among both humans and animals, putting a heavy burden on livestock and people worldwide. Given the increasing spread of brucellosis, it is crucial to develop improved vaccines for susceptible animals to reduce the disease’s impact.
Methods: In this study, we effectively used an immunoinformatics approach with advanced computer software to carefully identify and analyze important antigenic parts of Brucella abortus. Subsequently, we skillfully designed chimeric peptides to enhance the vaccine’s strength and effectiveness. We used computer programs to find four important parts of the Brucella bacteria that our immune system recognizes. Then, we carefully looked for eight parts that are recognized by a type of white blood cell called cytotoxic T cells, six parts recognized by T helper cells, and four parts recognized by B cells. We connected these parts together using a special link, creating a strong new vaccine. To make the vaccine even better, we added some extra parts called molecular adjuvants. These included something called human β-defensins 3 (hBD-3) that we found in a database, and another part that helps the immune system called PADRE. We attached these extra parts to the beginning of the vaccine. In a new and clever way, we made the vaccine even stronger by attaching a part from a mouse’s immune system to the end of it. This created a new kind of vaccine called MEV-Fc. We used advanced computer methods to study how well the MEV-Fc vaccine interacts with certain receptors in the body (TLR-2 and TLR-4).
Results: In the end, Immunosimulation predictions showed that the MEV-Fc vaccine can make the immune system respond strongly, both in terms of cells and antibodies.
Discussion: In summary, our results provide novel insights for the development of Brucella vaccines. Although further laboratory experiments are required to assess its protective effect.
Brucella is a Gram-negative bacterium that lives inside cells and can cause reproductive problems in animals and chronic illnesses in humans (1). This disease, known as brucellosis or by other names like Wave fever or Malta fever, is a widespread bacterial infection affecting both animals and humans in various regions around the world. It is considered one of the most common bacterial zoonotic diseases globally (2). Brucella has a strong ability to invade a specific type of white blood cell called macrophages, which makes it resistant to many antibiotics commonly used against other bacteria. As a result, this harmful pathogen poses a significant threat to global public health, causing considerable social and economic challenges (3).
Controlling brucellosis in livestock currently relies on using Brucella abortus S19, B. abortus RB51, and B. melitensis Rev-1 strains (4). However, completely removing the remaining virulence associated with these weakened vaccine strains is a challenging task. There is also a risk that these vaccine strains could infect humans, which might worsen the spread of the disease. Furthermore, these strains can induce abortions in pregnant animals, causing significant economic losses. Additionally, their presence complicates the accurate diagnosis and management of brucellosis (5, 6). Consequently, we are facing significant challenges when it comes to diagnosing and treating brucellosis, emphasizing the urgent need for a safe and effective vaccine or therapy (7).
In light of these challenges, peptide vaccines offer a promising approach to combat brucellosis. Not only do they stimulate strong antibody responses, but they also address the safety concerns associated with live vaccines (7). Peptide-based interventions provide a safer and practical alternative for dealing with the devastating impact of brucellosis.
In previous studies, researchers have explored a range of outer membrane proteins (OMPs) and effector proteins as potential immunodominant antigens against Brucella. Notably, two such proteins, OMP16 and OMP19, which are outer membrane lipoproteins, are prominently found on the surface of all Brucella strains (8). These lipoproteins are universally present in Brucella.OMP16, similar to a protein called peptidoglycan-associated lipoprotein (PAL) found in other Gram-negative bacteria, is highly conserved and plays a crucial role in maintaining the structural integrity and function of the outer membrane. Interestingly, OMP16 also acts as a pathogen-associated molecular pattern (PAMP) in Brucella abortus, which means it triggers the activation of dendritic cells (DCs) in the body, leading to a strong Th1 immune response (9). Encouragingly, recombinant OMP16 has been shown to generate a potent protective immune response in mice, making it a highly promising candidate for a vaccine (10).
In contrast, OMP19 is resistant to protease degradation, particularly following oral infection with Brucella abortus. Additionally, it acts as a protective shield for another protein called OMP25, preventing it from being degraded by proteases (11).
Intriguingly, It has been revealed that OMP19 inhibits MHC-II expression and hinders antigen presentation, prevents T cell recognition to evade host immunity and establishes chronic infections (12). Because of its strong protective qualities against Brucella, OMP19 looks like a very promising candidate for a vaccine to fight brucellosis (13).
OMP25 is an important protein on the outer membrane of Brucella abortus. It helps keep the cell’s outer covering strong and intact (14). Interestingly, OMP25 also plays a role in reducing the production of certain immune signals like tumor necrosis factor-alpha (TNF-α) and interleukin 12 (IL-12), which are typically produced when the body is fighting infections caused by other germs. This shows that OMP25 has a significant effect on the immune system (15). Studies have shown that vaccines made from OMP25’s DNA and protein can protect mice from Brucella abortus infection, making OMP25 a strong candidate for a vaccine (16, 17).
Another protein called Brucella ribosomal protein L7/L12 is known for being easily recognized by the immune system and staying similar across different Brucella types (18). This protein can activate a specific type of white blood cell called monocytes in animals with infections. This activation leads to the production of interferon-gamma, an important immune molecule that helps protect against Brucella abortus infection (19, 20). Because of these proteins’ ability to trigger immune responses, they seem like good options for designing vaccines that can delay or prevent brucellosis.
Antibodies are made of two parts: the antigen binding fragment (Fab) and the crystallizable fragment (Fc). Using Fc fragment fusion protein technology is a very effective way to make protein and peptide drugs last longer in the body. Important biological molecules found on the surface of cells, like cell receptors, cytokines, enzymes, and peptide antigens on harmful germs, can be combined with Fc parts. This makes these molecules more stable in the body and helps them last longer, making their effects stronger (21, 22). When we combine specific antigens with Fc parts, it creates a new and promising strategy for vaccines to fight diseases (23).
A study by David G. Alleva showed that when AKS-452 was fused with the Fc fragment, it produced much higher levels of neutralizing antibodies in mice compared to when Fc was not used. This significant boost in the body’s response highlights the enormous potential of using the immunoglobulin Fc fragment fusion in peptide vaccines (24). Using this method for making vaccines has a lot of promise for various treatments and preventive measures.
Previous studies have shown that Brucella multi-epitope vaccines (MEVs), created by predicting important parts recognized by the immune system, offer a level of immune protection, although not as effective as weakened vaccines. However, a new approach using peptide vaccines that include multi-epitope molecules fused to IgG-Fc for fighting Brucella infections has not been explored much. So, we combined the IgG Fc fragment with a multi-epitope structure, forming the peptide molecule MEV-Fc. This molecule includes important parts from different immune responses like CTL, HTL, B cells, hBD-3, PADRE, and IgG Fc.
