- 1Northwest Institute of Plateau Biology, Chinese Academy of Sciences, Xining, China
- 2University of Chinese Academy of Sciences, Beijing, China
- 3State Key Laboratory of Plateau Ecology and Agriculture, Qinghai University, Xining, China
Ruminal microbiota and metabolites play crucial roles in animal health and productivity. Exploring the dynamic changes and interactions between microbial community composition and metabolites is important for understanding ruminal nutrition and metabolism. Tibetan sheep (Ovis aries) are an important livestock resource on the Qinghai-Tibetan Plateau (QTP), and the effects of various dietary protein levels on ruminal microbiota and metabolites are still unknown. The aim of this study was to investigate the response of ruminal microbiota and metabolites to different levels of dietary protein in Tibetan sheep. Three diets with different protein levels (low protein 10.1%, medium protein 12.1%, and high protein 14.1%) were fed to Tibetan sheep. 16S rRNA gene sequencing and gas chromatography coupled with time-of-flight mass spectrometry (GC-TOF-MS) were used to study the profile changes in each group of ruminal microbes and metabolites, as well as the potential interaction between them. The rumen microbiota in all groups was dominated by the phyla Bacteroidetes and Firmicutes regardless of the dietary protein level. At the genus level, Prevotella_1, Rikenellaceae_RC9_gut_group and Prevotellaceae_UCG-001 were dominant. Under the same forage-to-concentrate ratio condition, the difference in the dietary protein levels had no significant impact on the bacterial alpha diversity index and relative abundance of the major phyla and genera in Tibetan sheep. Rumen metabolomics analysis revealed that dietary protein levels altered the concentrations of ruminal amino acids, carbohydrates and organic acids, and significantly affected tryptophan metabolism (p < 0.05). Correlation analysis of the microbiota and metabolites revealed positive and negative regulatory mechanisms. Overall, this study provides detailed information on rumen microorganisms and ruminal metabolites under different levels of dietary protein, which could be helpful in subsequent research for regulating animal nutrition and metabolism through nutritional interventions.
Introduction
Tibetan sheep are the most economically important domestic animals on the Qinghai-Tibetan Plateau (QTP), providing native Tibetan herders with meat, wool and milk (1). On the QTP, Tibetan sheep adapt well to plateau environments and poor feeding conditions, and they mainly search for forage in the alpine meadow (2, 3). Nonetheless, the QTP environment is extremely harsh, with heavy snowfalls during the long cool-season from November to May, with average temperatures ranging from −5 to −15°C. Climate conditions and fluctuations directly affect forage yield and quality (particularly crude protein content), and herbage biomass and nutritional status are insufficient to meet the daily nutritional requirements of grazing animals (4–6). A previous study showed that Tibetan sheep suffered serious live-weight loss (−20.54%) under traditional pastoral herding, resulting in severe economic loss during the cold season (7). In addition to growth performance and economic benefits, cold season grazing also reduces growth hormone levels and damages the immune defense system of Tibetan sheep (8). Therefore, scientific management and rearing are particularly important in Tibetan sheep production.
The rumen is the primary organ system for nutrient digestion and absorption in ruminants, and it contains abundant microbiota and metabolites. Rumen microorganisms play an important role in the fermentation of plant fibers and polysaccharides (9, 10). Previous studies have shown that rumen microbial community structure and function are influenced by different factors, such as host breed, sex, diet and external environment (11–13). As one of the most important factors, dietary nutrition can change the relative abundance of dominant bacterial groups (e.g., Bacteroidetes, Firmicutes, Proteobacteria) and metabolic functions (e.g., carbohydrate, amino acid, and energy metabolism) (14–16). Metabolomics is a newly emerging field, following the application of genomics and transcriptomics based on detection techniques and includes nuclear magnetic resonance (NMR), gas chromatography-mass spectrometry (GC-MS), and liquid chromatography-mass spectrometry (LC-MS) (17). Metabolite profiles include a huge array of organic endogenous metabolites that play a vital role in nutrient regulation in animals. Previous studies have reported that ruminal lipids, amino acids and carbohydrates change significantly by changing the dietary forage-to-concentrate ratio (18, 19).
Dietary protein level is considered an essential factor that affects the growth, development and health conditions of animals (20, 21). Proteins fed to ruminants are degraded by microbes into peptide-bound amino acids and free amino acids for microbial protein synthesis (22). However, the effect of dietary protein levels on the ruminal microbiota and metabolites of Tibetan sheep and the interaction between ruminal microbiota and metabolites remain unclear. Recent rapid developments and applications in multi-omics technology have been devoted to gaining a better understanding of the ruminal ecosystem, especially the relationships among the microbiota, metabolites and host (23, 24).
In the current study, we hypothesize that dietary protein levels would influence rumen microorganisms and ruminal metabolites in Tibetan sheep. 16S rRNA sequencing and GC-TOF-MS were used to determine the effects of three different dietary protein levels on the profiles of the ruminal microbiota and metabolites in Tibetan sheep. Furthermore, the potential relationships between the ruminal microbiota and metabolites were explored.
Materials and Methods
Ethics Statement
The animal experiments in this study were approved by the Experimental Animal Use Ethics Committee of the Northwest Institute of Plateau Biology, Chinese Academy of Sciences (Approval No. NWIPB20160302).
Experimental Design and Sample Collection
A detailed description of the experimental design has been previously provided (25). The experiment was conducted at the Haibei Demonstration Zone of Plateau Modern Ecological Husbandry Science and Technology in Qinghai Province (China) (36°55′N, 100°57′E, altitude at 3,100 m). A total of eighteen 1-yr-old healthy castrated Tibetan sheep with similar initial body weight (BW: 31.71 ± 0.72 kg) were randomly assigned to three different dietary treatment groups, with each group containing 6 sheep. All those sheep were bred in the same demonstration zone and under the same feeding management practices before the experiment. The protein levels of the three different diets were low protein 10.1% (LP), medium protein 12.1% (MP) and high protein 14.1% (HP). Diets were designed according to the National Research Council guidelines (26) (Table 1). The sheep were fed with the mixed diet twice daily at 08:00 and 17:00. The experiment was lasted 105 days after 15 days of adaptation to the experimental diets and all sheep were provided with water ad libitum. At the end of the experiment, rumen fluid samples were collected before the morning feeding using an oral stomach tube and placed in frozen tubes to avoid contamination. The samples were immediately frozen in liquid nitrogen, and stored at −80°C for microbiome and metabolome analysis.
