- 1College of Animal Science and Technology, Northwest A&F University, Xianyang, China
- 2National Beef Cattle Improvement Center, Northwest A&F University, Xianyang, China
As the main product of livestock, muscle itself plays an irreplaceable role in maintaining animal body movement and regulating metabolism. Therefore, it is of great significance to explore its growth, development and regeneration to improve the meat yield and quality of livestock. In this study, we attempted to use RNA-seq and ATAC-seq techniques to identify differentially expressed genes (DEGs) specifically expressed in bovine skeletal muscle as potential candidates for studying the regulatory mechanisms of muscle development. Microarray data from 8 tissue samples were selected from the GEO database for analysis. First, we obtained gene modules related to each tissue through WGCNA analysis. Through Gene Ontology (GO) functional annotation, the module of lightyellow (MElightyellow) was closely related to muscle development, and 213 hub genes were screened as follow-up research targets. Further, the difference analysis showed that, except for PREB, all other candidate hub genes were up-regulated (muscle group vs. other-group). ATAC-seq analysis showed that muscle-specific accessible chromatin regions were mainly located in promoter of genes related to muscle structure development (GO:0061061), muscle cell development (GO:0055001) and muscle system process (GO:0003012), which were involved in cAMP, CGMP-PKG, MAPK, and other signaling pathways. Next, we integrated the results of RNA-seq and ATAC-seq analysis, and 54 of the 212 candidate hub genes were identified as key regulatory genes in skeletal muscle development. Finally, through motif analysis, 22 of the 54 key genes were found to be potential target genes of transcription factor MEF2C. Including CAPN3, ACTN2, MB, MYOM3, SRL, CKM, ALPK3, MAP3K20, UBE2G1, NEURL2, CAND2, DOT1L, HRC, MAMSTR, FSD2, LRRC2, LSMEM1, SLC29A2, FHL3, KLHL41, ATXN7L2, and PDRG1. This provides a potential reference for studying the molecular mechanism of skeletal muscle development in mammals.
Introduction
The focus of the adjustment of the new agricultural industrial structure is to develop high-quality and efficient stock husbandry. Compared with pig and poultry breeding, cattle breeding can greatly improve the utilization of straw resources and reduce environmental pollution, so it plays an increasingly important role in the development of animal husbandry. As the largest tissue of cattle organisms, the growth, development and genetic characteristics of skeletal muscle affect and even determine the meat production performance of cattle (1). Many factors are closely related to muscle tenderness and affect meat product quality, such as intramuscular fat content, muscle fiber size, and intramuscular protein content and types (2–5). The growth and development of skeletal muscle is an extremely complex biological process, which is co-regulated by a variety of regulatory factors.
Transcription factors (TFs) are critical in muscle growth and development. Members of the myogenic regulatory factor (MRF) family are typical inducers of skeletal muscle development, including the early myogenic regulatory factors (MYOD, MYF5, MYF6, MRF4, et al.) and the late differentiation marker gene (MyoG) (6). Myocyte enhancer Factor-2 (MEF2) family (MEF2a, MEF2b, MEF2c, and MEF2d) is a kind of key transcription factor discovered after MyoD, which controls the expression of myogenic genes (7). The N-terminal of MEF2 contains a highly conserved MADS-Box domain and its adjacent MEF2 domain. The MADS-Box domain mediates protein dimerization, while the MEF2 domain influences its binding affinity with DNA and its interaction with cofactors (7–9). It has been reported that MEF2s was involved in regulating the proliferation and differentiation of myocyte mainly through participating in Ca2+-calmodulin-dependent protein kinases CaMK-histone deacetylases HDACs, Calconneurin, MAPK and other signaling pathways (10, 11). In addition, with the rise of RNA-seq technology, a large number of genes related to muscle development have been discovered successively, but the signal regulatory networks between genes and genes, transcription factors and target genes, and epistasis and genes are still poorly understood. Moreover, we focused more on finding the few regulators that play a dominant role in the complex regulatory network and exploring their relevant regulatory mechanisms in vivo.
As chromatin is the carrier of genes, the activation and silencing of genes will inevitably cause the remodeling of chromatin structure. ATAC-seq is a newly developed technique to study the open regions of genomic chromatin, which helps to elucidate the regulatory mechanisms of genes (12). In recent years, a large number of researchers began to explore the relationship between chromatin open region and gene expression, including domestic animals. A study found a group of highly functionally conserved gene regulatory elements in different tissues by comparing the genomes of three domestic animals (Gallus Galla, Sus Scrofa, Bos Taurus) to human and mouse genomes (13). Furthermore, by comparing chromatin accessibility in muscle, liver and hypothalamus of Bos indicus cattle, learned that MEF2 is the main regulator of muscle-specific open chromatin region (14). Combined with previous studies, we intend to further explore the key regulatory genes involved in skeletal muscle development by comparing the sequencing data of muscle and other tissues, and explore the transcription factors that may be regulated, which will provide some methodological guidance and reference value for the screening and functional research of core genes.
Materials and methods
Data acquisition
The workflow of this study was shown in Figure 1. The data used in this study was from GSE158430 of the GEO database (GEO Accession viewer (nih.gov) (13). GSE158430 is a SuperSeries and has three SubSeries (GSE158412, GSE158414, and GSE158416). GSE158412 dataset contains RNA sequencing data from 8 different tissues (liver, lung, spleen, skeletal muscle, subcutaneous adipose, cerebellum, brain cortex, and hypothalamus) of cattle, pigs, and chickens, with two biological replicates. GSE158414 dataset includes ATAT-seq data from different tissues of cattle and pigs with two biological replicates (except cerebellum tissue in cattle). GSE158416 was CTCF ChIP-seq in 8 different tissues of the above livestock species, which also has two biological replicates. We only downloaded the SRA format data of cattle in GSE158412 and GSE158414 for subsequent analysis (see Supplementary Table 1 for details). The SRA format was then converted to fastq format using sratools' fastq-dump command (15).
Expression matrix pre-processing
To obtain high-quality clean reads, Trimmomatic (v0.36) (16) and FastQC (http://www.bioinformatics.babraham.ac.uk/projects/fastqc/) were used to filter and evaluate the quality of sequencing reads. Clean reads were mapped to cattle (Bos taurus) reference genome from Ensembl Genome Browser 104 (http://asia.ensembl.org/index.html) using hisat2 (v2.1.0) (17). SAMtools (v1.7) was used to convert the sam files obtained from mapping to bam files (18). Two important values representing gene expression were tallied: reads count was quantified with featureCounts (v1.6.0) (19), The FPKM (fragments per kilobase of transcript per million fragments mapped) were calculated using StringTie (v2.1.2) (20). Further, the genes were annotated through Ensembl Genome Browser 104 database, and protein-coding genes (PCGs) were screened for WGCNA and Differently Expression analysis (21).
Weighted gene coexpression network analysis
The top 75% of PCGs with the highest median absolute deviation (MAD) were constructed the co-expression network with the value of log2(FPKM+1) using WGCNA package (v1.70) from Bioconductor in RStudio (v1.2) (http://www.rstudio.org), an integrated development environment for R (v4.0.2). Then, the weighted adjacency matrix of 16 tissue samples was constructed by the formula of the adjacency matrix: amn = |cor(mn)|β (amn, adjacency between m and n; cor(mn), Pearson's correlation between m and n; and β, soft-power threshold) (22). Subsequently, the adjacency matrix was transformed into a topological overlap matrix (TOM) to quantitatively describe the similarity in nodes by comparing the weighted correlation between two nodes and other nodes. Next, hierarchical clustering was used to identify modules according to the TOM matrix with the minModuleSize 100. Similar modules (>75%) were merged (abline = 0.25).
Identification of sample significant modules and hub genes
Module-sample associations were estimated according to TOM (|cor| > 0.5, P < 0.01). The same module of genes is generally considered to have a higher topological overlap similarity. For each expression profile, gene significance (GS, the correlation between expression profile and each sample) and module membership [MM, the correlation between the module eigengene (ME) and the gene expression profile] were used to identify the Module-sample correlation. Finally, hub genes screened with MM > 0.8 and GS > 0.6 with weighted P-value (P.weighted) < 0.01.
RNA differential expression analysis
The expression matrix of whole genes was used for further analysis of gene expression differences among different samples by DEseq2 in R software (23). False Discovery Rate (FDR) was got by adjusting the P-value. Genes with fold change > 2 and false discovery rate (FDR) < 0.05 were set as the threshold to be DEGs.
ATAC-seq analysis
First, FastQC was used to detect data quality. The clean reads were obtained by removing low-quality fragments using Fastp (v0.19.4) (24). BWA (Burrows-Wheeler Alignment) (v0.7.17) was used to map clean reads to cattle (Bos taurus) reference genome. Genrich (v0.6.1) was used to calling peaks for each sample (https://github.com/jsh58/Genrich). The different peaks were identified using DiffBind (https://bioconductor.org/packages/release/bioc/html/DiffBind.html) (v2.16.2) with the default parameters (25). ChIPseeeker (v1.24.0) (26) was used to annotate the differential peaks according to the Ensembl Genome Browser 104 (http://asia.ensembl.org/index.html) and org.Bt.eg.db (https://bioconductor.org/packages/release/data/annotation/html/org.Bt.eg.db.html). During the genome-wide annotation of peaks, the genome-wide functional regions were divided into promoter, dowstream, exon, intron and distal intergenic regions. The closest gene to each peak can be obtained according to the distance of each binding site to TSS, and their specific distribution of binding sites on the genome can be found out. This study considered the profile of peaks at their ± 3 kb of the transcription start site (TSS) as promoter genes or targets. The motifs were examined using the Multiple EM for Motif Elicitation (MEME) suite (https://meme-suite.org/meme/doc/meme-chip.html?man_type=web). Then, the Motif database scanning algorithm TOMTOM was used to predict transcription factors (TFs) (27). ATACseqQC (v1.12.5) was used for quality detection and motif footprint analysis (28). FIMO was used to scan binding locations of TFs in the promoter regions of DEGs (upstream 2,000 bp and downstream 100 bp) (29).
