- 1College of Animal Science and Technology, Northeast Agricultural University, Harbin, China
- 2Institute of Animal Husbandry, Heilongjiang Academy of Agricultural Sciences, Harbin, China
Goose is a seasonal breeding animal. Its reproduction is regulated by hypothalamus-pituitary-gonad axis and also affected by environmental factors such as light and location. Zi-goose is characterized with long egg-laying period and high egg-laying potential and belongs to the long-day type of seasonal breeding. In this study, the regulation mechanism of different lighting on reproductive performance of Zi-goose by using metabonomics analysis technology. In addition, 1,481 differential metabolites were screened out totally. 583 differential metabolites were identification in hypothalamus of Zi-goose. 196 differential metabolites were identification in pituitary of Zi-goose. 692 differential metabolites were identification in ovary of Zi-goose. Under red light condition for 12 h, expression of 433 differential metabolites were down-regulated and expression of 150 differential metabolites were up regulated in hypothalamus of Zi-goose, expression of 125 differential metabolites were down-regulated and expression of 71 differential metabolites were up-regulated in pituitary of Zi-goose, expression of 355 differential metabolites were down-regulated and expression of 337 differential metabolites were up-regulated in ovary of Zi-goose. 33 differential metabolites were closely associated with 1,264 transcripts and 400 homologous genes of related enzymes in hypothalamus of Zi-goose. 15 differential metabolites were closely associated with 163 transcripts and 47 homologous genes of related enzymes in pituitary of Zi-goose. 55 differential metabolites were closely associated with 1,255 transcripts and 360 homologous genes of related enzymes in ovary of Zi-goose. It was confirmed that four metabolic pathways were closely related to light regulation of reproductive performance of Zi-goose, namely GnRH signaling pathway, prolactin signaling pathway, thyroid hormone synthesis and ovarian steroidogenesis. Typical differential metabolites of arachidonic acid, glucose-6-phosphate, progesterone, glutathione, oxidized glutathione, testosterone, deoxyepiandrosterone and their related protein genes would play an important role in light regulation of reproductive performance of Zi-goose.
Introduction
Metabonomics is an essential component of systems biology (1). Its research idea is to follow the example of genomics and proteomics, using high-throughput and high-sensitivity modern analysis technology to quantitatively analyze all metabolites in the organism as well as to find the corresponding relationship between metabolites and physiological and pathological changes (2, 3). Metabolomics is the study of all metabolites in a sample at a certain time by LC-MS/GC-MS. Metabonomics magnifies the slight variations of gene and protein expression reflecting the physiological and pathological state of the body system (4). Through metabonomics analysis, the different metabolites between the experimental group and the control group could be further clarified, which provides a linkage for revealing the material and energy metabolism activities between the experimental and the control groups (5, 6).
In the previous studies, it has been indicated that 12 h red light was beneficial to improve the reproductive performance of Zi-geese, and the differentially expressed candidate genes and possible regulatory pathways of Zi-geese under different light conditions were analyzed by transcriptome sequencing technology (7, 8). To further reveal the mechanism of the 12 h red light improving the reproductive performance of geese, the samples of the hypothalamus, ovaries, and pituitaries from the Zi-geese during the peak laying season were studied through this research and those samples processed through the metabolomics, transcriptomics, and metabolomics combined analysis. This study was aimed to find out the differential metabolites in response to light regulation, and to clarify the regulatory genes of the differential metabolites and their differences in the regulation mode of reproductive performance for the geese, as well as to further analyze the regulation mechanism of different light conditions on reproductive performance of the Zi-geese.
Methods and Material
The Experimental Materials
Fifty layings Zi-geese were purchased from the Yuanfang geese industry in Harbin.
Animals Experiment
The fifty geese were randomly separated into two groups with 25 geese in each group. Those geese were respectively treated with the white incandescent lamp and red LED lamp set 12 h long. The experimental groups were shown in Table 1. Conventional feeding management, mixed group feeding of male and female geese, as well as the experimental diet, were shown in Table 2. At the end of the experiment, 3 geese in each treatment were randomly slaughtered. Thalamus, pituitary, and ovary tissues were sampled and put into an enzyme-free aseptic cryopreservation tube and quickly frozen in liquid nitrogen. After that, they were taken back to the laboratory and stored at −80°C for transcriptome and metabonomic analysis.
Preparation of the Detection Samples
In total, 60 mg of tissue samples were added to 600 uL of methanol, shaken for 30 s, and centrifuged at 4°C and 12,000 r/min for 10 min to obtain the sample, which was used for the UPLC-Q/TOF-MS detection.
