- 1Heilongjiang Animal Husbandry Service, Harbin, China
- 2College of Animal Science and Technology, Northeast Agricultural University, Harbin, China
- 3Bioinformatics Center, Northeast Agricultural University, Harbin, China
- 4School of mathematics, University of Edinburgh, Edinburgh, United Kingdom
- 5College of Animal Science and Technology, China Agricultural University, Beijing, China
Milk production and body conformation traits are critical economic traits for dairy cows. To understand the basic genetic structure for those traits, a genome wide association study was performed on milk yield, milk fat yield, milk fat percentage, milk protein yield, milk protein percentage, somatic cell score, body form composite index, daily capacity composite index, feed, and leg conformation traits, based on the Illumina Bovine HD100k BeadChip. A total of 57, 12 and 26 SNPs were found to be related to the milk production, somatic cell score and body conformation traits in the Holstein cattle. Genes with pleiotropic effect were also found in this study. Seven significant SNPs were associated with multi-traits and were located on the PLEC, PLEKHA5, TONSL, PTGER4, and LCORL genes. In addition, some important candidate genes, like GPAT3, CEBPB, AGO2, SLC37A1, and FNDC3B, were found to participate in fat metabolism or mammary gland development. These results can be used as candidate genes for milk production, somatic cell score, and body conformation traits of Holstein cows, and are helpful for further gene function analysis to improve milk production and quality.
Introduction
Milk is a source of nutrients essential for human growth and development. The milk production traits are important for the dairy industry. Body conformation traits have been applied in several countries with the development of dairy cattle breeding since they are closely related to the health (1), productivity (2), lifetime (3), and calving ease (4) of cows. Some studies have identified the genetic correlation between body conformation traits and first lactation milk yield to be between 0.48 and 0.54 (5). These correlations are therefore very important for the dairy industry to improve the milk production traits and body conformation traits.
The rapid development of sequencing technology has revealed the cause variants of complex traits using genome-wide association analysis (GWAS). A study by Schennink et al. (6) has revealed DGAT1 and SCD1 to be highly associated with the composition of milk-fat (long-chain fatty acid). Kiser et al. (7) verified the TFAP2A gene to be related to the production of colostrum in Jersey cattle. It reported the genes CDH2 and GABRG2 to be related to the milk fat percentage and milk protein traits, respectively, in dual-purpose Xinjiang brown cattle (8). Bouwman et al. (9) and Vanvanhossou et al. (10) have reported the VEPH1 gene to be associated with conformation. However, the identified genes have not explained all genetic variances. There is a need to continue the search for novel genes related to some quantitative traits.
This study conducted GWAS using the Illumina Bovine HD100k(100k) BeadChip, for identifying important candidate genes or variants related to milk production, somatic cell score, and body conformation traits. There was an expectation for discovering novel genetic variations or candidate genes.
Materials and methods
Animal population
This experiment involved 1,313 cows from 7 different pastures in Heilongjiang Province. The use and care of the animals in this study were approved by the Animal Care Advisory Committee, Northeast Agricultural University (Harbin, China), and all the experimental procedures were according to the university's guidelines for animal research.
Genotypes data
The samples were collected from the tail roots near the hips of the cows. The DNA in the hair was extracted and genotyped using Illumina Bovine HD100k BeadChip, containing 95,256 SNPs. The markers with minor allele frequencies <0.05 and call rates <0.90 were filtered out and individuals with a call rate of 0.80 or greater were selected. These SNPs were distributed across 29 chromosomes.
Population stratification
The SNP genotypes of these individuals were used to estimate the population stratification based on principal component analysis (PCA), and Plink (version 1.9) (11) was used to analyze a total of 1,310 cows with 86,645 markers covering the whole genome to study the population structure (12). The software uses the default matrix construction method to construct G matrix and get the PCA results. We used R language (version 4.1.2)—ggplot 2 package to draw pictures. The PCA scatterplots (Figure 1) illustrate a clear population structure for the 1,310 individuals in the seven pastures cattle herds that comprised our study population.
Genome-wide association analysis
Combination with dairy herd improvement data of National Holstein cows in China, this study estimated the genomic estimated breeding values (GEBVs) of all animal milk production traits, somatic cell score, and body conformation traits, using single-step genomic best linear unbiased prediction (ssGBLUP). The ssGBLUP was developed to integrate all the information including genotypes, phenotypes, and pedigree information in one step, and each SNP effect was calculated using the FarmCPU method (13) based on the predicted GEBVs. The ssGBLUP method is an improvement of BLUP, in which the pedigree relationship matrix a−1 matrix must be replaced by H−1 (14). The specific model is as follows:
Where y was each phenotypic value vector; b is the fixed effect of the field and the PCA effect to explain the population stratification, and u is a vector of animal effects. The e was a vector of random residual effects with e~N(0,I), and X, Z were incidence matrices for b and u, respectively.
Where, the A matrix is pedigree relationship matrix, A22 is a numerator relationship matrix for genotyped animals, and G is a genomic relationship matrix (15). G−1 was obtained as the inverse of a combination of the G matrix and the corresponding A matrix. The w is the weight of A22 in the matrix, the default value is 0.05. The τ and ω are 1. We use DMU software to calculate the GEBV value. Both G and H matrices were derived using software default parameter setting by DMU software. G was calulated as:
Where pi is the allele frequency at locus i in all genotyped animals, is a normalizing constant (16) that sums expected variances across markers scaling G toward the A matrix (17), D is weight for each locus(I if same variance assumed), W is a design matrix as follows):
Each SNP effect was calculated using the FarmCPU method (13) based on the predicted GEBVs. The FarmCPU method (13) in this study can be written as two models.
The y is the GEBV value. The pseudoQTN is significant marker from previous loops that is null when the model begins. SNPi is testing marker in each loop. The K is the kinship between each individuals. The e is residual vector.
For each trait, the threshold P-value for genome-wide significance was 5.99 × 10−7= 0.05/83446 using the Bonferroni multiple test method.