To understand how effective this combined molecule could be, we used predictive tools to study its features, structure, and how it interacts with immune receptors such as TLR2 and TLR4. Additionally, we used a tool called C-ImmSim server to simulate how the immune cells would respond after the molecule is given as a vaccine to mice (Figure 1). Our main goal in this study was to create a peptide molecule that could enhance the immune protection provided by Brucella subunit vaccines. This could be a groundbreaking approach in preventing brucellosis.
The amino acid sequences of the main Brucella antigens, specifically OMP16 (AEF59023.1), OMP19 (AAB06277.1), L7/L12 (AAL51929.1), and OMP25 (AFJ79953.1), were retrieved from the NCBI database and are detailed in Table 1. To determine whether these four proteins have antigenic properties, we employed the VaxiJen server v2.0, setting a threshold value at 0.5 (25).
To predict Cytotoxic T Lymphocyte (CTL) epitopes for the primary Brucella antigen, we utilized the IEDB MHC I server, accessible (26).1 The specific parameters we used were as follows: Prediction Method: IEDB recommended 2020.09 (NetMHCpan EL 4.1); Source species of MHC: Human; HLA allele reference set, and Length set to 9 and 10. We selected epitopes with a percentile rank of less than 0.5 for further analysis. We also used Class I Immunogenicity to predict immunogenicity and chose CTL epitopes with a percentile rank of less than 0.5 and an immunoscore greater than 0 for further analysis. To determine the antigenicity of these screened epitopes, we employed the VaxiJen server v2.0, setting a threshold at 0.5 (25). The epitopes that passed these criteria were considered as immunodominant CTL epitopes for the construction of MEV-Fc.
To predict Helper T Lymphocyte (HTL) epitopes for the dominant Brucella antigen, we utilized the IEDB MHCII server 2, which can be accessed (27).2 The specific parameters we used were as follows: Prediction Method: IEDB recommended 2.22; MHC source species: Human; MHC allele set selected as all reference. We set the epitope length to 15. The predicted epitopes were ranked based on percentile rank, and we selected those with a percentile rank of less than 0.5. To predict their antigenicity, we used the VaxiJen server v2.0 with a threshold set at 0.5 (25). We chose epitopes with an antigenicity value greater than 0.5, and we predicted their inducibility of IFN-γ using an epitope server (28) specifically designed for IFN-γ. Ultimately, the epitope that was predicted to induce IFN-γ was selected as the HTL immunodominant epitope for the construction of MEV-Fc.
To predict linear B-cell epitopes for the dominant Brucella antigen, we used the ABCpred server, which is accessible,3 with the following parameters: epitope length = 16 and screening threshold = 0.51. We employed thresholds ranging from +0.1 to +1.0 (29). The highest ABCpred score among these thresholds was selected as the immunodominant B-cell epitope for MEV (Multi-Epitope Vaccine) construction.
To create the MEV, we used an appropriate linker to connect the individual epitopes. This linker prevents these epitopes from interacting with each other. The MEV was formed by connecting the predicted and screened CTL epitopes, an HTL epitope, and a linear B-cell epitope using the GPGPG linker.
To further enhance its ability to trigger an immune response and ensure the persistence of the helper T cell 1 (TH1) response (30), we added the hBD-3 sequence (PDB ID: 1KJ6) and the PADRE sequence to the N-terminal end of the MEV, using EAAAK linkers. Finally, to make the peptide more effective at triggering an immune response and to make it last longer in the body, we added the sequence of the mouse immunoglobulin IgG Fc fragment (P01868-1) by connecting it to the C-terminus of the MEV with KK linkers. This resulted in the complete sequence of the vaccine construct.
We evaluated the properties of MEV-Fc using bioinformatic analysis software. Firstly, we analyzed its physical and chemical characteristics using the ProtParam server, accessible.4 Next, we conducted antimicrobial and immunogenicity analyses using the VaxiJen v2.0 server. We specifically utilized the IEDB I class immunogenicity module, with VaxiJen predictions showing an accuracy range of 70 to 89% (25). Subsequently, we predicted solubility using the SOLpro server, available,5 which demonstrated an overall accuracy of 74.15% (31). Finally, we performed sensitivity and toxicity analyses using AlergenFP v1.0, accessible (32),6 and ToxinPred, available (33).7
We predicted the secondary structure of MEV-Fc using the SOPMA secondary structure prediction tool. To forecast the tertiary structure of MEV-Fc, we utilized the Robetta server (34). To assess the quality of the constructed models, we employed the Prosa Web Server (35), the Ramachandran Plot, and the PROCHECK Server (36), which can be accessed.8 These tools help evaluate the correctness and quality of the protein’s three-dimensional structure. We further assessed the uncertainty of the tertiary structure through ERRAT, available.9 ERRAT examines the overall quality factor of non-bonded atomic interactions, with higher values indicating better quality (37).
B-cell epitopes are particular sites on antigens where B-cell antibodies can bind and trigger immune responses (38). In vaccine development, these epitopes play a crucial role in generating an effective immune response. To predict B-cell epitopes for MEV-Fc, we utilized the ElliPro server with default parameters (39).
Molecular docking is a fundamental and promising method for studying how peptides interact with human immune receptors like TLR2 and TLR4 (40). It offers a quick and cost-effective way to understand the detailed interactions at the atomic level within the structure of the antibody–antigen complex and its interface.
Here’s how we conducted the molecular docking analysis: We obtained the PDB files of TLR2 (PDB ID: 2Z7X) and TLR4 (PDB ID: 2Z63) from the NCBI Molecular Modeling Database (MMDB), which is accessible.10 Next, we performed ligand-receptor docking analysis using the ClusPro 2.0 online server, which you can find (41).11 We then examined the interaction surfaces of the resulting complexes using PDBE Pisa, available (42).12 Finally, we visualized the complexes using PyMOL software and analyzed them using Ligplot+ software.
We performed molecular dynamics simulations of the MEV-Fc-TLR2 and MEV-Fc-TLR4 complexes using the iMODSweb server. This server, which is accessible,13 allows us to explore possible trajectories between two conformations and enables interactive analysis of the resulting structures, animations, and trajectories in three dimensions. Importantly, it can effectively simulate and explore even large molecules (43).
We used the C-ImmSim server, which you can access,14 to predict the ability of MEV-Fc to stimulate the production of specific antibodies and various cytokines by immune cells (44). This server is capable of predicting the immune response of both B lymphocytes and T lymphocytes, including Th1 and Th2 lymphocytes.
In our simulation, we considered the recommended minimum interval between the initial and subsequent doses of most vaccines, which is 28 days (45). Therefore, we configured the simulation parameters as follows: three injections, with each injection spaced 28 days apart; a random seed value set to 12,345; a simulation volume of 50; and a total of 1,050 simulation steps. We kept the remaining parameters at their default values.