Analyse of the Components by UPLC-Q/TOF-MS
The analysis of the drug-containing plasma samples was performed in an LC-30A UPLC (Shimadzu, Kyoto, Japan) coupled hybrid quadrupole time-of-flight tandem mass spectrometer (LC/MS-Triple TOFTM 5600+, AB Sciex, Concord, ON, Canada) equipped with an electrospray ionization (ESI) interface (9, 10). The chromatographic conditions were were consistent with those described in our previously published article.
Construction of the Component-Target-Disease Network
Cytoscape V3.7.2 is an open-source software used to visualize complicated networks and integrate different types of attribute data (11). The aforementioned data were imported into Cytoscape V3.7.2, which was used to construct an active component-target-disease interaction network. The nodes indicate components or targets, and the edges indicate their relationships. The topological parameters, including “Degree”e “Closeness Centrality” and “Betweenness Centrality”e which can be used to evaluate the importance of components and targets, were calculated by Network Analyzer. The core components were screened as ligands for molecular docking based on the degree values. The degree value of a molecule represents the number of connections between the molecule and targets in the core architecture of the network.
Construction of the Protein–Protein Interaction (PPI) Network
To analyze the interactions between the CR component targets and primary dysmenorrhea-related targets, the overlapping targets were imported to the STRING database (https://string-db.org/, ver. 11.0). The organism was programmed to be “Anas”n and the confidence level was set as “highest confidence (0.900)”0 The PPI network was obtained from the targets most strongly associated with the overlapping targets. Cytoscape V3.7.2 was used to construct and visualize the PPI network. The top 10 correlated targets were identified by calculating the correlation degree of the target proteins in the PPI network.
GO and KEGG Enrichment Analyses
OmicShare (http://www.omicshare.com/tools) was used to visualize the results of Gene Ontology (GO) functional enrichment analysis and Kyoto Encyclopedia of Genes and Genomes (KEGG) pathway enrichment analysis (12). GO analysis included biological process (BP), molecular function (MF), and cellular component (CC) ontologies. The statistical significance threshold was set at P<0.05.
Results
The LC-MS Analysis of the Organ Metabolites