Gene ontology and kyoto encyclopedia of genes and genomes analysis
To explore the potential biological roles of module genes, Gene Ontology (GO) functional annotation for multiple gene lists was analyzed using clusterProfile (30). And the GO analysis for the Single-gene list was carried out by g:Profiler (https://biit.cs.ut.ee/gprofiler/gost) with the default parameters (31). KEGG pathway analyses were performed using KOBAS (32). We used a significant threshold P-value adjusted by Benjamini and Hochberg of 0.05 for KEGG to control the false discovery rate (FDR) and used Fisher's Exact Test as the statistical test method.
Real-time quantitative PCR
The total RNA from bovine tissues or cells was extracted by Trizol reagent kit (Invitrogen, Carlsbad, CA, USA) and then PrimeScript™ RT reagent Kit (Takara, Dalian, China) was used to synthesize cDNA. Real-time quantitative PCR (qRT-PCR) was used to measure the mRNA expression levels (Supplementary Table 2) with TB Green® Premix Ex Taq™ II (Takara, Dalian, China) and CFX Connect Real-Time PCR Detection System. The amplification procedure was as follows: 95°C, 30 s; followed by 40 cycles (95°C, 5 s; 60°C, 30 s) and stored at 4°. Quantitative results were analyzed by the 2−ΔΔCt method for relative expression. All samples contained 3 biological replicates and 3 technical replicates. All data are expressed as Mean ± SD. The unpaired two-tailed Student's t-test was used for statistical analysis. In this study, lowercase letters a-g were used to indicate significance (P < 0.05).
Results
Construction of co-expression network and identification of muscle-related modules
GSE158412 dataset contains 16 tissue samples of cattle. Before the analysis, we first tested the credibility of biological repetition of the samples by principal component analysis, and the result showed that there was clustering between 2 repeats of 8 tissues (Figure 2A). All samples could be used for analysis. After processing raw data, the gene expression matrix for 10,034 protein-coding genes was obtained. Among them, 7,525 genes (the top 75% of the median absolute deviation) were used to construct the weighted co-expression network. The Pearson's correlation coefficient (PCC) was performed to construct the sample clustering tree (Figure 2B). Then, WGCNA's pickSoftThreshold function was selected to estimate the best value of power (β). In this study, the best value of power was estimated as 9 (β = 9) (scale-free R2 = 0.85) (Figure 2C). The scale-free topology was performed to test the reliability of β = 9 (Figure 2D). Furthermore, the dynamic hierarchical tree cutting algorithm was used to detect common expression modules, and then similar modules (minimum height was set to 0.25) were combined (Figure 2E). Finally, a total of 18 modules were identified (Figures 2E,F). The eigengenes contained in each module was provided in Supplementary Table 3. The correlation between modules and samples was shown in Figure 3A. We found that four modules were highly correlated with muscle tissue, namely MEcyan (cor = 0.74, P = 0.001), MEmidnightblue (cor = 0.75, P = 8E-04), MEgrey60 (cor = 0.74, P = 0.001) and MElightyellow (cor = 0.97, P = 1E-09). The adjacency heatmap of the relationship for each model was shown in Figure 3B. In addition, GS and MM were highly correlated (cor = 0.89, P =1.8E-178), suggesting that genes that are highly correlated with the characteristics of muscle samples are also the most important (central) elements of modules associated with that sample (Figure 3C). Through roughly performing GO annotation on eigengenes of each module, we found that ME module with the highest correlation to muscle development, including regulation of muscle adaptation and muscle system process, etc. (Figure 3D). Therefore, this study finally extracted the MElightyellow module for further analysis. In MElightyellow, 213 out of 519 genes (~41%) met the screening conditions of hub genes (MM > 0.8, GS > 0.6, P.weighted < 0.01) in Supplementary Table 4.
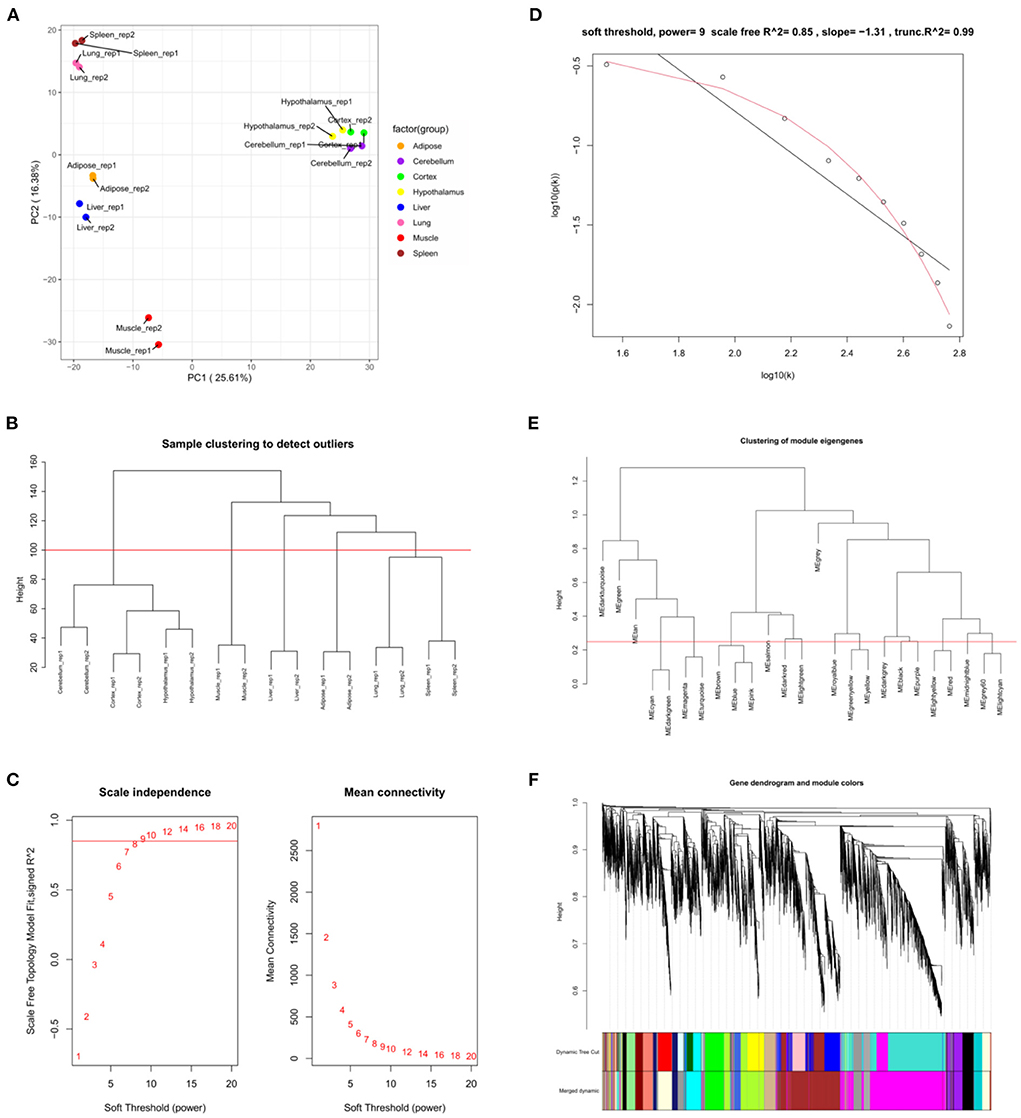
Figure 2. Weighted co-expression network analysis. (A) Principal component analysis of all samples. (B) Sample clustering tree. The red line represents the outlier elimination reference line; (C) Soft-threshold powers (β) filtering analysis. The mean connectivity (y-axis) for various soft-threshold powers (x-axis) was showed on the left, and the mean connectivity (y-axis) of different soft-thresholding power (x-axis) was showed on the right; (D) Checking the scale-free topology when β = 9. X-axis: the log10 (network connectivity), y-axis: log10 (the corresponding frequency distribution). The distribution approximates a straight line, which is called the approximately scale-free topology; (E) The clusting of module eigengenes. The red line represents the reference line for similar module merging; (F) The cluster dendrogram of modules.