QTLs annotation analysis
The cattle QTL data were downloaded from the Cattle QTL database (https://www.animalgenome.org/cgi-bin/QTLdb/BT/index) referred to as the ARS-UCD1.2 assembly. The square of the correlation coefficient (r2) between the two loci is used to evaluate the range of LD measurement, because r2 is considered to be more robust and not affected by changes in allele frequency and population size (18). Haploview software was used to calculate the genotype correlation coefficient (r2) between all SNP pairs in the cow population to estimate the LD of the whole genome, and the LD decay map with distance of the cow population was visualized.
Results
Population stratification
The phenomenon of group stratification is an important research problem in the study of group association (19). In order to determine the population stratification level, we drew the population structure by principal component analysis (PCA). The PCA scatterplots shows the population structure of a 1,300 individual composed of seven pastures (Figure 1). Different colors represent different pastures. It can be seen that it is mainly divided into three clusters, but most of the cows in the seven pastures are gathered together, and only a few cows are separated. These clusters indicate that, although individuals may come from different ranches, they still retain close genetic relationships.
The genome-wide association study
Basic descriptive statistics of milk production traits, somatic cell score and body conformation traits (see Table 1). A total of 86,645 SNPs were retained after quality control for the GWAS (Table 2). The average physical distance between the adjacent SNP markers was approximately 29.58 kb, ranging between 26.37 kb (BTA19) and 32.02 kb (BTA8).
The p-value profiles of all the SNP markers associated with each trait are represented in Figures 3, 4 and included the Manhattan and Quantile-Quantile plots. In total, 95 genome-wide significant SNPs were detected for the milk production traits, such as milk yield (MY), milk fat yield (FY), milk fat percentage (FP), milk protein yield (PY), milk protein percentage (PP), somatic cell score (SCS), and body conformation traits (body form composite index, BFCI; daily capacity composite index, DCCI; feed and leg conformation, FTLEG). There were 57, 12 and 26 SNPs related to milk production, somatic cell score and body conformation traits, respectively. Among them, we mainly focused on the first few significant SNPs in each trait. In addition, we also found seven SNPs that overlap with multiple traits, such as PLEC is related to MY, FP and PP, PLEKHA5 is related to FP and FY, TONSL is connected with FY and SCS, LCORL is correlated with DCCI and FTLEG, PYGB is related to BFCI and FTLEG, and PTGER4 is related to BFCI, and DCCI (see Table 3).
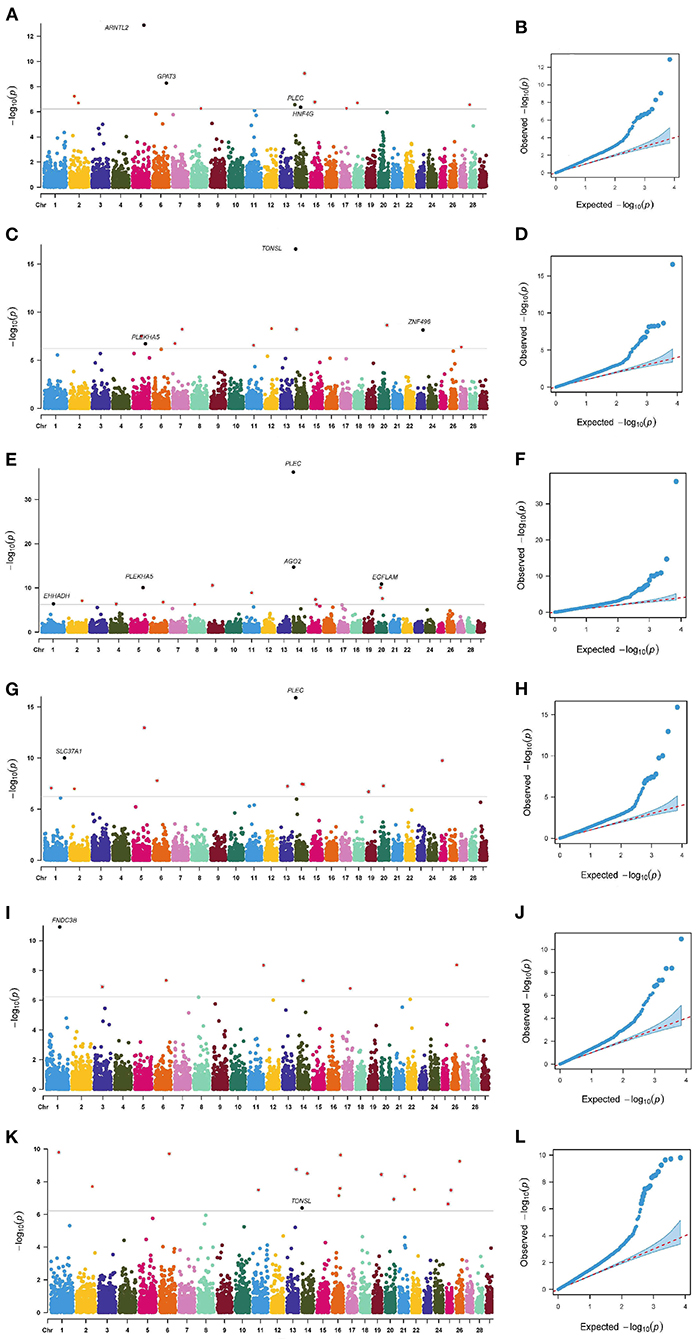
Figure 3. Manhattan plots and Quantile-Quantile plots for the milk production and somatic cell score traits. MY (A,B), FY (C,D), FP (E,F), PP (G,H), PY (I,J) and SCS (K,L).