Ultra-high performance liquid chromatography-tandem time of flight mass spectrometry (UPLC-Q-TOF/MS) of Waters was used for LC-MS analysis of samples, and quality control samples were used to test the performance of the method (13). According to the ion flow diagram of the base peak of the quality control sample in the positive and negative ion mode (Figures 1A,B), it can be known that the chromatographic peak was uniform, the number was large, the peak shape was good, there was no oversaturation tailing and other adverse phenomena, and the methodology and sample quality were qualified. PCA examination of the test results (Figure 1C) showed that all the points of the quality control sample (QC) were closely clustered, indicating that the whole experimental process has been well repeated and no abnormal data.
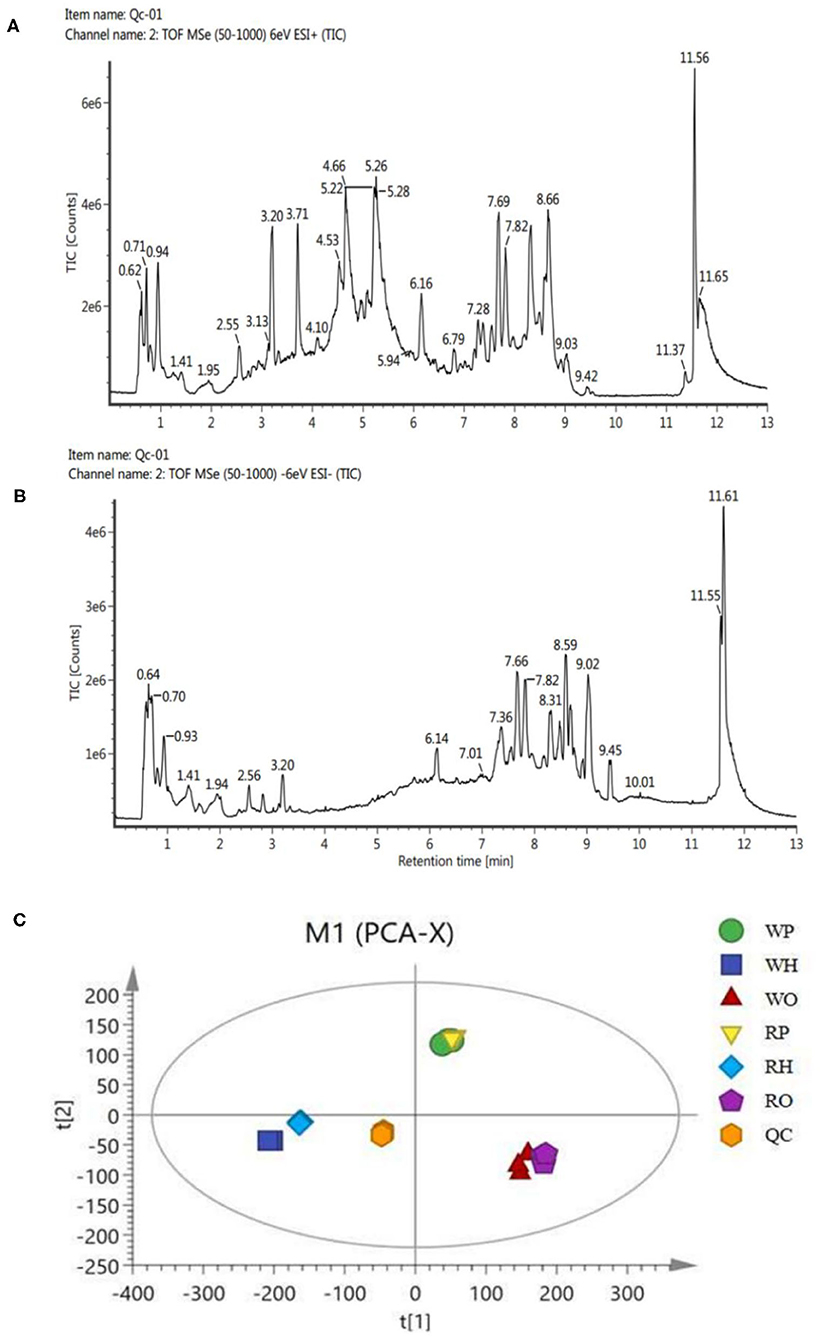
Figure 1. (A) BPI(+) ion flow chart of control samples. (B) BPI(-) ion flow chart of control samples. (C) PCA plots for all samples.
Identification of Metabolites and Screening of Differential Metabolites
As shown in Figure 2, 4,105 metabolites were identified based on the standard of Progenesis QI. On this basis, univariate statistical analysis was used to screen differential metabolites according to the difference multiple (FC > 2 or FC < 0.5) and P-value (P-value < 0.05, t-test). Through the experiment, 1481 differential metabolites were screened, among which 583 were screened from the hypothalamus of the candidate geese and 384 were unique; 196 differential metabolites and 117 unique differential metabolites were screened from the pituitaries of that; 692 differential metabolites and 513 unique differential metabolites were screened from the ovary of the Zi-geese. Additionally, 44 different metabolites were identification in the hypothalamus and pituitaries, 144 different metabolites were identification in the hypothalamus and ovary, 24 different metabolites were identification in pituitary and ovary, and 11 differential metabolites in the hypothalamus, pituitaries, and ovary.
Cluster Analysis of Differential Metabolites
According to the Cluster thermogram of differential metabolites, the accumulation of 125 differential metabolites in the pituitaries of the Zi-geese decreased under the 12 h red light, while the accumulation of 71 differential metabolites increased under the red light within 12 h (Figure 3A).The accumulation of 433 differential metabolites in the hypothalamus of the geese decreased under the light, while 150 differential metabolites increased under the same condition(Figure 3B). The accumulation of 355 differential metabolites in the ovary of the geese decreased under the 12 h red light, and 337 differential metabolites increased under the red light (Figure 3C). The accumulation of these differential metabolites in different organs will be the main basis for the improvement of reproductive performance of the Zi-geese under red light for 12 h.