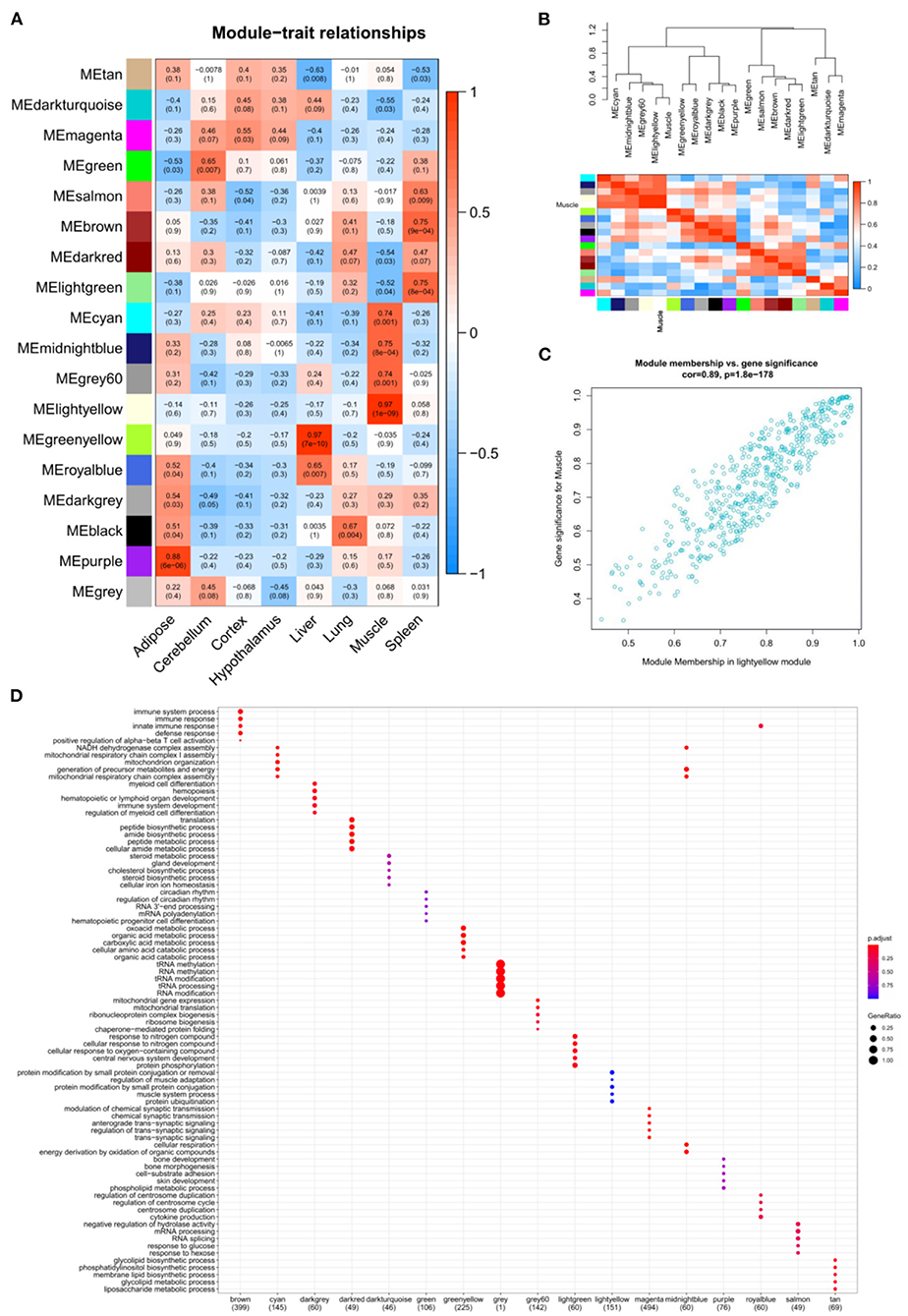
Figure 3. The relation analysis between modules and samples. (A) Heatmap of the module-sample correlation. Significance (P-value) was marked in parentheses. The color bar represented the magnitude of the correlation. Red: positive-correlation, blue: negative-correlation; (B) The adjacency heatmap of eigengene, including the module clustering tree (top) and the corresponding module clustering heatmap (below). The color bar represented the magnitude of the correlation. Red: positive-correlation, blue: negative-correlation; (C) The scatterplot of GS and MM in MElightyellow module; (D) The top 5 significant GO-BP analysis form each module eigengenes.
Screening of candidate hub genes with differential expression
In this section, 10,034 protein-coding genes were analyzed to identify muscle-specific DEGs. The result showed that a total of 4,815 DEGs were found, including 1,697 DEGs (up-regulated) in the muscle group and 3,118 DEGs (down-regulated) in the other-group. The analysis profile of DEGs was visualized in Figure 4A. The top 10 up-regulated DEGs were CKM, TNNC1, MYOT, SLN, ACTA1, MYL1, TNNC2, MYLPF, MYH2, and MYL2, and the top 10 down-regulated DEGs were GAD2, CLVS2, NRXN3, CELF3, ST8SIA3, GRM5, GRIN1, SCRT1, GRIN2A, and MYT1 (Table 1; Supplementary Table 5). GO and KEGG results are provided in the supplied materials (Supplementary Tables 6–9). Further analysis found that 212 candidate hub genes (no include PREB) related to muscle development were finally screened by integration difference analysis and WGCNA analysis (Figure 4B). GO analysis was performed on these 212 candidate hub genes with different changes, and the result showed that these genes were also enriched in terms related to muscle development (Figure 4C; Supplementary Table 10), demonstrating the feasibility of integrating differential analysis and WGCNA to obtain muscle-related hub genes.
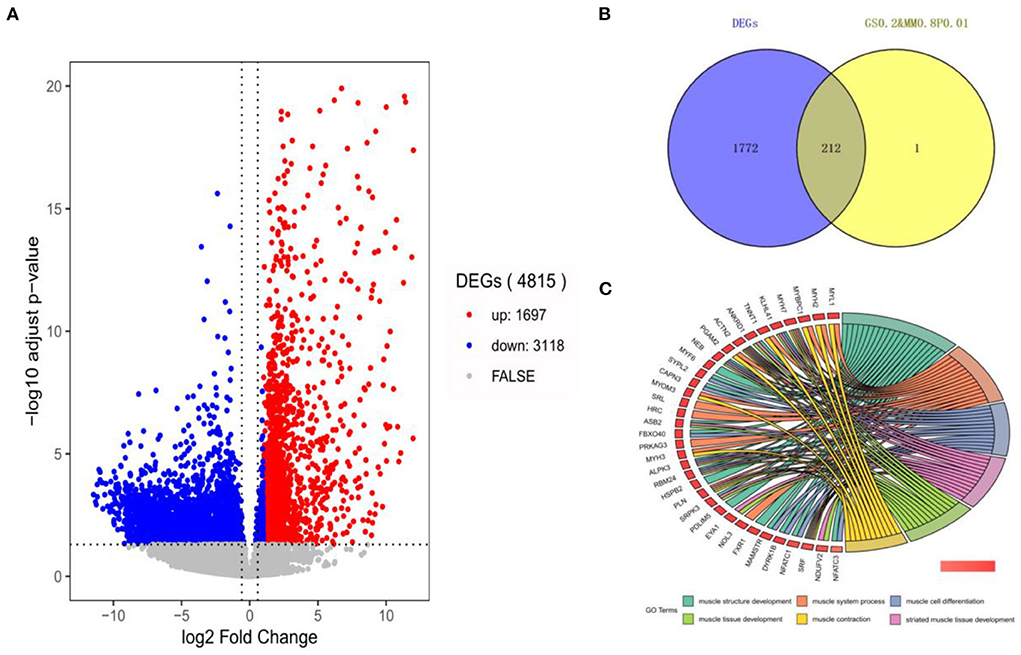
Figure 4. The analysis of DEGs by RNA-seq. (A) The volcano-plot of DEGs; (B) The overlap-plot of DEGs and candidate hub genes in MElightyellow; (C) The top 6 GO terms with the high significance of 212 candidate hub genes.
The landscape of genomic chromatin accessibility
To analyze the influence of chromatin open region on the differential expression of the candidate hub genes, the ATAC-seq technique was applied for further analysis. The raw data of GSE158414 were re-analyzed to obtain the open chromatin region of each sample. Simply, clean reads were first obtained by removing low-quality reads and adaptors, and then mapped to the reference genome (Bos taurus). Genrich (https://github.com/jsh58/Genrich) is a new software for analyzing ATAC-seq. It can remove mitochondrial reads, PCR repeats, multiple mapping reads, and multiple biological repeats with just one command. So, Genrich software was used to call peak in this study. The number of peaks for each sample was counted and stored in Supplementary Table 11. To determine whether the sequencing fragments were the open regions of the genome, all samples were selected for the analysis of the distribution of fragment lengths. In all samples analyzed, the 100 bp single-nucleosome fragments in leftmost were found, rather than single or multiple-nucleosome fragments, which indicates that these peaks were from open regions of the genome (Supplementary Figure 1). All results showed the good quality of ATAC-seq. The TSS of genes with active transcription is often open, so the signal profile near TSS is another important factor to identify the quality of ATAC-seq.
The correlative heatmap of tissues was shown significant differences between muscles and other tissues (Figure 5A). Next, through analyzing the differential accessible peaks, 8,963 different peaks were obtained (FDR < 0.05), including 4,564 muscle-affinitive peaks and 4,398 other-affinitive peaks in muscle vs. other. MA-plot of different peaks was shown in Figure 5B, and box-plot of binding affinity was shown in Figure 5C. The annotation result showed that most of the peaks were 10–100 kb away from TSS (Figure 5D). The distribution of these binding sites tends to be at the 3 'end of TSS, which is consistent with the results of previous studies (33). In addition, Figure 5E showed the peak enriched heatmap near TSS. Many peaks enriched near the TSS (±3 kb) of genes, indicating that transcription factors are likely to bind to these chromatin open regions.