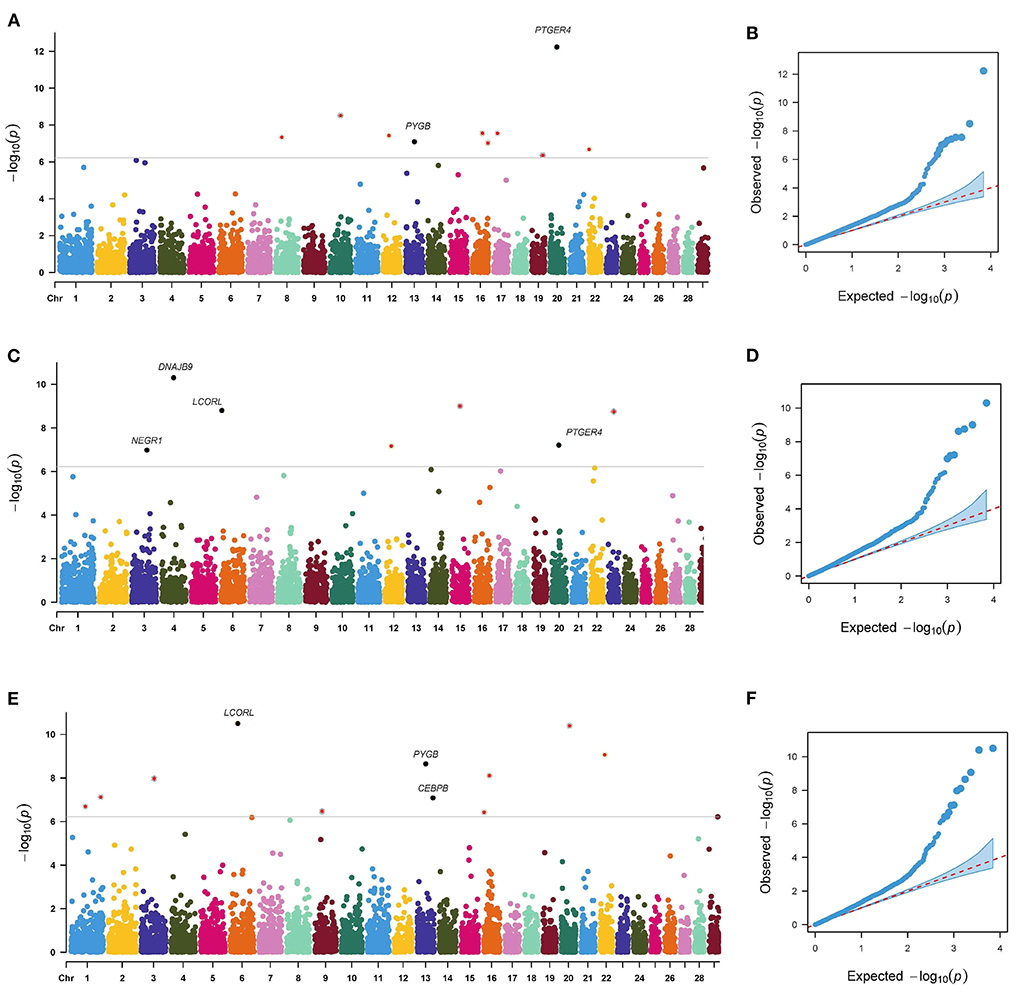
Figure 4. Manhattan plots and Quantile-Quantile plots for the body conformation traits. BFCI (A,B), DCCI (C,D) and FTLEG (E,F).
As shown in Tables 4, 5, 12, 11, 15, 11, 12, and 17 genome-wide significant SNPs were detected for MY, FY, FP, PY, PP, and SCS, respectively. These significant SNPs are mainly distributed in BTA 1, BTA 2, BTA 5, BTA 6, BTA 11, BTA 14, and BTA 20, with as many as 9 SNPs on BTA 14.
In addition, this study reported an interesting phenomenon where four SNPs were found to be related to multi-traits, including BovineHD0500025853 (BTA 5:90.66 Mb), BovineHD1400000206 (BTA 14:0.49 Mb), BovineHD1400000287 (BTA 14:0.88 Mb), and BovineHD1400011649 (BTA 14:38.57 Mb) (see Table 6). The bovinehd1400000287 SNP located in the 58th intron of the PLEC gene was found to be associated with MY, FP, and PP. The fat yield and the somatic cell score trait shared one SNP bovinehd1400000206 located 1.46 kb away from TONSL on BTA 14.
This study detected 10, 7, and 11 significant SNPs related to BFCI, DCCI, and FTLEG, respectively. There were 4 SNPs distributed on BTA 16. Three SNPs were found to be possibly as pleiotropism SNPs, including BovineHD4100004660 (BTA 6:38.22 Mb), BovineHD1300012605 (BTA 13:42.81 Mb) and BTA-50244-no-rs (BTA 20:34.30 Mb), respectively. Of these significant SNPs, the BTA-50244-no-rs SNP related to BFCI (P = 5.84E-13) was located downstream of the PTGER4 gene.
QTL annotation analysis
The LD of cows decreases with the increase of distance, when the distance is extended to 200 Kb, the decline rate of LD of cows tends to be gentle, and the average r2 value of cows is 0.3 at this time (show as Figure 2). The 100 Kb range of SNP upstream and downstream of significant trait association obtained from genome-wide association analysis is compared with the data that has been verified in the current cattle QTL database. Our significant SNPs associated with MY, FY, FP, PP and SCS overlapped with 1332, 1177, 3042, 1288, 24 QTLs, respectively. But there are also very few QTLs about body conformation traits overlapped with significant SNPs.
Discussion
Comparison with the other GWAS studies
In this study, FarmCPU was applied for screening the QTLs related to the milk production traits, health traits, and body conformation traits. A total of 95 significant SNPs were detected, located on the 93 candidate genes. Of these genes, EHHADH, SLC37A1, PLEKHA5, TONSL, PLEC, and IL5RA were reportedly related to milk production traits in other studies (15, 20, 21, 24, 39). However, this study did not detect some important candidate genes, such as DGAT1. Because in this study, the closest SNP on both flanks of DGAT1 are BovineHD1400000206 (109.2 kb) and ARS-BFGL-NGS-55227 (50.8 Mb), respectively. Of these, BovineHD1400000206 associated with fat yield (P value = 2.76E−17). But the nearest gene on this significantly SNP is the TONSL gene (1.65 kb), which is a neighboring gene to DGAT1. So, the DGAT1 gene was not detected in this study. The study by Ning et al. (40) used two models and a 70k SNP chip based on the Chinese Holsteins population and identified the DGAT1 gene to be related to milk (40). Kim et al. (41) also obtained DGAT1 affecting MY and FY in the Korean cattle population (41). Cole et al. (42) identified the PHKA2 gene to be highly significant for four body size traits (stature, strength, body depth, rump width) (42). The 770k BeadChip was used by An et al. (43) to identify five candidate genes (CSMD3, LAP3, SYN3, FAM19A5, and TIMP3) related to the body conformation traits. This study did not detect the above genes to be associated with body conformation traits.