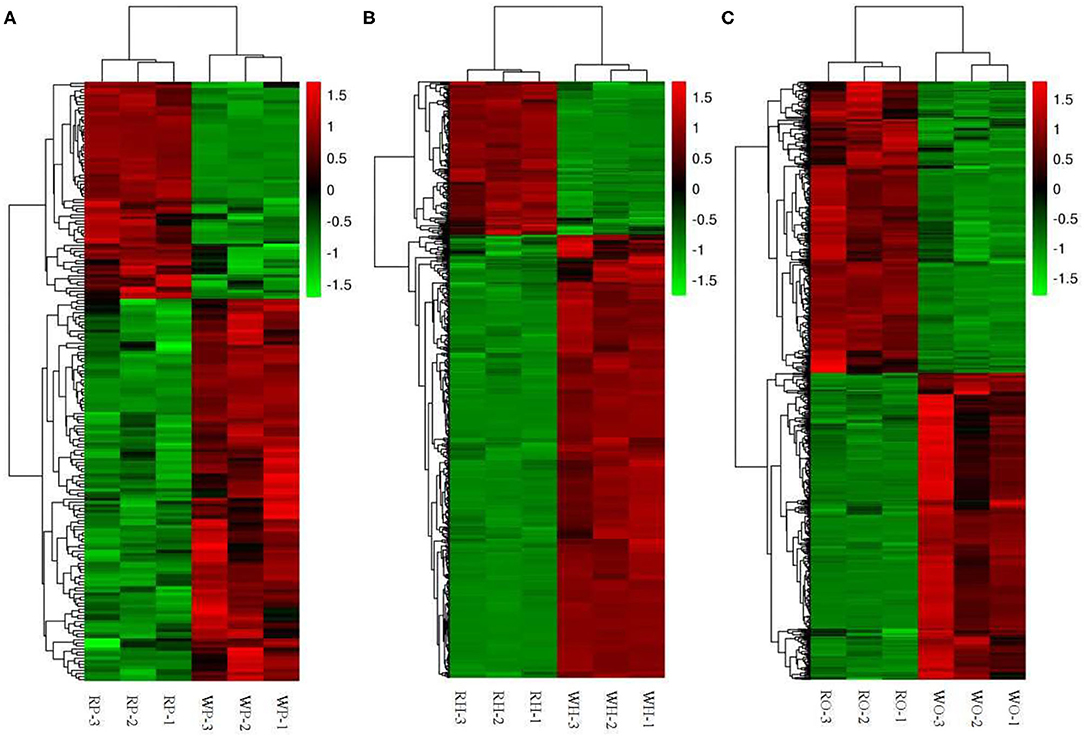
Figure 3. Cluster thermogram of differential metabolites. (A) pituitaries, (B) hypothalamus, (C) ovary.
Combined Analysis of Metabolites and Transcripts
The results of the combined analysis showed that 33 differential metabolites were closely related to 1,264 transcripts and 400 homologous genes of related enzymes in the hypothalamus of the geese (Table 2); In the Zi-geese' pituitaries, 15 differential metabolites were closely related to 163 transcripts and 47 homologous genes of related enzymes (Table 3); There were 55 differential metabolites in Zi-geese' ovary, which were closely related to 1,255 transcripts and 360 homologous genes of related enzymes (Table 4).
According to the results of differential expression between metabolites and corresponding transcripts (log2 (FC) > 1), 15 pairs of metabolites were further screened in the hypothalamus of Zi-geese. The co-expression of transcripts significantly responded to the changes of light conditions (Table 5), and the marker metabolites were nicotinamide adenine dinucleotide, pyruvate, L-glutamic acid, ascorbic acid, 6-phosphate glucose ester, arachidonic acid, prostaglandin E2, glutamic acid γ-Glutamate kinase and hydroquinone. Three pairs of metabolites were further screened out from the pituitary gland of Zi-geese. The co-expression of transcripts significantly responded to the changes of light conditions, and the marker metabolites involved were vanillic acid and adipic acid (Table 6). 186 pairs of metabolites were further screened out in the ovary of Zi-geese. The co-expression of transcripts significantly responded to the changes of light conditions, involving marker metabolites including glycine, L-aspartic acid, glutathione, L-serine, ascorbic acid, L-methionine, phosphoenolpyruvate, L-cysteine, oxidized glutathione, 5,10-methylenetetrahydrofolate, nicotinamide, glycolic acid, gluconolactone, sarcosine, nicotinic acid, hypoxanthine Inosine, pyridoxine, thiamine, xanthine, carnosine, guanosine, L-cystine, hydroquinone, testosterone, betaine aldehyde, guanidino acetic acid, gentian acid, cortisol, deoxycytidine, thiamine monohydrate, hydroxyproline, dehydroepiandrosterone, ossifying diol, xanthine nucleoside, pyroglutamine, dihydrozeatin, phenyl glyoxylic acid, deoxyinosine, 2,5-dihydroxybenzaldehyde, canfel Adipic acid α- Linolenic acid, hydroquinone, 6-( α- D-glucose-1-d-inositol) and 2-oxo-10-methylthiodecanoic acid (Table 7).
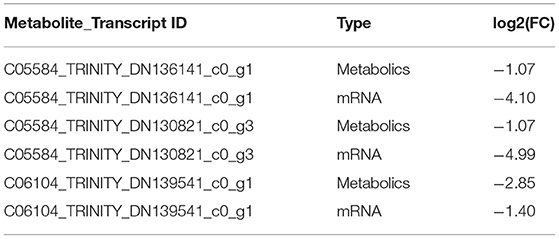
Table 6. Results of differential co-expression of hypothalamic metabolites and related transcripts in Zi-geese.

