- 1Laboratorio de Diagnóstico Genético Veterinario, Departamento de Genética, Universidad de Córdoba, Córdoba, Spain
- 2Cersyra de Valdepeñas, Instituto Regional de Investigación y Desarrollo Agroalimentario y Forestal Castilla La Mancha, Tomelloso, Spain
- 3Departamento de Agronomía, Escuela Técnica Superior de Ingeniería Agronómica, Universidad de Sevilla, Sevilla, Spain
- 4IGEVET (UNLP-CONICET LA PLATA), Facultad de Ciencias Veterinarias, Universidad Nacional de La Plata, La Plata, Argentina
- 5Asociación Nacional de Criadores de Caballos de Pura Raza Española, Sevilla, Spain
- 6Departamento de Producción Animal, Facultad de Ciencias Veterinarias, Universidad Nacional de La Plata, Buenos Aires, Argentina
- 7Consejo Nacional de Investigaciones Científicas y Técnicas (CONICET LA PLATA), La Plata, Argentina
The loss of genetic variability in livestock populations bred under strict selection processes is a growing concern, as it may lead to increased inbreeding values and lower fertility, as a consequence of the “inbreeding depression” effect. This is particularly important in horses, where inbreeding levels tend to rise as individuals become more and more closely related. In this study, we evaluated the effect of increased inbreeding levels on mare fertility by combining an SNP-based genomic approach using runs of homozygosity and the estimation of genetic breeding values for reproductive traits in a large population of Pura Raza Española mares. Our results showed a negative correlation between whole-genome homozygosity and fertility estimated breeding values (EBVs) at the genome level (ρ = −0.144). However, the analysis at chromosome level revealed a wide variability, with some chromosomes showing higher correlations than others. Interestingly, the correlation was stronger (−0.241) when we repeated the analysis in a reduced dataset including the 10% most and least fertile individuals, where the latter showed an increase in average inbreeding values (FROH) of around 30%. We also found 41 genomic regions (ROHi, runs of homozygosity islands) where homozygosity increased 100-fold, 13 of which were significantly associated with fertility after cross-validation. These regions encompassed 17 candidate genes previously related to oocyte and embryo development in several species. Overall, we demonstrated the relationship between increased homozygosis at the genomic level and fertility in mares. Our findings may help to deal with the occurrence of inbreeding depression, as well as further our understanding of the mechanisms underlying fertility in mares.
Introduction
The increase in average inbreeding rates has become a serious concern in wild and livestock populations over the past two decades (1, 2). This phenomenon leads to a decrease in phenotypic values in fitness and fertility traits (3) known as inbreeding depression. At the molecular level, inbreeding depression is triggered by differences in the homozygosity load in regions associated with the genetic architecture of the traits. For this reason, two individuals with the same inbreeding value (estimated from pedigree and/or molecular methodologies) may show increased homozygosity in different regions of the genome, thus affecting its phenotype to a different extent (4).
In horses, increased inbreeding is of particular concern. In closed studbooks, such as Pura Raza Española (PRE) (5) and Thoroughbreds (6), the ban on using non-registered individuals as breeders tends to drastically reduce the effective population sizes and increases the number of closely-related matings which are the main cause of this phenomenon. However, breeds with open studbooks, such as Polo ponies, have also been affected, probably by increased selection intensity in mares, driven by the use of large-scale embryo transfer programs and cloning (7, 8).
Reproductive traits are extremely sensitive to problems derived from high levels of inbreeding (9). This can be even more problematic in horses due to their moderate reproductive ability, probably due to matings being mostly chosen for a combination of sports performance, morphological traits and pedigree lineages, without taking into account fertility as a selection criterion (10). In addition, fertility in mares is difficult to evaluate on a population basis, since large, reliable phenotypic datasets are needed to analyze quantitative traits with low heritabilities, and these are extremely scarce (11). However, some phenotypic traits which can be estimated directly from pedigree records have recently been validated in large-scale studies assessing mare fertility (10, 12), providing an interesting method of obtaining highly accurate reproductive estimated breeding values (EBVs). Among these, a mare's reproductive efficiency (Re), estimated as the percent deviation from the optimal number of foals produced at the end of her reproductive life, has recently been proposed as a reliable alternative in terms of heritability and accuracy in the PRE breed (13), enabling us to determine estimated breeding values (EBV's) in large datasets of mares.
Nowadays, horse breeding is entering the genomic era at full gallop. The number of individuals genotyped has increased exponentially over recent years, and it is now common to find studies including genotypes of hundreds of individuals (14–17). For this reason, the approach to determining the potential causes of inbreeding depression is shifting from the analysis of pedigree records to the use of genomic estimations based on runs of homozygosity (ROH) (18). This Methodology was originally developed in humans (19) and is currently the state-of-the-art technique for analyzing the effects of inbreeding on livestock populations (20). In addition, ROH analysis allows us to associate increased homozygosity in a specific genomic region with a phenotypic effect in large populations (21). Using this approach, Nani and Peñagaricano (22) have recently determined the existence of specific genomic regions where increased homozygosity negatively affected fertility in Holstein bulls. Likewise, Metzger et al. (23) used a similar methodology to evaluate the effect of inbreeding in reproductive traits in a small population of horses. However, to our knowledge, this approach has not yet been used to evaluate the relationship between homozygosity and reproductive traits in large populations of mares.
In this study, we used an ROH-based methodology to analyze the relationship between homozygosity and fertility in a large cohort of PRE mares. In addition, we performed a complete genome scan to determine the existence, topological position, and putative function of genomic regions where increased homozygosity could negatively affect fertility.
Materials and Methods
Reproductive Phenotypes
The initial reproductive dataset included 344,707 foaling records from 78,986 breeding mares belonging to 8,133 studs included in the PRE studbook. Records were obtained from the breed's creation until 2020, and only those of mares born after 1970 were kept, when the breed's official parentage control was established. Mares whose main activity was not foal production (at least 10 foals per year) were excluded, as were mares mainly devoted to leisure or sports activities (first foaling after 7 years old, with an interval between first and second foaling, and last and penultimate foaling over the age of 5).