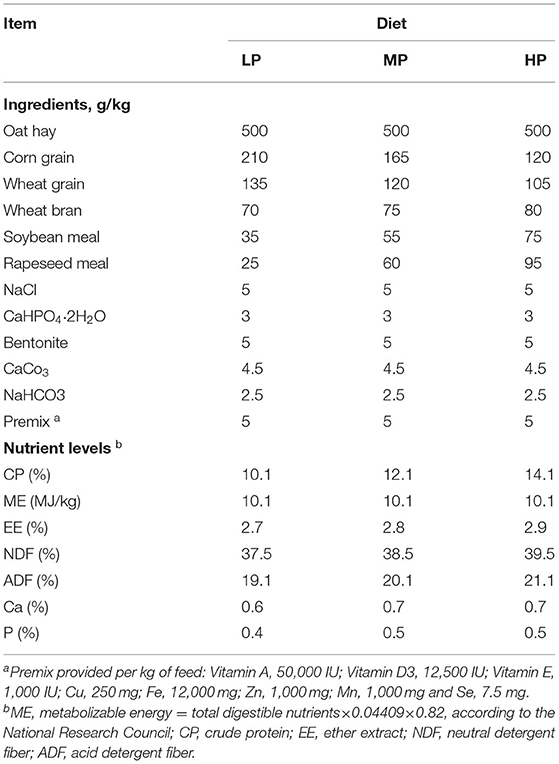
Table 1. Ingredients and nutrient levels of the experimental diets with three different protein levels (on a dry matter basis).
Microbiome Composition Analysis
Total genomic DNA was extracted from the rumen fluid samples using the bacterial genomic DNA extraction kit from TIANamp (TIANGEN, Beijing, China). The 16S rRNA gene targeting the V3-V4 region was amplified from the total genomic DNA and sequenced using the Illumina NovaSeq 6000 platform. After sequencing, the raw sequences were analyzed using USEARCH 10.0 and scripts written by Liu et al. (27). The quality of the paired-end Illumina reads was checked using FastQC v.0.11.5 (28) and processed using USEARCH. Unique reads were denoised into ASVs using unoise3 in USEARCH (29). A feature table was generated using VSEARCH (30). The SILVA v123 (31) database was used to classify the taxonomy of the representative sequences, and the plastids and non-bacteria were removed. Alpha diversity indices, including richness and the Shannon index, were calculated. For beta diversity, variations in microbial composition among the three different groups were investigated using constrained PCoA (CPCoA).
Metabolomics Data Analysis
Rumen fluid samples were centrifuged at 4 °C for 5 min at 10,000 rpm and transferred into a 1.5 ml tube, and pre-cold methanol with 10 μl internal standard 2-Chloro-L-phenylalanine was added. After centrifugation, 200 μl of supernatant was transferred to a fresh tube. Fifty microliters of each sample were removed and combined to prepare a quality control sample. After evaporation in a vacuum concentrator, 30 μl of methoxyamination hydrochloride was added and derivatized with 40 μl of BSTFA reagent at 70 °C for 1.5 h. All samples were then analyzed by GC-TOF-MS. The GC-TOF-MS analysis was performed using an Agilent 7890 GC-TOF-MS. The system used a DB-5MS capillary column (30 m ×250 μm ×0.25 μm). Chroma TOF (V 4.3x, LECO) and the LECO-Fiehn Rtx5 database were used for the raw data analysis, including peak extraction, baseline adjustment, deconvolution, alignment and integration. Finally, peaks detected in less than half of the quality control samples or RSD > 30% in the quality control samples were removed.
The resulting data were imported into software SIMCA 14.1 software (Umetrics, Umea, Sweden) for orthogonal projections to latent structures-discriminant analysis (OPLS-DA). Differential metabolites were identified by combining the VIP values obtained from the OPLS-DA analysis and t-test (VIP > 1.5 and p < 0.05). Differential metabolites were identified and validated using the Human Metabolome Database (HMDB; https://hmdb.ca/) and Kyoto Encyclopedia of Genes and Genomes (KEGG; https://www.kegg.jp/). The data analysis tool MetaboAnalyst 5.0 (https://www.metaboanalyst.ca/) was used to view the metabolic pathway distribution and enrichment of the differential metabolites.
Correlations Between Microbial Communities and Rumen Metabolites
Rumen metabolites with VIP > 1.5, p < 0.05, and the top 10 microbial genera were used for interactive analysis. Spearman's rank correlations and p-value were calculated using the GenesCloud tool, a website for microbial analysis (http://www.genescloud.cn).
Results
Sequencing and Diversity Estimates of Rumen Microbiomes
Totally 2,144,910 raw reads were obtained for the bacterial 16S rRNA genes from 18 rumen fluid samples using the sequencing procedure. After quality control, 2,046,323 high-quality reads were obtained (average of 85,263 reads per sample). A total of 3,921 ASVs were produced based on the results. The statistics of the bacterial alpha diversity indices (richness and Shannon index) for each sample were calculated, and the results are shown in Figures 1A,B. Richness and Shannon values were not significant among the three groups (p > 0.05). The result of beta diversity based on the CPCoA showed that the rumen microbiota of Tibetan sheep clustered three distinct parts, and these three groups were largely separated from each other with 15.1% of the variance (p = 0.0001) (Figure 1C).