Table 7. Results of differential co-expression of pituitary metabolites and related transcripts in Zi-geese.
According to the results of transcripts and metabolites pathway analysis, combined with transcripts and metabolites differential co-expression, there were four metabolic pathways closely relating to light regulation of reproductive performance of the geese has been selected, namely GnRH signaling pathway, prolactin signaling pathway, thyroxine synthesis pathway and ovarian steroid synthesis pathway (Figure 4). In the GnRH signaling pathway, arachidonic acid was a typical differential metabolite, and 36 genes and 112 proteins (transcripts) played corresponding roles in the pathway. The typical differential metabolites involved in the prolactin signaling pathway were glucose-6-phosphate and progesterone, and 15 genes and 67 proteins (transcripts) play corresponding roles in the pathway. In the thyroid hormone synthesis pathway, the typical differential metabolites involved in the hypothalamus were glucose-6-phosphate, while the typical differential metabolites involved in the ovary were glutathione and oxidized glutathione. Meanwhile, 20 genes and 77 proteins (transcripts) played the corresponding roles in the pathway. Arachidonic acid and progesterone were the typical differential metabolites in the hypothalamus, and 24 genes and 77 proteins (transcripts) played corresponding roles in the pathway; The typical differential metabolites involved in the ovary were testosterone and deoxyepiandrosterone, and 24 genes and 69 proteins (transcripts) played corresponding roles in the pathway.
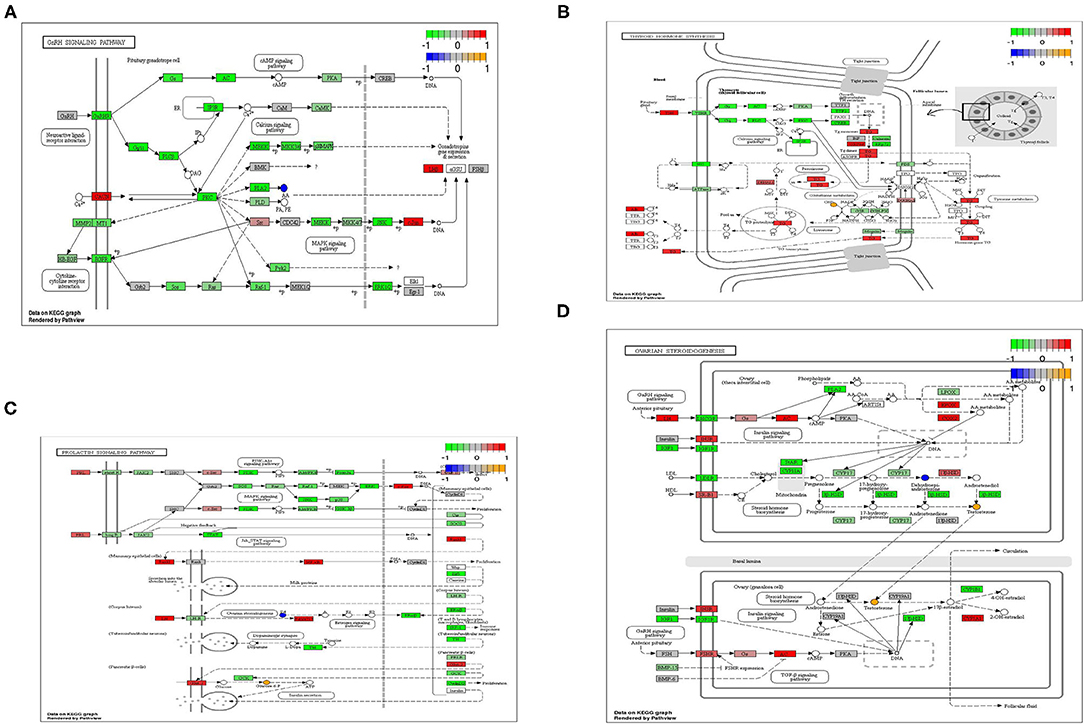
Figure 4. (A) GnRH signaling pathway of differential metabolites and differential transcripts. (B) PRL signaling pathway of differential metabolites and differential transcripts. (C) Thyroid hormone synthesis pathway of differential metabolites and differential transcripts. (D) Ovarian steroidogenesis pathway of differential metabolites and differential transcripts.