Individual horse fertility was determined by assessing the reproductive efficiency (Re), which was defined as the percentage relationship between the current and optimal parity number during a mare's entire reproductive life, or the last known age of mares which were still reproductively active. This reproductive trait was recently validated by our group as an indirect estimator of the mare fertility in a large population of PRE horses (13).
Genetic Evaluation and ReEBV Deregression
Measurements of ReEBV were estimated using a restricted maximum likelihood (REML) animal model as follows:
where yi is the vector of the dependent variable (Re), μ is the overall mean, b is a vector of fixed effects, including stud, size of the herd in which the mare was born and year of birth; age and F, are the age and inbreeding of the mare respectively, included as lineal covariates, u is the vector of random effects due to the additive animal genetic effect, and e is the vector of the residual error, while X and Z are incidence matrices that relate the fixed and random effects with the dependent variable.
The expected variances of the model are:
The pedigree data of mares and all known relatives (n = 87,227) included in the additive genetic relationship matrix (A) (24) averaged 5.65 and 9.46 complete and equivalent generations available, respectively, with a maximum of 16. All the data was provided by the Asociación Nacional de Criadores de Caballos de Pura Raza Española.
All the calculations were performed using the RENUMF90, PreGSF90, and AIREMLF90 modules from the BLUPF90 software family (25). As a final step, ReEBV were deregressed to pseudophenotypes (RedEBV), following the methodology proposed by Garrick et al. (26) using the DEPROOFSF90 module from the BLUPF90 software.
Molecular Analysis
The second step was to select 862 living individuals from 373 PRE herds for single nucleotide polymorphism (SNP) genotyping using diverse criteria, including sample availability, low average relatedness among individuals, and reliable RedEBV estimations (with minimum 85% accuracy).
SNP Genotyping
Blood from all the individuals selected for molecular analysis was collected by jugular venipuncture using sterile EDTA tubes. DNA was obtained using the Canvax blood DNA extraction kit (Canvax Biotech, Spain) in 200 μl of whole blood according to the manufacturer's protocol. Genotyping was performed using the HD Axiom™ Equine SNP Genotyping Array (Thermofisher, Madrid, Spain), following the manufacturer's recommendations, in a Genetitan™ platform (National Genotyping Center (CeGen), Santiago de Compostela, Spain). The array included variant calls for 670,776 SNPs located uniformly across the entire genome (27). The genotypes were called using the Axiom Analysis Suite 5.0 software (Thermofisher, Spain) following the “best genotyping practices” workflow with default parameters (dish quality control [DQC] ≥ 0.82; call rate[CR] ≥ 97). Only SNP markers showing a high-quality genotyping rate (SNP CR > 95%) and quality (Fisher's Linear Discriminant (FLD) > 3.6) were kept, according to the manufacturer's recommendations (28). We did not estimate minor allele frequency (MAF) or perform linkage disequilibrium (LD) filtering, following the latest ROH estimation guidelines (29). The final genomic dataset included 540,294 SNPs per individual, located in 32 chromosomes.
Individual Inbreeding Coefficients Based on ROH Estimation
Whole-genome homozygosity characterization was performed by analyzing the ROH load in the R statistical environment V4.1 (30). We first determined the number, position and length of ROHs per animal using the slidingRuns procedure in the DetectRUNS Package (31), with the following parameters: windowSize = 50, minSNP = 100, threshold = 0.05, maxGap = 100,000, minLengthBps = 1,000,000, maxOppWindow = 1, maxMissWindow = 1 and SNPinRuns = TRUE. These values were selected to minimize genotyping errors and to avoid detecting ROH identity-by-descent segments, following Meyermans et al. (29). Finally, the molecular inbreeding (FROH) (percentage of the genome covered by ROH) per individual was estimated at genome and chromosome levels, following McQuillan et al. (19).
Relationship Between Whole-Genome Homozygosity and Fertility
To test the relationship between whole-genome homozygosity and Re, we first estimated the non-linear correlations between FROH (whole and per chromosome) and RedEBV in the 862 individuals genotyped. Next, we selected a reduced dataset (RD) which included individuals showing the top (High-Fert) and bottom (Low-Fert) 10% Re pseudophenotypes (n = 172), to increase the power of the association test. In both cases, the estimations were performed using the nlcor package (32) from R. Finally, we compared FROH values (at the genome level and per chromosome) between High-Fert and Low-Fert individuals to find differences in homozygosity associated with variations in fertility traits.
Detection of Genomic Regions Significantly Associated With Fertility
The genomic regions of the RD in which the incidence of ROH increased significantly (ROH islands, ROHi) were determined using a permutation test (1,000,000 iterations per marker) as described by Goszczynski et al. (4). Only regions larger than 100,000 bp which showed a 100-fold increase [-log10(p-value) > 2] compared with a random occurrence probability were tagged as ROHi and further analyzed.
Validation of Significant ROHi in the Whole Population
The ROHi identified in the RD were validated in the whole dataset using the method described by Nani and Peñagaricano (22). Briefly, the association between each ROH region and the Re values was determined using a t-test; ROHs with a t-value ≥ 2 were confirmed as significantly associated with mare fertility.
Analysis of Significant Regions and Candidate Genes
The regions showing a significant association were transformed into GRanges objects and intersected with the most recent version of the horse genome [EquCab 3.0, (33)], using the HelloRanges package in R (34), retrieving all the genes located within the candidate regions. Thereafter, the functionality of each gene was annotated using DAVID (35) and PANTHER (36) bioinformatic resources. Finally, we performed a comprehensive literature review using public scientific databases such as PubMed and Scopus to outline the possible association between candidate genes and fertility.
Results
Genetic Characterization of Reproductive Traits
ReEBV heritability (h2) was 0.25 ± 0.0031, revealing a substantial genetic effect. In addition, the standard deviation was nearly 80 times lower, which demonstrates their reliability. In addition, the ReEBV′s of the individuals genotyped showed a normal distribution (Shapiro-Wilk test, P > 0.05), while displaying a wide range of variability (−15.16 to 25.52; Figure 1). Finally, the average values of the Low-Fert (n = 82; −15.16 to −3.60) and High-Fert (n = 82; 14.63 to 25.62) clusters were highly divergent, which allowed us to perform a more robust analysis in terms of fertility (Figure 1).