To obtain an optimized nucleotide sequence, we used the Java Codon Adaptation Tool (JCat), which is available.15 This tool quickly generates optimized codon sequences tailored to the chosen expression host, thereby enhancing the production yield of heterologous proteins (46). In this case, we selected E. coli (strain K12) as the preferred bacterial strain. The ideal Codon Adaptation Index (CAI) value is 1, and the GC content percentage should ideally fall within the range of 30 to 70%.
After obtaining the optimized gene sequence, we cloned it into the expression vector pET28a(+) using Hind III and BamHI digestion sites. Subsequently, we analyzed the cloned sequence using Snapgene software.
A set of four proteins was retrieved from the NCBI server, and subsequently, their antigenicity was predicted using VaxiJen (Table 1). Utilizing the IEDB MHCI and MHC II servers, we predicted a total of 308 CTL epitopes (Supplementary Table 1) and 89 HTL epitopes (Supplementary Table 2) with a percentile rank of 0.5. Among the 308 CTL epitopes, 57 epitopes with immunogenicity >0 and an antigenicity score > 1 were selected (Supplementary Table 3). Additionally, from the 89 HTL epitopes, 28 epitopes exhibiting positive induction of IFN-γ and an antigenicity score > 0 were selected (Supplementary Table 4). The ABCpred server was employed to predict 68 linear B cell epitopes (Supplementary Table 5). Ultimately, 18 epitopes were chosen as candidates for vaccine construction (Table 2), including eight epitopes with the highest immunogenicity or highest antigenicity score, six HTL epitopes with the highest antigenicity score or highest IFN-γ scores, and four B cell epitopes with the highest ABCpred prediction scores. The predicted and screened CTL epitopes, HTL epitopes, and linear B-cell epitopes were linked together using GPGPG linkers. The hBD-3 sequences and PADRE sequences were connected via EAAAK linkers, while the fc (PDB ID: 1KJ6) sequences were linked using KK linkers. The resulting peptide molecule was named MEV-Fc, and its schematic diagram and amino acid sequence are presented in Figure 2.
Figure 2. (A) Schematic diagram of the MEV-Fc construct. (B) Amino acid sequence of the MEV-Fc construct.
MEV-Fc, a protein we studied, has some important characteristics. It is composed of 600 amino acids and has a molecular weight of about 61.2 kilodaltons. Its isoelectric point (PI) is approximately 8.89, indicating its charge at a specific pH. It is relatively stable with an instability index of 24.06 and Aliphatic index of 57.35. In terms of its lifespan, MEV-Fc is estimated to last about 30 h in mammalian reticulocytes in vitro. It has a slight preference for water, making it slightly hydrophilic with a GRAVY value of −0.500 (Table 3). Moreover, it is highly likely to be soluble during production, with a probability of 0.976819.
Regarding its potential as an antigen, predictions suggest it has a high likelihood of triggering an immune response with an immunogenicity score of 4.18 and good antigenicity (1.0342). Importantly, MEV-Fc is considered non-sensitizing and non-toxic based on prediction, indicating its safety profile.
In summary, MEV-Fc possesses favorable characteristics, including stability, solubility, and the potential to elicit an immune response, making it a strong candidate for a peptide vaccine. Additionally, it is considered safe for use.
The results of the secondary structure prediction (Figure 3A) show that MEV-Fc’s conformation consists of 15.33% alpha helix (Hh), 19.17% extended strand (Ee), 7.17% beta-turn (Tt), and 58.33% random coil (Cc). To predict the tertiary structure of MEV-Fc, we used the Robetta server, and the resulting model was visualized using the Pymol software package (Figure 3B). To assess the model’s quality, we conducted ProSA-web analysis, which yielded a Z-Score of −7.88 (Figure 4A). This Z-Score falls within the normal range for native proteins of similar size, confirming the credibility of the model. Additionally, we generated a Ramachandran plot using the PROCHECK server to comprehensively evaluate the overall quality of the structural arrangement (Figure 4B). The analysis showed that 87.1% of residues were in the most favored regions, 10.2% in additional allowed regions, 1.2% in generously allowed regions, and 1.6% in disallowed regions.
Figure 3. Prediction of MEV-Fc secondary and tertiary structures. (A) The prediction of MEV-Fc secondary structure. (B) The 3D model showcases the MEV-Fc tertiary structure, providing both front view and back view perspectives.
Figure 4. (A) Z-score evaluation results: the x-axis represents the number of amino acids in the protein, while the y-axis represents the score. The figure displays regions marked by blue and gray spots, which indicate the expected scoring range for proteins. The black spots represent the target proteins. (B) Ramachandran plot: the plot illustrates the distribution of residues based on their conformational angles.
In conclusion, the ERRAT server indicated an overall quality factor of 86.531, surpassing the generally accepted threshold of 50 for a good model. Therefore, we can confidently consider the tertiary structure of MEV-Fc as a reliable and accurate model.
Using the ElliPro server, we identified a comprehensive set of 311 residues that make up discontinuous B cell epitopes (Supplementary Table 6). These epitopes had scores ranging from 0.524 to 0.854. Additionally, our prediction revealed 18 consecutive B cell epitopes (Supplementary Table 7), which varied in size from 4 to 42 residues, with scores ranging from 0.512 to 0.832.
We used a powerful tool called ClusPro 2.0 to understand how our vaccine interacts with TLR2 and TLR4, two important proteins. When we examined how the vaccine connects with TLR2, we got 10 different models. We found that one model stood out, showing 11 hydrogen bonds and 5 salt-bridge interactions (Figure 5; Supplementary Table 8). The strength of the bond, called binding free energy, was −10.4 kcal/mol, indicating a strong connection between MEV-Fc and TLR2. To better understand this interaction, we used Ligplot+ to create a clear 2D picture of how they bond (Figure 6).
Figure 5. (A) Outcome plots derived from the ClusPro molecular docking of the vaccine structures (cyan) and TLR2 receptors (red). (B) Analysis of interactions within the MEV-Fc-TLR2 complex and generation of their 3D images utilizing the PyMol visualization tool.
Figure 6. Further analysis of the interactions within the MEV-Fc-TLR2 complex and generation of their two-dimensional images using the Ligplot+ visualization tool.
In a similar way, we looked at how our vaccine interacts with TLR4. We also got 10 different models. One model showed an impressive 27 hydrogen bonds and 5 salt-bridge interactions (Figure 7; Supplementary Table 9). The strength of this bond, in terms of binding free energy, was even higher at −18.8 kcal/mol, suggesting a very strong link between MEV-Fc and TLR4. We used Ligplot+ again to create a clear 2D picture of this complex bond (Figure 8).
Figure 7. (A) Result plots generated from the molecular docking of Cluspro, displaying the vaccine structures in cyan and TLR4 receptors in red. (B) Analysis of the MEV-Fc-TLR4 complex interactions and generation of their 3D images using the PyMol visualization tool.