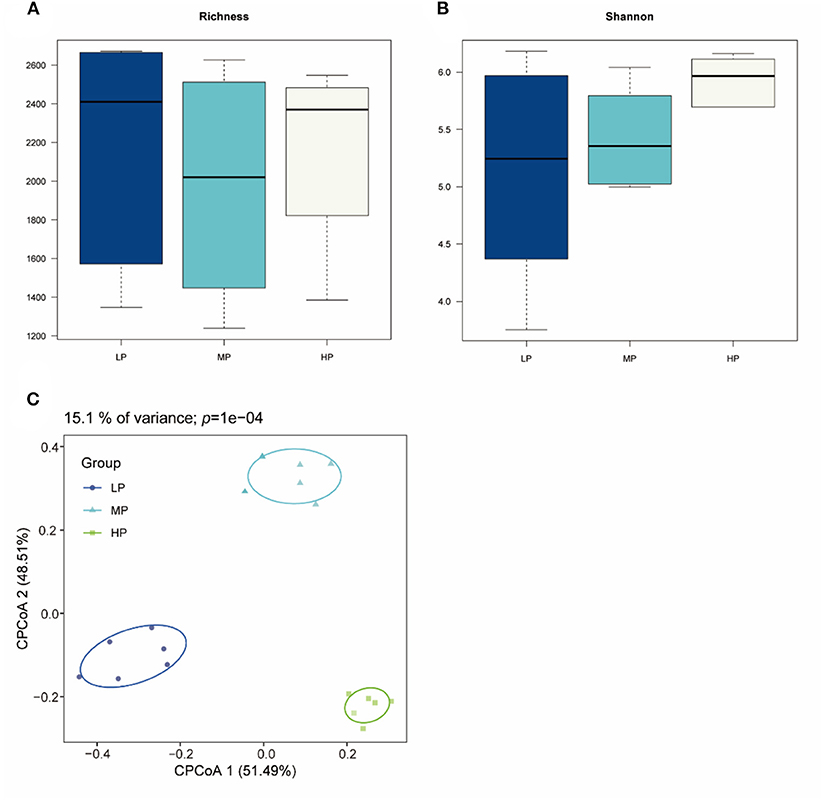
Figure 1. Bacterial diversity of rumen fluid samples among three different groups. (A) Richness index; (B) Shannon index; (C) CPCoA plot based on ASVs.
Bacterial Community Compositions
At the taxonomic level, 16 bacterial phyla, 26 classes, 37 orders, 56 families, and 163 genera were detected in the rumen microbiota of Tibetan sheep. At the phylum level (Figure 2A), Bacteroidetes (54.37–59.58%), Firmicutes (21.68–26.10%), Proteobacteria (8.81–18.46%), Actinobacteria (0.27–4.78%), Tenericutes (0.23–0.36%), Spirochaete (0.07–0.15%), Saccharibacteria (0.06–0.10%) and Candidate_division_SR1 (0.02–0.35%) were the dominant bacteria. Among these phyla, Bacteroidetes and Firmicutes had the highest relative abundances in all three groups. However, no significant between-group differences in relative abundance were detected at the phylum level. At the genus level (Figure 2B), Prevotella_1 (21.10–32.38%), Rikenellaceae_RC9_gut_group (4.94–7.52%), Prevotellaceae_UCG-001 (2.18–2.47%), Prevotellaceae_UCG-003 (1.28–2.61%) and Christensenellaceae_R-7_group (1.45–2.45%) were the dominant bacteria. In addition, the relative abundance of these dominant bacterial genera was also not significantly affected by dietary protein levels (p > 0.05).
Identification and Quantification of GC-TOF-MS Metabolites in the Rumen
In total, 411 valid peaks were identified in 18 rumen fluid samples. After rigorous quality control and identification, 189 metabolites, including organic acids and derivatives, organoheterocyclic compounds, organic oxygen compounds, benzenoids, organic oxygen compounds, lipids, lipid-like molecules and benzenoids, were obtained from the metabolomics library of the three groups, which shared the same metabolite categories.
For further analysis, OPLS-DA was conducted to characterize the differences in rumen metabolic profiles between the different groups. The parameters for the assessment of the OPLS-DA model in differentiating the three groups is represented by validation plots (Figure 3). The corresponding R2Y values of the OPLS-DA model for LP vs. HP, MP vs. HP and LP vs. MP were 0.954, 0.98 and 0.835, respectively. This indicates that this model can be used to identify differences between the groups. OPLS-DA results also showed that these groups had distinctly different metabolite compositions.
Rumen Metabolomic Profiles
Based on the statistical analysis results and the VIP values obtained from OPLS-DA, 17 metabolites (p < 0.05, and VIP > 1.5) were found to be significantly different in the comparisons of HP vs. LP, MP vs. LP, and HP vs. MP. Among these, three metabolites were classified into benzenoids (super class level; the same as below); four were classified into lipids and lipid-like molecules; three were classified into organic acids and derivatives; three were classified into organic oxygen compounds; three were classified into oganioheterocyclic compounds; and one was classified into homogeneous non-metal compounds.
With an increase in dietary protein level, 17 metabolites showed an increase (Figure 4; Supplementary Table 1). Compared with the LP group, three metabolites (beta-Alanine, Hydroxypropanedioic acid and 5-Hydroxyindoleacetic acid) in the HP group increased significantly (VIP > 1.5, p < 0.05). Compared with the MP group, six metabolites (D-Mannose, Allose, Phenylethylamine, Indan-1-ol, D-Maltose and Maltulose) in the HP group increased significantly (VIP > 1.5, p < 0.05). Compared with the LP group, eight metabolites (Pyrrole-2-carboxylic acid, Indoleacetic acid, 3-Hydroxypalmitic acid, 2,2-Dimethylsuccinic acid, Maleamate, 3-Hydroxynorvaline, Hydroxylamine and 4-Methylcatechol) in the MP group increased significantly (VIP > 1.5, p < 0.05).
Metabolic Pathways of Differential Metabolites
Differential metabolites in rumen fluid samples from the three groups were analyzed using MetaboAnalyst 5.0 software to reveal their association with metabolic pathways (Figure 5). According to KEGG pathway identification, seven pathways (tryptophan metabolism, phenylalanine metabolism, starch and sucrose metabolism, pantothenate and CoA biosynthesis, beta-alanine metabolism, propanoate metabolism and pyrimidine metabolism) were identified. Tryptophan metabolism was the most altered metabolic pathway among the three groups (p < 0.05).
Relationship Between the Ruminal Microbiome and Metabolome
Based on the results of Spearman correlation coefficients and p values, and clear positive and negative correlations were detected between the main ruminal microbiota and differential metabolites (Figure 6). For example, Rikenellaceae_RC9_gut_group was positively associated with 5-Hydroxyindoleacetic acid, D-Mannose, Pyrrole-2-carboxylic acid, Maleamate, 3-Hydroxynorvaline and Hydroxylamine (p < 0.05). Ruminococcus_2 was positively correlated with Allose and Maltulose levels (p < 0.05). Christensenellaceae_R-7_group was positively correlated with beta-Alanine, Hydroxypropanedioic acid and Indoleacetic acid (p < 0.05). Ruminococcaceae_UCG-014 was positively correlated with beta-Alanine and Hydroxypropanedioic acid levels (p < 0.05). Ruminococcaceae_NK4A214_group was positively correlated with Hydroxypropanedioic acid, D-Mannose and Phenylethylamine (p < 0.05) while Quinella was negatively associated with beta-Alanine levels (p < 0.05).