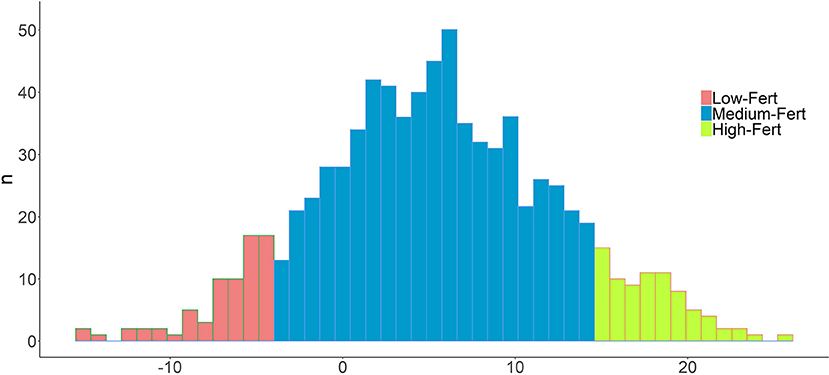
Figure 1. Distribution of Reproductive efficacy pseudophenotypes (RedEBV) in the whole population. Values of the top and bottom 10% individuals are shown in green and red, respectively. A normal distribution is followed (Shapiro test).
Estimations of Molecular Homozygosity (ROH Analysis)
The average FROH in the whole population was high (0.136 ± 0.07). However, it was nearly 30% lower in High-Fert individuals (FROH = 0.102 ± 0.06) compared with Low-Fert (FROH = 0.141 ± 0.07). Similar results were obtained in the analysis per chromosome, in which High-Fert individuals showed a lower FROH than Low-Fert in 19 chromosomes (t-test; P < 0.05) (Figure 2; Supplementary Table 1).
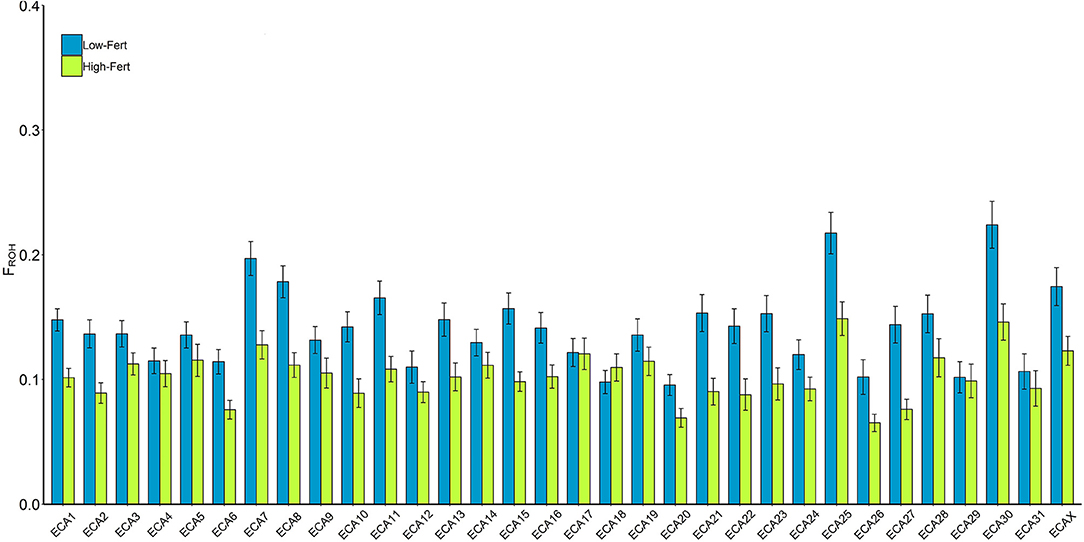
Figure 2. FROH per chromosome in low (blue) and high (green) fertility groups. The values were estimated as the percentage of each chromosome covered per ROH. *Indicates significant (p < 0.05) differences within chromosomes between groups.
Correlation Between Genomic Homozygosity and Re
The correlation between Re and the whole genomic homozygosity estimate (FROH) was negative and highly significant (ρ = −0.147, P < 0.0001, Figure 3). As expected, this value was even more negative in the RD (ρ = −0.241; P < 0.01). However, differences were not evenly detected across the different chromosomes (Supplementary Table 2). For example, the correlations per chromosome estimated in the whole population were negative but highly variable (ranging from −0.033 in ECA30 to −0.134 in ECA15), whereas correlations in the RD showed a similar pattern, but were higher than those observed in the previous analysis. Interestingly, the correlations between Re and FROH were only significant in 18 and 15 of the 32 chromosomes evaluated in the whole population and RD, respectively, suggesting the existence of genomic regions more or less involved in the genetic control of fertility.
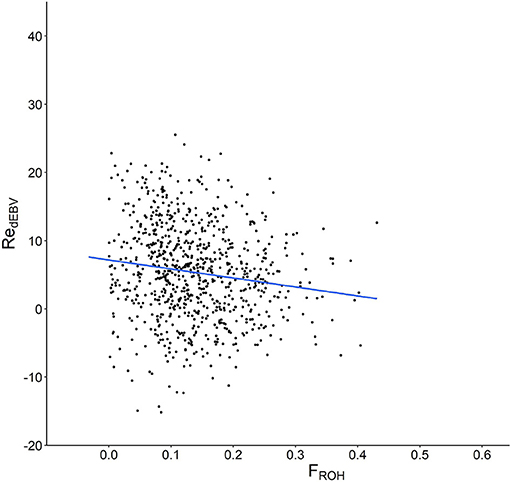
Figure 3. Scatter plot showing the relationship between molecular homozygosity (FROH) in the x axis and the estimated breeding values for mare fertility (RedEBV) in the y axis.