Discussion
Currently, the widely applied metabonomics research methods include Nuclear Magnetic Resonance (NMR), GC-TOF/MS, and UPLCQ-TOF/MS (14, 15). In comparison, nuclear magnetic resonance (NMR) technology is widely used for its advantages of non-destructive, absolute quantitative, and outstanding repeatability (16). However, meanwhile, its disadvantages are very prominent, such as low detection sensitivity, unable to detect extremely trace and low concentration substances; Although GC-TOF/MS technology can identify more complex metabolites with low cost, the pretreatment is complicated which increases the possibility of errors (17); In recent years, UPLC-Q-TOF/MS technology has been widely used in biomedicine, food science, agricultural science and other research fields by its properties of “separation capability”, “reliability”, and “sensitivity” (18). Through this experiment, UPLC-Q-TOF/MS technology was used to study the metabonomics of the hypothalamus, pituitary, and ovary of Zi-geese under the different light conditions. 18 samples were analyzed, and a quality control sample (QC) was inserted into every 6 samples for quality control to investigate the repeatability of the whole analysis procedure. According to the ion flow diagram of the base peak under the positive and negative ion mode of the quality control sample, the chromatographic peaks were uniform, large in number, good in shape, without oversaturation, tailing, and other adverse phenomena. At the same time, the PCA examination of the test results also showed that all the points of the quality control sample (QC) were closely clustered, which indicated that the whole experimental process has outstanding repeatability and no abnormal data. The results showed that the quality of the test sample was qualified, and the analysis technology was practical and effective. Through the transcriptomic analysis, 1,481 differential metabolites were screened out, among which 583 were screened out from the hypothalamus of Zi-geese and 384 were unique; 196 differential metabolites and 117 unique differential metabolites were screened from the pituitaries of Zi-geese; 692 differential metabolites and 513 unique differential metabolites were screened from the ovary of the geese. The results showed that the changes in light conditions could significantly affect the metabolic processes related to the reproductive performance of Zi-geese.
To further understand the inner mechanism of light regulation on reproductive performance of Zi-geese, the transcriptomics and metabonomics combined analysis technology has been used to analyze the differentially expressed genes and differentially accumulated metabolite information of the test samples for gene-gene, gene-metabolite, metabolite-metabolite “co-expression” analysis, to identify the key metabolic pathway and figure out the main regulatory factors and structural genes. The regulation mechanism was analyzed and verified by physiological, biochemical, genetic, and molecular biology methods. Through transcriptomic and metabonomic analysis, 204 pairs of metabolites were found. The co-expression of transcripts identified four metabolic pathways closely related to the reproductive performance of Zi-geese, which pointed out the direction and goal for understanding the internal mechanism of light regulation of reproductive performance of Zi-geese.
Since the change of light conditions can affect the reproductive performance of poultry and the expression of related genes, the change of light conditions would inevitably lead to the change of metabolites related to reproductive performance. To further reveal the possible mechanism of red light for 12 h improving reproductive performance of Zi-geese, the metabonomics of hypothalamus, pituitary, and ovary samples of Zi-geese were studied. The results showed that 583 differential metabolites were found in the hypothalamus of the geese, and 384 were unique; 196 differential metabolites and 117 unique differential metabolites were screened from the pituitaries of the geese; 692 differential metabolites and 513 unique differential metabolites were screened from the ovaries of Zi Goose. This confirmed that there were significant differences in material metabolism between red light and white light at the peak laying period. Further analysis showed that 433 differentially expressed metabolites were down-regulated and 150 differentially expressed metabolites were uP-regulated in the hypothalamus of Zi-geese under red light for 12 h; 125 differential metabolites were down-regulated and 71 differential metabolites were up-regulated; 355 differentially expressed metabolites were down-regulated and 337 differentially expressed metabolites were up-regulated. The amount of these different metabolites might be the main substances that affect the reproductive performance of Zi-geese, and it is also the main object that needs to be focused on in the future. Co-expression of differentially expressed genes and metabolites was confirmed by transcriptome and metabolomic analysis, which provided the guarantee for mining core metabolic pathways and typical metabolites. The results showed that there were 15, 3, and 186 pairs of metabolites in the hypothalamus, pituitaries, and ovaries of Zi-geese. Four metabolic pathways were closely related to the light regulation of reproductive performance in the geese, including the GnRH signaling pathway, prolactin signaling pathway, thyroxine synthesis pathway, and ovarian steroid synthesis pathway. The differential metabolites involved in these metabolic pathways are arachidonic acid, glucose-6-phosphate, progesterone, glutathione, oxidized glutathione, testosterone, and deoxyepiandrosterone, which would play an essential role in the light regulation of the reproductive performance of Zi geese.