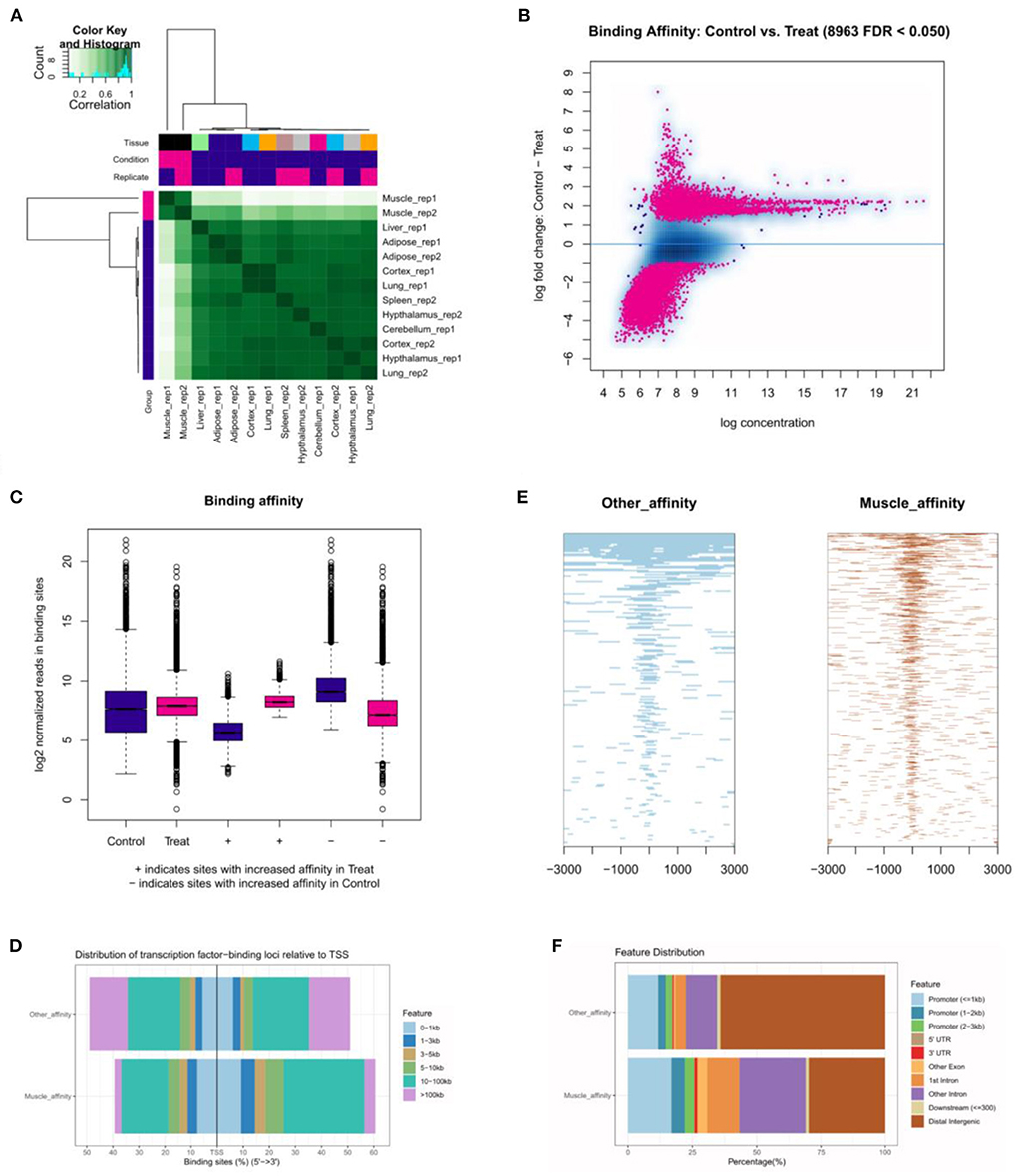
Figure 5. The landscape of genomic chromatin accessibility. (A) The correlative heatmap of tissues; (B) MA plot of difference peaks analysis; (C) The box-plot of binding affinity; (D) The location distribution of different peaks distance TSS; (E) The heatmap of peaks around TSS; (F) The distribution of function regions of different peaks.
To further explore the functional differences between muscle-affinitive peaks and other-affinitive peaks, we performed gene functional region annotation, and found that compared with other-affinitive peaks, muscle-affinitive peaks were enriched in promoter and exon regions significantly. Enrichment of distal intergenic was significantly reduced (Figure 5F). Peaks located in the promoter (called promoter-peaks) are critical to gene expression, so we extracted the genes in the promoter region for GO and KEGG analysis. The GO-BP analysis indicated that muscle-affinitive genes were related to muscle structure development (GO:0061061), muscle cell development (GO:0055001), muscle system process (GO:0003012), and so on (Figure 6A). KEGG analysis found muscle-affinitive genes were involved in cAMP, cGMP-PKG, MAPK, and other related functional pathways (Figure 6B). In addition, we found that several key TFs of muscle development were found to be significantly enriched at these ATAC-seq peaks (Figure 6C), suggesting muscle development may be related to the binding of transcription factors to open regions of chromatin.
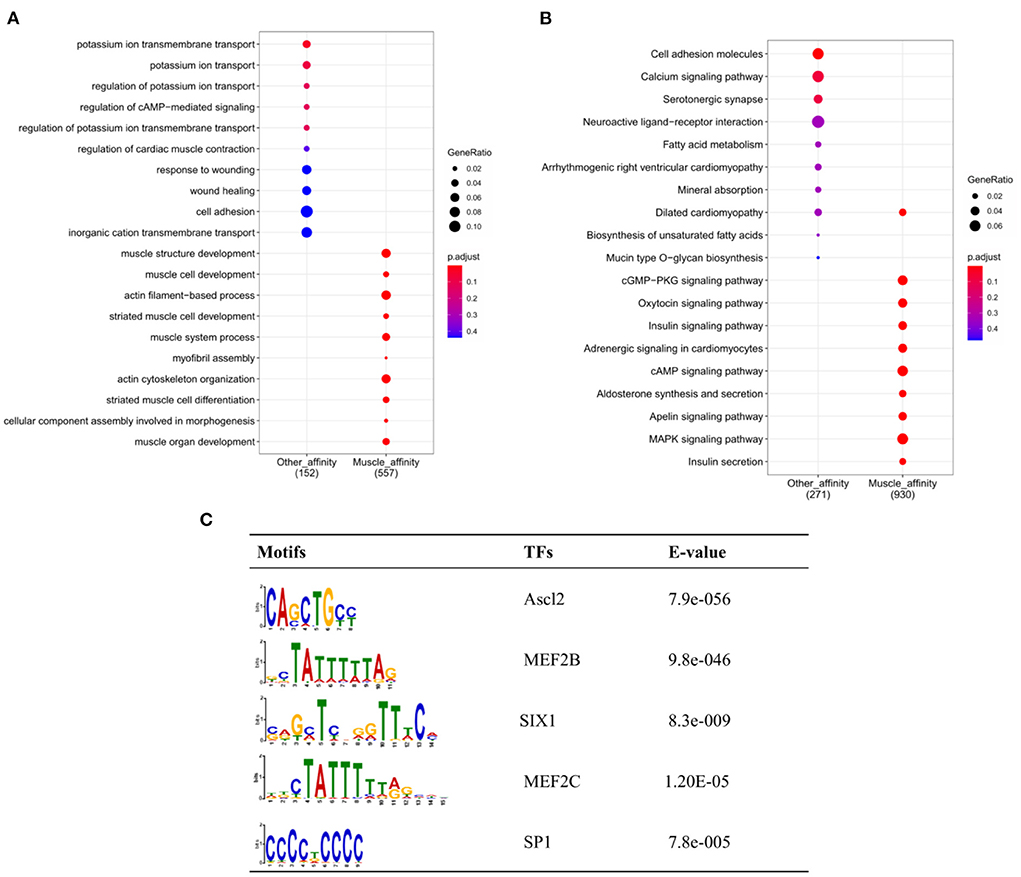
Figure 6. Genomic enrichment analysis. (A) GO-BP analysis of different peaks; (B) Enrichment analysis of KEGG pathway; (C) The top 5 predicted binding known motif in the open chromatin region.
Integration analysis of ATAC-seq and RNA-seq
To further determine the relationship between gene expression and open chromatin regions, we overlapped the analysis results from RNA-seq and ATAC-seq, respectively. Fifty-four common muscle-regulated genes were found (Figure 7A). The expression heatmap of these muscle-regulated genes was shown in Figure 7B, and we found these genes have a significant difference between muscle and other tissues. The top 20 genes with high differential expression were shown in Figure 7C, including MYOT, MYOM3, Mymyon (MB), Kelch Like Family Member 41 (KLHL41), Actinin Alpha 2 (ACTN2), and CAPN3 and other muscle-specific expression genes. Again, TF binding analysis was performed on the open chromatin regions corresponding to these 54 genes. The promoter-peaks of 44 of the 54 genes (~81.48%) were enriched by MEF2C which was the only transcription factor discovered (Figure 7D). The motif footprint analysis of MEF2C was showed in Figure 7E. In addition, we extracted the promoter regions of the 54 genes (upstream 2,000 bp and downstream 100 bp) using bedtools, and analyzed whether the MEF2C promoter is binding in these genes. Twenty-two of 54 hub genes (50%) were found to have MEF2C binding sites (P < 0.0001) (Figure 7F). By visually selecting the interaction between these genes and the target genes in Melightylow with the value of weight > 0.3, MB, HRC, KLHL41, SRL, and ALPK3 was found that occupy important positions in the regulatory network (Figure 7G). They are muscle-specific and muscle-regulated genes, suggesting that the combination of ATAC-seq and RNA-seq make our screening more accurate.