Detection of ROHi and Candidate Genes Associated With Fertility
In total, 41 genomic regions (covering 39.04 Mb in 17 chromosomes) showed a 100-fold increase in ROH abundance in the RD (P < 0.01; Figure 4; Supplementary Table 2). Only 13 of these regions (located on six chromosomes) were significantly associated with RedEBV after validation in the whole population (n = 862; t-test, P < 0.01, Table 1). Of these, three different regions—located in ECA3, 7, and 25—included 17 genes previously associated with biological processes related to female fertility, including oocyte and embryo development.
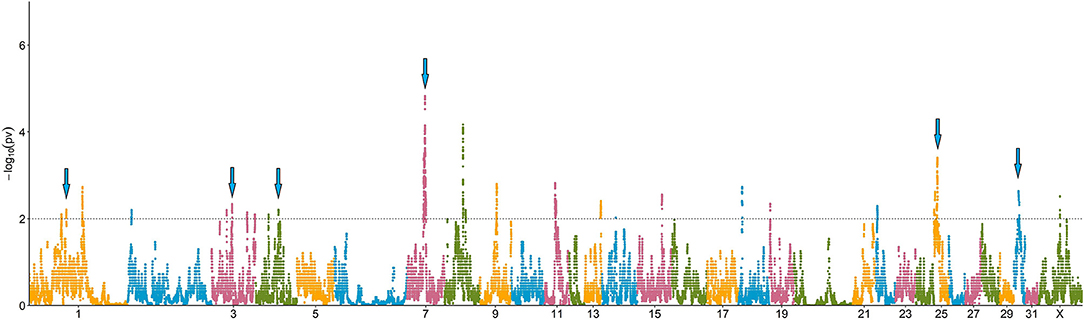
Figure 4. Manhattan plot depicting the incidence of ROH per marker (estimated as -log10(p-value) using a permutation test). Significance was set at a 100-fold increase (dotted line). Cyan arrows show ROHi statistically associated with Re after validation.
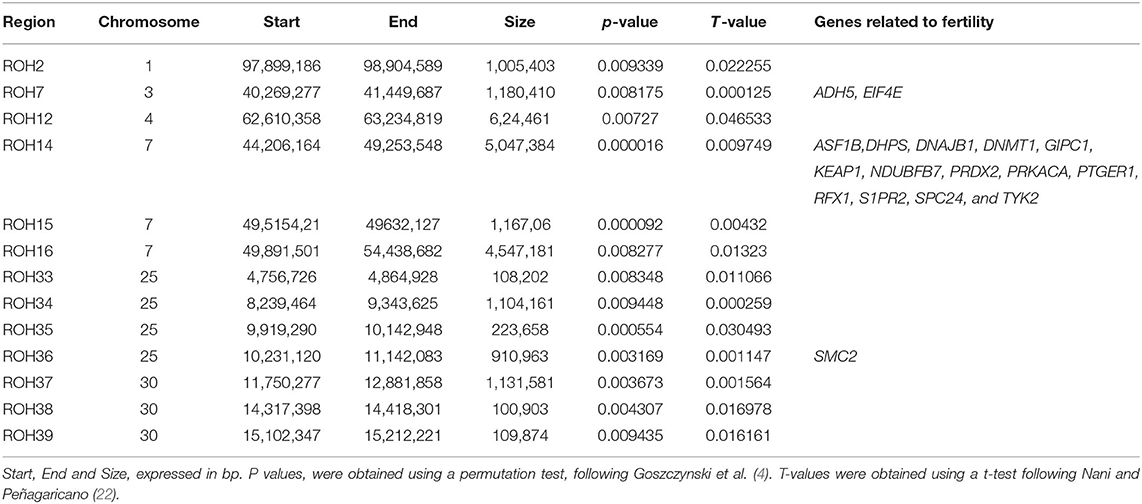
Table 1. ROH islands significantly associated with RedEBV validated in the whole population (n = 862).
Discussion
In this study, we measured the potential effect of increased homozygosity on fertility in mares using a combined approach of high-throughput genomic analysis and genetic estimations of fertility. We explored further into which putative mechanisms, biological processes and genes might be involved in this phenotypic effect by analyzing two groups of individuals with divergent fertility EBVs. Although the negative relationship between inbreeding and fertility has already been demonstrated (37–40), this is the first analysis to use a genomic approach in mares. In addition, our analysis was performed in a breed with a high rate of inbred individuals (41), as well as a wide genetic variability for fertility in mares (10), making it a very interesting experimental model.
Relationship Between Homozygosity and Fertility at Genome and Chromosome Levels
Our results demonstrated a strong, negative correlation between genomic homozygosity (FROH) and fertility (RedEBV), in line with previous reports in this species (39, 40). However, this is the first time this correlation has been obtained by combing genetic estimations and an SNP-based molecular approach. In addition, the analysis of the RD showed a ≈ 40% decrease in homozygosity rates in High-Fert mares, suggesting that the relationship between fertility and inbreeding depression in mares may be based on a threshold model (42), which could explain why the effect is more noticeable when inbreeding values rise above certain values.
Interestingly, the correlations between fertility and homozygosity at chromosome level were highly variable in both whole and reduced datasets. Chromosomal variability in terms of ROH has been recently reported in several horse breeds, such as Mangalarga (43), Halflinger (44), and Norwegian–Swedish (45), although it was not related to changes in fertility in these cases. However, a similar variable pattern in the association between homozygosity load at chromosome level and fertility was reported by Martikainen et al. (46) in Finnish cows. Since reproductive traits are polygenic, some genomic regions obviously explain better the changes observed in breeding values than others. This fact is also supported in our study by the fact that the chromosomes showing the strongest (ECA15) and weakest (ECA18 and ECA29) correlations remained unchanged, regardless of the dataset analyzed. However, the lack of correlation observed in some chromosomes also coincides with the hypothesis proposed by Howard et al. (47), who demonstrated that this null effect could be explained by the co-existence of ROH and homozygous variants in the same region, with unfavorable and favorable outcomes, thus producing a neutral effect. In addition, this variable pattern can also explain the variability in the inbreeding depression load recently reported by our group (41), where some highly inbred individuals showed an increased incidence of morphological defects, whereas others with similar inbreeding values did not. Finally, it is important to mention that the highest correlations between fertility and genomic homozygosity were obtained in the analysis of whole-genome data rather than per chromosome. This fact suggests we should be cautious when using chromosome-level homozygosity values as predictors of inbreeding depression in polygenic traits (or at least in fertility), since genes from several chromosomes probably contribute to the inbreeding depression in fertility and should therefore be taken into account globally.