These inconsistencies might be due to differences in the detection platforms or algorithms used in the corresponding analysis, changes in the genetic background of the analyzed cattle, differences in the size and structure of the study population, or random or technical errors in some analyses. This also indicated that there are many important genetic markers or candidate genes in the bovine genome that are yet to be discovered.
Genetic analysis of pleiotropic genes
Organisms have hundreds of thousands of genes and tens of thousands of phenotypes. The relationship between genes and epigenetic factors is complex. There are various associations such as pleiotropism, multigenic effect, polygene effect and so on. Pleiotropy is defined as the phenomenon where a single locus affects two or more distinct phenotypic traits (44, 45). It is common in nature. For example, the DGAT1 gene is related to milk yield (40) and fat yield (26, 41). The genes PIK3R6 and PIK3R1 showed direct functional associations with height and body size (10). Production and health constitute fundamental dairy functions while body conformation traits are related to the functionality of the cow's body. So, the milk production traits and body conformation traits of dairy cows tend to complement each other. Certain identified regions related to conformation traits overlap with the performance traits such as reproduction (46), and milk production (47). Some genes in these regions were also involved in regulating the cell cycle or cell division, homeostasis, and lipid metabolism (10).
This study also reported this interesting phenomenon where the PLEC, PLEKHA5, and TONSL genes were found to belong to the pleiotropism gene for milk traits, and the LCORL, and PTGER4 were pleiotropic genes for the body conformation traits. The PLEC gene (Plectin) can interlink different elements of the cytoskeleton. The PLEC gene was found to be associated with multiple traits, like MY, FP, and PP. Dan Wang et al. (20) also detected PLEC to have potential effects on the MY, FP, and PP traits, which could be useful for molecular breeding for milk production in Chinese Holsteins. The PLEKHA5 gene, located on BTA 5, was predicted to enable the activity of binding phosphatidylinositol phosphate (48). Jiang et al. (21) showed the PLEKHA5 gene to be significantly associated with FP using two different methods using 294,079 Holstein cows. The TONSL protein was considered to be an NF-κ negative regulator of B mediated transcription. Peters et al. (24), Nayeri et al. (25), and Atashi et al. (49) found this gene to be related to milk yield and the TONSL gene was found to reportedly affect the gamma-linolenic acid, long-chain saturated fatty acids and milk fat percent of the Canadian Holstein cows (26). Interesting, the TONSL gene is a neighboring gene to DGAT1 (flanking <200 kb), associated with the fat percentage of milk (26).
Some studies on the LCORL gene showed it to affect human height (27), pig body length (28), horse height (29), chicken carcass weight (30), and the growth and development of cattle (31). This gene might have been a novel loci associated with the human skeletal frame size (32). PTGER4 encodes a protein that is one of the members of the G-protein coupled receptor family, which imparts relaxation to the smooth muscle (34), leading to the phosphorylation of glycogen synthase kinase-3 (35), involved in osteoporosis (36), and regulating lipid droplet size and mitochondrial activity in the white adipose tissue (37, 38).
Important candidate genes related to the fat metabolism or mammary gland development
Fatty acids are essential components of milk with known positive associations with human cardiovascular diseases and so on. This study identified genes such as GPAT3, ARNTL2, EHHADH, CEBPB, DNAJB9, ZNF496, AGO2, GALNT18, and NEGR1 as critical for obesity traits or adipose metabolism (see Table 7).
GPAT3 is highly expressed in the adipose tissue with an important role in adipogenesis (50). This gene can be regulated by folic acid for controlling lactation and metabolic function of the dairy cows (51) and is also involved in fat and lipid metabolism in the Yunling cattle (52). EHHADH involved in fatty acid oxidation is essential for producing medium-chain dicarboxylic acids (54). Hence, this gene has a key impact on the characteristics of milk fatty acid traits in Chinese Holstein (55). In porcine adipogenesis, EHHADH has been proposed to be a pivotal gene in the fat-related pathway (56). The DNAJB9 gene is reportedly one of the prognostic biomarkers of breast cancer (58). Interestingly, DNAJB9 and DNAJB6 are members of the DNAJ gene family, with sequence similarity. The expression level of DNAJB6 in the chicken abdominal adipose tissue was significantly negatively correlated with the abdominal fat weight (59). ZNF496 is reportedly associated with milk concentration (milk fat) and fertility (60). According to Gao et al. (62), the GALNT18 gene was associated with milk protein and fat traits.
According to the known gene functions, some candidate genes were expressed in the mammary gland, such as the SLC37A1, and FNDC3B genes (see Table 7). SLC37A1, over-expressed in the bovine mammary tissue relative to the 17 other tissue types (66) transports glucose-6-phosphate in one direction and phosphorus in the other (68). Glucose is known to be essential for lactose synthesis in mammary cells. Kemper et al. (66) identified the causative mutation increasing the expression of SLC37A1 leading to an increase in milk yield and decreasing the phosphorus concentration.
QTLs result overlapped with GWAS
Although many quantitative trait loci (QTLs) related to economically important traits in dairy cows have been identified, due to insufficient sample size and insufficient marker density used in QTL mapping research in history, not all genetic variations of these traits have been captured (69), in the study, we used GWAS to analyze the milk production traits, body conformation traits and somatic cells of dairy cows, and most of the results were also verified in the QTL analysis of dairy cows. Interestingly, our study found many SNP related to pleiotropy, but no repeated QTL regions were found in the QTL analysis (70). Also found the same phenomenon in the study of multiple traits of beef cattle. With these results, we can get some inspiration in verifying QTLs of some characteristics of interest shared among varieties (71).