Figure 8. Analysis of the MEV-Fc-TLR4 complex interactions using the Ligplot+ visualization tool, resulting in two-dimensional images of the interactions.
We used a tool called IModS to simulate how the molecules in our vaccine move and behave. In Figures 9 A-F, we show the results of this simulation. In Figure A, we can see how our vaccine (MEV-Fc) behaves when it is connected to TLR2. We used a method called normal mode analysis (NMA) to understand how flexible the proteins are. Figure B shows specific values related to this analysis for the MEV-Fc-TLR2 complex. In Figure C, variance plots showed a cumulative or individual variance of MEV-Fc-TLR2 complex with green or purple, respectively. In Figure D, we compare the actual movement (B factor) with what we predicted through NMA. Figure E displays a map that highlights how different parts of the complex move together. The red areas show significant motions happening together. Lastly, Figure F provides a diagram showing how the parts of the docked protein complexes are interconnected, like springs with varying stiffness. Darker areas indicate stiffer connections. Similar results were obtained for TLR4 (Supplementary Figure 1).
Figure 9. Molecular dynamics simulation results of MEV-Fc-TLR2. (A) Deformability analysis. (B) Eigenvalues of the simulation. (C) Variance plots, indicating individual (red) and cumulative (green) variances. (D) B-factor comparison. (E) Covariance map showing correlated (red), uncorrelated (white), and anticorrelated (blue)movements. (F) Elastic network representation, with darker gray areas indicating higher rigidity.
We used a tool called C-ImmSim to predict how the immune system in mice would respond to the MEV-Fc vaccine. Here are the key findings (Figure 10): (1) Antibody Levels: The results showed that the levels of IgM and IgG antibodies increased progressively after three rounds of vaccine injections (Figure 10A). This suggests that the vaccine triggered an antibody response in mice. (2) B-Cells: B-cells, which are responsible for producing antibodies, became more active with each vaccine injection, reaching their highest level after the final injection (Figure 10B). (3) Helper T Cells (TH-Cells): Both the total and memory populations of helper T cells (TH-cells) increased significantly (Figure 10C). The active TH-cell population also expanded robustly, reaching its peak after the third immunization (Figure 10D). (4) Cytotoxic T Cells (TC Cells): The count of active cytotoxic T lymphocytes (TC cells) increased gradually after each immunization injection (Figure 10E). (5) Cytokines: There was a notable increase in the levels of interferon-γ and IL-2, which are immune system signaling molecules, in response to antigen stimulation (Figure 10F). These findings suggest that the MEV-Fc vaccine could elicit a strong and diverse immune response in mice, involving antibodies, B-cells, helper T cells, cytotoxic T cells, and cytokines.
Figure 10. Immune simulation. (A) Levels of immunoglobulins in different states after antigen stimulation. (B) Distribution of B-cell subtypes in different states after antigen stimulation. (C) Count of CD4 T-helper lymphocytes after antigen stimulation. (D) Distribution of CD4 T-helper lymphocytes divided by activation status after antigen stimulation. (E) Count of CD8 T-cytotoxic lymphocytes divided by activation status. (F) Production of multiple cytokines following antigen stimulation.
Codon optimization is a technique used to improve the way a particular genetic sequence is expressed by enhancing translational efficiency (47). In this context, we optimized the codon usage in the MEV-Fc construct sequence using a tool called JCat. This optimization resulted in a Codon Adaptation Index (CAI) value of 1, and the GC content was measured at 56.99%, which falls within the desirable range. These findings suggest that the optimized sequence is likely to be efficiently expressed in the E. coli expression system. You can see the result in (Figure 11), which shows the expression vector pET 28a(+) with the inserted fragment of the multi-epitope vaccines.
Figure 11. In silico cloning. The multi-epitope vaccine sequence (highlighted in pink) was successfully inserted into the pET28a (+) expression vector at the HindIII and BamHI restriction endonuclease cleavage sites.
Brucellosis is a complex disease that poses a significant health risk to both humans and livestock (48). Vaccines are considered the most cost-effective way to prevent diseases caused by infectious agents (49). Subunit vaccines, in particular, show promise due to their safety, non-infectious nature, inability to revert to a virulent form, and precise control over desired effects (50). However, developing a safe and effective subunit vaccine against Brucella for use in humans and animals has been challenging (51, 52). Advances in bioinformatics, structural biology, and computational tools have transformed vaccine design (53). Bioinformatics has been used extensively to predict and design vaccines for various pathogens, including bacteria, viruses, fungi, and cancer (54).
In this context, we focused on Brucella abortus proteins OMP16, OMP19, OMP25, and L7/L12, which are known for their strong immunogenicity and potential to induce immune protection. We developed a multi-epitope vaccine based on these proteins and confirmed its immunogenicity. This vaccine has the potential to provide broad protection against Brucella infection.
Brucella, an intracellular and facultative intracellular parasite, has the ability to replicate within specialized or nonprofessional phagocytes (55). It has been demonstrated that cell-mediated immunity, particularly involving macrophages and T cells, plays a crucial role in immunoprotection against Brucella and other intracellular bacterial pathogens (56). B lymphocytes, are pivotal components of humoral immunity, producing antigen-specific antibodies that play a critical role in eliminating Brucella infection (57, 58). Therefore, an ideal anti-Brucella vaccine should encompass both T-cell and B-cell epitopes. In our study, we employed epitope prediction techniques to identify CD4+ and CD8+ short peptide sequences within the antigen, targeting both CTL and HTL epitopes (59). B cell epitopes can be recognized and bind to antibodies (on the surface of B cells or free antibodies), stimulating the immune system’s response against pathogens (38). By incorporating B cell epitopes, the vaccine can more effectively activate B cells and induce humoral immunity. Consequently, utilizing the IEDB server, we identified 8 dominant CTL epitopes and 6 dominant HTL epitopes from the 4 proteins. Additionally, the ABCpred server was employed to identify 4 dominant linear B cell epitopes present in all four proteins.
The choice of a suitable linker is crucial when combining different components in a vaccine (60). It helps prevent unintended interactions and improves how the vaccine is processed and presented to the immune system. In our vaccine design, we added the Fc sequence to the vaccine to address the issue of the vaccine’s short lifespan and prevent its breakdown in the body. Fc fragment fusion proteins have been studied as potential vaccines or treatments for various diseases like influenza, tuberculosis, and swine fever (61–63). While Fc fragments can help with antigen presentation by binding to receptors on immune cells, this may not be enough to activate certain immune cells and promote the desired immune response. To boost the immune response, we paired our vaccine with adjuvants and molecular agonists (64). We chose β-defensin as an adjuvant because it has antimicrobial properties and can modulate the immune system (65). Additionally, we included the PADRE sequence, which acts as an activator of certain immune receptors, to enhance the vaccine’s long-term effectiveness (66). With these modifications, we successfully created a peptide vaccine consisting of 600 amino acids.