Effect of Increased Homozygosity on Fertility at Genome Level
Pryce et al. (48) demonstrated that inbreeding depression can be reduced in cattle by avoiding the increased homozygosity in small, specific areas of the genome. These regions, currently known as ROHi, are nowadays the state-of-the-art methodology for studying the mechanisms affected by inbreeding in a given trait (20). However, they can only be reliably measured by using fine-scale genomic methodology and analyzing large populations. In horses, this approach has been used recently to measure regions associated with inbreeding depression in morphological traits in breeds bred for different purposes (21, 43), as well as to evaluate the effect of inbreeding on fertility in stallions (23). Thus far, this methodological approach has not been used in the analysis of fertility in mares.
Our experimental design included the analysis of 172 individuals, showing the highest and lowest RedEBV values from a large population of 850 animals. This methodology, which includes divergent phenotypes, is commonly used in GWAS studies to increase detection power (49) and to determine the effect of inbreeding on fertility in bulls (22); however, it had not been previously used to evaluate the effect of inbreeding depression on female fertility in livestock species. Using this methodology, we detected 41 regions in which the homozygosity rate (at SNP level) had increased significantly (over 100 times greater than the rate of chance occurrence); in addition, 13 of them were associated with changes in fertility, after validation in the whole population (850 horses). This finding concurs with Pryce et al. (48), who demonstrated that increased homozygosity in certain regions can explain the occurrence of inbreeding depression in a specific trait better than others. However, it also highlights the fact that, in order to obtain more reliable results, the analysis of inbreeding depression should be performed in small genomic regions rather than at chromosome or whole genome levels. In addition, the fact that only 13 of 41 regions detected were associated with variation in the fertility of the mare is in agreement with Nani and Peñagaricano (22), who demonstrated that performing a cross-validation test of each specific ROHi against the genetic (or phenotypic) value in a large population is a vital step to avoid the detection of false-positive associations between inbreeding and phenotypes.
Functional Analysis of Candidate Regions
Three genomic regions associated with changes in fertility (ROH7, 14, and 36) contained 17 genes previously related to female reproductive competence in several species. None of them had been previously mapped as QTLs or harbored mutations and/or indels associated with fertility in horses (11). Interestingly, these three regions were among the four regions most closely associated with RedEBV. However, there were no genes associated with fertility in the 10 remaining regions, which showed an association with RedEBVw of a lower magnitude.
The genomic region showing the most significant association with RedEBV was located on ECA3, including two major genes related to female fertility: alcohol dehydrogenase 5 (ADH5) and eukaryotic translation initiation factor 4E (EIF4E). In mice, inhibition of the ADH family reduced the de-novo production of retinol acid in the ovaries and the response to treatment with equine chorionic gonadotropin (50), resulting in decreased developmental competence and quality of the oocytes ovulated. Similarly, eIF4E, known as cap-binding protein, has been associated with the onset of translational activity and meiotic maturation in mammalian oocytes of several species by repressing early oogenesis (51–53). However, when it becomes phosphorylated, the limitation is released (54). This metabolic step has been described as crucial for efficiently initiating the translation and activation of the MAP kinase pathway in the oocyte, which increases the synthesis of several proteins related to spindle formation, allowing the acceleration of oocyte maturation after progesterone induction (52).
ECA25, another region associated with fertility, includes the structural maintenance of chromosomes 2 (SMC2) gene. Its main function is to encode one of the subunits which form the canonical condensin complex (condensin I) in mitotic and meiotic cells (55). Condensin I is required for many functions of meiotic chromosome dynamics (chromosome individualization, resolution, and segregation) and therefore plays a key role in oogenesis progress and blastocyst formation (56).
However, it was a 5Mb genomic region located in ECA 7 which showed the greatest abundance of genes related to female reproduction, although they all showed more indirect associations with fertility, rather than a direct and causative association. Among these, PTGER1 (Subtype 1 Prostaglandin E Receptor 1) is related to ovarian regulation and the onset of decidualization during the pre and post-implantation periods in mice (57); ASF1B modulates and stabilizes chromatin remodeling during the replication-dependent assembly (58), DNAJB1 and NDUFB7 are related to oocyte aging and insulin-dependent changes in mitochondrial activity, respectively, in cattle (59, 60); and GIPC1 has been identified as a part of the IGF1 receptor on Xenopus spp. during oocyte metabolism (61). Similarly, the expression of PRDX2 in external vesicles has been suggested as a predictor of embryo implantation ability (62), while RFX1 and PRKA2 were related to embryonic development failure and lethality in mice (63, 64). The fact that none of these genes were mentioned as pivotal infertility processes suggests that they may be involved in the modulation of fertility in mares by a polygenic pathway, via small genetic effects (11). Despite the fact that this hypothesis should be treated with caution since it was not validated in our study, it supports the idea that using individuals with a reduced inbreeding load (13) for fertility traits may prove an interesting option for mitigating the effect of inbreeding on mare fertility.
In conclusion, we demonstrated for the first time the existence of a negative relationship between increased homozygosity at the genomic level and fertility in a large population of mares. In addition, we proved the existence of small genomic regions in which increased homozygosity negatively affects their fertility to a greater extent. Finally, our functional analysis showed that several genes related to fertility in other species were located within the genomic regions, showing the strongest association with fertility. Our findings can be therefore considered a first step toward determining putative genomic regions which account for the relationship between inbreeding and fertility in mares, which in turn may help to limit the effect of inbreeding depression on fertility in mares.
Data Availability Statement
The original contributions presented in the study are included in the article/Supplementary Material, further inquiries can be directed to the corresponding author/s.
Ethics Statement
Ethical review and approval was not required for the animal study. DNA samples, and genealogical and reproductive data were provided by the ANCCE studbook.
