- 1College of Animal Science and Technology, Yangzhou University, Yangzhou, China
- 2Joint International Research Laboratory of Agriculture and Agri-Product Safety of Ministry of Education of China, Yangzhou University, Yangzhou, China
- 3International Joint Research Laboratory in Universities of Jiangsu Province of China for Domestic Animal Germplasm Resources and Genetic Improvement, Yangzhou University, Yangzhou, China
Sheep milk production is a dynamic and multifactorial trait regulated by diverse biological mechanisms. To improve the quality and production of sheep milk, it is necessary to understand the underlying non-coding transcriptomic mechanisms. In this study, ribonucleic acid-sequencing (RNA-seq) was used to profile the expression of microRNAs (miRNAs) and circular RNAs (circRNAs) in the sheep mammary gland at three key lactation time points (perinatal period, PP; early lactation, EL; and peak lactation, PL). A total of 2,369 novel circRNAs and 272 miRNAs were profiled, of which 348, 373, and 36 differentially expressed (DE) circRNAs and 30, 34, and 7 DE miRNAs were detected in the comparison of EL vs. PP, PL vs. PP, and PL vs. EL, respectively. A series of bioinformatics analyses including functional enrichment, machine learning prediction, and competing endogenous RNA (ceRNA) network analyses were conducted to identify subsets of the potential candidate miRNAs (e.g., oar_miR_148a, oar_miR_362, and oar_miR_432) and circRNAs (e.g., novel_circ_0011066, novel_circ_0010460, and novel_circ_0006589) involved in sheep mammary gland development. Taken together, this study offers a window into the dynamics of non-coding transcriptomes that occur during sheep lactation and may provide further insights into miRNA and circRNA that influence sheep mammary gland development.
Introduction
Sheep have been used to supply dairy products for centuries and rank fourth in global milk production (1). For these reasons, increasing the yield and quality of sheep milk is a desirable goal in the dairy sheep industry. However, milk production is a dynamic and multifactorial trait regulated by diverse molecular mechanisms and has a moderate heritability (2), which emphasizes the importance of precise selection for sheep milk production.
With the development of nucleic acid sequencing technologies, high-throughput sequencing has allowed for in-depth investigation of coding and non-coding transcripts in the lactating mammary gland of dairy species (3–5). In a previous study (6), we profiled the expression of messenger ribonucleic acids (mRNAs) and long non-coding RNAs (lncRNAs) in the sheep mammary gland during different lactation periods. However, due to the lack of information on non-coding RNAs unrelated to lncRNAs, such as microRNAs (miRNAs) and circular RNAs (circRNAs), the study only provided a partial view of the transcriptomic profile of the sheep mammary gland.
Circular RNA, a recently discovered non-coding RNA, has received considerable attention in mammary gland research. Xu et al. (7) identified a significantly greater number of circRNAs in the human mammary gland than in other tissues such as adrenal glands and thyroid, and similar results were found in sheep (3), cattle (8), and rats (9), indicating the vital roles of circRNAs in the mammary glands of various species. Moreover, some circRNAs that share miRNA recognition elements with miRNA target genes can enhance the expression of those genes by acting as miRNA sponges (10), initially modulating the cross talk between circRNA, miRNA, and its target genes in what is referred to as a “competitive endogenous RNA interaction” (ceRNA). Circ003429 enhances the expression of YAP1 by sponging miR-199a-3p during fatty acid synthesis in dairy goats (11). In dairy cows, circ11103 regulates milk fat metabolism via the miR-128/PPARGC1A axis (12). Collectively, these studies highlight the coordinated regulation of mammary gland development by circRNAs and miRNAs. However, the specific roles of these molecules remain largely unknown, especially in dairy sheep.
Numerous studies have well investigated the roles of circRNAs (8, 13) and miRNAs (14) in mammary gland development in various species, based on the universal characteristics of circRNAs and miRNAs and their potential ceRNA regulation in mammary glands. However, few reports have described dynamic RNA expression profiles or associated mechanisms in the sheep mammary gland during different lactation periods.
The mammary gland is a key organ related to lactation in mammals, and milk yield is largely controlled by mammary epithelial cells (MECs). From the beginning of pregnancy to the end of the perinatal period (PP), the mammary gland develops further and a rapid proliferation of MECs takes place (15). After parturition, MECs differentiate into secretory cells, regulate lactation, and remain stable during lactation. During peak lactation (PL) to late lactation, milk yield begins to decrease and apoptosis of MECs begins (16). Hence, we selected three key time points in the development of MECs to study the molecular mechanisms underlying sheep lactation: perinatal period, early lactation (EL), and PL. In this study, RNA-seq was used to profile the expression of miRNAs and circRNAs in the sheep mammary gland at three key lactation points. A series of bioinformatics and machine learning approaches were used to identify key circRNAs and miRNAs involved in mammary gland development, and a network of ceRNAs was constructed to better understand their roles in sheep lactation.
Materials and methods
Sample collection
All experimental sheep were supplied by Zhenjiang Wan Shan Hong Bian Agricultural Co., Ltd. (Zhenjiang, Jiangsu province, China). Detailed information on the experimental sheep can be found in our previous report (6).
Briefly, mammary gland biopsy tissues were collected from first-time pregnant Hu ewes with similar pregnancy dates and litter size, at three important lactation periods: 5 days before expected parturition (perinatal period, PP), 6 days postpartum (EL), and 25 days postpartum (PL). The collected mammary gland biopsy tissues were snap-frozen in liquid nitrogen and stored at −80°C before RNA extraction.
RNA extraction and sequencing
Ribonucleic acid (RNA) was extracted from the stored mammary gland biopsy tissues with the TRIzol reagent (Invitrogen, Carlsbad, CA, USA). The quality and integrity of the isolated RNA were examined with an RNA Nano 6000 Assay kit and Agilent 2100 Bioanalyzer, respectively.
MicroRNAs libraries were constructed with the NEB Next® Multiplex Small RNA Library Prep Set for Illumina® (NEB, Ipswich, MA, USA). circRNA libraries were constructed with the NEBNext® Ultra™ Directional RNA Library Prep kit for Illumina® (NEB). The miRNA and circRNA libraries were sequenced on the Illumina HiSeqTM 2500 platform (a single-end 50 bp strategy and a paired-end 150 bp strategy for miRNA and circRNA sequences, respectively) by Beijing Novogene Technology Co., Ltd. (Beijing, China).
Raw reads were generated in the FASTQ format, and reads containing poly-N, adapters, or poly A, T, C, or G and low-quality reads were removed by fastp (17). The clean reads obtained were mapped to the Ovis aries reference genome (Oar_v4.0) using Hisat2 (18). miRbase 20.0, as a known miRNA alignment, was used as a reference, miRDeep2 (19) was used to assemble miRNA transcripts, and srna-tools-cli was used to identify the potential miRNAs and draw their secondary structure. miREvo (20) and miRDeep2 were used to distinguish novel miRNA candidates from the transcripts by examining their secondary structure. circRNA candidates were distinguished from transcripts with find_circ (21) and CIRI2 (22), and detailed parameters used for the aforementioned software are provided in Supplementary Table S1.
Differentially expressed transcripts
The transcripts per million (TPM) parameter was used to estimate the expression levels of miRNA and circRNA transcripts. Multiple comparisons were used to identify differentially expressed (DE) circRNAs and miRNAs among the PP, EL, and PL groups using the DEseq R library (23). Transcripts were deemed significantly differentially expressed (DE) when the threshold of the adjusted p-value (adjusted p-value with the false discovery rate (FDR) approach) was < 0.05.
Gene ontology and Kyoto encyclopedia of genes and genomes enrichment
The target genes of DE miRNAs (predicted with miRanda and RNAhybird) and the parental genes of DE circRNAs were functionally annotated. The GOseq R library (24) and KO-Based Annotation System (KOBAS) (25) were used to determine gene ontology (GO) and Kyoto Encyclopedia of Genes and Genomes (KEGG) enrichment, respectively. Fisher's exact test with the FDR multiple test correction was used to assess statistical significance (p < 0.01), and the detailed parameters used for GOseq and KOBAS are provided in Supplementary Table S1.
Investigation of sheep lactation biomarkers using machine learning approaches
To identify non-coding RNA biomarkers for predicting sheep lactation, a two-step decision tree machine learning method entitled Random Forest-XGBoost (RX) was used. The R library randomForest (RF) and XGBoost were used for analysis. A detailed strategy for RX was described in our previous study (26).
In brief, a range of parameters (Ntree and mtry for RF and colsample and eta for XGBoost) was systematically evaluated by examining the out-of-bag (OOB) error rate to determine the derive minimum hyperparameter values required for RF and XGBoost.
To identify the biomarkers of sheep lactation, all samples were divided into three classes according to lactation stage (PP, EL, or PL). The miRNA and circRNA expression data sets were first offered to Random Forest to select variables (miRNAs and circRNAs) with positive values for their variable importance measures (VIMs). The positive-VIM subset of variables was then assessed with XGBoost. Similarly, XGBoost generated VIMs for the variables designated “Gain.”