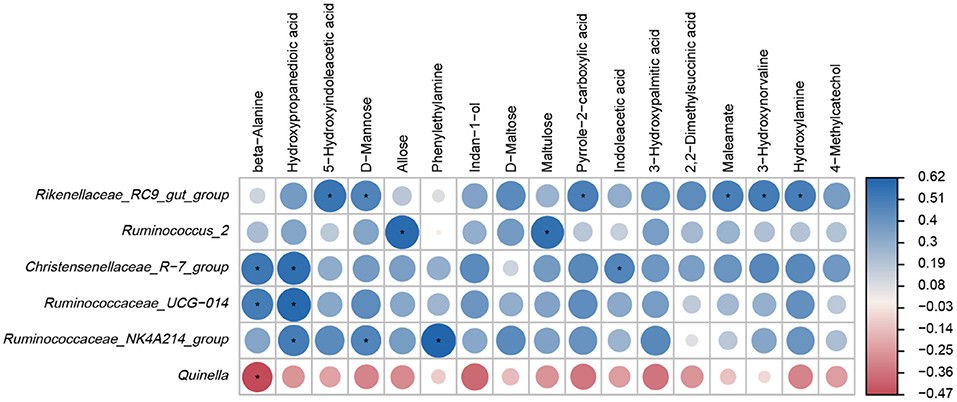
Figure 6. Heatmap of Spearman correlations between main genera and differential metabolites. *p < 0.05.
Discussion
Rumen bacterial communities and metabolites play important roles in the growth, development and organismal health of ruminants (32, 33). The objective of this study was to investigate the effects of different dietary protein levels with the same metabolizable energy level on ruminal microbiota and metabolites in Tibetan sheep using 16S rRNA sequencing and GC-TOF-MS and to detect the potential relationships between ruminal bacteria and metabolites.
In this study, the dietary protein levels did not have a significant influence on alpha diversity (based on the richness and Shannon index) or the relative abundance of the main bacterial phyla and genera. A previous study showed that under the same forage-to-concentrate ratio, the alteration of dietary energy level had no significant influence on the alpha diversity and bacterial community structure in Holstein heifers (34). This finding is consistent with research that shows that changing one nutrient content of the diet (e.g., protein or energy) under the same food ingredients was not sufficient to cause a strong fluctuation in the ruminal microbiota. Several studies (18, 35–37) showed that the dietary forage-to-concentrate ratio under the same food ingredients was the most critical environmental factors shaping rumen microbial structures and composition. In our study, Bacteroidetes, Firmicutes, and Proteobacteria were the predominant bacterial phyla in the rumen of Tibetan sheep, and their relative abundances did not show significant impact among the three groups. Bacteroidetes and Firmicutes play a critical role in microbial ecology and are involved in the decomposition of fibrous and non-fibrous diets (38, 39). The phylum Proteobacteria is the largest phylum of bacteria, including many pathogenic bacteria, such as Escherichia coli, Salmonella, Vibrio cholerae and Helicobacter pylori. Although the relative abundance of Proteobacteria is much lower compared to the Bacteroidetes and Firmicutes, it still plays an important role in rumen metabolism, such as biofilm formation and digestion of soluble carbohydrates (40). Besides, Bacteroidetes and Proteobacteria are the two major N-metabolizing microbial communities (41). Liu et al. (42) demonstrated that Tibetan sheep fed high-concentrate (45–60%) diets significantly increased the relative abundance of Bacteroidetes and reduced the Proteobacteria to adapt to a diet containing more non-fibrous carbohydrates and polysaccharides. At the genus level, the dominant genera (e.g., Prevotella_1, Rikenellaceae_RC9_gut_group, Prevotellaceae_UCG-001, Prevotellaceae_UCG-003 and Christensenellaceae_R-7_group) were also not influenced by dietary protein levels. Prevotella has been found predominant in the rumen of sheep and can enhance the capacity to utilize starch and non-cellulosic polysaccharides and promote the production of total volatile fatty acids (VFA) (43, 44). Moreover, Prevotella can ferment proteins and attain a N balance status in the gut (45). Rikenellaceae_RC9_gut_group, which is the main gene of Rikenellaceae, plays an important role in the fermentation of carbohydrates and crude protein (46, 47). Fan et al. (8) also revealed that Tibetan sheep could enhance forage degradation and fermentation by increasing the relative abundance of Bacteroidetes, Prevotella_1, and Prevotellaceae_UCG-003 during the summer season compared with the winter season. Christensenellaceae are belonged to Firmicutes, which widespread in the intestines and mucous membranes of humans and animals and are important for host health due to several enzymes (48). Overall, Bacteroidetes, Firmicutes and Prevotella were the most dominant bacterial taxa in the rumen of Tibetan sheep, corroborating the results of previous studies on goats (49), cows (50), beef cattle (51) and yaks (52), indicating that these bacteria play a major role in immunity, health, and the ecological function of the gastrointestinal tract in ruminants. In the present study, Tibetan sheep had a strong capacity to digest protein in the feed and optimize dietary amino acid utilization with the assistance of ruminal microorganisms.