Conclusion
This study was found out the differential metabolites in response to light regulation, and to clarify the regulatory genes of the differential metabolites and their differences in the regulation mode of reproductive performance for the geese. In this study,1,481 differential metabolites were screened, among which 583 differential metabolites were screened from the hypothalamus of seeded goose, and 384 differential metabolites were endemic to the hypothalamus of seeded goose. A total of 196 differential metabolites were screened out from the pituitary gland of the goose, and 117 unique differential metabolites were found. 692 differential metabolites were screened from seed goose egg nests, and 513 differential metabolites were endemic. The accumulation of 433 differential metabolites in the hypothalamus of seed geese decreased under 12 h red light, while the accumulation of 150 differential metabolites increased under 12 h red light. The accumulation of 125 differential metabolites in pituitary gland decreased under 12 h red light condition, while the accumulation of 71 differential metabolites increased under 12 h red light condition. The accumulation of 355 differential metabolites in the nest of seed goose eggs decreased under 12 h red light, while the accumulation of 337 differential metabolites increased under 12 h red light. The results of joint analysis showed that 33 differential metabolites were closely related to the homologous genes of 1,264 transcripts and 400 related enzymes in the hypothalamus of Seed Geese. There were 15 differential metabolites closely related to 163 transcripts and 47 homologous genes of related enzymes in pituitary gland. There were 55 differential metabolites associated with 1,255 transcripts and 360 homologous genes of related enzymes in the nest of seed goose egg. In the hypothalamus, pituitary and ovary of seed geese, 15, 3 and 186 metabolite transcripts were screened out in response to the change of light conditions. Four metabolic pathways were determined to be closely related to light regulation of reproductive performance of seed geese, namely GnRH signaling pathway, prolactin signaling pathway, thyroxine synthesis pathway and ovarian steroid synthesis pathway. Canonical differential metabolites such as arachidonic acid, glucose 6-phosphate, progesterone, glutathione, glutathione oxidase, testosterone and deoxyepandrosterone, as well as their related genes and protein genes, will play an important role in light regulation of reproductive performance of seed geese.
Data Availability Statement
The original contributions presented in the study are included in the article/supplementary material, further inquiries can be directed to the corresponding author/s.
Author Contributions
LM: research concept, methodology, data extraction, analysis, and draft writing. ZX: resource searching, verification, formal analysis, supervision, and manuscript reviewing and editing. LG: resources, methodology, project administration, supervision, and manuscript reviewing and editing. ZG: resource searching, manuscript reviewing and editing, and methodology. All authors contributed to the article and approved the submitted version.
Funding
The research was supported by the research business expenses of the Scientific Research Institutes of Heilongjiang Province (No. CZKYF2021B003) and National Modern Waterfowl Industry Technical System Special Project (No. CARS-42-24).
Conflict of Interest
The authors declare that the research was conducted in the absence of any commercial or financial relationships that could be construed as a potential conflict of interest.
Publisher's Note
All claims expressed in this article are solely those of the authors and do not necessarily represent those of their affiliated organizations, or those of the publisher, the editors and the reviewers. Any product that may be evaluated in this article, or claim that may be made by its manufacturer, is not guaranteed or endorsed by the publisher.
References
1. Ma L, Wang X, Chai J. Application and research progress of metabonomics in the diagnosis and treatment of burn injury. Chin J Injury Repair and Wound Healing (Chinese). (2017) 12:212–5.
2. Ghaderi A, Mojtahedi Z. Proteomics studies in oncology towards personalized medicine in public health: Opportunities and challenges for OMICS research in Iran. Curr Pharmacogen Pers Med. (2012) 10:265–70. doi: 10.2174/187569212803901747
3. Guo X, Hao Y, Kamilijiang M, Hasimu A, Yuan J, Wu G, et al. Potential predictive plasma biomarkers for cervical cancer by 2D-DIGE proteomics and ingenuity pathway analysis. Tumour Biol. (2015) 36:11–20. doi: 10.1007/s13277-014-2772-5
4. Akdeniz M, Yener I, Ertas A, Firat M, Resitoglu B, Hasimi N, et al. Biological and chemical comparison of natural and cultivated samples of satureja macrantha. Rec Nat Prod. (2021) 15:568–84. doi: 10.25135/rnp.237.21.02.1957
5. Lu X, Zhao X, Bai C, Zhao C, Lu G, Xu G. LC-MS-based metabonomics analysis. J chromatogr B. (2008) 866:64–76. doi: 10.1016/j.jchromb.2007.10.022
6. Griffin JL. Metabonomics: NMR spectroscopy and pattern recognition analysis of body fluids and tissues for characterisation of xenobiotic toxicity and disease diagnosis. Curr Opin Chem Biol. (2003) 7:648–54. doi: 10.1016/j.cbpa.2003.08.008
7. Kang B, Jiang D, Liu B, Yang H. Quantitative studies on expression level of FTH and eight ESTs mRNA in the ovaries of Zi Geese. Chin J Anim Husbandry (Chinese). (2011) 47:16–9.