The VIM value of an individual variable (circRNA or miRNA) denotes the relative contribution of the variable to each decision tree; the higher the VIM value, the more important the variable is to distinguish different classes (sheep lactation stages). Therefore, variables with a high “Gain” were prioritized as the potential non-coding RNA biomarkers of sheep lactation.
ceRNA network construction
Messenger RNA (mRNA) expression data sets for the sheep mammary gland during different lactation stages were obtained in our previous study and are available on: https://www.ncbi.nlm.nih.gov/, PRJNA759095. First, miRanda (27), and RNAhybrid (28) were used to predict miRNA-binding seed sequence sites and target mRNAs or circRNAs, detailed parameters for miRanda and RNAhybrid are provided in Supplementary Table S1. The miRNA–mRNA and miRNA–circRNA interaction pairs that shared the same miRNAs were then selected for subsequent analysis as candidate competing endogenous interactions. Pearson's correlation coefficients (PCCs) and corrected p-value (adjusted with Benjamini and Hochberg's approach) were calculated for the expression of the candidate circRNAs, miRNAs, and mRNAs. Finally, negatively regulated miRNA–mRNA/circRNA pairs with PCC < −0.75 and a corrected p < 0.05 were selected to establish ceRNA networks with the Cytoscape software (29).
Sequencing data validation
Five miRNAs and circRNAs were randomly chosen for the validation of the RNA-seq data, GAPDH and U6 were selected as the reference gene, and the primers were designed with Primer Premier 5 software (Supplementary Table S2).
Total RNA was extracted from the mammary gland biopsy samples with the TRIzol reagent according to the manufacturer's instructions. The extracted RNA was then reverse transcribed into complementary DNA (cDNA) with FastKing gDNA Dispelling RT SuperMix (Vazyme Biotech, Nanjing, Jiangsu, China), according to the manufacturer's instructions.
Real-time quantitative polymerase chain reaction (PCR) was performed in triplicate with cDNA as a template. The 2−ΔΔCt method (30) was used to calculate relative expression levels. The results were presented as fold changes in relative expression levels, using the GraphPad Prism 6 software.
Results
An overview of the sequencing data
In the miRNA libraries, the average rates of clean reads were 96.54% (EL), 96.03% (PL), and 96.41% (PP), and the average mapping rates were 95.71% (EL), 96.17% (PL), and 95.12% (PP). In the circRNA libraries, the average rates of clean reads were 97.23% (EL), 96.98% (PL), and 97.68% (PP), and the average mapping rates were 83.23% (EL), 80.03% (PL), and 81.71% (PP). Detailed information on the circRNA and miRNA libraries is given in Supplementary Table S3.
Based on the results of miREvo, miRDeep2, find_circ, and CIRI2, a total of 2,369 novel circRNAs and 272 miRNAs (140 annotated miRNAs and 132 novel miRNAs) were identified. The majority of circRNAs were 200–400 nt long, whereas the majority of miRNAs were 20–24 nt long. The average length of circRNAs was 326.69 nt, whereas miRNAs had an average length of 21.76 nt (Figure 1).
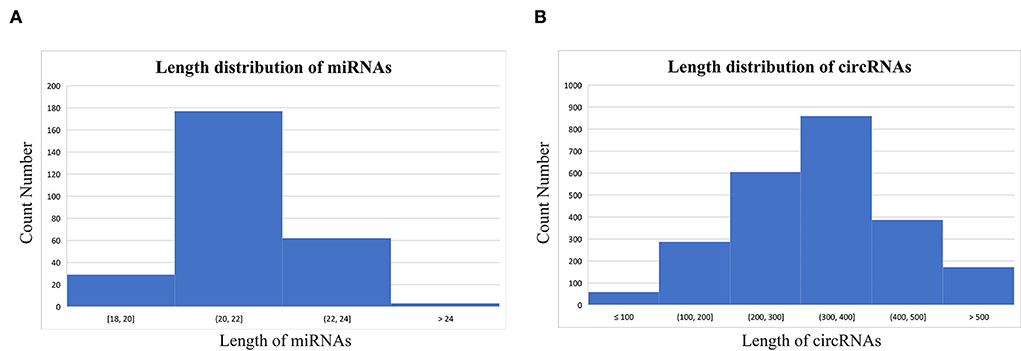
Figure 1. Length distribution of the distinguished micro ribonucleic acids (miRNAs) (A) and circular RNAs (circRNAs) (B).
Expression profiles of miRNAs and circRNAs
Transcripts per million was used to normalize the expression of miRNA and circRNA transcripts, based on which DEseq was used to identify DE miRNAs and DE circRNAs among the PP, EL, and PL groups. Detailed information on the miRNA and circRNA is given in Supplementary Table S4, and the results of DE analysis are given in Supplementary Table S5. Pearson's correction between the individual samples are shown in Supplementary Figure S1.
Of these DE miRNAs, 30, 34, and 7 were detected in the comparison of EL vs. PP (Figure 2A), PL vs. PP (Figure 2B), and PL vs. EL (Figure 2C), respectively. No miRNA was DE in all three comparisons (Figure 2D). Based on the adjusted p-value, the top three most DE miRNAs were oar_miR_370_3p, oar_miR_148a, and novel_miR_175 in the comparison of EL vs. PP. In the comparison of PL vs. PP, the top three most DE miRNAs were oar_miR_148a, oar_miR_370_3p, and oar_miR_99a. In the comparison of PL vs. EL, the top three most DE miRNAs were oar-miR-218a, oar-miR-3959-3p, and oar-miR-181a.
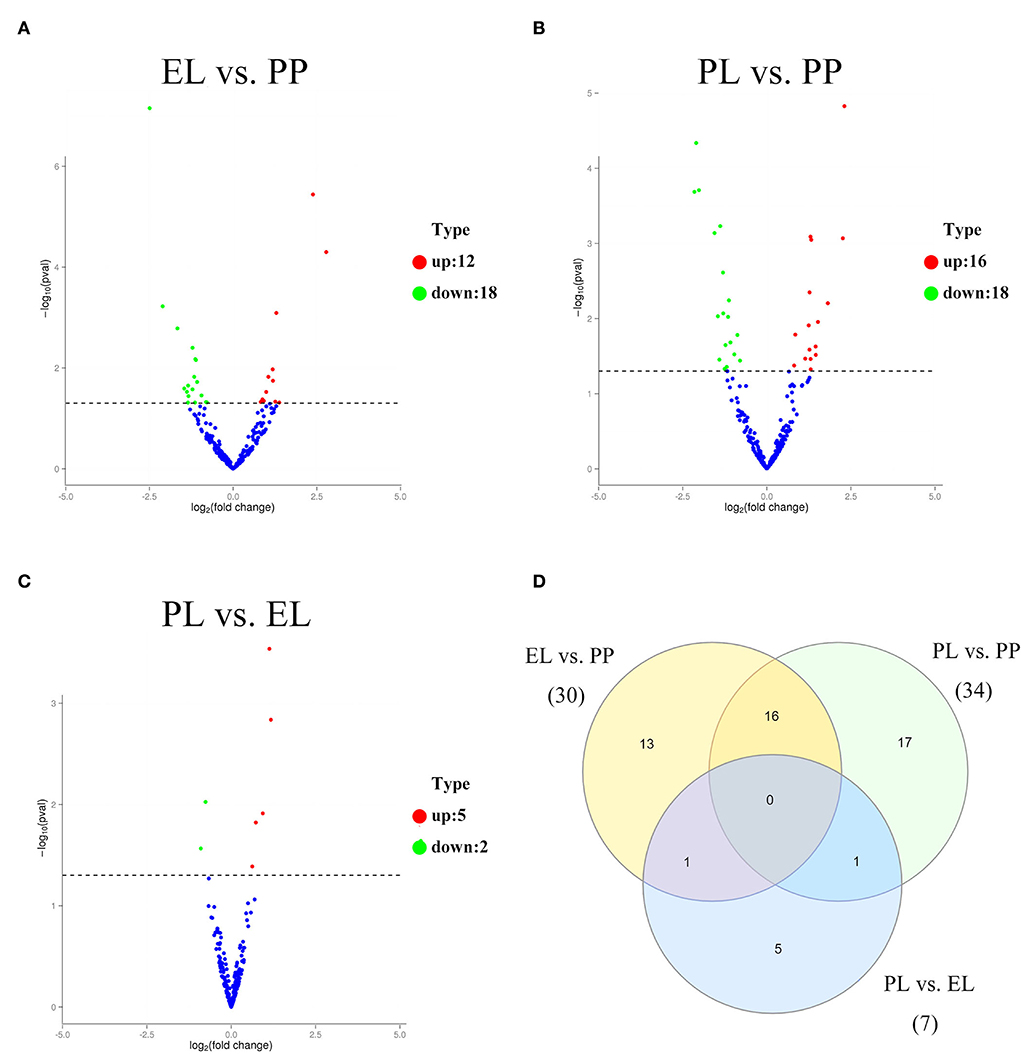
Figure 2. The volcano plot of differentially expressed (DE) miRNAs identified in early lactation (EL) vs. perinatal period (PP) (A), peak lactation (PL) vs. PP (B), and PL vs. EL comparisons (C). A Venn diagram of DE miRNAs in all three comparisons (D).
Of the DE circRNAs, 348, 373, and 36 DE circRNAs were detected in the comparison of EL vs. PP (Figure 3A), PL vs. PP (Figure 3B), and PL vs. EL (Figure 3C), respectively. A Venn diagram of DE circRNAs in different comparison groups showed that novel_circ_0010160 was DE in all three comparisons (Figure 3D).