Our study showed that the ruminal metabolite composition was significantly different among the three treatment groups based on OPLS-DA analysis, suggesting that the dietary nutrient content is likely to alter the rumen metabolomic profiles of Tibetan sheep. These results were comparable with previous study of Liu et al. (53), who found that feed type could significantly change the metabolites and functional pathway of yaks. In the present study, 17 metabolic biomarkers showed significant differences between the different groups, including benzenoids, lipids and lipid-like molecules, organic acids and derivatives, organic oxygen compounds, organoheterocyclic compounds and homogeneous non-metal compounds. Notably, all 17 metabolites showed a clear increasing trend with increasing dietary protein levels. Among these metabolites, beta-Alanine and Phenylethylamine are important amino acids for animals and enriched in sheep with the higher dietary protein. Beta-Alanine is a non-essential amino acid that is synthesized in the liver tissue and is presumed to be an intermediate required for the synthesis of acrylamide and acetonitrile (54, 55). Previous studies have confirmed that beta-Alanine can improve the digestibility of soluble starch and readily fermentable carbohydrates and induce the transition from VFAs to carbohydrates (53, 56). Phenylethylamine is an essential amino acid for animals and can synthesize neurotransmitters and hormones, and participates in glucose metabolism and fat metabolism (57). In addition to these amino acids, several carbohydrates, such as D-Mannose, Allose and D-Maltose, were also enriched in the higher dietary protein groups. The main function of these carbohydrates is to provide energy for animal growth and development (58). For example, D-Mannose is an important monosaccharide for protein glycosylation in mammals and thought to promote immunity and boost energy metabolism in organisms (59, 60). KEGG analysis revealed that tryptophan metabolism was the most altered metabolic pathway among the three groups. Tryptophan metabolism is a multi-pathway and complex process that occurs in the host and its intestinal symbiotic microbiota. Several metabolites generated by the tryptophan pathway have various effects on immune function, including tryptophan metabolism in animals (61). It was found that intestinal microorganisms such as bacteria, fungi and protozoa could contribute to the formation of key tryptophan metabolites, such as anabolic D-Tryptophan in the microbial community, small peptides synthesized by fungi, indoles and their derivatives (62). The same tryptophan metabolites produced by animal intestinal bacteria are indole derivatives, such as indoleacetic acid (IAA), indole sulfuric acid (ISA), indole-3-acetaldehyde (IAAId) and tryptamine (63). In addition, tryptophan metabolites of intestinal microbes could affect host physiological health by stimulating target gene expression, modulating the mucosal immune system, and targeting specific receptors. In our study, the content of indoleacetic acid showed higher concentration in the rumen of Tibetan sheep fed the higher dietary protein level. Based on the above results, we speculate that higher dietary protein may regulate nutrient absorption and growth performance through the composition and function of ruminal metabolites.
Metabolomics is used to study the small-molecule metabolites changes in animals produced by the external disturbance and can reflect the state of physical function more accurately. Compared to microorganisms, the metabolome can reflect the most intuitive physiological state of the animal. Therefore, the rumen metabolomic profiles were more sensitive to different dietary protein levels than bacterial community compositions in the present study. Additionally, an association was found between the structure of the rumen microbiota and metabolic profiles. These results were consistent with those in donkeys (64), yaks (65), Holstein heifers (18), mice (66) and humans (67), revealing a close relationship between microbiota, metabolites and organismal health. Our study also found significant positive correlations between the dominant bacterial groups and differential metabolites using Spearman correlation analysis. Our previous study demonstrated that higher dietary protein levels could improve growth performance, carcass performance and meat quality (25). Based on these findings, it is speculated that rumen bacterial groups may promote nutrient absorption capability by positively regulating the concentration of amino acids (e.g., beta-Alanine, Phenylethylamine), carbohydrates (e.g., D-Mannose, Allose, D-Maltose, Maltulose) and organic acids (e.g., Hydroxypropanedioic acid, Indoleacetic acid, and Maleamate), and promote the nutrient absorption and growth performance of Tibetan sheep.
Conclusions
In summary, we applied multi-omics analysis combined with microbiome and metabolomics analyses to investigate the effects of dietary protein levels on ruminal microbial communities and metabolites in Tibetan sheep. Under the same forage-to-concentrate ratio condition, the difference in dietary protein levels had no significant impact on rumen bacterial groups. Meanwhile, with increasing dietary protein levels, the concentrations of metabolites related to nutrient absorption significantly increased. In addition, the dominant microbiota was associated with different metabolites, indicating a close link between microbes and metabolites. Taking the above points into consideration, the higher protein levels (12.1 and 14.1%) were recommended as the appropriate dietary protein level in Tibetan sheep during the cold season. This study allowed us to gain a better understanding of ruminal microbial and metabolic functions and can lead to improvements in the protein level requirements within Tibetan sheep diets and nutritional regulation.
Data Availability Statement
The datasets presented in this study can be found in online repositories. The names of the repository/repositories and accession number(s) can be found below: https://www.ncbi.nlm.nih.gov/, PRJNA801776.
Ethics Statement
The animal study was reviewed and approved by Experimental Animal Use Ethics Committee of the Northwest Institute of Plateau Biology, Chinese Academy of Sciences.
Author Contributions
XW, SX, and TX: conception and experiment design. XW, TX, XZ, NZ, and YG: experiment conduction. XW, HL, and XZ: statistical analysis. SK, LH, and QZ: resources. XW: writing original draft preparation. All authors have read and agreed to the published version of the manuscript.
Funding
This work was funded by the Strategic Leading Science and Technology Program of the Chinese Academy of Sciences (XDA2005010406 and XDA23060603), Platform of Adaptive Management on Alpine Grassland-livestock System (2020-ZJ-T07), and Joint Research Project of Sanjiangyuan National Park (LHZX-2020-7).
Conflict of Interest
The authors declare that the research was conducted in the absence of any commercial or financial relationships that could be construed as a potential conflict of interest.
Publisher's Note
All claims expressed in this article are solely those of the authors and do not necessarily represent those of their affiliated organizations, or those of the publisher, the editors and the reviewers. Any product that may be evaluated in this article, or claim that may be made by its manufacturer, is not guaranteed or endorsed by the publisher.
Supplementary Material
The Supplementary Material for this article can be found online at: https://www.frontiersin.org/articles/10.3389/fvets.2022.922817/full#supplementary-material
References
1. Zhou JW, Guo XS, Degen AA, Zhang Y, Liu H, Mi JD, et al. Urea kinetics and nitrogen balance and requirements for maintenance in Tibetan sheep when fed oat hay. Small Ruminant Res. (2015) 129:60–8. doi: 10.1016/j.smallrumres.2015.05.009
2. Langda S, Zhang C, Zhang K, Gui B, Ji D, Deji C, et al. Diversity and composition of rumen bacteria, fungi, and protozoa in goats and sheep living in the same high-altitude pasture. Animals. (2020) 10:186. doi: 10.3390/ani10020186
3. Yang C, Gao P, Hou F, Yan T, Chang S, Chen X, et al. Relationship between chemical composition of native forage and nutrient digestibility by Tibetan sheep on the Qinghai-Tibetan Plateau. J Anim Sci. (2018) 96:1140–9. doi: 10.1093/jas/sky002
4. Long RJ, Apori SO, Castro FB, Ørskov ER. Feed value of native forages of the Tibetan Plateau of China. Anim Feed Sci Technol. (1999) 80:101–13. doi: 10.1016/S0377-8401(99)00057-7
5. Zhao X, Zhou X. Ecological basis of alpine meadow ecosystem management in Tibet: Haibei alpine meadow ecosystem research station. Ambio. (1999) 28:642–7.