8. Chang S, Zhuang Z, Lin M, Cheng C, Lin T, Jea Y, et al. Effects of monochromatic light sources on sex hormone levels in serum and on semen quality of ganders. Anim Reprod Sc. (2016) 167:96–102. doi: 10.1016/j.anireprosci.2016.02.012
9. Yu C, Xu T, Zhang X, Dong M, Niu Y. Differential mechanism of toxicity in normal and blood-stasis mice following Rhizoma Curcumae exposure based on “YOU-GU-WU-YUN” theory. Acta Pharm Sin (Chinese). (2019) 54:329–34.
10. Xu Y, Zhao R, Hu Q. Effect of Curcumae Rhizoma on the pharmacokinetics of methotrexate in normal and blood stasis rats. J Guangdong Pharm Univ (Chinese). (2016) 32:403–5.
11. Baumbach J, Apeltsin L. Linking Cytoscape and the corynebacterial reference database CoryneRegNet. BMC Genomics. (2008) 9:184. doi: 10.1186/1471-2164-9-184
12. Jiang X, Lu H, Xu Z, Zhang Y, Zhao Q. Network pharmacology-based research uncovers cold resistance and thermogenesis mechanism of Cinnamomum cassia. Fitoterapia. (2020) 149:104824. doi: 10.1016/j.fitote.2020.104824
13. Singh J, Jayaprakasha GK, Patil BS. Rapid ultra-high-performance liquid chromatography/quadrupole time-of-flight tandem mass spectrometry and selected reaction monitoring strategy for the identification and quantification of minor spinacetin derivatives in spinach. Rapid Commu Mass Spectrom. (2017) 31:1803–12. doi: 10.1002/rcm.7967
14. Powers R, Siegel MM. 5-Applications of nuclear magnetic resonance and mass spectrometry to anticncer drug discovery. In: Adjei AA, Buolamwini JK. The strategies for discovery and clinical testing—novel anticancer agents. Cambridge: Academic Press (2006). p. 107–90. doi: 10.1016/B978-012088561-9/50006-5
15. Lalwani S, Venditto VJ, Chouai A, Rivera GE, Shaunak S, Simanek EE. Electrophoretic behavior of anionic triazine and PAMAM dendrimers: methods for improving resolution and assessing purity using capillary electrophoresis. Macromolecules. (2009) 42:3152–61. doi: 10.1021/ma802250c
16. Li P, Chen A, Fu Y, Han W. Study on determining Nuclear Magnetic Resonance (NMR) T_2 cutoff combined with the oil displacing water experiments. Prog Geophys (Chinese). (2019). 34:1050–4.
17. Libardoni M, Hasselbrink E, Waite JH, Sacks R. At-column heating and a resistively heated, liquid-cooled thermal modulator for a low-resource bench-top GC × GC. J Sep Sci. (2015) 29: 1001–8. doi: 10.1002/jssc.200500298
18. Huang Q, Zhang F, Liu S, Jiang Y, Ouyang D. Systematic investigation of the pharmacological mechanism for renal protection by the leaves of Eucommia ulmoides Oliver using UPLC-Q-TOF/MS combined with network pharmacology analysis. Biomed Pharmacother. (2021) 140:111735. doi: 10.1016/j.biopha.2021.111735
Keywords: Zi-goose, lighting, reproduction performance, metabolome, pathogenesis
Citation: Manyu L, Xiuhua Z, Guojun L and Guixue Z (2022) Impacts of Colored Light-Emitting Diode Illumination on the Reproductive Performance and Bioactive Constituents and the Molecular Mechanism of Hypothalamus Gland in Zi-geese. Front. Vet. Sci. 9:874679. doi: 10.3389/fvets.2022.874679
Received: 12 February 2022; Accepted: 02 March 2022;
Published: 11 April 2022.
Edited by:
Kun Li, Nanjing Agricultural University, ChinaReviewed by:
Muhammad Akhtar, Huazhong Agricultural University, ChinaHuanmin Yang, Heilongjiang Bayi Agricultural University, China
Copyright © 2022 Manyu, Xiuhua, Guojun and Guixue. This is an open-access article distributed under the terms of the Creative Commons Attribution License (CC BY). The use, distribution or reproduction in other forums is permitted, provided the original author(s) and the copyright owner(s) are credited and that the original publication in this journal is cited, in accordance with accepted academic practice. No use, distribution or reproduction is permitted which does not comply with these terms.
*Correspondence: Zhang Guixue, Z3h6aGFuZ0BuZWF1LmVkdS5jbg==