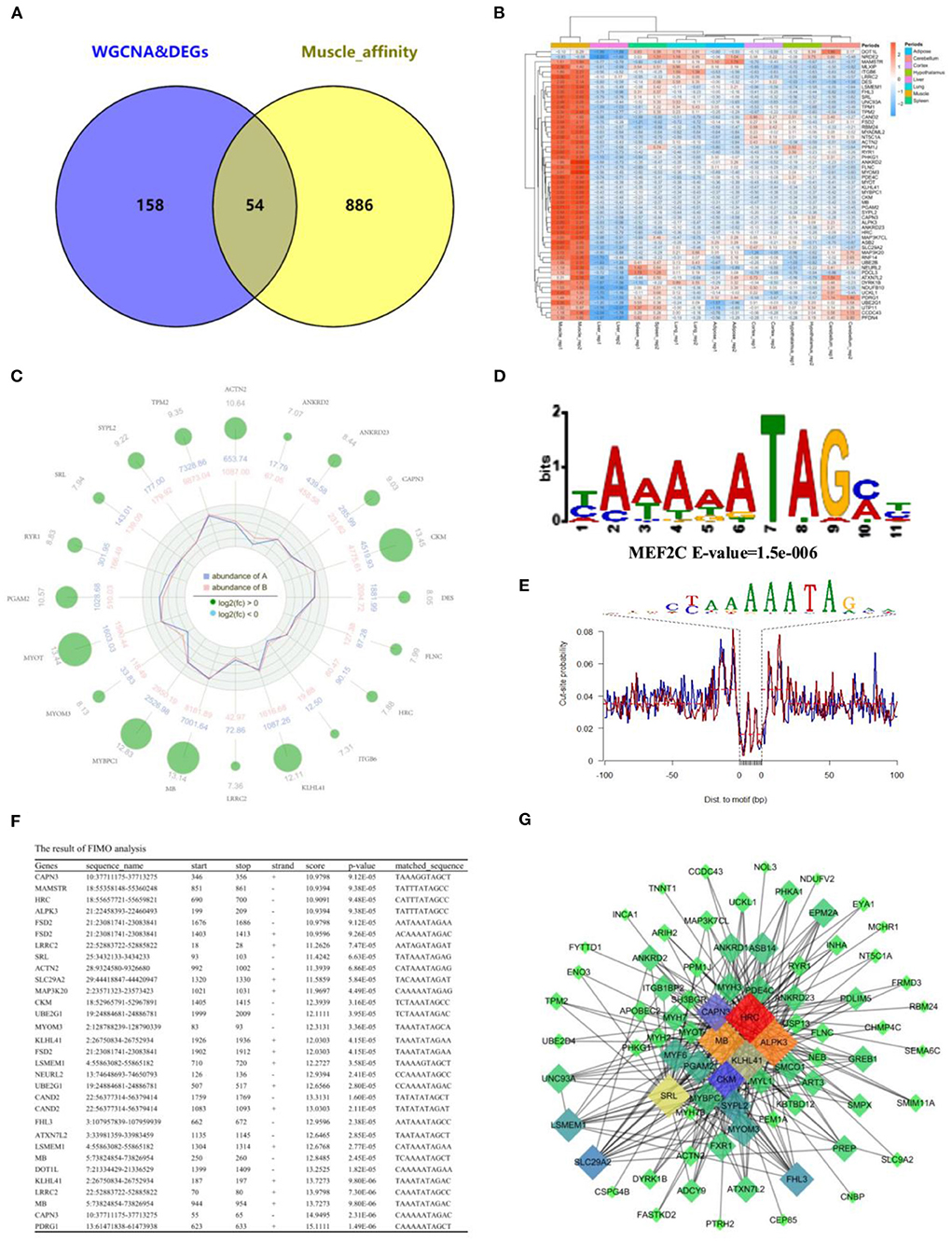
Figure 7. Integration of RNA-Seq and ATAC-Seq. (A) Venn plot of ATAC-seq and RNA-seq, and 54 common genes were found; (B) Heatmap plot of common genes; (C) Radar plot of the top 20 common genes; (D) Motif enrichment analysis of common genes around open chromatin regions. MEF2C was found to be significantly enriched; (E) The motif footprint analysis of MEF2C; (F) Motif Scanning of MEF2C in promoter region of common gene using FIMO; (G) The subregulatory network of 22 predicted MEF2C potential target genes in the MElight module was derived from WGCNA analysis.
Validate the expression of hub genes
To further confirm our results, 4 high-expression hub genes in muscle, including CAPN3 and KLHL41 targeted by MEF2C, and dual specificity tyrosine phosphorylation regulated kinase 1B (DYRK1B) and MLX interacting protein (MLXIP), were randomly selected for qRT-PCR. The results showed that the expression of these four genes in muscle tissue was significantly higher than that in other tissues, which was highly consistent with the results of RNA-seq (Figure 8A). In addition, we quantified the expression of these four genes in the process of myocyte differentiation, and found that their expression levels increased with the increase of the degree of differentiation of muscle cells, suggesting these genes might also play a crucial role in the differentiation (Figure 8B). Therefore, the screening of muscle-affinitive hub genes through ATAC-seq and RNA-seq can be further studied in the future on the molecular mechanism of muscle proliferation and differentiation.
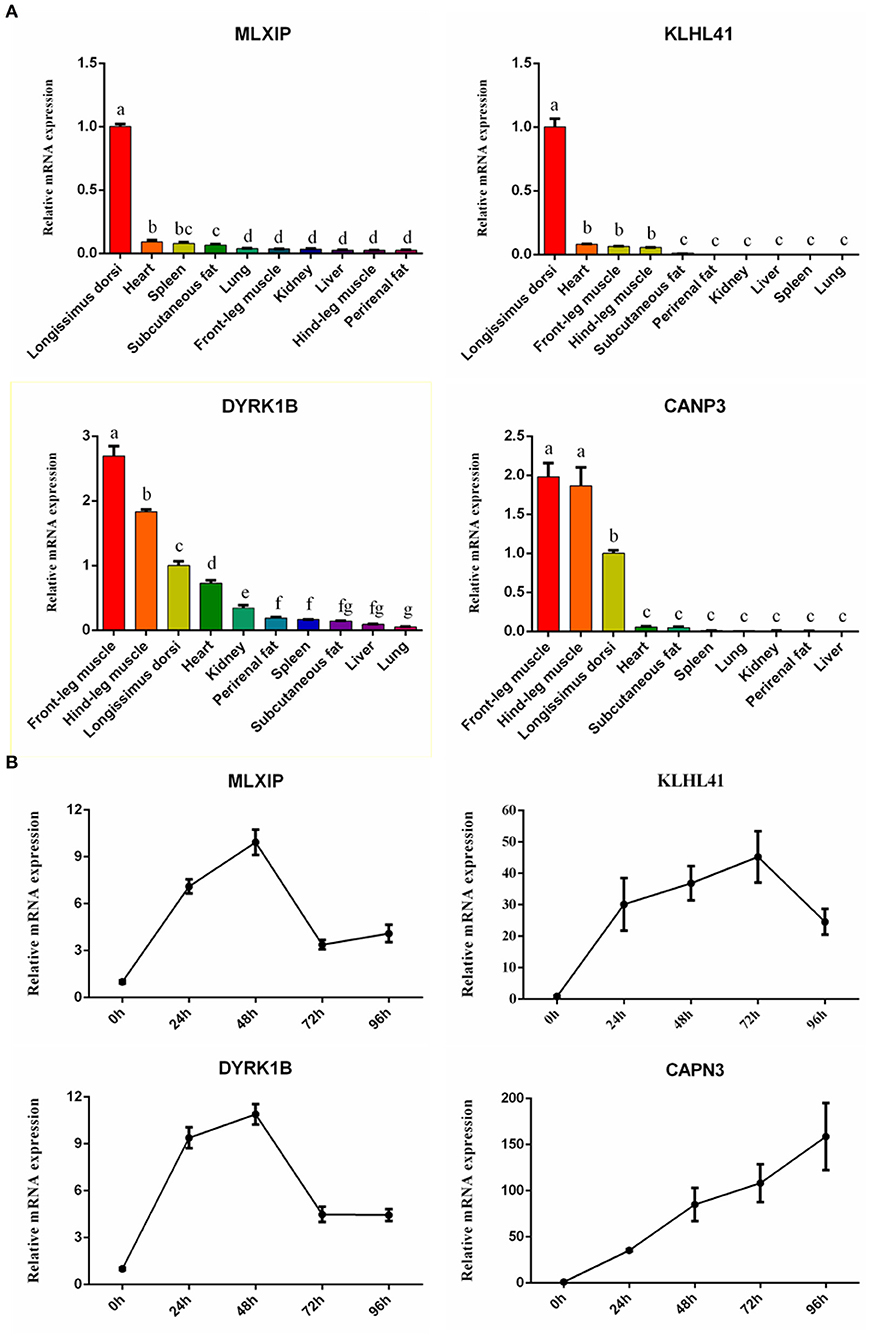
Figure 8. The relative expression of hub genes by qRT-PCR. (A) The bar plots represented tissue expression profiles. (B) The line charts represented myogenic cell differentiation expression profiles.