6. Xue B, Zhao XQ, Zhang YS. Seasonal changes in weight and body composition of yak grazing on alpine-meadow grassland in the Qinghai-Tibetan Plateau of China. J Anim Sci. (2005) 83:1908–13. doi: 10.2527/2005.8381908x
7. Xu T, Xu S, Hu L, Zhao N, Liu Z, Ma L, et al. Effect of dietary types on feed intakes, growth performance and economic benefit in Tibetan sheep and yaks on the Qinghai-Tibet Plateau during cold season. PLoS ONE. (2017) 12:e0169187. doi: 10.1371/journal.pone.0169187
8. Fan Q, Cui X, Wang Z, Chang S, Wanapat M, Yan T, et al. Rumen microbiota of Tibetan sheep (Ovis aries) adaptation to extremely cold season on the Qinghai-Tibetan Plateau. Front Vet Sci. (2021) 8:554. doi: 10.3389/fvets.2021.673822
9. Vahidi MF, Gharechahi J, Behmanesh M, Ding X-Z, Han J-L, Salekdeh GH. Diversity of microbes colonizing forages of varying lignocellulose properties in the sheep rumen. PeerJ. (2021) 9:e10463. doi: 10.7717/peerj.10463
10. Yang C, Tsedan G, Liu Y, Hou F. Shrub coverage alters the rumen bacterial community of yaks (Bos grunniens) grazing in alpine meadows. J Anim Sci Technol. (2020) 62:504. doi: 10.5187/jast.2020.62.4.504
11. Ma L, Xu S, Liu H, Xu T, Hu L, Zhao N, et al. Yak rumen microbial diversity at different forage growth stages of an alpine meadow on the Qinghai-Tibet Plateau. PeerJ. (2019) 7:e7645. doi: 10.7717/peerj.7645
12. Zhang T, Mu Y, Zhang D, Lin X, Wang Z, Hou Q, et al. Determination of microbiological characteristics in the digestive tract of different ruminant species. Microbiologyopen. (2019) 8:e00769. doi: 10.1002/mbo3.769
13. Zhao S, Min L, Zheng N, Wang J. Effect of heat stress on bacterial composition and metabolism in the rumen of lactating dairy cows. Animals. (2019) 9:925. doi: 10.3390/ani9110925
14. Park T, Ma L, Ma Y, Zhou X, Bu D, Yu Z. Dietary energy sources and levels shift the multi-kingdom microbiota and functions in the rumen of lactating dairy cows. J Anim Sci Biotechnol. (2020) 11:1–16. doi: 10.1186/s40104-020-00461-2
15. Wang Q, Zeng Y, Zeng X, Wang X, Wang Y, Dai C, et al. Effects of dietary energy levels on rumen fermentation, gastrointestinal tract histology and bacterial community diversity in fattening male Hu lambs. Front Microbiol. (2021) 12:695445. doi: 10.3389/fmicb.2021.695445
16. Zhao ZW, Ma ZY, Wang HC, Zhang CF. Effects of rumen-protected methionine and lysine supplementation on milk yields and components, rumen fermentation, and the rumen microbiome in lactating yaks (Bos grunniens). Anim Feed Sci Technol. (2021) 277:114972. doi: 10.1016/j.anifeedsci.2021.114972
17. Foroutan A, Fitzsimmons C, Mandal R, Piri-Moghadam H, Zheng J, Guo A, et al. The bovine metabolome. Metabolites. (2020) 10:233. doi: 10.3390/metabo10060233
18. Zhang J, Shi H, Wang Y, Li S, Cao Z, Ji S, et al. Effect of dietary forage to concentrate ratios on dynamic profile changes and interactions of ruminal microbiota and metabolites in Holstein heifers. Front Microbiol. (2017) 8:2206. doi: 10.3389/fmicb.2017.02206
19. Zhang R, Zhu W, Jiang L, Mao S. Comparative metabolome analysis of ruminal changes in Holstein dairy cows fed low-or high-concentrate diets. Metabolomics. (2017) 13:1–15. doi: 10.1007/s11306-017-1204-0
20. Tamminga S. Nutrition management of dairy cows as a contribution to pollution control. J Dairy Sci. (1992) 75:345–57. doi: 10.3168/jds.S0022-0302(92)77770-4
21. Schroeder GF, Titgemeyer EC, Awawdeh MS, Smith JS, Gnad DP. Effects of energy level on methionine utilization by growing steers. J Anim Sci. (2006) 84:1497–504. doi: 10.2527/2006.8461497x
22. Xie Y, Xu Q, Wu Y, Huang X, Liu J. Duodenum has the greatest potential to absorb soluble non-ammonia nitrogen in the nonmesenteric gastrointestinal tissues of dairy cows. J Zhejiang Univ Sci B. (2015) 16:503–10. doi: 10.1631/jzus.B1400299
23. Gharechahi J, Salekdeh GH. A metagenomic analysis of the camel rumen's microbiome identifies the major microbes responsible for lignocellulose degradation and fermentation. Biotechnol Biofuels. (2018) 11:1–19. doi: 10.1186/s13068-018-1214-9
24. Xue M-Y, Sun H-Z, Wu X-H, Liu J-X, Guan LL. Multi-omics reveals that the rumen microbiome and its metabolome together with the host metabolome contribute to individualized dairy cow performance. Microbiome. (2020) 8:1–19. doi: 10.1186/s40168-020-00819-8
25. Wang X, Xu T, Zhang X, Geng Y, Kang S, Xu S. Effects of dietary protein levels on growth performance, carcass traits, serum metabolites, and meat composition of Tibetan sheep during the cold season on the Qinghai-Tibetan Plateau. Animals. (2020) 10:801. doi: 10.3390/ani10050801
26. Council NR. Nutrient Requirements of Small Ruminants: Sheep, Goats, Cervids, and New World Camelids. Washingto, DC: The National Academies Press (2007).