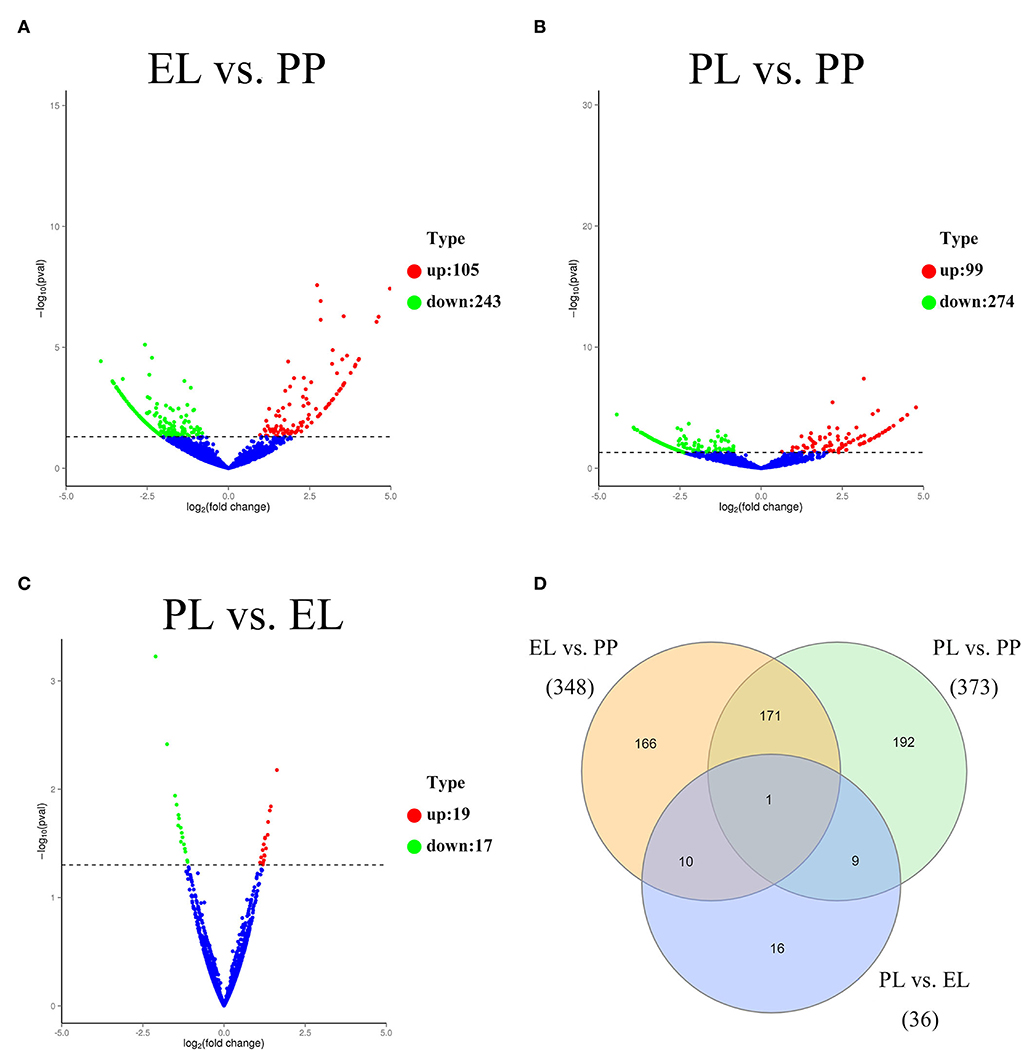
Figure 3. The volcano plot of DE circRNAs identified in the EL vs. PP (A), PL vs. PP (B), and PL vs. EL comparisons (C). A Venn diagram of DE circRNAs in all three comparisons (D).
Based on the adjusted p-value, the top three most DE circRNAs were novel_circ_0010649, novel_circ_0010160, and novel_circ_0001655 in the comparison of EL vs. PP. In the comparison of PL vs. PP, the top three most DE circRNAs were novel_circ_0010252, novel_circ_0010649, and novel_circ_0010642. In the comparison of PL vs. EL, the top three most DE circRNAs were novel_circ_0000578, novel_circ_0000885, and novel_circ_0001489.
Heat maps of DE miRNAs (Figure 4A) and DE circRNAs (Figure 4B) indicated clearly different non-coding transcriptomic profiles in the non-lactation period (PP) and the lactation period (EL and PL), but there was no obvious difference between the profiles of EL and PL.
GO and KEGG enrichment
Gene ontology and KEGG enrichment analyses of the target genes of DE miRNAs and the parental genes of DE circRNAs were performed. These results are given in Supplementary Table S6.
In the comparison of EL vs. PP, the target genes of the DE miRNAs were significantly enriched in 33 GO terms (Figure 5A), and the most enriched GO terms were virus maturation (GO: 0019075) in a biological process (BP), ESCRT I complex (GO: 0000813) in a cellular component (CC), and mannose-6-phosphate isomerase activity (GO: 0004476) in the molecular function (MF). The parental genes of the DE circRNAs were significantly enriched in 127 GO terms, and the most enriched GO terms were mitotic CC organization or biogenesis (GO: 0071840) in BP, membrane-bounded organelle (GO: 0043227) in MF, and chromatin binding (GO: 0003682) in MF (Figure 5C).
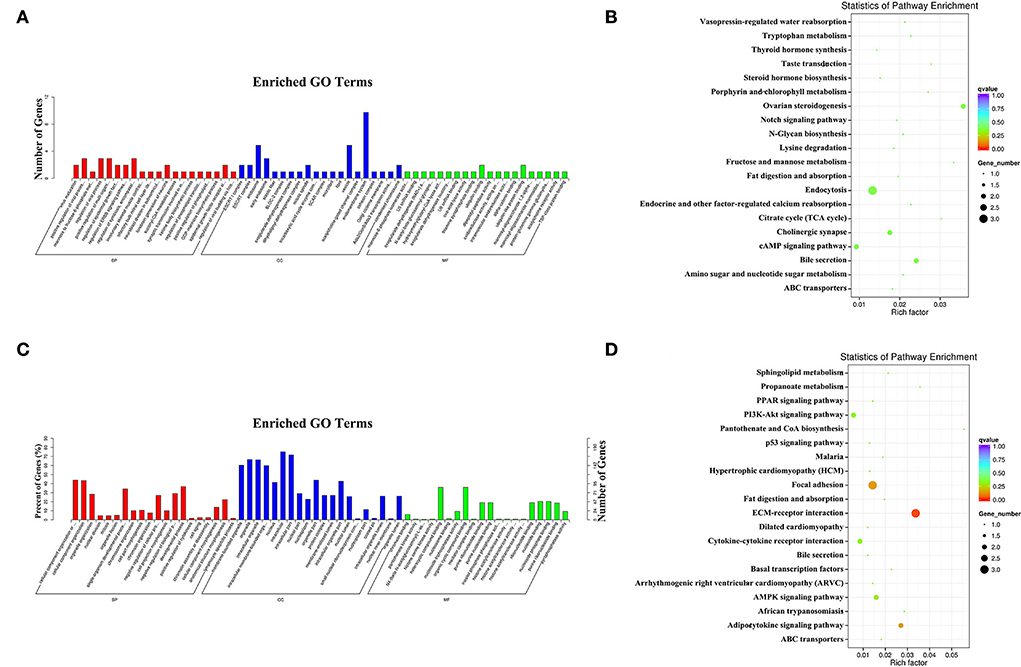
Figure 5. The most enriched gene ontology (GO) terms (A) and Kyoto Encyclopedia of Genes and Genomes (KEGG) pathways (B) of the target genes of the DE miRNAs identified in the EL vs. PP comparison. The most enriched GO terms (C) and KEGG pathways (D) of the parental genes of the DE circRNAs identified in the EL vs. PP comparison.
In the KEGG enrichment analysis, the target genes of the DE miRNAs were significantly enriched in four KEGG pathways (Figure 5B), the three most enriched KEGG pathways were ovarian steroidogenesis (oas04913), bile secretion (oas04976), and endocytosis (oas04144). The parental genes of the DE circRNAs were significantly enriched in five KEGG pathways (Figure 5D), the top three enriched KEGG pathways were ECM–receptor interaction (oas04512), focal adhesion (oas04510), and adipocytokine signaling pathway (oas04920).
In the PL vs. PP comparison, the target genes of the DE miRNAs were significantly enriched in 85 GO terms, and the most enriched GO terms were negative regulation of axon regeneration (GO: 0048681) in BP, anchored component of the external side of plasma membrane (GO: 0031362) in CC, and chondroitin sulfate binding (GO: 0035374) in MF (Figure 6A). The parental genes of the DE circRNAs were significantly enriched in 72 GO terms, and the most enriched GO terms were CC organization or biogenesis (GO: 0071840) in BP, membrane-bounded organelle (GO: 0043227) in CC, and N4-(beta-N-acetylglucosaminyl)-L-asparaginase activity (GO: 0003948) in MF (Figure 6C).
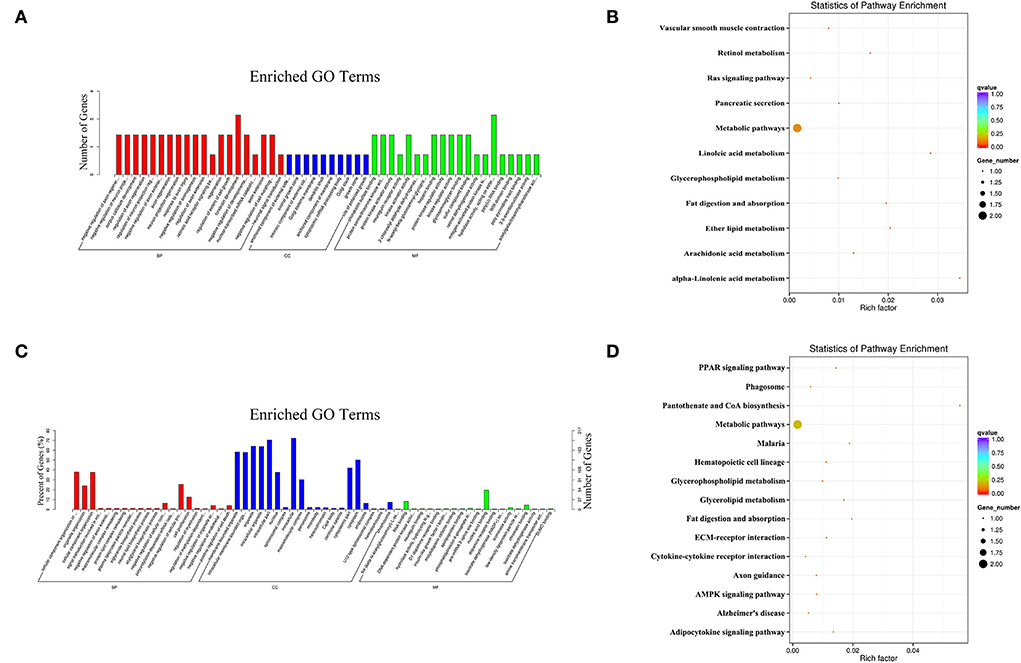
Figure 6. The most enriched GO terms (A) and KEGG pathways (B) of the target genes of the DE miRNAs identified in PL vs. PP comparison. The most enriched GO terms (C) and KEGG pathways (D) of the parental genes of the DE circRNAs identified in the PL vs. PP comparison.