Author Contributions
SD-P, AM, MR, and MV: project conceptualization and experimental design. MR, AE, SD-P, and AM: methodology. AE, MV, and NL: data gathering. NL, FA, MR, and SD-P: data Analysis. NL, AM, MR, and SD-P: manuscript write and review. MV, AM, and SD-P: funding acquisition. AM and SD-P: project management. SD-P: project coordination. All authors contributed to the article and approved the submitted version.
Funding
Funds for this project were obtained from an AGL2017-84217-P grant (MINECO, Spain, PI: AM) and a PICT-2018-0227 grant (ANPCyT, Argentina, PI: SD-P).
Conflict of Interest
The authors declare that the research was conducted in the absence of any commercial or financial relationships that could be construed as a potential conflict of interest.
Publisher's Note
All claims expressed in this article are solely those of the authors and do not necessarily represent those of their affiliated organizations, or those of the publisher, the editors and the reviewers. Any product that may be evaluated in this article, or claim that may be made by its manufacturer, is not guaranteed or endorsed by the publisher.
Acknowledgments
The authors would like to thank the Asociación Nacional de Criadores de Caballos de Pura Raza Española for providing the samples and reproductive data. NL is a MINECO Ph.D. fellow (PRE2018-083492). FA is a PUE-CONICET Ph.D. fellow. Thanks also go to A. Di Maggio for manuscript correction and editing.
Supplementary Material
The Supplementary Material for this article can be found online at: https://www.frontiersin.org/articles/10.3389/fvets.2022.754028/full#supplementary-material
References
1. Leroy G. Inbreeding depression in livestock species: review and meta-analysis. Anim Genet. (2014) 45:618–28. doi: 10.1111/age.12178
2. Keller LF, Waller DM. Inbreeding effects in wild populations. Trends Ecol Evol. (2002) 17:230–41. doi: 10.1016/S0169-5347(02)02489-8
3. Howard JT, Pryce JE, Baes C, Maltecca C. Invited review: inbreeding in the genomics era: Inbreeding, inbreeding depression, and management of genomic variability. J Dairy Sci. (2017) 100:6009–24. doi: 10.3168/jds.2017-12787
4. Goszczynski D, Molina A, Terán E, Morales-Durand H, Ross P, Cheng H, et al. Runs of homozygosity in a selected cattle population with extremely inbred bulls: descriptive and functional analyses revealed highly variable patterns. PLoS One. (2018) 13:e0200069. doi: 10.1371/journal.pone.0200069
5. Perdomo-González DI, Sánchez-Guerrero MJ, Molina A, Valera M. Genetic structure analysis of the pura raza español horse population through partial inbreeding coefficient estimation. Animals. (2020) 10:1360. doi: 10.3390/ani10081360
6. Todd ET, Hamilton NA, Velie BD, Thomson PC. The effects of inbreeding on covering success, gestation length and foal sex ratio in Australian thoroughbred horses. BMC Genet. (2020) 21:41. doi: 10.1186/s12863-020-00847-1
7. Azcona F, Valera M, Molina A, Trigo P, Peral García P, Solé M, et al. Impact of reproductive biotechnologies on genetic variability of Argentine Polo horses. Livest Sci. (2020) 231. doi: 10.1016/j.livsci.2019.103848
8. Gambini A, Maserati M. A journey through horse cloning. Reproduct Fertil Develop. (2018) 30:8–17. doi: 10.1071/RD17374
9. Amos W, Worthington Wilmer J, Fullard K, Burg TM, Croxall JP, Bloch D, et al. The influence of parental relatedness on reproductive success. Proc R Soc B Biol Sci. (2001) 268:2021–7. doi: 10.1098/rspb.2001.1751
10. Gómez MD, Sánchez MJ, Bartolomé E, Cervantes I, Poyato-Bonilla J, Demyda-Peyrás S, et al. Phenotypic and genetic analysis of reproductive traits in horse populations with different breeding purposes. Animal. (2020) 14:1351–61. doi: 10.1017/S1751731120000087
11. Laseca N, Anaya G, Peña Z, Pirosanto Y, Molina A, Demyda Peyrás S. Impaired reproductive function in equines: from genetics to genomics. Animals. (2021) 11: doi: 10.3390/ani11020393
12. Mantovani R, Folla F, Pigozzi G, Tsuruta S, Sartori C. Genetics of lifetime reproductive performance in italian heavy draught horse mares. Animals. (2020) 10:1–14. doi: 10.3390/ani10061085
13. Perdomo-González DI, Molina A, Sánchez-Guerrero MJ, Bartolomé E, Varona L, Valera M. Genetic inbreeding depression load for fertility traits in Pura Raza Española mares. J Anim Sci. (2021) 99:skab316. doi: 10.1093/jas/skab316
14. McGivney BA, Han H, Corduff LR, Katz LM, Tozaki T, MacHugh DE, et al. Genomic inbreeding trends, influential sire lines and selection in the global thoroughbred horse population. Sci Rep. (2020) 10:466. doi: 10.1038/s41598-019-57389-5
15. Ricard A, Duluard A. Genomic analysis of gaits and racing performance of the French trotter. J Anim Breed Gen. (2021) 138:204–22. doi: 10.1111/jbg.12526
16. Sole M, Ablondi M, Binzer-Panchal A, Velie BD, Hollfelder N, Buys N, et al. Inter- and intra-breed genome-wide copy number diversity in a large cohort of European equine breeds. BMC Genom. (2019) 20:759. doi: 10.1186/s12864-019-6141-z
17. Poyato-Bonilla J, Laseca N, Demyda Peyrás S, Molina A, Valera M. 500 years of breeding in the Carthusian Strain of Pura Raza Español horse: an evolutional analysis using genealogical genomic data. J Anim Breed Gen. (2021) 139:84–99. doi: 10.1111/jbg.12641
18. Curik I, Ferencakovic M, Solkner J. Genomic dissection of inbreeding depression: a gate to new opportunities. Rev Brasil Zoot. (2017) 46:773–82. doi: 10.1590/s1806-92902017000900010
19. McQuillan R, Leutenegger AL, Abdel-Rahman R, Franklin CS, Pericic M, Barac-Lauc L, et al. Runs of homozygosity in European populations. Am J Hum Genet. (2008) 83:359–72. doi: 10.1016/j.ajhg.2008.08.007
20. Gorssen W, Meyermans R, Janssens S, Buys N. A publicly available repository of ROH islands reveals signatures of selection in different livestock and pet species. Genet Sel Evol. (2021) 53:2. doi: 10.1186/s12711-020-00599-7
21. Grilz-Seger G, Druml T, Neuditschko M, Mesaric M, Cotman M, Brem G. Analysis of ROH patterns in the Noriker horse breed reveals signatures of selection for coat color and body size. Anim Genet. (2019) 50:334–46. doi: 10.1111/age.12797
22. Nani JP, Peñagaricano F. Whole-genome homozygosity mapping reveals candidate regions affecting bull fertility in US Holstein cattle. BMC Genom. (2020) 21:338. doi: 10.1186/s12864-020-6758-y
23. Metzger J, Karwath M, Tonda R, Beltran S, Agueda L, Gut M, et al. Runs of homozygosity reveal signatures of positive selection for reproduction traits in breed and non-breed horses. BMC Genom. (2015) 16:764. doi: 10.1186/s12864-015-1977-3
24. Henderson CR. A simple method for computing the inverse of a numerator relationship matrix used in prediction of breeding values. Biometrics. (1976) 32:69−83. doi: 10.2307/2529339
25. Misztal I, Tsuruta S, Strabel T, Auvray B, Druet T, Lee DH. BLUPF90 and related programs, 7th World Congress on Genetics Applied to Livestock Production, Montpellier, France (2002).