We conducted further analysis to assess the physical and chemical properties, sensitization, and toxicity of MEV-Fc. Previous research suggests that vaccine proteins should have a molecular mass of less than 110 kDa (67). MEV-Fc has a predicted molecular weight of about 61.2 kDa, which falls within this desirable range. It also shows good antigenic properties, stability, hydrophilicity, solubility, and low sensitivity with an antigenicity score of 1.0342.
When we looked at its secondary structure, we found that MEV-Fc is composed of 15.33% alpha helix, 7.17% beta turns, and 58.33% random coils. These structural elements play a crucial role in how antibodies recognize the vaccine when the body is infected (40). The presence of beta turns and random coils in protein vaccines helps create antigenic epitopes (68).
We also generated a 3D model of the Brucella vaccine using the Robetta server. The Z score of −7.88 indicates that the protein structure prediction is accurate. In a Ramachandran plot analysis, over 98% of residues were located in favorable and permissive regions. This confirms the high accuracy and confidence of the predicted tertiary structure of MEV-Fc, making it a strong foundation for an effective vaccine model.
Protein–protein docking has become an important tool in the fields of immunoinformatics and pharmacological research. TLR2 and TLR4 are particularly important because they play a crucial role in generating specific T cells and are essential for the body’s defense against Brucella infection (69, 70). Therefore, we selected TLR2 and TLR4 as the receptors for molecular docking with MEV-Fc. Through molecular docking and subsequent molecular dynamics simulations, the stability of the MEV-Fc-TLR complex interactions was assessed. The docking results revealed the presence of an atomistic interaction interface between MEV-Fc and TLR2/TLR4, indicating a strong interaction between them, it indicates that MEV-Fc can activate the TLR2/TLR4 receptor signaling pathway and activate the immune response.
As mentioned earlier, Brucella is a type of bacteria that can survive and multiply within cells. To combat this, stimulating a T-cell-dependent immune response is crucial, as it hinders the bacteria’s growth within cells (71). B cells are also vital for the body’s defense by producing specific proteins that aid in immunity (59). The predictions regarding MEV-Fc showed that it effectively triggered both the innate and adaptive immune responses in mice. Notably, it increased various cell populations related to immunity and promoted the production of antibodies (Ig). The predicted results also indicated a significant rise in interferon-gamma and IL-2 levels. Interferon-gamma is important for fighting bacterial infections as it activates macrophages, aiding in the elimination of intracellular pathogens (72).
To ensure effective transcription and translation, we used JCAT software for codon optimization. The goal was to predict the best way to express the MEV-Fc construct in the E. coli K12 strain. The results showed a CAI value of 1 and a GC content of 56.99%. This suggests a high chance of successful and efficient expression in the E. coli expression system. Experimental verification of the immunogenicity and safety of the vaccine candidates designed and constructed in this study is required. The next step in this study will therefore be to perform high throughput cloning of the constructed vaccine candidates, expression of the purified recombinant proteins, immunization of the animals, and immunological assessments to ensure the true potential of the designed Brucella epitope vaccine.
In summary, this study introduces a novel vaccine candidate called MEV-Fc, designed specifically to prevent brucellosis. MEV-Fc includes 8 CTL epitopes, 6 HTL epitopes, 4 B cell epitopes, along with adjuvants and Fc fragments. MEV-Fc shows strong antigenicity and immunogenicity, and it is safe with no sensitizing or toxic effects. Importantly, MEV-Fc has a strong affinity for both TLR2 and TLR4, indicating a stable interaction. Additionally, MEV-Fc triggers a robust innate and adaptive immune response, leading to increased levels of Th1-type cytokines like interferon-gamma and IL-2. These promising findings suggest that MEV-Fc could be an excellent vaccine candidate for preventing brucellosis. But in vivo studies and laboratory data are also required to confirm vaccine efficacy.
The datasets presented in this study can be found in online repositories. The names of the repository or repositories and accession number(s) can be found in the Supplementary material.
JS and JY presented the concept and edited the article. AW and YW presented the concept, analyzed the data, and wrote the manuscript. AA and ZX designed the figures and edited the Manuscript. DZ and KZ review the manuscript and contributed to the data analysis. All authors contributed to the article and approved the submitted version.
This research was supported by Program of Shihezi University International Science and Technology Cooperation Promotion (GJHZ202203).
The authors declare that the research was conducted in the absence of any commercial or financial relationships that could be construed as a potential conflict of interest.
All claims expressed in this article are solely those of the authors and do not necessarily represent those of their affiliated organizations, or those of the publisher, the editors and the reviewers. Any product that may be evaluated in this article, or claim that may be made by its manufacturer, is not guaranteed or endorsed by the publisher.