Conclusions
A total of 95 significant SNPs were identified to be related to the milk production, somatic cell score, and body conformation traits in Holstein cattle. Among them, 7 significant SNPs located on the PLEC, PLEKHA5, TONSL, PTGER4, and LCORL genes showed pleiotropic effects on milk production or body conformation traits. In addition, some important candidate genes, including GPAT3, CEBPB, AGO2, SLC37A1, and FNDC3B, were also found to be related to the fat metabolism or involved in mammary gland development. The above genes however need to be consolidated as new potential genes through future validation.
Data availability statement
The original contributions presented in the study are included in the article or supplementary material, the variation data reported in this article have been deposited in the Genome Variation Map (GVM) in Big Data Center, Beijing Institute of Genomics (BIG), and Chinese Academy of Sciences, under accession numbers GVM000388 that are publicly accessible at https://bigd.big.ac.cn/gvm/getProjectDetail?project=GVM000388. The Bioproject accession number is PRJCA011726. Further inquiries can be directed to the corresponding author.
Ethics statement
The animal study was reviewed and approved by Animal Care Advisory Committee, Northeast Agricultural University (Harbin, China).
Author contributions
ZW, PW, and XL conceived the study and participated in its design. YZ, JW, QK, and XN were involved in the acquisition of data. XL, JW and QZ performed all data analysis. XL and ZW drafted the manuscript. ZW, PW, XL, YZ, CZ, QK, and XN contributed to the writing and editing. All authors read and approved the final manuscript.
Funding
This work was financially supported by the Natural Science Foundation of China (No. 32070571), the Academic Backbone Project of Northeast Agricultural University (No. 15XG14), and the NEAU Research Founding for Excellent Young Teachers (2010RCB29).
Acknowledgments
The authors are grateful to all the members of the Dairy Breeding Group of the Heilongjiang Provincial Husbandry Department for collecting the data. We appreciate the support by the Project of Science and Education Office in the Department of Agriculture and Rural Affairs of Heilongjiang Province (Application of the genomic breeding technology for modernized large-scale dairy farm in Heilongjiang Province).
Conflict of interest
The authors declare that the research was conducted in the absence of any commercial or financial relationships that could be construed as a potential conflict of interest.
Publisher's note
All claims expressed in this article are solely those of the authors and do not necessarily represent those of their affiliated organizations, or those of the publisher, the editors and the reviewers. Any product that may be evaluated in this article, or claim that may be made by its manufacturer, is not guaranteed or endorsed by the publisher.
References
1. Chapinal N, Koeck A, Sewalem A, Kelton DF, Mason S, Cramer G, et al. Genetic parameters for hoof lesions and their relationship with feet and leg traits in Canadian Holstein cows. J Dairy Sci. (2013) 96:2596–04. doi: 10.3168/jds.2012-6071
2. Lund T, Miglior F, Dekkers JCM, Burnside EB. Genetic-relationships between clinical mastitis, somatic-cell count, and udder conformation in Danish Holsteins. Livest Prod Sci. (1994) 39:243–51. doi: 10.1016/0301-6226(94)90203-8
3. Vollema AR, Van Der Beek S, Harbers AG, De Jong G. Genetic evaluation for longevity of Dutch dairy bulls. J Dairy Sci. (2000):2629–39. doi: 10.3168/jds.S0022-0302(00)75156-3
4. Kadarmideen HN, Wegmann S. Genetic parameters for body condition score and its relationship with type and production traits in Swiss Holsteins. J Dairy Sci. (2003) 86:3685–93. doi: 10.3168/jds.S0022-0302(03)73974-5
5. Short TH, Lawlor TJ. Genetic parameters of conformation traits, milk yield, and herd life in Holsteins. J Dairy Sci. (1992) 75:1987–8. doi: 10.3168/jds.S0022-0302(92)77958-2
6. Schennink A, Stoop WM, Visker MH, van der Poel JJ, Bovenhuis H, van Arendonk JA. Short communication: genome-wide scan for bovine milk-fat composition. II Quantitative trait loci for long-chain fatty acids. J Dairy Sci. (2009) 92:4676–82. doi: 10.3168/jds.2008-1965
7. Kiser JN, Cornmesser MA, Gavin K, Hoffman A, Moore DA, Neibergs HL. Rapid communication: genome-wide association analyses identify loci associated with colostrum production in Jersey cattle1. J Anim Sci. (2019) 97:1117–23. doi: 10.1093/jas/sky482
8. Zhou J, Liu L, Chen CJ, Zhang M, Lu X, Zhang Z, et al. Genome-wide association study of milk and reproductive traits in dual-purpose Xinjiang Brown cattle. BMC Genomics. (2019) 20:827. doi: 10.1186/s12864-019-6224-x
9. Bouwman AC, Daetwyler HD, Chamberlain AJ, Ponce CH, Sargolzaei M, Schenkel FS, et al. Meta-analysis of genome-wide association studies for cattle stature identifies common genes that regulate body size in mammals. Nat Genet. (2018) 50:362–7. doi: 10.1038/s41588-018-0056-5
10. Vanvanhossou SFU, Scheper C, Dossa LH, Yin T, Brügemann K, König S, et al. Multi-breed GWAS for morphometric traits in four Beninese indigenous cattle breeds reveals loci associated with conformation, carcass and adaptive traits. BMC Genomics. (2020) 21:783. doi: 10.1186/s12864-020-07170-0
11. Purcell S, Neale B, Todd-Brown K, Thomas L, Ferreira MA, Bender D, et al. PLINK: a tool set for whole-genome association and population-based linkage analyses. Am J Hum Genet. (2007) 81:559–75. doi: 10.1086/519795