In terms of KEGG enrichment, the target genes of the DE miRNAs were significantly enriched in eight KEGG pathways (Figure 6B), and the three most enriched KEGG pathways were alpha-linolenic acid metabolism (oas00592), linoleic acid metabolism (oas00591), and ether lipid metabolism (oas00565). The parental genes of the DE circRNAs were significantly enriched in six KEGG pathways (Figure 6D), and the three most enriched KEGG pathways were pantothenate and CoA biosynthesis (oas00770), fat digestion and absorption (oas04975), and malaria (oas05144).
In the PL vs. EL comparison, the target genes of the DE miRNAs were significantly enriched in 76 GO terms, and the most enriched GO terms were malonyl-CoA biosynthetic process (GO: 2001295) in BP, SMAD2–SMAD3 protein complex (GO: 0071144) in CC, and progesterone receptor binding (GO: 0033142) in MF (Figure 7A). The parental genes of the DE circRNAs were significantly enriched in 72 GO terms, and the most enriched GO terms were organelle organization (GO: 0006996) in BP, cytosol (GO: 0005829) in CC, and inositol 1,4,5 trisphosphate binding (GO: 0070679) in MF (Figure 7C).
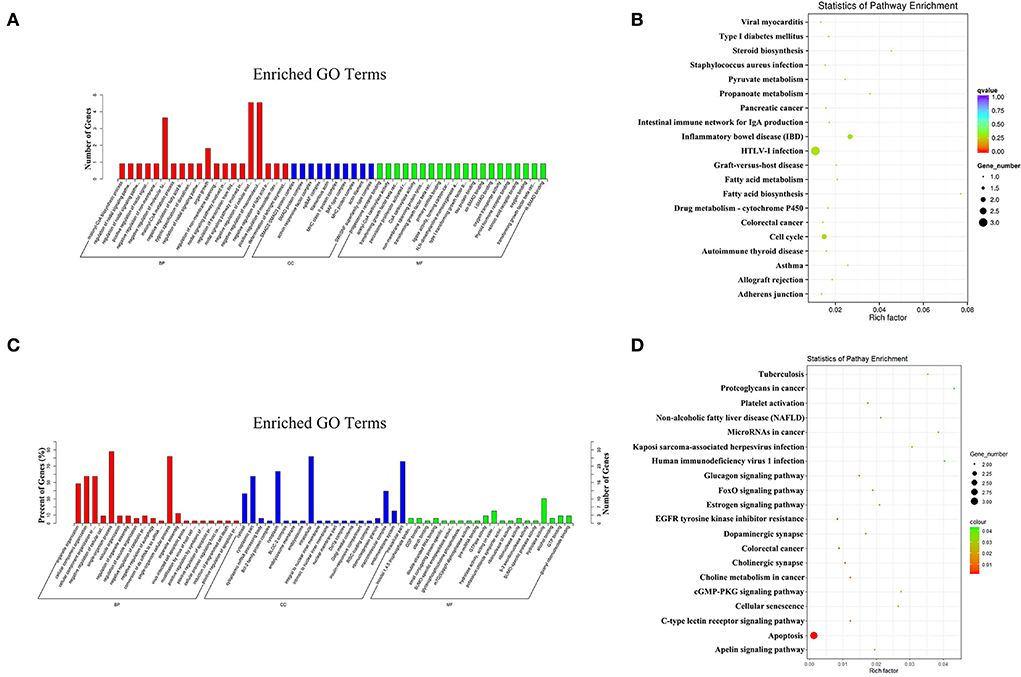
Figure 7. The most enriched GO terms (A) and KEGG pathways (B) of the target genes of the DE miRNAs identified in PL vs. EL comparison. The most enriched GO terms (C) and KEGG pathways (D) of the parental genes of the DE circRNAs identified in PL vs. EL comparison.
In terms of KEGG enrichment, the target genes of the DE miRNAs were significantly enriched in five KEGG pathways (Figure 7B), and the three most enriched KEGG pathways were inflammatory bowel disease (IBD, oas05321), HTLV-I infection (oas05166), and fatty acid biosynthesis (oas00061). Non KEGG pathway was significantly enriched for the parental genes of the DE circRNAs (Figure 7D), the three most enriched KEGG pathways were apoptosis (oas04210), epidermal growth factor receptor (EGFR) tyrosine kinase inhibitor resistance (oas01521), and colorectal cancer (oas05210).
Identification of sheep lactation biomarkers using machine learning approaches
Parameters used in the present study was selected with a systematic evaluation of a range of hyperparameter values. The detailed parameter training results and biomarker identification results are given in Supplementary Table S7.
To identify miRNA biomarkers, 114 positive-VIM miRNAs were first selected with Random Forest, and then 38 of those miRNAs were further selected with XGBoost. Three miRNAs with the highest Gain values (Figure 8A) were oar_miR_362 (0.16), novel_miR_370 (0.13), and oar_miR_758_3p (0.08).
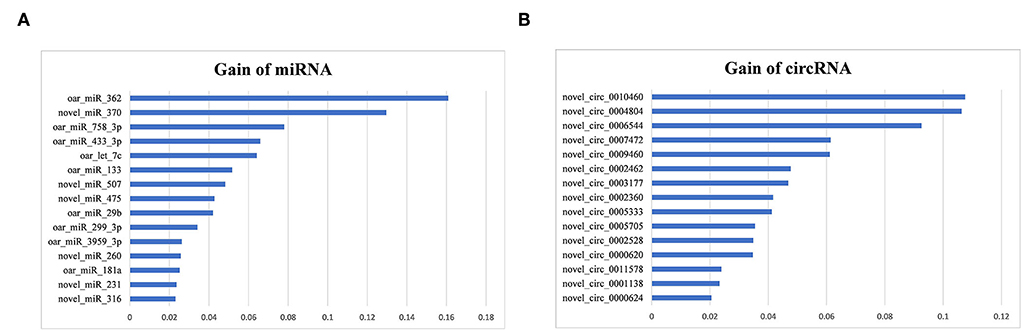
Figure 8. Gain values of the top miRNA (A) and circRNA (B) biomarkers of sheep lactation identified.
To identify circRNA biomarkers, 885 positive-VIM circRNAs were first selected with Random Forest, then 42 of those circRNAs were further selected with XGBoost. Three circRNAs with the highest Gain values (Figure 8B) were novel_circ_0010460 (0.11), novel_circ_0004804 (0.11), and novel_circ_0006544 (0.09).
ceRNA network
When we combined the results of miRanda, RNAhybrid, calculated PCC, and adjusted p-values, 130 miRNA–circRNA interactions and 68 miRNA–mRNA interactions were identified. ceRNA networks were then constructed based on the shared miRNAs, and we finally obtained 73 competing circRNA–miRNA–mRNA triplets containing 27 circRNAs, 15 miRNAs, and 36 mRNAs Figure 9. Within these, the most strongly connected candidates circRNA, miRNA, and mRNA were novel_circ_0006589 (13), oar_miR_432 (31), and PRADC1 (12), respectively. Detailed results are given in Supplementary Table S8.
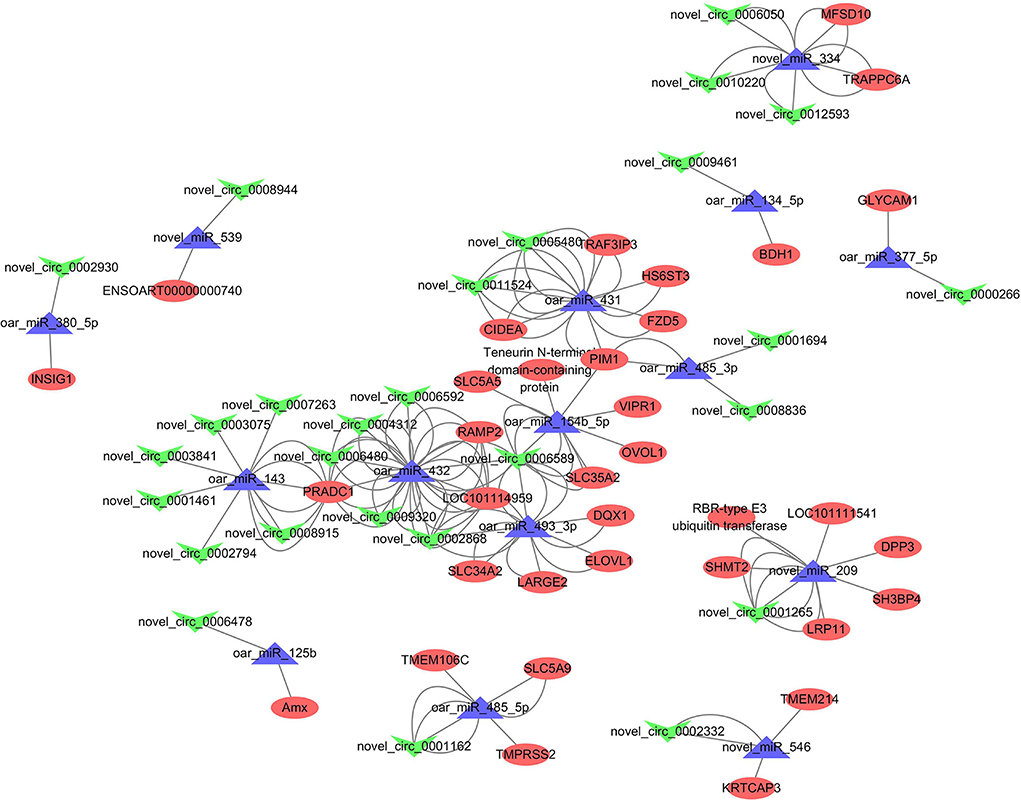
Figure 9. Competing endogenous RNA (ceRNA) networks, in which the “V” shape (green), triangle (purple), and circle (red) represent circRNAs, miRNAs, and messenger RNAs (mRNAs), respectively.