The Supplementary material for this article can be found online at: https://www.frontiersin.org/articles/10.3389/fvets.2023.1238634/full#supplementary-material
1. ^http://tools.iedb.org/mhci/
2. ^http://tools.iedb.org/mhcii/
3. ^https://webs.iiitd.edu.in/raghava/abcpred/ABC_submission.html
4. ^https://www.expasy.org/resources/protparam
5. ^http://scratch.proteomics.ics.uci.edu//
6. ^http://ddg-pharmfac.net/AllergenFP/
7. ^http://crdd.osdd.net/raghava/toxinpred/
8. ^https://saves.mbi.ucla.edu/
9. ^http://services.mbi.ucla.edu/ERRAT/
10. ^https://www.ncbi.nlm.nih.gov/structure/
11. ^https://cluspro.bu.edu/login.php?redir=/home.php
12. ^https://www.ebi.ac.uk/pdbe/pisa/
13. ^https://imods.iqfr.csic.es/
1. Byndloss, MX , and Tsolis, RM . Brucella spp. virulence factors and immunity. Ann Rev Anim Biosci. (2016) 4:111–27. doi: 10.1146/annurev-animal-021815-111326
2. Boschiroli, ML , Foulongne, V , and O'Callaghan, D . Brucellosis: a worldwide zoonosis. Curr Opin Microbiol. (2001) 4:58–64. doi: 10.1016/s1369-5274(00)00165-x
3. Celli, J . The changing nature of the Brucella-containing vacuole. Cell Microbiol. (2015) 17:951–8. doi: 10.1111/cmi.12452
4. Lalsiamthara, J , and Lee, JH . Brucella lipopolysaccharide reinforced Salmonella delivering Brucella immunogens protects mice against virulent challenge. Vet Microbiol. (2017) 205:84–91. doi: 10.1016/j.vetmic.2017.05.012
5. Godfroid, J , Scholz, HC , Barbier, T , Nicolas, C , Wattiau, P , Fretin, D, et al. Brucellosis at the animal/ecosystem/human interface at the beginning of the 21st century. Prev Vet Med. (2011) 102:118–31. doi: 10.1016/j.prevetmed.2011.04.007
6. Dorneles, EMS , Sriranganathan, N , and Lage, AP . Recent advances in Brucella abortus vaccines. Vet Res. (2015) 46:76. doi: 10.1186/s13567-015-0199-7
7. Avila-Calderón, ED , Lopez-Merino, A , Sriranganathan, N , Boyle, SM , and Contreras-Rodríguez, A . A history of the development of Brucella vaccines. Biomed Res Int. (2013) 2013:743509. doi: 10.1155/2013/743509
8. Tibor, A , Decelle, B , and Letesson, JJ . Outer membrane proteins Omp10, Omp16, and Omp19 of Brucella spp. are lipoproteins. Infect Immun. (1999) 67:4960–2. doi: 10.1128/iai.67.9.4960-4962.1999
9. Godlewska, R , Wiśniewska, K , Pietras, Z , and Jagusztyn-Krynicka, EK . Peptidoglycan-associated lipoprotein (pal) of gram-negative bacteria: function, structure, role in pathogenesis and potential application in immunoprophylaxis. FEMS Microbiol Lett. (2009) 298:1–11. doi: 10.1111/j.1574-6968.2009.01659.x
10. Alizadeh, H , Dezfulian, M , Rahnema, M , Fallah, J , and Esmaeili, D . Protection of BALB/c mice against pathogenic Brucella abortus and Brucella melitensis by vaccination with recombinant Omp16. Iran J Basic Med Sci. (2019) 22:1302–7. doi: 10.22038/ijbms.2019.36369.8665
11. Pasquevich, KA , Carabajal, MV , Guaimas, FF , Bruno, L , Roset, MS , Coria, LM, et al. Omp19 enables Brucella abortus to evade the antimicrobial activity from Host's proteolytic Defense system. Front Immunol. (2019) 10:1436. doi: 10.3389/fimmu.2019.01436
12. Barrionuevo, P , Cassataro, J , Delpino, MV , Zwerdling, A , Pasquevich, KA , García Samartino, C, et al. Brucella abortus inhibits major histocompatibility complex class II expression and antigen processing through interleukin-6 secretion via toll-like receptor 2. Infect Immun. (2007) 76:250–62. doi: 10.1128/iai.00949-07
13. Pasquevich, KA , Ibañez, AE , Coria, LM , García Samartino, C , Estein, SM , Zwerdling, A, et al. An oral vaccine based on U-Omp19 induces protection against B. abortus mucosal challenge by inducing an adaptive IL-17 immune response in mice. PLoS One. (2011) 6:e16203. doi: 10.1371/journal.pone.0016203
14. Cloeckaert, A , Vizcaíno, N , Paquet, J-Y , Bowden, RA , and Elzer, PH . Major outer membrane proteins of Brucella spp.: past, present and future. Vet Microbiol. (2002) 90:229–47. doi: 10.1016/s0378-1135(02)00211-0
15. Jubier-Maurin, V , Boigegrain, RA , Cloeckaert, A , Gross, A , Alvarez-Martinez, MT , Terraza, A, et al. Major outer membrane protein Omp25 of Brucella suis is involved in inhibition of tumor necrosis factor alpha production during infection of human macrophages. Infect Immun. (2001) 69:4823–30. doi: 10.1128/iai.69.8.4823-4830.2001
16. Commander, NJ , Spencer, SA , Wren, BW , and MacMillan, AP . The identification of two protective DNA vaccines from a panel of five plasmid constructs encoding Brucella melitensis 16M genes. Vaccine. (2006) 25:43–54. doi: 10.1016/j.vaccine.2006.07.046
17. Goel, D , and Bhatnagar, R . Intradermal immunization with outer membrane protein 25 protects Balb/c mice from virulent B. abortus 544. Mol Immunol. (2012) 51:159–68. doi: 10.1016/j.molimm.2012.02.126
18. Golshani, M , Rafati, S , Siadat, SD , Nejati-Moheimani, M , Shahcheraghi, F , Arsang, A, et al. Improved immunogenicity and protective efficacy of a divalent DNA vaccine encoding Brucella L7/L12-truncated Omp31 fusion protein by a DNA priming and protein boosting regimen. Mol Immunol. (2015) 66:384–91. doi: 10.1016/j.molimm.2015.04.015
19. Oliveira, SC , Zhu, Y , and Splitter, GA . Recombinant L7/L12 ribosomal protein and gamma-irradiated Brucella abortus induce a T-helper 1 subset response from murine CD4+ T cells. Immunology. (1994) 83:659–64.
20. Oliveira, SC , and Splitter, GA . Immunization of mice with recombinant L7/L12 ribosomal protein confers protection against Brucella abortus infection. Vaccine. (1996) 14:959–62. doi: 10.1016/0264-410x(96)00018-7
21. Jafari, R , Zolbanin, NM , Rafatpanah, H , Majidi, J , and Kazemi, T . Fc-fusion proteins in therapy: an updated view. Curr Med Chem. (2017) 24:1228–37. doi: 10.2174/0929867324666170113112759
22. Kontermann, RE . Strategies for extended serum half-life of protein therapeutics. Curr Opin Biotechnol. (2011) 22:868–76. doi: 10.1016/j.copbio.2011.06.012
23. Ye, L , Zeng, R , Bai, Y , Roopenian, DC , and Zhu, X . Efficient mucosal vaccination mediated by the neonatal fc receptor. Nat Biotechnol. (2011) 29:158–63. doi: 10.1038/nbt.1742