26. Garrick DJ, Taylor JF, Fernando RL. Deregressing estimated breeding values and weighting information for genomic regression analyses. Genet Sel Evol. (2009) 41:55. doi: 10.1186/1297-9686-41-55
27. Schaefer RJ, Schubert M, Bailey E, Bannasch DL, Barrey E, Bar-Gal GK, et al. Developing a 670k genotyping array to tag ~2M SNPs across 24 horse breeds. BMC Genom. (2017) 18:565. doi: 10.1186/s12864-017-3943-8
29. Meyermans R, Gorssen W, Buys N, Janssens S. How to study runs of homozygosity using PLINK? a guide for analyzing medium density snp data in livestock and pet species. BMC Genom. (2020) 21:94. doi: 10.1186/s12864-020-6463-x
30. R-Core-Team. R: A language environment for statistical computing V4.1.0 “Camp Pontanezen”. In: V. R Foundation for Statistical Computing, Austria (Ed.), 2021.
31. Biscarini F, Cozzi P, Gaspa G, Marras G. DetectRUNS: Detect runs of homozygosity and runs of heterozygosity in diploid genomes in R 2018.
33. Beeson SK, Schaefer RJ, Mason VC, McCue ME. Robust remapping of equine SNP array coordinates to EquCab3. Anim Genet. (2019) 50:114–5. doi: 10.1111/age.12745
35. Huang da W, Sherman BT, Lempicki RA. Systematic and integrative analysis of large gene lists using DAVID bioinformatics resources. Nat Protoc. (2009) 4:44–57. doi: 10.1038/nprot.2008.211
36. Mi H, Huang X, Muruganujan A, Tang H, Mills C, Kang D, et al. PANTHER version 11: expanded annotation data from Gene Ontology and Reactome pathways, and data analysis tool enhancements. Nucleic Acids Res. (2017) 45:D183–D9. doi: 10.1093/nar/gkw1138
37. Cothran EG, Maccluer JW, Weitkamp LR, Pfennig DW, Boyce AJ. Inbreeding and reproductive performance in standardbred horses. J Hered. (1984) 75:220–4. doi: 10.1093/oxfordjournals.jhered.a109916
38. Valera M, Blesa F, Santos RD, Molina A. Genetic study of gestation length in andalusian and arabian mares. Anim Reprod Sci. (2006) 95:75–96. doi: 10.1016/j.anireprosci.2005.09.008
39. Sairanen J, Nivola K, Katila T, Virtala AM, Ojala M. Effects of inbreeding and other genetic components on equine fertility. Animal. (2009) 3:1662–72. doi: 10.1017/S1751731109990553
40. Mueller-Unterberg M, Wallmann S, Distl O. Effects of inbreeding and other systematic effects on fertility of Black Forest Draught horses in Germany. Acta Vet Scand. (2017) 59:70. doi: 10.1186/s13028-017-0338-4
41. Poyato-Bonilla J, Perdomo-González DI, Sánchez-Guerrero MJ, Varona L, Molina A, Casellas J, et al. Genetic inbreeding depression load for morphological traits and defects in the Pura Raza Española horse. Genet Sel Evol. (2020) 52:62. doi: 10.1186/s12711-020-00582-2
42. Frankham R. Inbreeding and extinction: a threshold effect. Conserv Biol. (1995) 9:792–9. doi: 10.1046/j.1523-1739.1995.09040792.x
43. dos Santos WB, Schettini GP, Fonseca MG, Pereira GL, Chardulo LAL, Neto ORM, et al. Fine-scale estimation of inbreeding rates, runs of homozygosity and genome-wide heterozygosity levels in the Mangalarga Marchador horse breed. J Anim Breed Gen. (2020) 138:13. doi: 10.1111/jbg.12508
44. Grilz-Seger G, Mesarič M, Cotman M, Neuditschko M, Druml T, Brem G. Runs of homozygosity and population history of three horse breeds with small population size. J. Equine Vet Sci. (2018) 71:27–34. doi: 10.1016/j.jevs.2018.09.004
45. Velie BD, Solé M, Fegraeus KJ, Rosengren MK, Røed KH, Ihler CF, et al. Genomic measures of inbreeding in the norwegian-swedish coldblooded trotter and their associations with known QTL for reproduction and health traits. Genet Sel Evol. (2019) 51:22. doi: 10.1186/s12711-019-0465-7
46. Martikainen K, Sironen A, Uimari P. Estimation of intrachromosomal inbreeding depression on female fertility using runs of homozygosity in Finnish Ayrshire cattle. J Dairy Sci. (2018) 101:11097–107. doi: 10.3168/jds.2018-14805
47. Howard JT, Tiezzi F, Huang Y, Gray KA, Maltecca C. A heuristic method to identify runs of homozygosity associated with reduced performance in livestock. J Anim Sci. (2017) 95:4318–32. doi: 10.2527/jas2017.1664
48. Pryce JE, Haile-Mariam M, Goddard ME, Hayes BJ. Identification of genomic regions associated with inbreeding depression in Holstein and Jersey dairy cattle. Genet Sel Evol. (2014) 46:71. doi: 10.1186/s12711-014-0071-7
49. Visscher PM, Wray NR, Zhang Q, Sklar P, McCarthy MI, Brown MA, et al. 10 Years of GWAS Discovery: Biology, Function, Translation. Am J Hum Genet. (2017) 101:5–22. doi: 10.1016/j.ajhg.2017.06.005