Validation of RNA-seq data
The expression levels of selected non-coding RNAs determined with RNA-seq and quantitative PCR (qPCR) are presented in Figure 10. The results show that the expression of both the selected circRNAs and miRNAs was similar in the RNA-seq and qPCR analyses, confirming the accuracy of our sequencing data.
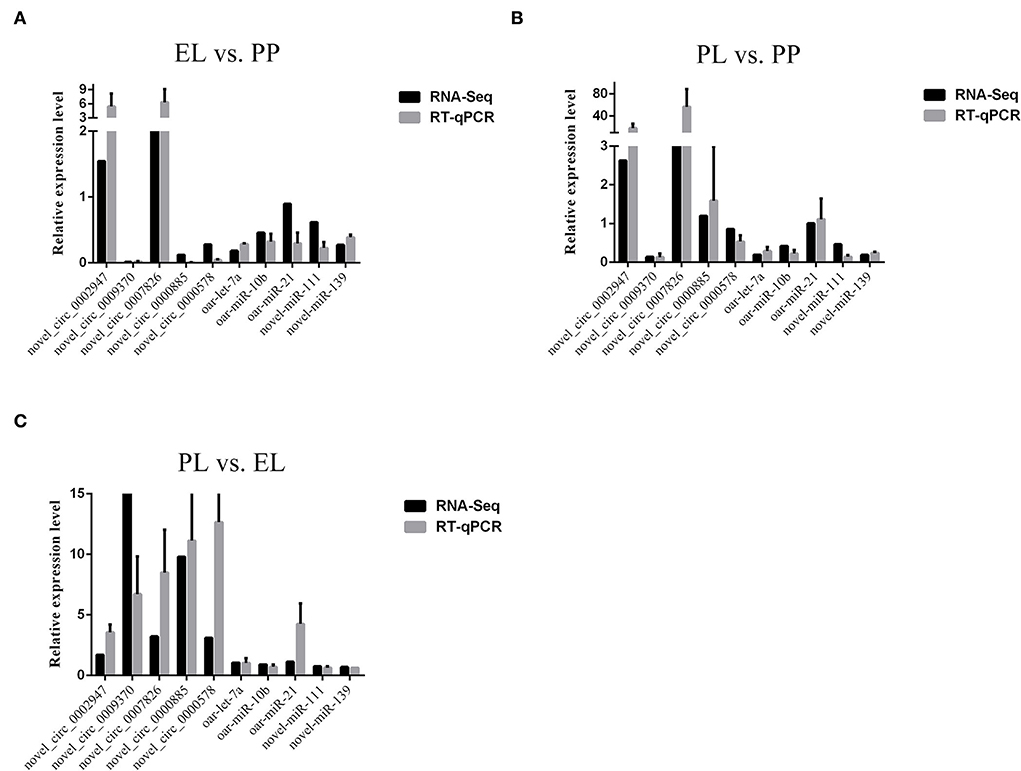
Figure 10. Comparisons of the results of RNA-sequencing (RNA-seq) and quantitative PCR (qPCR) analyses of selected non-coding RNAs in the EL vs. PP (A), PL vs. PP (B), and PL vs. EL comparisons (C).
Discussion
Lactation is a dynamic and multifactorial process in mammary gland development (32). At the transcription level, mammary gland development is regulated by a number of genes and non-coding transcripts, including DGAT (33), miR-143 (34), lncRNA Neat1 (35), and circ11103 (12). In our previous study (6), we systemically investigated the expression profiles of mRNAs and lncRNAs at three key points during mammary gland development in sheep (PP, EL, and PL), and detected a number of candidate genes and lncRNAs. However, the transcriptomic mechanisms that underlie mammary gland development are not fully understood, especially the roles of non-coding transcripts. In the present study, we investigated the expression profiles of miRNAs and circRNAs in PP, EL, and PL, to determine how miRNAs and circRNAs functions in mammary gland development, and their regulatory roles in controlling the expression of lactation-related genes.
Expression profiles of circRNAs and miRNAs
In total, 2,369 circRNAs and 272 miRNAs were annotated. Compared with a previous transcriptome study on dairy cattle (36) and other sheep breeds (31), which identified over 4,000 circRNAs in the mammary gland, remarkably fewer circRNAs were identified in the present study. A possible explanation for this discrepancy is that various animal models differ in their properties.
Among the annotated circRNAs, the most highly expressed circRNAs (according to the average TPM) were novel_circ_0011066, novel_circ_0011021, and novel_circ_0010252, whose parental genes are SLTM, USP3, and SLC39A8, respectively. It is noteworthy that SLTM, USP3, and SLC39A8 are closely related to the differentiation of mammary stem cells, mammary epithelial cell cycle, and mammary gland expansion (37–39). Moreover, Ahmad showed that circ_87295 from USP3 was highly expressed in the mammary gland of Kashmiri cattle (13), and Hao et al. showed that circ_011411 from SLC39A8 was highly expressed in the mammary gland of small-tailed Han sheep (3). Taken together, these data suggest that these circRNAs and their parental genes function similarly in mammary gland development across species. The most highly expressed of the annotated miRNAs were oar_miR_148a, oar_miR_143, and oar_miR_30a. A previously published study demonstrated that these miRNAs are involved in mammary gland development. For example, miR_148a regulates milk triacylglycerol synthesis in goat mammary epithelial cells (40), miR_143 regulates milk fat synthesis in bovine mammary epithelial cells (41), and the miR_30 family is involved in fatty acid metabolism in the mammary gland (42). Although the specific functions of these highly expressed circRNAs and miRNAs in sheep are still largely unclear, our results suggest that they act as principal regulators during mammary gland development, as they do in other species. Of course, in-depth work is still required to confirm this proposition.
When DEseq was applied, 30, 34, and 7 DE miRNAs, and 348, 373, and 36 DE circRNAs were detected in the comparison of EL vs. PP, PL vs. PP, and PL vs. EL, respectively. Consistent with our previous study on lncRNAs and mRNAs, clearly different expression profiles of both circRNAs and miRNAs were revealed between the non-lactation period (PP) and the lactation periods (EL and PL). Our results imply that the transcriptomic status of the mammary gland during lactation may be relatively stable, in terms of both coding and non-coding transcripts. It is noteworthy that novel_circ_0010160 was found to be DE in all three comparisons. Specifically, the expression level of novel_circ_0010160 rapidly decreased from the PP to the EL and then slowly increased from EL to PL. Previous studies on TBC1D14 (parental gene of novel_circ_0010160) demonstrated its important role in cancer cell autophagy (43, 44). This evidence suggests that novel_circ_0010160 may also function similarly in sheep MEC autophagy and would make a prime candidate for future research, especially in the non-lactation period.
When the non-lactation period and lactation periods were compared (EL vs. PP and PL vs. PP), 171 shared DE circRNAs and 16 shared DE miRNAs were identified. Of these, the most upregulated circRNA and miRNA (ranked by fold changes and adjusted p-value) were novel_circ_0011345 and oar_miR_148a, respectively. The parental gene of novel_circ_0011345 is feline leukemia virus subgroup C receptor-related protein 2 (FLVCR2). Little is known about the roles of this circRNA and its parental gene FLVCR2 in lactation, but the strong expression of novel_circ_0011345 in the lactation period suggests that it acts as a principal regulator during mammary gland development. As mentioned earlier, miR_148a has been shown to play an important role in mammary metabolism during lactation (45), and it is highly probable that miR_148a also acts as a key regulator of sheep milk production. The most downregulated circRNA was novel_circ_0005886, whose parental gene is zinc-finger 532 (ZNF532), a member of the C2H2-type zinc-finger family. Previous studies conducted in mice have suggested that the expression of circRNAs from ZNF532 is positively associated with cell apoptosis and pyroptosis (46). Considering the diverse roles of the zinc-finger family in mammary gland cell organization (47), it is therefore conceivable that novel_circ_0005886 also has certain effects on sheep milk production, probably by regulating the cellular BPs of sheep mammary epithelial cells. The most strongly downregulated miRNA, oar_miR_99a, showed markedly higher expression in PP than in lactation periods. Little is known about oar_miR_99a in mammary gland development, but a similar study conducted by Laurent et al. in the mammary glands of Prealpes-du-Sud ewes showed that miR_99a is also highly expressed in the non-lactation period (48). Collectively, these findings suggest that miR_99a is a prime candidate for future research on mammary gland development, particularly during the onset and early stage of lactation.