24. Alleva, DG , Delpero, AR , Scully, MM , Murikipudi, S , Ragupathy, R , Greaves, EK, et al. Development of an IgG-fc fusion COVID-19 subunit vaccine, AKS-452. Vaccine. (2021) 39:6601–13. doi: 10.1016/j.vaccine.2021.09.077
25. Doytchinova, IA , and Flower, DR . VaxiJen: a server for prediction of protective antigens, tumour antigens and subunit vaccines. BMC Bioinfo. (2007) 8:4. doi: 10.1186/1471-2105-8-4
26. Kim, Y , Ponomarenko, J , Zhu, Z , Tamang, D , Wang, P , Greenbaum, J, et al. Immune epitope database analysis resource. Nucleic Acids Res. (2012) 40:W525–30. doi: 10.1093/nar/gks438
27. Zhang, Q , Wang, P , Kim, Y , Haste-Andersen, P , Beaver, J , Bourne, PE, et al. Immune epitope database analysis resource (IEDB-AR). Nucleic Acids Res. (2008) 36:W513–8. doi: 10.1093/nar/gkn254
28. Dhanda, SK , Vir, P , and Raghava, GPS . Designing of interferon-gamma inducing MHC class-II binders. Biol Direct. (2013) 8:30. doi: 10.1186/1745-6150-8-30
29. Saha, S , and Raghava, GPS . Prediction of continuous B-cell epitopes in an antigen using recurrent neural network. Proteins: Struc, Func Bioinfo. (2006) 65:40–8. doi: 10.1002/prot.21078
30. Cuspoca, AF , Díaz, LL , Acosta, AF , Peñaloza, MK , Méndez, YR , Clavijo, DC, et al. An Immunoinformatics approach for SARS-CoV-2 in Latam populations and multi-epitope vaccine candidate directed towards the World’s population. Vaccine. (2021) 9:581. doi: 10.3390/vaccines9060581
31. Magnan, CN , Randall, A , and Baldi, P . SOLpro: accurate sequence-based prediction of protein solubility. Bioinformatics. (2009) 25:2200–7. doi: 10.1093/bioinformatics/btp386
32. Saha, S , and Raghava, GPS . AlgPred: prediction of allergenic proteins and mapping of IgE epitopes. Nucleic Acids Res. (2006) 34:W202–9. doi: 10.1093/nar/gkl343
33. Gupta, S , Kapoor, P , Chaudhary, K , Gautam, A , Kumar, R , Null, N, et al. In silico approach for predicting toxicity of peptides and proteins. PLoS One. (2013) 34:W202–9. doi: 10.1371/journal.pone.0073957
34. Baek, M , DiMaio, F , Anishchenko, I , Dauparas, J , Ovchinnikov, S , Lee, GR, et al. Accurate prediction of protein structures and interactions using a three-track neural network. Science. (2021) 373:871–6. doi: 10.1126/science.abj8754
35. Wiederstein, M , and Sippl, MJ . ProSA-web: interactive web service for the recognition of errors in three-dimensional structures of proteins. Nucleic Acids Res. (2007) 35:W407–10. doi: 10.1093/nar/gkm290
36. Laskowski, RA , MacArthur, MW , Moss, DS , and Thornton, JM . PROCHECK: a program to check the stereochemical quality of protein structures. J Appl Crystallogr. (1993) 26:283–91. doi: 10.1107/s0021889892009944
37. Colovos, C, Yeates TO . Verification of protein structures: patterns of nonbonded atomic interactions. Protein Sci. (1993) 2:1511–9. doi: 10.1002/pro.5560020916
38. Alghamdi, W , Attique, M , Alzahrani, E , Ullah, MZ , and Khan, YD . LBCEPred: a machine learning model to predict linear B-cell epitopes. Brief Bioinform. (2022) 23:bbac035. doi: 10.1093/bib/bbac035
39. Ponomarenko, J , Bui, H-H , Li, W , Fusseder, N , Bourne, PE , Sette, A, et al. ElliPro: a new structure-based tool for the prediction of antibody epitopes. BMC Bioinfo. (2008) 23:bbac035. doi: 10.1186/1471-2105-9-514
40. Cheng, P , Wang, L , and Gong, W . In silico analysis of peptide-based biomarkers for the diagnosis and prevention of latent tuberculosis infection. Front Microbiol. (2022) 13:947852. doi: 10.3389/fmicb.2022.947852
41. Kozakov, D , Hall, DR , Xia, B , Porter, KA , Padhorny, D , Yueh, C, et al. The ClusPro web server for protein-protein docking. Nat Protoc. (2017) 12:255–78. doi: 10.1038/nprot.2016.169
42. Krissinel, E , and Henrick, K . Inference of macromolecular assemblies from crystalline state. J Mol Biol. (2007) 372:774–97. doi: 10.1016/j.jmb.2007.05.022
43. Lopéz-Blanco, JR , Garzón, JI , and Chacón, P . iMod: multipurpose normal mode analysis in internal coordinates. Bioinformatics. (2011) 27:2843–50. doi: 10.1093/bioinformatics/btr497
44. Rapin, N , Lund, O , Bernaschi, M , and Castiglione, F . Computational immunology meets bioinformatics: the use of prediction tools for molecular binding in the simulation of the immune system. PLoS One. (2010) 5:e9862. doi: 10.1371/journal.pone.0009862
45. Castiglione, F , Mantile, F , De Berardinis, P , and Prisco, A . How the interval between prime and boost injection affects the immune response in a computational model of the immune system. Comput Math Methods Med. (2012) 2012:842329. doi: 10.1155/2012/842329
46. Grote, A , Hiller, K , Scheer, M , Munch, R , Nortemann, B , Hempel, DC, et al. JCat: a novel tool to adapt codon usage of a target gene to its potential expression host. Nucleic Acids Res. (2005) 33:W526–31. doi: 10.1093/nar/gki376
47. Qian, W , Yang, J-R , Pearson, NM , Maclean, C , and Zhang, J . Balanced codon usage optimizes eukaryotic translational efficiency. PLoS Genet. (2012) 8:e1002603. doi: 10.1371/journal.pgen.1002603
48. Yin, D , Bai, Q , Li, L , Xu, K , and Zhang, J . Study on immunogenicity and antigenicity of a novel brucella multiepitope recombined protein. Biochem Biophys Res Commun. (2021) 540:37–41. doi: 10.1016/j.bbrc.2020.12.098
49. Greenwood, B . The contribution of vaccination to global health: past, present and future. Philosop Trans Royal Society B: Biolog Sci. (2014) 369:20130433. doi: 10.1098/rstb.2013.0433
50. Ficht, TA , Kahl-McDonagh, MM , and Arenas-Gamboa, AM . Rice-Ficht AC Brucellosis: the case for live, attenuated vaccines, 27. Vaccine. (2009) 27:D40–3. doi: 10.1016/j.vaccine.2009.08.058
51. Hou, H , Liu, X , and Peng, Q . The advances in brucellosis vaccines. Vaccine. (2019) 37:3981–8. doi: 10.1016/j.vaccine.2019.05.084
52. Moyle, PM , and Toth, I . Modern subunit vaccines: development, components, and research opportunities. ChemMedChem. (2013) 8:360–76. doi: 10.1002/cmdc.201200487
53. Kardani, K , Bolhassani, A , and Namvar, A . An overview of in silico vaccine design against different pathogens and cancer. Expert Rev Vaccines. (2020) 19:699–726. doi: 10.1080/14760584.2020.1794832