Discussion
Different from other tissues, the development of muscle, the main product of beef cattle, directly affects the economic value of beef. To improve beef yield from a genetic perspective, many novel techniques have been applied to explore the mechanism of muscle development in cattle. Among them, bulk RNA-seq has been widely promoted as a routine bulk screening technique for muscle-related genes (34, 35). However, the differentially expressed genes screened by it are often in thousands, and it is still difficult to obtain the core regulatory genes from them. In contrast, weighted gene co-expression network analysis (WGCNA) could be used to characterize correlation patterns between genes and samples (21). Candidate hub genes were identified according to the endogeneity of gene-sets and the association between gene-sets and samples (36). Compared to focusing only on differentially expressed genes, WGCNA is able to use genome-wide information to obtain our gene sets of interest, which not only narrows the screening scope but also makes the analysis more accurate. This technique has been applied extensively to plants, humans, mice, poultry, and livestock (36–41).
In this study, we analyzed the association of genes with different tissues using WGCNA, and obtained 4 module gene-sets with high positive correlation with muscle tissue. Combined with the results of GO functional annotation, we finally targeted MElightyellow as the study target, from which we screened 213 candidate hub genes (GS > 0.6, MM > 0.8) involved in the positive regulation of muscle development. Compared with other tissues, 212 of 213 (99.53%) candidate hub genes were up-regulated expression in muscle [log2(FC) > 1, FDR < 0.05], which not only verified the robustness of WGCNA analysis, but also provided a reliable collection for the subsequent study.
In eukaryotes, DNA and histones are tightly bound and stored in nucleosomes (chromatin), chromatin structure, nucleosome location and histone modifications affect DNA transcription. Chromatin accessibility is closely related to the binding of regulatory elements or transcription factors, which are particularly important for gene activation and repression. ATAC-seq, an innovative technique for detecting chromatin accessibility, has been increasingly applied to determine the mechanisms of gene expression regulation with RNA-seq (42). In this study, we found that the chromatin open regions of muscle tissue were significantly different from other tissues. The results of functional annotation revealed that muscle-affinitive peaks were more enriched in exons and promoter regions compared to other tissues, suggesting that these chromatin-open regions are associated with gene expression regulation. In addition, we performed GO and KEGG analysis of DEGs around promoter-peaks and found that muscle-affinitive peaks were enriched on the hub genes specifically expressed by muscle as expected. It was involved in the regulation of cAMP, cGMP-PKG, and MAPK signaling pathways. Similar results were obtained in early studies on mouse tissue-specific genes (43). Among them, MAPK signaling pathway plays a crucial role in in the formation, regeneration, movement and injury repair of skeletal muscle (44–46).
Combined with ATAC-seq, 54 genes with high expression in muscle were identified as muscle-regulated hub genes. To further analyze the regulatory mechanism of these 54 hub genes in the development of skeletal muscle, we conducted motif analysis of the chromatin open region fragments. Twenty-two of the 54 genes were predicted to be regulated by MEF2C. This is consistent with earlier tissue analysis in mice (47). MEF2C is a member of the myocyte enhancer factor 2 family, which itself lacks myogenic activity and was initially considered to regulate muscle development by activating the transcriptional activity of bHLH myogen proteins (such as MyoD and MyoG) (48–50). Herein, we analyzed the possible MEF2C involved in downstream target genes, we broadly classified them into 3 major categories: 1. The muscle-specific genes, including CAPN3, ACTN2, MB, KLHL41, Cullin associated and neddylation dissociated 2 (CAND2), Myomesin 3 (MYOM3) and sarcalumenin (SRL); 2. The genes of kinases and epigenetic enzymes, including creatine kinase, M-Type (CKM), alpha kinase 3 (ALPK3), mitogen-activated protein kinase kinase kinase 20 (MAP3K20), ubiquitin conjugating enzyme E2 G1 (UBE2G1), Neuralized E3 Ubiquitin protein ligase 2 (NEURL2) and DOT1 like Histone lysine methyltransferase (DOT1L); 3. other genes with transcriptional regulation functions, including Histidine rich calcium binding protein (HRC), MEF2 activating motif and SAP domain containing transcriptional regulator (MAMSTR), fibronectin type III and SPRY domain containing 2 (FSD2), leucine rich repeat containing 2 (LRRC2), leucine rich single-pass membrane protein 1 (LSMEM1), solute carrier family 29 member 2 (SLC29A2), four and a half LIM domains 3 (FHL3), Ataxin 7 like 2 (ATXN7L2) and p53 and DNA damage regulated 1 (PDRG1).
In this study, some well-known myogenic genes have been reported to be involved in myoblast proliferation and differentiation dependent on the transcriptional regulation of MEF2, such as CAPN3, MB, Myomesin, and CKM (51–54). More interestingly, HRC, a direct target gene of MEF2, encodes histidine-rich calcium-binding protein (HRCBP) involved in the regulation of muscle development mainly in the sarcoplasmic reticulum of cardiac and skeletal muscles and in the calcium bodies of smooth muscle (55, 56). MASTR, a coactivator of MEF2, is involved in the regulation of myoblast proliferation and differentiation by encoding a cofactor that stimulates MEF2C (57, 58). These studies confirm the reliability of the results of this study. Other genes have not been reported to interact directly with MEF2C, but many have been reported to be involved in the regulation of muscle. It has been reported that ACTN2 and its family gene ACTN3 encode myosin α-actin-2 and α-actin-3 proteins, respectively, which constitute the Z-line in mammalian skeletal muscle fibers (59). SRL is a Ca2+-binding protein localized in the sarcoplasmic reticulum (SR) affecting skeletal muscle movement (60). MAP3K20 is involved in the regulation of the JNK/MAPK signaling pathway, which plays a role in muscle development and regeneration (61). UBE2G1 encodes an E2 ubiquitin-coupled enzyme that functions mainly in the ubiquitin-proteasome system, which is involved in muscle degradation and regeneration (62). NEURL2 is capable of encoding proteins involved in the regulation of myogenic fiber organization and ubiquitin-mediated degradation of β-linked proteins during myogenesis (63). DOT1L is a key epigenetic gene that mediates H3K79me2 modifications involved in cardiomyocyte differentiation (64). LRRC2 is a member of the LRRC family and its role in epigenetic modifications in skeletal muscle has also been reported (65). KLHL41 is predominantly expressed in skeletal muscle and is essential for the maintenance of skeletal muscle integrity and myogenic fiber formation (66, 67). FHL3 has been reported to regulate myogenic differentiation and muscle-specific gene expression by acting as a transcriptional co-activator or co-repressor (68). FSD2 is highly expressed in cardiac and skeletal muscle as a candidate gene affecting sarcomere traits in animals (69). CAND2 and is a muscle-specific expression gene mediated by mTORC1 that affects cardiac remodeling but not skeletal muscle (70). ALPK3 has been reported to be closely associated with familial cardiomyopathy, but its role in skeletal muscle has not been reported (71). In this study, ALPK3 was located at the core of the interaction network of candidate genes with MB, HRC, SRL, and KLHL41, so we speculate that it also is irreplaceable for skeletal development. LSMEM1, SLC29A2, ATXN7L2, and PDRG1 have not been directly reported to be associated with muscle development. In conclusion, these genes are worthy of our attention and their potential regulatory role with transcription factors and their epistatic modifications in muscle development is of great interest to explore.
Notably, this study used other tissues as the background and only contested the analysis of genes with positive regulation with muscle. In fact, there are many more information that can be mined to be further analyzed and verified, such as other tissue-related genes, functional analysis of other modular genes, and joint analysis with other epistatic data.
Data availability statement
The datasets presented in this study can be found in online repositories. The names of the repository/repositories and accession number(s) can be found in the article/Supplementary material.
Ethics statement
The animal study was reviewed and approved by Ethics Committee of Northwest A&F University.
Author contributions
JW, BL, and LZ conceived and designed the experiments. JW wrote the manuscript. XY, CL, SR, YP, and KZ contributed sample collection and reagents preparation, and analyzed the data. JW, BL, XY, CL, SR, YP, KZ, and LZ revised the manuscript. All authors reviewed the manuscript. All authors contributed to the article and approved the submitted version.
Funding
This work was supported by the Natural Science Foundation of China (31972994), Key Research and Development Program of Ningxia Province (2019BEF02004), National Beef and Yak Industrial Technology System (CARS-37), Key Research and Development Program of Shaanxi Province (2022NY-050 and 2022ZDLNY01-01), Transformation Project of Shaanxi Province (NYKJ-2018-LY09) and Special Project for the Central Government to Guide Local Science and Technology Development (2060404-51301).
Conflict of interest
The authors declare that the research was conducted in the absence of any commercial or financial relationships that could be construed as a potential conflict of interest.
Publisher's note
All claims expressed in this article are solely those of the authors and do not necessarily represent those of their affiliated organizations, or those of the publisher, the editors and the reviewers. Any product that may be evaluated in this article, or claim that may be made by its manufacturer, is not guaranteed or endorsed by the publisher.