As noted in the abovementioned sections, small subsets of DE circRNAs and miRNAs were identified within the lactation periods. This finding raises the question of why sheep milk production varies in different lactation periods. The comparison of PL and EL identified DE circRNAs and miRNAs, which provide clues about the modification of the non-coding transcriptomic profile during lactation. The most upregulated (PL high) circRNA and miRNA were novel_circ_0001786 and oar_miR_218a, respectively. The parental gene for novel_circ_0001786 is LSM14A, and most research on these transcripts to date has focused on their roles in immunity (49, 50). However, their strong expression during PL, which corresponds to an intensive phase of proliferation in the mammary gland, suggests that the expression of novel_circ_0001786 and oar_miR_218a contribute to milk production in sheep, or at least some aspects of it.
The expression of the most downregulated transcripts, novel_circ_0006360 and oar-miR-181a, rapidly decreased from EL to PL. The parental gene of novel_circ_0006360 is PCM1, a key gene in both mammary gland development and breast cancer (51), which is related to cell proliferation and the cell cycle. Therefore, novel_circ_0006360 may act as a principal regulator in mammary gland development and may function similarly to its parental gene: PCM1. miR-181a, a star miRNA, has been shown to be associated with multiple mammary gland-related BPs, including milk fat biosynthesis (52), the heat stress response (53), and the mammary immune system (54). However, it is noteworthy that numerous studies have shown that the expression miR-181a is suppressed during the dry period relative to the lactation period in dairy cattle (55) and goats (56), which is inconsistent with our findings. Based on these findings, we speculate that miR-181a may play opposite roles as it does in other species, and may be involved in multiple functions that regulate mammary gland development.
Functional enrichment of DE miRNAs and DE circRNAs
To further define the biological functions of DE miRNAs and DE circRNAs, GO and KEGG functional enrichment analyses were conducted on the target genes of DE miRNAs and the parental genes of DE circRNAs.
In comparisons between PP and lactation periods, the GO annotation showed that the target genes of DE miRNAs and the parental genes of DE circRNAs were mainly involved in cellular progress, such as intracellular organelle, membrane-bounded organelle, and the endosome. In comparisons of lactation periods (PL vs. EL), the target genes of DE miRNAs and the parental genes of DE circRNAs were significantly enriched in metabolic processes in the mammary gland during lactation, such as negative regulation of single-organism process, cellular metabolic process, and macromolecule metabolic process. Consistent with previous findings in the dairy goat (57), these results suggest that the identified miRNAs and circRNAs differentially expressed in the non-lactation and lactation periods mainly contributed to diverse lactation-related cellular processes, especially in the organelles. Meanwhile, miRNAs and circRNAs differentially expressed in different lactation periods were found to regulate metabolism-related processes.
The KEGG enrichment analysis showed that the target genes of DE miRNAs and the parental genes of DE circRNAs identified in the comparison between PP and lactation periods were mainly enriched in ECM–receptor interactions and the AMPK signaling pathway. ECM–receptor interactions are important components of focal adhesions, which are involved in the migration of mammary epithelial cells during lactation (58). The AMPK signaling pathway is important in cellular energy sensing and in the regulation of glucose supply and utilization in the lactating mammary gland (59). Collectively, the DE miRNA and DE circRNAs identified here may act as regulators of diverse cellular processes and stimulate the development of mammary glands. Similar to the results of the GO enrichment analysis, the target genes of DE miRNAs and the parental genes of DE circRNAs when the lactation periods were compared were significantly enriched in metabolism- and cellular biology-related KEGG pathways, including fatty acid metabolism, the cell cycle, and apoptosis. Our results identified the regulatory roles of these DE miRNAs and DE circRNAs in the cell cycle and fatty acid metabolism, which may be responsible for differences in milk yield and milk components in the EL and PL periods.
Identification of sheep lactation biomarkers using machine learning approach
Previously, we compared the classification accuracy of several decision tree-based machine learning approaches (Random Forest, XGBoost, and RX) and DE transcript identification methods (edgeR and t-test) in subsets of transcriptomic data. The results showed that a method combining Random Forest and XGBoost (RX) outperformed the other four methods (Random Forest, XGBoost, t-test, and edgeR) with the highest classification accuracy and showed biological value in the prediction of multiple traits such as feed efficiency (26) and Escherichia coli infection (60).
For these reasons, RX was used to identify the biomarkers of sheep lactation in this study. Of all identified biomarkers, oar_miR_362 and novel_circ_0010460 outperformed all candidate transcripts with the highest “Gain” value, indicating the importance of oar_miR_362 and novel_circ_0010460 in distinguishing the different lactation periods. Although the specific roles of oar_miR_362 and novel_circ_0010460 in lactation are still unknown, their high gain values show their power to distinguish different lactation periods, and are also evidence for the critical roles of oar_miR_362 and novel_circ_0010460 in mammary gland development. The algorithm underlying the decision tree also demonstrates the strong interactivity between the top biomarkers and the other identified candidate biomarkers, indicating additional roles for oar_miR_362 and novel_circ_0010460 in sheep lactation. Of course, systematic functional verification is still required to clarify the biological roles of oar_miR_362 and novel_circ_0010460 in sheep lactation.
ceRNA network
In recent decades, many studies have demonstrated that circRNAs can function as competing endogenous RNAs, sharing miRNA recognition elements, and regulating target gene expression at different stages of mammary gland development (10, 36). To clarify the circRNA-related ceRNA crosstalk underlying mammary gland development, we constructed ceRNA networks of circRNA–miRNA–mRNA triplets.
A total of 73 competing circRNA–miRNA–mRNA triplets were identified, within which several key regulators were found, including miR-143 [lipid droplet formation, (41)], miR-125b [mammary inflammatory response (61)], SLC family members [cell cycle (62)] and OVOL1 [mammary epithelial–mesenchymal transition, (63)]. The most connected regulators in the ceRNA networks were novel_circ_0006589, oar_miR_432, and PRADC1. Previous studies have shown that these transcripts have been widely investigated in cancers (64, 65), whereas little is known about their functions in the mammary gland. Our findings provide basic evidence that these most strongly connected transcripts in the network are probably the key ceRNA regulators in sheep mammary gland development. Further research is required to confirm our hypothesis.
Conclusion
In conclusion, this study has characterized the expression profile of miRNAs and circRNAs in the mammary gland of Hu sheep during different lactation periods for the first time. By combining a DE analysis, functional enrichment, machine learning prediction, and a ceRNA network analysis, we identified subsets of candidate miRNAs (e.g., oar_miR_148a, oar_miR_362, and oar_miR_432) and circRNAs (e.g., novel_circ_0011066, novel_circ_0010460, and novel_circ_0006589) involved in the development of the sheep mammary gland. Consequently, our study provides a foundation for future research on the non-coding molecular mechanisms underlying sheep lactation.
Data availability statement
The datasets presented in this study can be found in online repositories. The names of the repository/repositories and accession number(s) can be found below: https://www.ncbi.nlm.nih.gov/, PRJNA853925 and https://www.ncbi.nlm.nih.gov/, PRJNA854211.
Ethics statement
The animal study was reviewed and approved by Experimental Animal Welfare and Ethical of Institute of Animal Science, Yangzhou University.
Author contributions
Conceptualization: WC, XL, XC, ZY, SW, and WS. Data curation and formal analysis: WC, XG, and XL. Supervision and funding acquisition: WS. Writing—original draft: WC. Writing—review and editing: WC and WS. All authors read and approved the final manuscript.
Funding
This work was supported by the National Natural Science Foundation of China-CGIAR (32061143036), National Natural Science Foundation of China (31872333 and 32172689), Major New Varieties of Agricultural Projects in Jiangsu province (PZCZ201739), The Projects of Domesticated Animals Platform of the Ministry of Science, Key Research and Development Plan (modern agriculture) in Jiangsu province (BE2018354), Jiangsu Agricultural Science and Technology Innovation Fund [CX182003], Jiangshu 333 Distinguished Talents Project Foundation [(2022) 2-323], and Jiangsu Postgraduate Research and Innovation Program (KYCX21_3260).
Acknowledgments
We thank Janine Miller, PhD, of Liwen Bianji (Edanz) (www.liwenbianji.cn) for editing the English text of a draft of this manuscript.
Conflict of interest
The authors declare that the research was conducted in the absence of any commercial or financial relationships that could be construed as a potential conflict of interest.
Publisher's note
All claims expressed in this article are solely those of the authors and do not necessarily represent those of their affiliated organizations, or those of the publisher, the editors and the reviewers. Any product that may be evaluated in this article, or claim that may be made by its manufacturer, is not guaranteed or endorsed by the publisher.
Supplementary material
The Supplementary Material for this article can be found online at: https://www.frontiersin.org/articles/10.3389/fvets.2022.983562/full#supplementary-material
Supplementary Figure S1. Pearson correction of miRNAs and circRNAs between different samples.
Supplementary Table S1. Detailed information of version and parameters of used software.
Supplementary Table S2. Sequence and designed primers of selected micro ribonucleic acids (miRNAs) and circular RNAs (circRNAs).
Supplementary Table S3. An overview of miRNA/circRNA sequencing data.
Supplementary Table S4. Detailed information of annotated miRNAs and circRNAs.
Supplementary Table S5. Differentially expressed (DE) miRNAs and circRNAs.
Supplementary Table S6. Detailed enrichment results of the target genes of the DE miRNAs and parental genes of the DE circRNAs.
Supplementary Table S7. Detailed results of RX.