50. Kawai T, Yanaka N, Richards JS, Shimada M. De novo-synthesized retinoic acid in ovarian antral follicles enhances fsh-mediated ovarian follicular cell differentiation and female fertility. Endocrinology. (2016) 157:2160–72. doi: 10.1210/en.2015-2064
51. Ellederova Z, Kovarova H, Melo-Sterza F, Livingstone M, Tomek W, Kubelka M. Suppression of translation during in vitro maturation of pig oocytes despite enhanced formation of cap-binding protein complex eIF4F and 4E-BP1 hyperphosphorylation. Mol Reprod Dev. (2006) 73:68–76. doi: 10.1002/mrd.20368
52. Romasko EJ, Amarnath D, Midic U, Latham KE. Association of maternal mRNA and phosphorylated EIF4EBP1 variants with the spindle in mouse oocytes: Localized translational control supporting female meiosis in mammals. Genetics. (2013) 195:349–58. doi: 10.1534/genetics.113.154005
53. Tomek W, Sterza FAM, Kubelka M, Wollenhaupt K, Torner H, Anger M, et al. Regulation of translation during in vitro maturation of bovine oocytes: The role of MAP kinase, eIF4E (cap binding protein) phosphorylation, and eIF4E-BP1. Biol Reprod. (2002) 66:1274–82. doi: 10.1095/biolreprod66.5.1274
54. Rhoads RE EIF4E: New family members new binding partners new roles. J. Biol. Chem. (2009) 284:16711–15. doi: 10.1074/jbc.R900002200
55. Lee J, Ogushi S, Saitou M, Hirano T. Condensins I and II are essential for construction of bivalent chromosomes in mouse oocytes. Mol Biol Cell. (2011) 22:3465–77. doi: 10.1091/mbc.e11-05-0423
56. Zhou TP, Zhang D, Cai LB, Xu YX, Shao L, Liu KL, et al. Expression of target genes in cumulus cells derived from human oocytes with and without blastocyst formation. Reprod Dev Med. (2019) 3:84–8. doi: 10.4103/2096-2924.262387
57. Yang ZM, Das SK, Wang J, Sugimoto Y, Ichikawa A, Dey SK. Potential sites of prostaglandin actions in the periimplantation mouse uterus: differential expression and regulation of prostaglandin receptor genes. Biol Reprod. (1997) 56:368–79. doi: 10.1095/biolreprod56.2.368
58. El-Gammal Z, AlOkda A, El-Badri N. Role of human oocyte-enriched factors in somatic cell reprograming. Mech Ageing Dev. (2018) 175:88–99. doi: 10.1016/j.mad.2018.05.009
59. Itami N, Kawahara-Miki R, Kawana H, Endo M, Kuwayama T, Iwata H. Age-associated changes in bovine oocytes and granulosa cell complexes collected from early antral follicles. J Assist Reprod Genet. (2014) 31:1079–88. doi: 10.1007/s10815-014-0251-y
60. Laskowski D, Båge R, Humblot P, Andersson G, Sirard MA, Sjunnesson Y. Insulin during in vitro oocyte maturation has an impact on development, mitochondria, and cytoskeleton in bovine day 8 blastocysts. Theriogenology. (2017) 101:15–25. doi: 10.1016/j.theriogenology.2017.06.002
61. Booth RA, Cummings C, Tiberi MJohné Liu X. GIPC participates in G protein signaling downstream of insulin-like growth factor 1 receptor. J Biol Chem. (2002) 277:6719–25. doi: 10.1074/jbc.M108033200
62. Rai A, Poh QH, Fatmous M, Fang H, Gurung S, Vollenhoven B, et al. Proteomic profiling of human uterine extracellular vesicles reveal dynamic regulation of key players of embryo implantation and fertility during menstrual cycle. Proteomics. (2021) 21:e2000211. doi: 10.1002/pmic.202000211
63. Feng C, Xu W, Zuo Z. Knockout of the regulatory factor X1 gene leads to early embryonic lethality. Biochem Biophys Res Commun. (2009) 386:715–7. doi: 10.1016/j.bbrc.2009.06.111
Keywords: runs of homozygosity, genomics, reproductive efficiency, mares, inbreeding, SNP, fertility, ROHi
Citation: Laseca N, Molina A, Ramón M, Valera M, Azcona F, Encina A and Demyda-Peyrás S (2022) Fine-Scale Analysis of Runs of Homozygosity Islands Affecting Fertility in Mares. Front. Vet. Sci. 9:754028. doi: 10.3389/fvets.2022.754028
Received: 05 August 2021; Accepted: 10 January 2022;
Published: 17 February 2022.
Edited by:
Regiane R. Santos, Schothorst Feed Research, NetherlandsReviewed by:
Abouzar Najafi, University of Tehran, IranFilippo Biscarini, National Research Council (CNR), Italy
Copyright © 2022 Laseca, Molina, Ramón, Valera, Azcona, Encina and Demyda-Peyrás. This is an open-access article distributed under the terms of the Creative Commons Attribution License (CC BY). The use, distribution or reproduction in other forums is permitted, provided the original author(s) and the copyright owner(s) are credited and that the original publication in this journal is cited, in accordance with accepted academic practice. No use, distribution or reproduction is permitted which does not comply with these terms.
*Correspondence: Sebastián Demyda-Peyrás, c2RlbXlkYUBmY3YudW5scC5lZHUuYXI=