27. Liu Y, Qin Y, Chen T, Lu M, Qian X, Guo X, et al. A practical guide to amplicon and metagenomic analysis of microbiome data. Protein Cell. (2021) 12:315. doi: 10.1007/s13238-020-00724-8
28. Andrews S. FastQC: A Quality Control tool for High Throughput Sequence Data. Babraham: Babraham Bioinformatics (2013).
29. Edgar RC, Flyvbjerg H. Error filtering, pair assembly and error correction for next-generation sequencing reads. Bioinformatics. (2015) 31:3476–82. doi: 10.1093/bioinformatics/btv401
30. Rognes T, Flouri T, Nichols B, Quince C, Mahé F. VSEARCH a versatile open source tool for metagenomics. PeerJ. (2016) 4:e2584. doi: 10.7717/peerj.2584
31. Quast C, Pruesse E, Yilmaz P, Gerken J, Schweer T, Yarza P, et al. The SILVA ribosomal RNA gene database project: improved data processing and web-based tools. Nucleic Acids Res. (2012) 41:D590–D6. doi: 10.1093/nar/gks1219
32. Xue BC, Zhang JX, Wang ZS, Wang LZ, Peng QH Da LC, et al. Metabolism response of grazing yak to dietary concentrate supplementation in warm season. Animal. (2021) 15:100175. doi: 10.1016/j.animal.2021.100175
33. Goad DW, Goad CL, Nagaraja TG. Ruminal microbial and fermentative changes associated with experimentally induced subacute acidosis in steers. J Anim Sci. (1998) 76:234–41. doi: 10.2527/1998.761234x
34. Bi Y, Zeng S, Zhang R, Diao Q, Tu Y. Effects of dietary energy levels on rumen bacterial community composition in Holstein heifers under the same forage to concentrate ratio condition. BMC Microbiol. (2018) 18:1–11. doi: 10.1186/s12866-018-1213-9
35. Wang H, Pan X, Wang C, Wang M, Yu L. Effects of different dietary concentrate to forage ratio and thiamine supplementation on the rumen fermentation and ruminal bacterial community in dairy cows. Anim Prod Sci. (2014) 55:189–93. doi: 10.1071/AN14523
36. Han X, Li B, Wang X, Chen Y, Yang Y. Effect of dietary concentrate to forage ratios on ruminal bacterial and anaerobic fungal populations of cashmere goats. Anaerobe. (2019) 59:118–25. doi: 10.1016/j.anaerobe.2019.06.010
37. Chen H, Wang C, Huasai S, Chen A. Effects of dietary forage to concentrate ratio on nutrient digestibility, ruminal fermentation and rumen bacterial composition in Angus cows. Sci Rep. (2021) 11:1–11. doi: 10.1038/s41598-021-96580-5
38. Shanks OC, Kelty CA, Archibeque S, Jenkins M, Newton RJ, McLellan SL, et al. Community structures of fecal bacteria in cattle from different animal feeding operations. Appl Environ Microbiol. (2011) 77:2992–3001. doi: 10.1128/AEM.02988-10
39. Zhang X-L, Xu T-W, Wang X-G, Geng Y-Y, Liu H-J, Hu L-Y, et al. The effect of transitioning between feeding methods on the gut microbiota dynamics of yaks on the Qinghai-Tibet Plateau. Animals. (2020) 10:1641. doi: 10.3390/ani10091641
40. Shin N-R, Whon TW, Bae J-W. Proteobacteria: microbial signature of dysbiosis in gut microbiota. Trends Biotechnol. (2015) 33:496–503. doi: 10.1016/j.tibtech.2015.06.011
41. Xie B, Lv Z, Hu C, Yang X, Li X. Nitrogen removal through different pathways in an aged refuse bioreactor treating mature landfill leachate. Appl Microbiol Biotechnol. (2013) 97:9225–34. doi: 10.1007/s00253-012-4623-x
42. Liu H, Xu T, Xu S, Ma L, Han X, Wang X, et al. Effect of dietary concentrate to forage ratio on growth performance, rumen fermentation and bacterial diversity of Tibetan sheep under barn feeding on the Qinghai-Tibetan plateau. PeerJ. (2019) 7:e7462. doi: 10.7717/peerj.7462
43. Bekele AZ, Koike S, Kobayashi Y. Genetic diversity and diet specificity of ruminal Prevotella revealed by 16S rRNA gene-based analysis. FEMS Microbiol Lett. (2010) 305:49–57. doi: 10.1111/j.1574-6968.2010.01911.x
44. Lv X, Chai J, Diao Q, Huang W, Zhuang Y, Zhang N. The signature microbiota drive rumen function shifts in goat kids introduced to solid diet regimes. Microorganisms. (2019) 7:516. doi: 10.3390/microorganisms7110516
45. Gibson SA, McFarlan C, Hay S, MacFarlane GT. Significance of microflora in proteolysis in the colon. Appl Environ Microbiol. (1989) 55:679–83. doi: 10.1128/aem.55.3.679-683.1989
46. Tian Y, Zhang H, Zheng L, Li S, Hao H, Yin M, et al. Process analysis of anaerobic fermentation exposure to metal mixtures. Int J Environ Res Public Health. (2019) 16:2458. doi: 10.3390/ijerph16142458
47. Liu T, Ahn H, Sun W, McGuinness LR, Kerkhof LJ, H4ggblom MM. Identification of a Ruminococcaceae species as the methyl tert-butyl ether (MTBE) degrading bacterium in a methanogenic consortium. Environ Sci Technol. (2016) 50:1455–64. doi: 10.1021/acs.est.5b04731
48. Waters JL, Ley RE. The human gut bacteria Christensenellaceae are widespread, heritable, and associated with health. BMC Biol. (2019) 17:1–11. doi: 10.1186/s12915-019-0699-4
49. Lei Y, Zhang K, Guo M, Li G, Li C, Li B, et al. Exploring the spatial-temporal microbiota of compound stomachs in a pre-weaned goat model. Front Microbiol. (2018) 9:1846. doi: 10.3389/fmicb.2018.01846
50. Shabat SKB, Sasson G, Doron-Faigenboim A, Durman T, Yaacoby S, Berg Miller ME, et al. Specific microbiome-dependent mechanisms underlie the energy harvest efficiency of ruminants. ISME J. (2016) 10:2958–72. doi: 10.1038/ismej.2016.62