Supplementary Table S8. Information of circRNA–miRNA–mRNA competing endogenous RNA (ceRNA) network.
References
1. Mohapatra A, Shinde AK, Singh R. Sheep milk: A pertinent functional food. Small Ruminant Res. (2019) 181:6–11. doi: 10.1016/j.smallrumres.2019.10.002
2. Inman JL, Robertson C, Mott JD, Bissell MJ. Mammary gland development: cell fate specification, stem cells and the microenvironment. Development. (2015) 142:1028–42. doi: 10.1242/dev.087643
3. Hao ZY, Zhou HT, Hickford JGH, Gong H, Wang JQ, Hu JQ, et al. Identification and characterization of circular RNA in lactating mammary glands from two breeds of sheep with different milk production profiles using RNA-Seq. Genomics. (2020) 112:2186–93. doi: 10.1016/j.ygeno.2019.12.014
4. Billa PA, Faulconnier Y, Ye T, Bes S, Pires J, Leroux C. Deep RNA-Seq reveals genetics and nutritional regulation of miRNomes in mammary gland of lactating Holstein and Montbeliarde cows. J Dairy Sci. (2019) 102:289–289. doi: 10.1186/s12864-019-5987-4
5. Crookenden MA, Moyes KM, Kuhn-Sherlock B, Lehnert L, Walker CG, Loor JJ, et al. Transcriptomic analysis of circulating neutrophils in metabolically stressed peripartal grazing dairy cows. J Dairy Sci. (2019) 102:7408–20. doi: 10.3168/jds.2019-16367
6. Chen WH, Lv XY, Wang Y, Zhang XJ, Wang SH, Hussain Z, et al. Transcriptional profiles of long non-coding RNA and mRNA in sheep mammary gland during lactation period. Front Genet. (2020) 11:946. doi: 10.3389/fgene.2020.00946
7. Xu TY, Wu J, Han P, Zhao ZM, Song XF. Circular RNA expression profiles and features in human tissues: a study using RNA-seq data. Bmc Genomics. (2017) 18:680. doi: 10.1186/s12864-017-4029-3
8. Zhang CL, Wu H, Wang YH, Zhu SQ, Liu JQ, Fang XT, et al. Circular RNA of cattle casein genes are highly expressed in bovine mammary gland. J Dairy Sci. (2016) 99:4750–60. doi: 10.3168/jds.2015-10381
9. Zhang CL, Wu H, Wang YH, Zhao L, Fang XT, Chen CF, et al. Expression Patterns of Circular RNAs from primary kinase transcripts in the mammary glands of lactating rats. J Breast Cancer. (2015) 18:235–41. doi: 10.4048/jbc.2015.18.3.235
10. Ma DX, Zhao Y, Yu S, Zhang HF, Cheng M, Cao HF Li Q, et al. CircRNA as CeRNA mediated by microRNA may be involved in goat lactation. Small Ruminant Res. (2019) 171:63–72. doi: 10.1016/j.smallrumres.2018.12.009
11. Jiao PX, Zhang MM, Wang ZW, Liang GG, Xie XL, Zhang YG, et al. Circ003429 regulates unsaturated fatty acid synthesis in the dairy goat mammary gland by interacting with miR-199a-3p, targeting the YAP1 Gene. Int J Molec Sci. (2022) 23:4068. doi: 10.3390/ijms23074068
12. Chen Z, Lu QY, Liang YS, Cui XS, Wang XL, Mao YJ, et al. Circ11103 interacts with miR-128/PPARGC1A to regulate milk fat metabolism in dairy cows. J Agr Food Chem. (2021) 69:4490–500. doi: 10.1021/acs.jafc.0c07018
13. Hussain MI, Shah RA, Ganai NA. Genome wide expression analysis of circular RNAs in mammary epithelial cells of cattle revealed difference in milk synthesis. Peerj. (2022) 10:e13029. doi: 10.7717/peerj.13029
14. Dysin AP, Barkova OY, Pozovnikova MV. The role of microRNAs in the mammary gland development, health, and function of cattle, goats, and sheep non-coding. Rna. (2021) 7:78. doi: 10.3390/ncrna7040078
15. Tsugami Y, Suzuki N, Kawahara M, Suzuki T, Nishimura T, Kobayashi K. Establishment of an in vitro culture model to study milk production and the blood-milk barrier with bovine mammary epithelial cells. Anim Sci J. (2020) 91:e13355. doi: 10.1111/asj.13355
16. Rios AC, Fu NY, Jamieson PR, Pal B, Whitehead L, Nicholas KR, et al. Essential role for a novel population of binucleated mammary epithelial cells in lactation. Nat Commun. (2016) 7:11400. doi: 10.1038/ncomms11400
17. Chen S, Zhou Y, Chen Y, Gu J. fastp: an ultra-fast all-in-one FASTQ preprocessor. Bioinformatics. (2018) 34:884–90. doi: 10.1093/bioinformatics/bty560
18. Kim D, Landmead B, Salzberg SL, HISAT. a fast spliced aligner with low memory requirements. Nat Methods. (2015) 12:357–U121. doi: 10.1038/nmeth.3317
19. Friedlander MR, Mackowiak SD Li N, Chen W, Rajewsky N. miRDeep2 accurately identifies known and hundreds of novel microRNA genes in seven animal clades. Nucleic Acids Res. (2012) 40:37–52. doi: 10.1093/nar/gkr688
20. Wen M, Shen Y, Shi SH, Tang T. miREvo: an integrative microRNA evolutionary analysis platform for next-generation sequencing experiments. Bmc Bioinformatics. (2012) 13:140. doi: 10.1186/1471-2105-13-140
21. Memczak S, Jens M, Elefsinioti A, Torti F, Krueger J, Rybak A, et al. Circular RNAs are a large class of animal RNAs with regulatory potency. Nature. (2013) 495:333–8. doi: 10.1038/nature11928
22. Gao Y, Wang JF, Zhao FQ. CIRI: an efficient and unbiased algorithm for de novo circular RNA identification. Genome Biol. (2015) 16:4. doi: 10.1186/s13059-014-0571-3
23. Wang LK, Feng ZX, Wang X, Wang XW, Zhang XG. DEGseq: an R package for identifying differentially expressed genes from RNA-seq data. Bioinformatics. (2010) 26:136–8. doi: 10.1093/bioinformatics/btp612
24. Young MD, Wakefield MJ, Smyth GK, Oshlack A. Gene ontology analysis for RNA-seq: accounting for selection bias. Genome Biol. (2010) 11:R14. doi: 10.1186/gb-2010-11-2-r14
25. Xie C, Mao XZ, Huang JJ, Ding Y, Wu JM, Dong S, et al. 2.0: a web server for annotation and identification of enriched pathways and diseases. Nucleic Acids Research. (2011) 39:W316–22. doi: 10.1093/nar/gkr483
26. Chen WH, Alexandre PA, Ribeiro G, Fukumasu H, Sun W, Reverter A, et al. Identification of predictor genes for feed efficiency in beef cattle by applying machine learning methods to multi-tissue transcriptome data. Front Genet. (2021) 12:619857. doi: 10.3389/fgene.2021.619857
27. Enright AJ, John B, Gaul U, Tuschl T, Sander C, Marks DS. MicroRNA targets in Drosophila. Genome Biol. (2004) 5:R1. doi: 10.1186/gb-2003-5-1-r1
28. Rehmsmeier M, Steffen P, Hochsmann M, Giegerich R. Fast and effective prediction of microRNA/target duplexes. Rna. (2004) 10:1507–17. doi: 10.1261/rna.5248604
29. Shannon P, Markiel A, Ozier O, Baliga NS, Wang JT, Ramage D, et al. Cytoscape: A software environment for integrated models of biomolecular interaction networks. Genome Res. (2003) 13:2498–504. doi: 10.1101/gr.1239303
30. Livak KJ, Schmittgen TD. Analysis of relative gene expression data using real-time quantitative PCR and the 2(T)(-Delta Delta C) method. Methods. (2001) 25:402–8. doi: 10.1006/meth.2001.1262
31. Wang J, Zhou H, Hickford JGH, Hao Z, Gong H, Hu J, et al. Identification and characterization of circular RNAs in mammary gland tissue from sheep at peak lactation and during the nonlactating period. J Dairy Sci. (2021) 104:2396–409. doi: 10.3168/jds.2020-18911
32. Paten AM, Duncan EJ, Pain SJ, Peterson SW, Kenyon PR, Blair HT, et al. Functional development of the adult ovine mammary gland-insights from gene expression profiling. Bmc Genomics. (2015) 16:748. doi: 10.1186/s12864-015-1947-9
33. Liu JJ, Wang ZQ, Li J, Li H, Yang LG. Genome-wide identification of Diacylglycerol Acyltransferases (DGAT) family genes influencing Milk production in Buffalo. Bmc Genetics. (2020) 21:26. doi: 10.1186/s12863-020-0832-y
34. Vailati-Riboni M, Farina G, Batistel F, Heiser A, Mitchell MD, Crookenden MA, et al. Far-off and close-up dry matter intake modulate indicators of immunometabolic adaptations to lactation in subcutaneous adipose tissue of pasture-based transition dairy cows. J Dairy Sci. (2017) 100:2334–50. doi: 10.3168/jds.2016-11790
35. Standaert L, Adriaens C, Radaelli E, Van Keymeulen A, Blanpain C, Hirose T, et al. The long noncoding RNA Neat1 is required for mammary gland development and lactation. Rna. (2014) 20:1844–9. doi: 10.1261/rna.047332.114
36. Gao QS, Wang HY, Guo ML, Arbab AAI, Nazar M, Li MX, et al. Identification and characterization of circular RNAs in mammary tissue from holstein cows at early lactation and non-lactation. Biomolecules. (2022) 12:478. doi: 10.3390/biom12030478