12. Reich D, Price AL, Patterson N. Principal component analysis of genetic data. Nat Genet. (2008) 40:491–92.
13. Liu X, Huang M, Fan B, Buckler ES, Zhang Z. Iterative usage of fixed and random effect models for powerful and efficient genome-wide association studies. PLoS Genet. (2016) 12:e1005767. doi: 10.1371/journal.pgen.1005767
14. Aguilar I, Misztal I, Johnson DL, Legarra A, Tsuruta S. Lawlor TJ. Hot topic: a unified approach to utilize phenotypic, full pedigree, and genomic information for genetic evaluation of Holstein final score. (2010) 93:743–52. doi: 10.3168/jds.2009-2730
15. Iung LHS, Petrini J, Ramírez-Díaz J, Salvian M, Rovadoscki GA, Pilonetto F„ et al. Genome-wide association study for milk production traits in a Brazilian Holstein population. J Dairy Sci. (2019) 102:5305–14. doi: 10.3168/jds.2018-14811
16. Weng Z, Zhang Z, Ding X, Fu W, Ma P, Wang C, et al. Application of imputation methods to genomic selection in Chinese Holstein cattle. J Anim Sci Biotechnol. (2012) 3:6. doi: 10.1186/2049-1891-3-6
17. VanRaden PM. Efficient methods to compute genomic predictions. J Dairy Sci. (2008) 91:4414–23. doi: 10.3168/jds.2007-0980
18. Zhao H, Nettleton D, Soller M, Dekkers JC. Evaluation of linkage disequilibrium measures between multi-allelic markers as predictors of linkage disequilibrium between markers and QTL. Genet Res. (2005) 86:77–87. doi: 10.1017/S001667230500769X
19. Wu C, DeWan A, Hoh J, Wang Z. A comparison of association methods correcting for population stratification in case-control studies. Ann Hum Genet. (2011)75:418–27. doi: 10.1111/j.1469-1809.2010.00639.x
20. Wang D, Ning C, Liu JF, Zhang Q, Jiang L. Short communication: replication of genome-wide association studies for milk production traits in Chinese Holstein by an efficient rotated linear mixed model. J Dairy Sci. (2019) 102:2378–83. doi: 10.3168/jds.2018-15298
21. VanRaden PM, Cole JB, Da Y. A large-scale genome-wide association study in US Holstein Cattle. Front Genet. (2019) 10:412. doi: 10.3389/fgene.2019.00412
22. Selvanayagam T, Walker S, Gazzellone MJ, Kellam B, Cytrynbaum C, Stavropoulos DJ, et al. Genome-wide copy number variation analysis identifies novel candidate loci associated with pediatric obesity. Eur J Hum Genet. (2018) 26:1588–96. doi: 10.1038/s41431-018-0189-0
23. Ramayo-Caldas Y, Fortes MR, Hudson NJ, Porto-Neto LR, Bolormaa S, Barendse W, et al. A marker-derived gene network reveals the regulatory role of PPARGC1A, HNF4G, and FOXP3 in intramuscular fat deposition of beef cattle. J Anim Sci. (2014) 92:2832–45. doi: 10.2527/jas.2013-7484
24. Peters SO, Kizilkaya K, Ibeagha-Awemu EM, Sinecen M, Zhao X. Comparative accuracies of genetic values predicted for economically important milk traits, genome-wide association, and linkage disequilibrium patterns of Canadian Holstein cows. J Dairy Sci. (2021) 104:1900–16. doi: 10.3168/jds.2020-18489
25. Nayeri S, Sargolzaei M, Abo-Ismail MK, May N, Miller SP, Schenkel F, et al. Genome-wide association for milk production and female fertility traits in Canadian dairy Holstein cattle. BMC Genet. (2016) 17:75. doi: 10.1186/s12863-016-0386-1
26. Ibeagha-Awemu EM, Peters SO, Akwanji KA, Imumorin IG, Zhao X. High density genome wide genotyping-by-sequencing and association identifies common and low frequency SNPs, and novel candidate genes influencing cow milk traits. Sci Rep. (2016) 6:31109. doi: 10.1038/srep31109
27. Sovio U, Bennett AJ, Millwood IY, Molitor J, O'Reilly PF, Timpson NJ, et al. Genetic determinants of height growth assessed longitudinally from infancy to adulthood in the northern Finland birth cohort 1966. PLoS Genet. (2009) 5:e1000409. doi: 10.1371/journal.pgen.1000409
28. Rubin CJ, Megens HJ, Martinez Barrio A, Maqbool K, Sayyab S, Schwochow D, et al. Strong signatures of selection in the domestic pig genome. Proc Natl Acad Sci U S A. (2012) 109:19529–36. doi: 10.1073/pnas.1217149109
29. Signer-Hasler H, Flury C, Haase B, Burger D, Simianer H, Leeb T, et al. A genome-wide association study reveals loci influencing height and other conformation traits in horses. PLoS ONE. (2012) 7:e37282. doi: 10.1371/journal.pone.0037282
30. Liu R, Sun Y, Zhao G, Wang F, Wu D, Zheng M, et al. Genome-wide association study identifies Loci and candidate genes for body composition and meat quality traits in Beijing-You chickens. PLoS ONE. (2013) 8:e61172. doi: 10.1371/journal.pone.0061172
31. Weikard R, Altmaier E, Suhre K, Weinberger KM, Hammon HM, Albrecht E, et al. Metabolomic profiles indicate distinct physiological pathways affected by two loci with major divergent effect on Bos taurus growth and lipid deposition. Physiol Genomics. (2010) 42A:79–88. doi: 10.1152/physiolgenomics.00120.2010
32. Soranzo N, Rivadeneira F, Chinappen-Horsley U, Malkina I, Richards JB, Hammond N, et al. Meta-analysis of genome-wide scans for human adult stature identifies novel Loci and associations with measures of skeletal frame size. PLoS Genet. (2009) 5:e1000445. doi: 10.1371/journal.pgen.1000445
33. Altemus MA, Goo LE, Little AC, Yates JA, Cheriyan HG, Wu ZF, et al. Breast cancers utilize hypoxic glycogen stores via PYGB, the brain isoform of glycogen phosphorylase, to promote metastatic phenotypes. PLoS ONE. (2019) 14:e0220973. doi: 10.1371/journal.pone.0220973
34. Tilley SL, Hartney JM, Erikson CJ, Jania C, Nguyen M, Stock J, et al. Receptors and pathways mediating the effects of prostaglandin E2 on airway tone. Am J Physiol Lung Cell Mol Physiol. (2003) 284:L599–606.