51. Chen F, Cheng G, Xu Y, Wang Y, Xia Q, Hu S. Rumen microbiota distribution analyzed by high-throughput sequencing after oral doxycycline administration in beef cattle. Front Vet Sci. (2020) 7:251. doi: 10.3389/fvets.2020.00251
52. Xue D, Chen H, Luo X, Guan J, He Y, Zhao X. Microbial diversity in the rumen, reticulum, omasum, and abomasum of yak on a rapid fattening regime in an agro-pastoral transition zone. J Microbiol. (2018) 56:734–43. doi: 10.1007/s12275-018-8133-0
53. Liu C, Wu H, Liu S, Chai S, Meng Q, Zhou Z. Dynamic alterations in yak rumen bacteria community and metabolome characteristics in response to feed type. Front Microbiol. (2019) 10:1116. doi: 10.3389/fmicb.2019.01116
54. Hoffman JR, Emerson NS, Stout JR. β-alanine supplementation. Curr Sports Med Rep. (2012) 11:189–95. doi: 10.1249/JSR.0b013e3182604983
55. Matthews MM, Traut TW. Regulation of N-carbamoyl-beta-alanine amidohydrolase, the terminal enzyme in pyrimidine catabolism, by ligand-induced change in polymerization. J Biol Chem. (1987) 262:7232–7. doi: 10.1016/S0021-9258(18)48228-2
56. Saleem F, Ametaj BN, Bouatra S, Mandal R, Zebeli Q, Dunn SM, et al. A metabolomics approach to uncover the effects of grain diets on rumen health in dairy cows. J Dairy Sci. (2012) 95:6606–23. doi: 10.3168/jds.2012-5403
57. Li H, Yu Q, Li T, Shao L, Su M, Zhou H, et al. Rumen microbiome and metabolome of Tibetan Sheep (Ovis aries) reflect animal age and nutritional requirement. Front Vet Sci. (2020) 7:609. doi: 10.3389/fvets.2020.00609
58. Jones SA, Jorgensen M, Chowdhury FZ, Rodgers R, Hartline J, Leatham MP, et al. Glycogen and maltose utilization by Escherichia coli O157: H7 in the mouse intestine. Infect Immun. (2008) 76:2531–40. doi: 10.1128/IAI.00096-08
59. Zhang L, Wang Y, Liu D, Luo L, Wang Y, Ye C. Identification and characterization of ALS genes involved in D-Allose metabolism in lineage II strain of Listeria monocytogenes. Front Microbiol. (2018) 9:621. doi: 10.3389/fmicb.2018.00621
60. Wen X, Hu Y, Zhang X, Wei X, Wang T, Yin S. Integrated application of multi-omics provides insights into cold stress responses in pufferfish Takifugu fasciatus. BMC Genomics. (2019) 20:1–15. doi: 10.1186/s12864-019-5915-7
61. Alcazar O, Hernandez LF, Tschiggfrie A, Muehlbauer MJ, Bain JR, Buchwald P, et al. Feasibility of localized metabolomics in the study of pancreatic islets and diabetes. Metabolites. (2019) 9:207. doi: 10.3390/metabo9100207
62. Kepert I, Fonseca J, Müller C, Milger K, Hochwind K, Kostric M, et al. D-tryptophan from probiotic bacteria influences the gut microbiome and allergic airway disease. Journal of Allergy and Clinical Immunology. (2017) 139:1525–35. doi: 10.1016/j.jaci.2016.09.003
63. Zhang J, Zhu S, Ma N, Johnston LJ, Wu C, Ma X. Metabolites of microbiota response to tryptophan and intestinal mucosal immunity: A therapeutic target to control intestinal inflammation. Med Res Rev. (2021) 41:1061–88. doi: 10.1002/med.21752
64. Zhang C, Zhang C, Wang Y, Du M, Zhang G, Lee Y. Dietary energy level impacts the performance of donkeys by manipulating the gut microbiome and metabolome. Front Vet Sci. (2021) 8:694357. doi: 10.3389/fvets.2021.694357
65. Zhang X, Xu T, Wang X, Geng Y, Zhao N, Hu L, et al. Effect of dietary protein levels on dynamic changes and interactions of ruminal microbiota and metabolites in yaks on the Qinghai-Tibetan Plateau. Front Microbiol. (2021) 12:684340. doi: 10.3389/fmicb.2021.684340
66. Lu K, Abo RP, Schlieper KA, Graffam ME, Levine S, Wishnok JS, et al. Arsenic exposure perturbs the gut microbiome and its metabolic profile in mice: an integrated metagenomics and metabolomics analysis. Environ Health Perspect. (2014) 122:284–91. doi: 10.1289/ehp.1307429
Keywords: Tibetan sheep, rumen, microbiota, metabolomics, dietary protein
Citation: Wang X, Xu T, Zhang X, Zhao N, Hu L, Liu H, Zhang Q, Geng Y, Kang S and Xu S (2022) The Response of Ruminal Microbiota and Metabolites to Different Dietary Protein Levels in Tibetan Sheep on the Qinghai-Tibetan Plateau. Front. Vet. Sci. 9:922817. doi: 10.3389/fvets.2022.922817
Received: 18 April 2022; Accepted: 31 May 2022;
Published: 29 June 2022.
Edited by:
Rita Payan Carreira, University of Evora, PortugalReviewed by:
Manuela Oliveira, Universidade de Lisboa, PortugalNeeta Agarwal, Indian Veterinary Research Institute (IVRI), India
Copyright © 2022 Wang, Xu, Zhang, Zhao, Hu, Liu, Zhang, Geng, Kang and Xu. This is an open-access article distributed under the terms of the Creative Commons Attribution License (CC BY). The use, distribution or reproduction in other forums is permitted, provided the original author(s) and the copyright owner(s) are credited and that the original publication in this journal is cited, in accordance with accepted academic practice. No use, distribution or reproduction is permitted which does not comply with these terms.
*Correspondence: Shixiao Xu, sxxu@nwipb.cas.cn