37. dos Santos CO, Rebbeck C, Rozhkova E, Valentine A, Samuels A, Kadiri LR, et al. Molecular hierarchy of mammary differentiation yields refined markers of mammary stem cells. P Natl Acad Sci USA. (2013) 110:7123–30. doi: 10.1073/pnas.1303919110
38. Wu YY, Qin JY Li FB, Yang CY Li Z, Zhou ZM, Zhang HL, Lie YX, et al. USP3 promotes breast cancer cell proliferation by deubiquitinating KLF5. J Biol Chem. (2019) 294:17837–47. doi: 10.1074/jbc.RA119.009102
39. Elsasser TH, Evock-Clover CM, Van Tassell CP, Sonstegard TS, Fernandes VM, Capuco AV. Effects of increased milking frequency on gene expression in the bovine mammary gland. Bmc Genomics. (2008) 9:362. doi: 10.1186/1471-2164-9-362
40. Chen Z, Luo J, Sun S, Cao DY, Shi HP, Loor JJ. miR-148a and miR-17-5p synergistically regulate milk TAG synthesis via PPARGC1A and PPARA in goat mammary epithelial cells. RNA Biol. (2017) 14:326–38. doi: 10.1080/15476286.2016.1276149
41. Wu ZQ, Wang YJ, Wang M, Yang WC. MiR-143 regulates milk fat synthesis by targeting Smad3 in bovine mammary epithelial cells. Animals-Basel. (2020) 10:1453. doi: 10.3390/ani10091453
42. Qiu HL, Ma LV, Luo J, Sun S, Kang K, Gou D, Loor JJ. miR-30e-5p and miR-15a synergistically regulate fatty acid metabolism in goat mammary epithelial cells via LRP6 and YAP1. Int J Molec Sci. (2016) 17:1909. doi: 10.3390/ijms17111909
43. Lu T, Li Y, Pan M, Yu D, Wang Z, Liu C, et al. TBC1D14 inhibits autophagy to suppress lymph node metastasis in head and neck squamous cell carcinoma by downregulating macrophage erythroblast attacher. Int J Biol Sci. (2022) 18:1795–812. doi: 10.7150/ijbs.68992
44. Lamb CA, Nuhlen S, Judith D, Frith D, Snijders AP, Behrends C, et al. TBC1D14 regulates autophagy via the TRAPP complex and ATG9 traffic. EMBO J. (2016) 35:281–301. doi: 10.15252/embj.201592695
45. Do DN Li R, Dudemaine PL, Ibeagha-Awemu EM. MicroRNA roles in signalling during lactation: an insight from differential expression, time course and pathway analyses of deep sequence data. Sci Rep. (2017) 7:44605. doi: 10.1038/srep44605
46. Jiang Q, Liu C, Li CP, Xu SS, Yao MD, Ge HM, et al. Circular RNA-ZNF532 regulates diabetes-induced retinal pericyte degeneration and vascular dysfunction. J Clin Invest. (2020) 130:3833–47. doi: 10.1172/JCI123353
47. McCormick NH, Hennigar SR, Kiselyov K, Kelleher SL. The biology of zinc transport in mammary epithelial cells: implications for mammary gland development, lactation, and involution. J Mammary Gland Biol. (2014) 19:59–71. doi: 10.1007/s10911-013-9314-4
48. Galio L, Droineau S, Yeboah P, Boudiaf H, Bouet S, Truchet S, et al. MicroRNA in the ovine mammary gland during early pregnancy: spatial and temporal expression of miR-21, miR-205, and miR-200. Physiol Genomics. (2013) 45:151–61. doi: 10.1152/physiolgenomics.00091.2012
49. Zhang L, Fu Y, Zhang R, Guan Y, Jiang N, Zheng N, et al. Nonstructural protein NSs hampers cellular antiviral response through LSm14A during severe fever with thrombocytopenia syndrome virus infection. J Immunol. (2021) 207:590–601. doi: 10.4049/jimmunol.2100148
50. Tan Y, Zhang W, Wu HY, Xia J, Zhang HB, Liu MW, et al. Effects of emodin on intestinal mucosal barrier by the upregulation of miR-218a-5p expression in rats with acute necrotizing pancreatitis. Int J Immunopathol Pharmacol. (2020) 34:2058738420941765. doi: 10.1177/2058738420941765
51. Tian YP, Chen BZ, Guan PF, Kang YJ, Lu ZX. A prognosis classifier for breast cancer based on conserved gene regulation between mammary gland development and tumorigenesis: a multiscale statistical model. PLoS ONE. (2013) 8:e60131. doi: 10.1371/journal.pone.0060131
52. Lian S, Guo JR, Nan XM, Ma L, Loor JJ, Bu DP. MicroRNA Bta-miR-181a regulates the biosynthesis of bovine milk fat by targeting ACSL1. J Dairy Sci. (2016) 99:3916–24. doi: 10.3168/jds.2015-10484
53. Zheng Y, Chen KL, Zheng XM Li HX, Wang GL. Identification and bioinformatics analysis of microRNAs associated with stressand immune response in serum of heat-stressed and normal Holstein cows. Cell Stress Chaperon. (2014) 19:973–81. doi: 10.1007/s12192-014-0521-8
54. Li ZX, Wang HL, Chen L, Wang LJ, Liu XL, Ru CX, et al. Identification and characterization of novel and differentially expressed microRNAs in peripheral blood from healthy and mastitis Holstein cattle by deep sequencing. Anim Genet. (2014) 45:20–7. doi: 10.1111/age.12096
55. Wang M, Moisa S, Khan MJ, Wang J, Bu D, Loor JJ. MicroRNA expression patterns in the bovine mammary gland are affected by stage of lactation. J Dairy Sci. (2012) 95:6529–35. doi: 10.3168/jds.2012-5748
56. Li Z, Lan X, Guo W, Sun J, Huang Y, Wang J, et al. Comparative transcriptome profiling of dairy goat microRNAs from dry period and peak lactation mammary gland tissues. PLoS ONE. (2012) 7:e52388. doi: 10.1371/journal.pone.0052388
57. Yu S, Zhao Y, Lai FN, Chu MQ, Hao YN, Feng YN, et al. LncRNA as ceRNAs may be involved in lactation process. Oncotarget. (2017) 8:98014–28. doi: 10.18632/oncotarget.20439
58. Ivanova E, Le Guillou S, Hue-Beauvais C, Le Provost F. Epigenetics: new insights into mammary gland biology. Genes-Basel. (2021) 12:231. doi: 10.3390/genes12020231
59. Heng JH, Chen JM, Chen F, Guan WT, Zhang SH. Current evidences and future perspectives for AMPK in the regulation of milk production and mammary gland biology. Front Cell Dev Biol. (2020) 8:530. doi: 10.3389/fcell.2020.00530
60. Chen WH, Lv XY, Zhang WB, Hu TY, Cao XK, Ren ZM, et al. Non-coding transcriptome provides novel insights into the Escherichia coli F17 susceptibility of sheep. Lamb Biology-Basel. (2022) 11:348. doi: 10.3390/biology11030348
61. Valmiki S, Ahuja V, Puri N, Paul J. miR-125b and miR-223 contribute to inflammation by targeting the key molecules of NF kappa B pathway. Front Med-Lausanne. (2020) 6:313. doi: 10.3389/fmed.2019.00313
62. Lesniewski LA, Seals DR, Walker AE, Henson GD, Blimline MW, Trott DW, et al. Dietary rapamycin supplementation reverses age-related vascular dysfunction and oxidative stress, while modulating nutrient-sensing, cell cycle, and senescence pathways. Aging Cell. (2017) 16:17–26. doi: 10.1111/acel.12524
63. Fan C, Wang Q, van der Zon G, Ren J, Agaser C, Slieker RC, et al. OVOL1 inhibits breast cancer cell invasion by enhancing the degradation of TGF-beta type I receptor. Signal Transduct Target Ther. (2022) 7:126. doi: 10.1038/s41392-022-00944-w
64. Chen L, Kong G, Zhang C, Dong H, Yang C, Song G, et al. MicroRNA-432 functions as a tumor suppressor gene through targeting E2F3 and AXL in lung adenocarcinoma. Oncotarget. (2016) 7:20041–53. doi: 10.18632/oncotarget.7884
Keywords: sheep, mammary gland, lactation, circRNA, miRNA, machine learning, ceRNA
Citation: Chen W, Gu X, Lv X, Cao X, Yuan Z, Wang S and Sun W (2022) Non-coding transcriptomic profiles in the sheep mammary gland during different lactation periods. Front. Vet. Sci. 9:983562. doi: 10.3389/fvets.2022.983562
Received: 01 July 2022; Accepted: 14 September 2022;
Published: 08 November 2022.
Edited by:
Meng-Hua Li, Institute of Zoology (CAS), ChinaReviewed by:
Rui Su, Inner Mongolia Agricultural University, ChinaGaoliang Bao, Gansu Agricultural University, China
Xiaolong Kang, Ningxia University, China
Copyright © 2022 Chen, Gu, Lv, Cao, Yuan, Wang and Sun. This is an open-access article distributed under the terms of the Creative Commons Attribution License (CC BY). The use, distribution or reproduction in other forums is permitted, provided the original author(s) and the copyright owner(s) are credited and that the original publication in this journal is cited, in accordance with accepted academic practice. No use, distribution or reproduction is permitted which does not comply with these terms.
*Correspondence: Wei Sun, ZGt4bXN1bndlaSYjeDAwMDQwOzE2My5jb20=