35. Fujino H, West KA, Regan JW. Phosphorylation of glycogen synthase kinase-3 and stimulation of T-cell factor signaling following activation of EP2 and EP4 prostanoid receptors by prostaglandin E2. J Biol Chem. (2002) 277:2614–9. doi: 10.1074/jbc.M109440200
36. Li M, Healy DR, Li Y, Simmons HA, Crawford DT, Ke HZ, et al. Osteopenia and impaired fracture healing in aged EP4 receptor knockout mice. Bone. (2005) 37:46–54. doi: 10.1016/j.bone.2005.03.016
37. Ying F, Cai Y, Cai Y, Wang Y, Tang EHC. Prostaglandin E receptor subtype 4 regulates lipid droplet size and mitochondrial activity in murine subcutaneous white adipose tissue. FASEB J. (2017) 31:4023–36. doi: 10.1096/fj.201700191R
38. Lv X, Gao F, Li TP, Xue P, Wang X, Wan M, et al. Skeleton interoception regulates bone and fat metabolism through hypothalamic neuroendocrine NPY. Elife. (2021) 10:e70324. doi: 10.7554/eLife.70324
39. Zhou C, Li C, Cai W, Liu S, Yin H, Shi S, et al. Genome-wide association study for milk protein composition traits in a Chinese Holstein Population using a single-step approach. Front Genet. (2019) 10:72. doi: 10.3389/fgene.2019.00072
40. Ning C, Wang D, Zheng X, Zhang Q, Zhang S, Mrode R, et al. Eigen decomposition expedites longitudinal genome-wide association studies for milk production traits in Chinese Holstein. Genet Sel Evol. (2018) 50:12. doi: 10.1186/s12711-018-0383-0
41. Kim S, Lim B, Cho J, Lee S, Dang CG, Jeon JH, et al. Genome-Wide identification of candidate genes for milk production traits in Korean Holstein Cattle. Animals (Basel). (2021) 11:1392. doi: 10.3390/ani11051392
42. Cole JB, Wiggans GR, Ma L, Sonstegard TS, Lawlor TJ Jr, Crooker BA, et al. Genome-wide association analysis of thirty one production, health, reproduction and body conformation traits in contemporary US Holstein cows. BMC Genomics. (2011) 12:408.
43. An B, Xia J, Chang T, Wang X, Xu L, Zhang L, et al. Genome-wide association study reveals candidate genes associated with body measurement traits in Chinese Wagyu beef cattle. Anim Genet. (2019) 50:386–90. doi: 10.1111/age.12805
44. Carter AJ, Nguyen AQ. Antagonistic pleiotropy as a widespread mechanism for the maintenance of polymorphic disease alleles. BMC Med Genet. (2011) 12:160. doi: 10.1186/1471-2350-12-160
45. Stearns FW. One hundred years of pleiotropy: a retrospective. Genetics. (2010) 186:767–73. doi: 10.1534/genetics.110.122549
46. Dzidic A, Kuehnl J, Simic M, Bruckmaier RM. Effects of short and long milking intervals on milking characteristics and changes of milk constituents during the course of milking in crossbred Istrian × Awassi × East-Friesian ewes. J Dairy Res. (2022) 16:1–6. doi: 10.1017/S0022029922000036
47. Oberbauer AM, Berry SL, Belanger JM, McGoldrick RM, Pinos-Rodriquez JM, Famula TR. Determining the heritable component of dairy cattle foot lesions. J Dairy Sci. (2013) 96:605–13. doi: 10.3168/jds.2012-5485
48. Yamada K, Nomura N, Yamano A, Yamada Y, Wakamatsu N. Identification and characterization of splicing variants of PLEKHA5 (Plekha5) during brain development. Gene. (2012) 492:270. doi: 10.1016/j.gene.2011.10.018
49. Atashi H, Salavati M, De Koster J, Ehrlich J, Crowe M, Opsomer G, et al. Genome-wide association for milk production and lactation curve parameters in Holstein dairy cows. J Anim Breed Genet. (2020) 137:292–304.