54. Raoufi, E , Hemmati, M , Eftekhari, S , Khaksaran, K , Mahmodi, Z , Farajollahi, MM, et al. Epitope prediction by novel Immunoinformatics approach: a state-of-the-art review. Int J Peptide Res Therapeutics. (2019) 26:1155–63. doi: 10.1007/s10989-019-09918-z
55. Detilleux, PG , Deyoe, BL , and Cheville, NF . Entry and intracellular localization of Brucella spp. in Vero cells: fluorescence and electron microscopy. Vet Pathol. (1990) 27:317–28. doi: 10.1177/030098589002700503
56. de Figueiredo, P , Ficht, TA , Rice-Ficht, A , Rossetti, CA , and Adams, LG . Pathogenesis and immunobiology of brucellosis: review of Brucella-host interactions. Am J Pathol. (2015) 185:1505–17. doi: 10.1016/j.ajpath.2015.03.003
57. Goenka, R , Guirnalda, PD , Black, SJ , and Baldwin, CL . B lymphocytes provide an infection niche for intracellular bacterium Brucella abortus. J Infect Dis. (2012) 206:91–8. doi: 10.1093/infdis/jis310
58. Goenka, R , Parent, MA , Elzer, PH , and Baldwin, CL . B cell-deficient mice display markedly enhanced resistance to the intracellular bacterium Brucella abortus. J Infect Dis. (2011) 203:1136–46. doi: 10.1093/infdis/jiq171
59. Rawal, K , Sinha, R , Abbasi, BA , Chaudhary, A , Nath, SK , Kumari, P, et al. Identification of vaccine targets in pathogens and design of a vaccine using computational approaches. Sci Rep. (2021) 11:17626. doi: 10.1038/s41598-021-96863-x
60. Sanches, RCO , Tiwari, S , Ferreira, LCG , Oliveira, FM , Lopes, MD , Passos, MJF, et al. Immunoinformatics design of multi-epitope peptide-based vaccine against Schistosoma mansoni using transmembrane proteins as a target. Front Immunol. (2021) 12:621706. doi: 10.3389/fimmu.2021.621706
61. Loureiro, S , Ren, J , Phapugrangkul, P , Colaco, CA , Bailey, CR , Shelton, H, et al. Adjuvant-free immunization with hemagglutinin-fc fusion proteins as an approach to influenza vaccines. J Virol. (2010) 85:3010–4. doi: 10.1128/jvi.01241-10
62. Soleimanpour, S , Farsiani, H , Mosavat, A , Ghazvini, K , Eydgahi, MRA , Sankian, M, et al. APC targeting enhances immunogenicity of a novel multistage fc-fusion tuberculosis vaccine in mice. Appl Microbiol Biotechnol. (2015) 99:10467–80. doi: 10.1007/s00253-015-6952-z
63. Li, J , Li, X , Ma, H , Ren, X , Hao, G , Zhang, H, et al. Efficient mucosal vaccination of a novel classical swine fever virus E2-fc fusion protein mediated by neonatal fc receptor. Vaccine. (2020) 38:4574–83. doi: 10.1016/j.vaccine.2020.05.013
64. Meza, B , Ascencio, F , Sierra-Beltrán, AP , Torres, J , and Angulo, C . A novel design of a multi-antigenic, multistage and multi-epitope vaccine against Helicobacter pylori: an in silico approach. Infect Genet Evol. (2017) 49:309–17. doi: 10.1016/j.meegid.2017.02.007
65. García-Valtanen, P , Martinez-Lopez, A , Ortega-Villaizan, M , Perez, L , Coll, JM , and Estepa, A . In addition to its antiviral and immunomodulatory properties, the zebrafish β-defensin 2 (zfBD2) is a potent viral DNA vaccine molecular adjuvant. Antivir Res. (2013) 101:136–47. doi: 10.1016/j.antiviral.2013.11.009
66. Solanki, V , and Tiwari, V . Subtractive proteomics to identify novel drug targets and reverse vaccinology for the development of chimeric vaccine against Acinetobacter baumannii. Sci Rep. (2018) 8:9044. doi: 10.1038/s41598-018-26689-7
67. Barh, D , Barve, N , Gupta, K , Chandra, S , Jain, N , Tiwari, S, et al. Exoproteome and secretome derived broad spectrum novel drug and vaccine candidates in Vibrio cholerae targeted by Piper betel derived compounds. PLoS One. (2013) 8:e52773. doi: 10.1371/journal.pone.0052773
68. Yu, M , Zhu, Y , Li, Y , Chen, Z , Li, Z , Wang, J, et al. Design of a Recombinant Multivalent Epitope Vaccine Based on SARS-CoV-2 and its variants in Immunoinformatics approaches. Front Immunol. (2022) 13:884433. doi: 10.3389/fimmu.2022.884433
69. Dominguez-Flores, A , Rodríguez López, GM , Soria-Castro, R , López-Santiago, R , Rodríguez-Cortés, O , Pérez-Tapia, SM, et al. Brucella abortus induces mast cell activation through TLR-2 and TLR-4. Microb Pathog. (2023) 176:106005. doi: 10.1016/j.micpath.2023.106005
70. Li, J-Y , Liu, Y , Gao, X-X , Gao, X , and Cai, H . TLR2 and TLR4 signaling pathways are required for recombinant Brucella abortus BCSP31-induced cytokine production, functional upregulation of mouse macrophages, and the Th1 immune response in vivo and in vitro. Cell Mol Immunol. (2023) 11:477–94. doi: 10.1038/cmi.2014.28
71. Skendros, P , Pappas, G , and Boura, P . Cell-mediated immunity in human brucellosis. Microbes Infect. (2010) 13:134–42. doi: 10.1016/j.micinf.2010.10.015
Keywords: Brucella , vaccine, epitope, immunoinformatics, IgG-fc
Citation: Wu A, Wang Y, Ali A, Xu Z, Zhang D, Zhumanov K, Sheng J and Yi J (2023) Design of a multi-epitope vaccine against brucellosis fused to IgG-fc by an immunoinformatics approach. Front. Vet. Sci. 10:1238634. doi: 10.3389/fvets.2023.1238634
Received: 12 June 2023; Accepted: 27 September 2023;
Published: 23 October 2023.
Edited by:
Xiaoyu Niu, North Carolina Agricultural and Technical State University, United StatesReviewed by:
Saurabh Gupta, GLA University, IndiaCopyright © 2023 Wu, Wang, Ali, Xu, Zhang, Zhumanov, Sheng and Yi. This is an open-access article distributed under the terms of the Creative Commons Attribution License (CC BY). The use, distribution or reproduction in other forums is permitted, provided the original author(s) and the copyright owner(s) are credited and that the original publication in this journal is cited, in accordance with accepted academic practice. No use, distribution or reproduction is permitted which does not comply with these terms.
*Correspondence: Jinliang Sheng, MTU3MjYyMTIxMUBxcS5jb20=; Jihai Yi, NzI0MDUwNjQ1QHFxLmNvbQ==
Disclaimer: All claims expressed in this article are solely those of the authors and do not necessarily represent those of their affiliated organizations, or those of the publisher, the editors and the reviewers. Any product that may be evaluated in this article or claim that may be made by its manufacturer is not guaranteed or endorsed by the publisher.
Research integrity at Frontiers
Learn more about the work of our research integrity team to safeguard the quality of each article we publish.