Supplementary material
The Supplementary Material for this article can be found online at: https://www.frontiersin.org/articles/10.3389/fvets.2022.925590/full#supplementary-material
References
1. Picard B, Berri C, Lefaucheur L, Molette C, Sayd T, Terlouw C. Skeletal muscle proteomics in livestock production. Brief Funct Genom. (2010) 9:259–78. doi: 10.1093/bfgp/elq005
2. Zhang Z, Liao Q, Sun Y, Pan T, Liu S, Miao W, et al. Lipidomic and transcriptomic analysis of the longissimus muscle of luchuan and duroc pigs. Front Nutr. (2021) 8:667622. doi: 10.3389/fnut.2021.667622
3. Yang XJ, Albrecht E, Ender K, Zhao RQ, Wegner J. Computer image analysis of intramuscular adipocytes and marbling in the longissimus muscle of cattle. J Anim Sci. (2006) 84:3251–8. doi: 10.2527/jas.2006-187
4. Huo W, Weng K, Gu T, Zhang Y, Zhang Y, Chen G, et al. Effect of muscle fiber characteristics on meat quality in fast- and slow-growing ducks. Poult Sci. (2021) 100:101264. doi: 10.1016/j.psj.2021.101264
5. Ohlendieck K. Skeletal muscle proteomics: current approaches, technical challenges and emerging techniques. Skeletal Muscle. (2011) 1:6. doi: 10.1186/2044-5040-1-6
6. Esteves de Lima J, Relaix F. Master regulators of skeletal muscle lineage development and pluripotent stem cells differentiation. Cell Regen. (2021) 10:31. doi: 10.1186/s13619-021-00093-5
7. Taylor MV, Hughes SM. Mef2 and the skeletal muscle differentiation program. Semin Cell Dev Biol. (2017) 72:33–44. doi: 10.1016/j.semcdb.2017.11.020
8. Molkentin JD, Black BL, Martin JF, Olson EN. Mutational analysis of the DNA binding, dimerization, and transcriptional activation domains of MEF2C. Mol Cell Biol. (1996) 16:2627–36. doi: 10.1128/MCB.16.6.2627
9. Black BL, Olson EN. Transcriptional control of muscle development by myocyte enhancer factor-2 (MEF2) proteins. Ann Rev Cell Dev Biol. (1998) 14:167–96. doi: 10.1146/annurev.cellbio.14.1.167
10. Schiaffino S, Serrano A. Calcineurin signaling and neural control of skeletal muscle fiber type and size. Trends Pharmacol Sci. (2002) 23:569–75. doi: 10.1016/S0165-6147(02)02111-9
11. McKinsey TA, Zhang CL, Olson EN. MEF2: a calcium-dependent regulator of cell division, differentiation and death. Trends Biochem Sci. (2002) 27:40–7. doi: 10.1016/S0968-0004(01)02031-X
12. Luo L, Gribskov M, Wang S. Bibliometric review of ATAC-Seq and its application in gene expression. Brief Bioinform. (2022) 23:bbac061. doi: 10.1093/bib/bbac061
13. Kern C, Wang Y, Xu X, Pan Z, Halstead M, Chanthavixay G, et al. Functional annotations of three domestic animal genomes provide vital resources for comparative and agricultural research. Nat Commun. (2021) 12:1821. doi: 10.1038/s41467-021-22100-8
14. Alexandre PA, Naval-Sánchez M, Menzies M, Nguyen LT, Porto-Neto LR, Fortes MRS, et al. Chromatin accessibility and regulatory vocabulary across indicine cattle tissues. Genome Biol. (2021) 22:273. doi: 10.1186/s13059-021-02489-7
15. Leinonen R, Sugawara H, Shumway M, International Nucleotide Sequence Database C. The sequence read archive. Nucleic Acids Res. (2011) 39:D19–21. doi: 10.1093/nar/gkq1019
16. Bolger AM, Lohse M, Usadel B. Trimmomatic: a flexible trimmer for illumina sequence data. Bioinformatics. (2014) 30:2114–20. doi: 10.1093/bioinformatics/btu170
17. Kim D, Langmead B, Salzberg SL. HISAT: a fast spliced aligner with low memory requirements. Nat Methods. (2015) 12:357–60. doi: 10.1038/nmeth.3317
18. Li H, Handsaker B, Wysoker A, Fennell T, Ruan J, Homer N, et al. The sequence alignment/Map format and SAMtools. Bioinformatics. (2009) 25:2078–9. doi: 10.1093/bioinformatics/btp352
19. Liao Y, Smyth GK, Shi W. featureCounts: an efficient general purpose program for assigning sequence reads to genomic features. Bioinformatics. (2014) 30:923–30. doi: 10.1093/bioinformatics/btt656
20. Pertea M, Pertea GM, Antonescu CM, Chang TC, Mendell JT, Salzberg SL. StringTie enables improved reconstruction of a transcriptome from RNA-seq reads. Nat Biotechnol. (2015) 33:290–5. doi: 10.1038/nbt.3122
21. Langfelder P, Horvath S. WGCNA: an R package for weighted correlation network analysis. BMC Bioinformatics. (2008) 9:559. doi: 10.1186/1471-2105-9-559
22. Tian Z, He W, Tang J, Liao X, Yang Q, Wu Y, et al. Identification of important modules and biomarkers in breast cancer based on WGCNA. OncoTargets therapy. (2020) 13:6805–17. doi: 10.2147/OTT.S258439
23. Love MI, Huber W, Anders S. Moderated estimation of fold change and dispersion for RNA-seq data with DESeq2. Genome Biol. (2014) 15:550. doi: 10.1186/s13059-014-0550-8
24. Li H, Durbin R. Fast and accurate short read alignment with Burrows-Wheeler transform. Bioinformatics. (2009) 25:1754–60. doi: 10.1093/bioinformatics/btp324
25. Johnston D, Kim J, Taylor JF, Earley B, McCabe MS, Lemon K, et al. ATAC-Seq identifies regions of open chromatin in the bronchial lymph nodes of dairy calves experimentally challenged with bovine respiratory syncytial virus. BMC Genomics. (2021) 22:14. doi: 10.1186/s12864-020-07268-5
26. Yu G, Wang L-G, He Q-Y. ChIPseeker: an R/Bioconductor package for ChIP peak annotation, comparison and visualization. Bioinformatics. (2015) 31:2382–3. doi: 10.1093/bioinformatics/btv145
27. Bailey TL, Williams N, Misleh C, Li WW. MEME: discovering and analyzing DNA and protein sequence motifs. Nucleic Acids Res. (2006) 34:W369–73. doi: 10.1093/nar/gkl198
28. Ou J, Liu H, Yu J, Kelliher MA, Castilla LH, Lawson ND, et al. ATACseqQC: a Bioconductor package for post-alignment quality assessment of ATAC-seq data. BMC Genomics. (2018) 19:169. doi: 10.1186/s12864-018-4559-3
29. Grant CE, Bailey TL, Noble WS. FIMO: scanning for occurrences of a given motif. Bioinformatics. (2011) 27:1017–8. doi: 10.1093/bioinformatics/btr064
30. Yu G, Wang LG, Han Y, He QY. clusterProfiler: an R package for comparing biological themes among gene clusters. OMICS. (2012) 16:284–7. doi: 10.1089/omi.2011.0118
31. Raudvere U, Kolberg L, Kuzmin I, Arak T, Adler P, Peterson H, et al. g:Profiler: a web server for functional enrichment analysis and conversions of gene lists (2019 update). Nucleic Acids Res. (2019) 47:W191–8. doi: 10.1093/nar/gkz369
32. Xie C, Mao X, Huang J, Ding Y, Wu J, Dong S, et al. KOBAS 2.0: a web server for annotation and identification of enriched pathways and diseases. Nucleic Acids Res. (2011) 39:W316–22. doi: 10.1093/nar/gkr483
33. Yang H, Li G, Qiu G. Bioinformatics analysis using ATAC-seq and RNA-seq for the identification of 15 gene signatures associated with the prediction of prognosis in hepatocellular carcinoma. Front Oncol. (2021) 11:726551. doi: 10.3389/fonc.2021.726551
34. Owens NDL, De Domenico E, Gilchrist MJ. An RNA-seq protocol for differential expression analysis. Cold Spring Harbor Protocols. (2019) 2019:pdb-rot098368. doi: 10.1101/pdb.prot098368
35. Mortazavi A, Williams BA, McCue K, Schaeffer L, Wold B. Mapping and quantifying mammalian transcriptomes by RNA-Seq. Nat Methods. (2008) 5:621–8. doi: 10.1038/nmeth.1226
36. Ding H, Lin Y, Zhang T, Chen L, Zhang G, Wang J, et al. Transcriptome analysis of differentially expressed mRNA related to pigeon muscle development. Animals. (2021) 11:2311. doi: 10.3390/ani11082311
37. Yang J, Ren Y, Zhang D, Chen X, Huang J, Xu Y, et al. Transcriptome-based WGCNA analysis reveals regulated metabolite fluxes between floral color and scent in Narcissus tazetta flower. Int J Mol Sci. (2021) 22:8249. doi: 10.3390/ijms22158249
38. Chen L, Bai J, Li Y. miR-29 mediates exercise-induced skeletal muscle angiogenesis by targeting VEGFA, COL4A1 and COL4A2 via the PI3K/Akt signaling pathway. Mol Med Rep. (2020) 22:661–70. doi: 10.3892/mmr.2020.11164
39. Ren K, Wang L, Wang L, Du Q, Cao J, Jin Q, et al. Investigating transcriptional dynamics changes and time-dependent marker gene expression in the early period after skeletal muscle injury in rats. Front Genet. (2021) 12:650874. doi: 10.3389/fgene.2021.650874