50. Shan D, Li JL, Wu L, Li D, Hurov J, Tobin JF, et al. GPAT3 and GPAT4 are regulated by insulin-stimulated phosphorylation and play distinct roles in adipogenesis. J Lipid Res. (2010) 1:1971–81. doi: 10.1194/jlr.M006304
51. Khan MZ, Liu L, Zhang Z, Khan A, Wang D, Mi S, et al. Folic acid supplementation regulates milk production variables, metabolic associated genes and pathways in perinatal Holsteins. J Anim Physiol Anim Nutr (Berl). (2020) 104:483–92. doi: 10.1111/jpn.13313
52. Zhang F, Hanif Q, Luo X, Jin X, Zhang J, He Z, et al. Muscle transcriptome analysis reveal candidate genes and pathways related to fat and lipid metabolism in Yunling cattle. Anim Biotechnol. (2021) 7:1–8. doi: 10.1080/10495398.2021.2009846
53. Costa-Urrutia P, Colistro V, Jiménez-Osorio AS, Cárdenas-Hernández H, Solares-Tlapechco J, Ramirez-Alcántara M, et al. Genome-wide association study of body mass index and body fat in Mexican-Mestizo Children. Genes (Basel). (2019) 10:945. doi: 10.3390/genes10110945
54. Houten SM, Denis S, Argmann CA, Jia Y, Ferdinandusse S, Reddy JK, et al. Peroxisomal L-bifunctional enzyme (Ehhadh) is essential for the production of medium-chain dicarboxylic acids. J Lipid Res. (2012) 53:1296–303. doi: 10.1194/jlr.M024463
55. Li C, Sun D, Zhang S, Wang S, Wu X, Zhang Q, et al. Genome wide association study identifies 20 novel promising genes associated with milk fatty acid traits in Chinese Holstein. PLoS ONE. (2014) 9:e96186. doi: 10.1371/journal.pone.0096186
56. Zhu J, Yang Z, Hao W, Li J, Wang L, Xia J, et al. Characterization of a read-through fusion transcript, BCL2L2-PABPN1, involved in porcine Adipogenesis. Genes (Basel). (2022) 13:445. doi: 10.3390/genes13030445
57. Lv H, Meng Q, Wang N, Duan X, Hou X, Lin Y. Cell death-inducing DNA fragmentation factor-α-like effector C (CIDEC) regulates acetate- and β-hydroxybutyrate-induced milk fat synthesis by increasing FASN expression in mammary epithelial cells of dairy cows. J Dairy Sci. (2021) 104:6212–21. doi: 10.3168/jds.2020-18975
58. Ou Yang TH, Cheng WY, Zheng T, Maurer MA, Anastassiou D. Breast cancer prognostic biomarker using attractor metagenes and the FGD3-SUSD3 metagene. Cancer Epidemiol Biomarkers Prev. (2014) 23:2850–56. doi: 10.1158/1055-9965.EPI-14-0399
59. Jin P, Wu X, Xu S, Zhang H, Li Y, Cao Z, et al. Differential expression of six genes and correlation with fatness traits in a unique broiler population. Saudi J Biol Sci. (2017) 24:945–9. doi: 10.1016/j.sjbs.2015.04.014
60. Golik M, Glick G, Reicher S, Shirak A, Ezra E, Zeron Y, et al. Differential expression of ruminant ZNF496 variants: association with quantitative trait locus affecting bovine milk concentration and fertility. J Dairy Sci. (2011) 94:2092–102. doi: 10.3168/jds.2010-3655
61. Bhattacharjee J, Borra VJ, Salem ESB, Zhang C, Murakami K, Gill RK, et al. Hepatic ago2 regulates PPARα for oxidative metabolism linked to glycemic control in obesity and post bariatric surgery. Endocrinology. (2021) 162:bqab007. doi: 10.1210/endocr/bqab007
62. Gao Y, Jiang J, Yang S, Hou Y, Liu GE, Zhang S, et al. CNV discovery for milk composition traits in dairy cattle using whole genome resequencing. BMC Genomics. (2017) 18:265. doi: 10.1186/s12864-017-3636-3
63. Thorleifsson G, Walters GB, Gudbjartsson DF, Steinthorsdottir V, Sulem P, Helgadottir A, et al. Genome-wide association yields new sequence variants at seven loci that associate with measures of obesity. Nat Genet. (2009) 41:18–24. doi: 10.1038/ng.274
64. Speliotes EK, Willer CJ, Berndt SI, Monda KL, Thorleifsson G, Jackson AU, et al. Association analyses of 249,796 individuals reveal 18 new loci associated with body mass index. Nat Genet. (2010) 42:937–48. doi: 10.1038/ng.686
65. Mägi R, Manning S, Yousseif A, Pucci A, Santini F, Karra E, et al. Contribution of 32 GWAS-identified common variants to severe obesity in European adults referred for bariatric surgery. PLoS ONE. (2013) 8:e70735. doi: 10.1371/journal.pone.0070735
66. Kemper KE, Reich CM, Bowman PJ, Vander Jagt CJ, Chamberlain AJ, Mason BA, et al. Improved precision of QTL mapping using a nonlinear Bayesian method in a multi-breed population leads to greater accuracy of across-breed genomic predictions. Genet Sel Evol. (2015) 47:29. doi: 10.1186/s12711-014-0074-4
67. Choudhary RK, Capuco AV. Expression of NR5A2, NUP153, HNF4A, USP15 and FNDC3B is consistent with their use as novel biomarkers for bovine mammary stem/progenitor cells. J Mol Histol. (2021) 52:289–300. doi: 10.1007/s10735-020-09948-8
68. Pan CJ, Chen SY, Jun HS, Lin SR, Mansfield BC, Chou JY. SLC37A1 and SLC37A2 are phosphate-linked, glucose-6-phosphate antiporters. PLoS ONE. (2011) 6:e23157.
69. Saatchi M, Schnabel RD, Taylor JF, Garrick DJ. Large-effect pleiotropic or closely linked QTL segregate within and across ten US cattle breeds. BMC Genomics. (2014) 15:442. doi: 10.1186/1471-2164-15-442
70. Saatchi M, Beever JE, Decker JE, Faulkner DB, Freetly HC, Hansen SL, et al. QTLs associated with dry matter intake, metabolic mid-test weight, growth and feed efficiency have little overlap across 4 beef cattle studies. BMC Genomics. (2014) 15:1004. doi: 10.1186/1471-2164-15-1004
Keywords: milk production traits, body conformation traits, pleiotropic effect, genome-wide association study, Holstein cattle
Citation: Wang P, Li X, Zhu Y, Wei J, Zhang C, Kong Q, Nie X, Zhang Q and Wang Z (2022) Genome-wide association analysis of milk production, somatic cell score, and body conformation traits in Holstein cows. Front. Vet. Sci. 9:932034. doi: 10.3389/fvets.2022.932034
Received: 29 April 2022; Accepted: 09 September 2022;
Published: 04 October 2022.
Edited by:
Xiangdong Ding, China Agricultural University, ChinaReviewed by:
Yao Jiang, China Agricultural University, ChinaYuchun Pan, Shanghai Jiao Tong University, China
Copyright © 2022 Wang, Li, Zhu, Wei, Zhang, Kong, Nie, Zhang and Wang. This is an open-access article distributed under the terms of the Creative Commons Attribution License (CC BY). The use, distribution or reproduction in other forums is permitted, provided the original author(s) and the copyright owner(s) are credited and that the original publication in this journal is cited, in accordance with accepted academic practice. No use, distribution or reproduction is permitted which does not comply with these terms.
*Correspondence: Zhipeng Wang, d2FuZ3poaXBlbmcmI3gwMDA0MDtuZWF1LmVkdS5jbg==
†These authors have contributed equally to this work and share first authorship