40. Zhao X, Hu H, Lin H, Wang C, Wang Y, Wang J. Muscle transcriptome analysis reveals potential candidate genes and pathways affecting intramuscular fat content in pigs. Front Genet. (2020) 11:877. doi: 10.3389/fgene.2020.00877
41. Bao Q, Zhang X, Bao P, Liang C, Guo X, Chu M, et al. Using weighted gene co-expression network analysis (WGCNA) to identify the hub genes related to hypoxic adaptation in yak (Bos grunniens). Genes Genom. (2021) 43:1231–46. doi: 10.1007/s13258-021-01137-5
42. Buenrostro JD, Wu B, Chang HY, Greenleaf WJ. ATAC-seq: a method for assaying chromatin accessibility genome-wide. Curr Protoc Mol Biol. (2015) 109:21–9. doi: 10.1002/0471142727.mb2129s109
43. Raza SHA, Liang C, Guohua W, Pant SD, Mohammedsaleh ZM, Shater AF, et al. Screening and identification of muscle-specific candidate genes via mouse microarray data analysis. Front Vet Sci. (2021) 8:794628. doi: 10.3389/fvets.2021.794628
44. Keren A, Tamir Y, Bengal E. The p38 MAPK signaling pathway: a major regulator of skeletal muscle development. Mol Cell Endocrinol. (2006) 252:224–30. doi: 10.1016/j.mce.2006.03.017
45. Tomida T, Adachi-Akahane S. Roles of p38 MAPK signaling in the skeletal muscle formation, regeneration, and pathology. Nihon yakurigaku zasshi Folia pharmacologica Japonica. (2020) 155:241–7. doi: 10.1254/fpj20030
46. Kramer HF, Goodyear LJ. Exercise, MAPK, and NF-kappaB signaling in skeletal muscle. J Appl Physiol. (2007) 103:388–95. doi: 10.1152/japplphysiol.00085.2007
47. Liu C, Wang M, Wei X, Wu L, Xu J, Dai X, et al. An ATAC-seq atlas of chromatin accessibility in mouse tissues. Sci Data. (2019) 6:65. doi: 10.1038/s41597-019-0071-0
48. Molkentin JD, Black BL, Martin JF, Olson EN. Cooperative activation of muscle gene expression by MEF2 and myogenic bHLH proteins. Cell. (1995) 83:1125–36. doi: 10.1016/0092-8674(95)90139-6
49. Wang DZ, Valdez MR, McAnally J, Richardson J, Olson EN. The Mef2c gene is a direct transcriptional target of myogenic bHLH and MEF2 proteins during skeletal muscle development. Development. (2001) 128:4623–33. doi: 10.1242/dev.128.22.4623
50. Cante-Barrett K, Pieters R, Meijerink JP. Myocyte enhancer factor 2C in hematopoiesis and leukemia. Oncogene. (2014) 33:403–10. doi: 10.1038/onc.2013.56
51. Wu R, Wang J, Yao J, Dong Z, Liu Y, Liu M. MEF2A regulates Calpain 3 expression in L6 myoblasts. Gene. (2018) 668:204–10. doi: 10.1016/j.gene.2018.05.056
52. Grayson J, Williams RS, Yu YT, Bassel-Duby R. Synergistic interactions between heterologous upstream activation elements and specific TATA sequences in a muscle-specific promoter. Mol Cell Biol. (1995) 15:1870–8. doi: 10.1128/MCB.15.4.1870
53. Potthoff MJ, Arnold MA, McAnally J, Richardson JA, Bassel-Duby R, Olson EN. Regulation of skeletal muscle sarcomere integrity and postnatal muscle function by Mef2c. Mol Cell Biol. (2007) 27:8143–51. doi: 10.1128/MCB.01187-07
54. Rampalli S, Li L, Mak E, Ge K, Brand M, Tapscott SJ, et al. p38 MAPK signaling regulates recruitment of Ash2L-containing methyltransferase complexes to specific genes during differentiation. Nat Struct Mol Biol. (2007) 14:1150–6. doi: 10.1038/nsmb1316
55. Anderson JP, Dodou E, Heidt AB, De Val SJ, Jaehnig EJ, Greene SB, et al. HRC is a direct transcriptional target of MEF2 during cardiac, skeletal, and arterial smooth muscle development in vivo. Mol Cell Biol. (2004) 24:3757–68. doi: 10.1128/MCB.24.9.3757-3768.2004
56. Lee HG, Kang H, Kim DH, Park WJ. Interaction of HRC (Histidine-rich Ca2+-binding protein) and triadin in the lumen of sarcoplasmic reticulum*. J Biol Chem. (2001) 276:39533–8. doi: 10.1074/jbc.M010664200
57. Zhang L, Silva TC, Young JI, Gomez L, Schmidt MA, Hamilton-Nelson KL, et al. Epigenome-wide meta-analysis of DNA methylation differences in prefrontal cortex implicates the immune processes in Alzheimer's disease. Nat Commun. (2020) 11:6114. doi: 10.1038/s41467-020-19791-w
58. Creemers EE, Sutherland LB, Oh J, Barbosa AC, Olson EN. Coactivation of MEF2 by the SAP domain proteins myocardin and MASTR. Mol Cell. (2006) 23:83–96. doi: 10.1016/j.molcel.2006.05.026
59. Harada N, Gotoda Y, Hatakeyama A, Nakagawa T, Miyatake Y, Kuroda M, et al. Differential regulation of Actn2 and Actn3 expression during unfolded protein response in C2C12 myotubes. J Muscle Res Cell Motility. (2020) 41:199–209. doi: 10.1007/s10974-020-09582-7
60. Yoshida M, Minamisawa S, Shimura M, Komazaki S, Kume H, Zhang M, et al. Impaired Ca2+ store functions in skeletal and cardiac muscle cells from sarcalumenin-deficient mice. J Biol Chem. (2005) 280:3500–6. doi: 10.1074/jbc.M406618200
61. Yan J, Yang Y, Fan X, Liang G, Wang Z, Li J, et al. circRNAome profiling reveals circFgfr2 regulates myogenesis and muscle regeneration via a feedback loop. J Cachexia Sarcopenia Muscle. (2022) 13:696–712. doi: 10.1002/jcsm.12859
62. Santos Silva DBd, Fonseca LFS, Magalhães AFB, Muniz MMM, Baldi F, Ferro JA, et al. Transcriptome profiling of muscle in Nelore cattle phenotypically divergent for the ribeye muscle area. Genomics. (2020) 112:1257–63. doi: 10.1016/j.ygeno.2019.07.012
63. Nastasi T, Bongiovanni A, Campos Y, Mann L, Toy JN, Bostrom J, et al. Ozz-E3, a muscle-specific ubiquitin ligase, regulates β-Catenin degradation during myogenesis. Dev Cell. (2004) 6:269–82. doi: 10.1016/S1534-5807(04)00020-6
64. Cattaneo P, Kunderfranco P, Greco C, Guffanti A, Stirparo GG, Rusconi F, et al. DOT1L-mediated H3K79me2 modification critically regulates gene expression during cardiomyocyte differentiation. Cell Death Diff. (2016) 23:555–64. doi: 10.1038/cdd.2014.199
65. Ehrlich KC, Lacey M, Ehrlich M. Epigenetics of skeletal muscle-associated genes in the ASB, LRRC, TMEM, and OSBPL gene families. Epigenomes. (2020) 4:1. doi: 10.3390/epigenomes4010001
66. Pak JH. KLHL41 in Skeletal Muscle Development. Boston, MA: Boston University Theses & Dissertations (2019).
67. Ramirez-Martinez A, Cenik BK, Bezprozvannaya S, Chen B, Bassel-Duby R, Liu N, et al. KLHL41 stabilizes skeletal muscle sarcomeres by nonproteolytic ubiquitination. Elife. (2017) 6:e26439. doi: 10.7554/eLife.26439
68. Zhang Y, Li W, Zhu M, Li Y, Xu Z, Zuo B. FHL3 differentially regulates the expression of MyHC isoforms through interactions with MyoD and pCREB. Cell Signal. (2016) 28:60–73. doi: 10.1016/j.cellsig.2015.10.008
69. Lim K-S, Lee K-T, Lee S-W, Chai H-H, Jang G, Hong K-C, et al. Genomic structure, expression and association study of the porcine FSD2. Mol Biol Rep. (2016) 43:1011–8. doi: 10.1007/s11033-016-4029-4
70. Górska AA, Sandmann C, Riechert E, Hofmann C, Malovrh E, Varma E, et al. Muscle-specific Cand2 is translationally upregulated by mTORC1 and promotes adverse cardiac remodeling. EMBO Rep. (2021) 22:e52170. doi: 10.15252/embr.202052170
Keywords: muscle, RNA-seq, WGCNA, hub genes, ATAC-seq, transcription factor
Citation: Wang J, Li B, Yang X, Liang C, Raza SHA, Pan Y, Zhang K and Zan L (2022) Integration of RNA-seq and ATAC-seq identifies muscle-regulated hub genes in cattle. Front. Vet. Sci. 9:925590. doi: 10.3389/fvets.2022.925590
Received: 21 April 2022; Accepted: 19 July 2022;
Published: 11 August 2022.
Edited by:
Fazul Nabi, Lasbela University of Agriculture, Water and Marine Sciences, PakistanReviewed by:
XiuKai Cao, Yangzhou University, ChinaAli Raza Jahejo, Shanxi Agricultural University, China
Ayman Hassan Abd El-Aziz, Damanhour University, Egypt
Copyright © 2022 Wang, Li, Yang, Liang, Raza, Pan, Zhang and Zan. This is an open-access article distributed under the terms of the Creative Commons Attribution License (CC BY). The use, distribution or reproduction in other forums is permitted, provided the original author(s) and the copyright owner(s) are credited and that the original publication in this journal is cited, in accordance with accepted academic practice. No use, distribution or reproduction is permitted which does not comply with these terms.
*Correspondence: Linsen Zan, zanlinsen@163.com
†These authors have contributed equally to this work