- 1Engineering and Technology Research Center of Traditional Chinese Veterinary Medicine of Gansu Province, Lanzhou Institute of Husbandry and Pharmaceutical Sciences, Chinese Academy of Agricultural Sciences (CAAS), Lanzhou, China
- 2College of Veterinary Medicine, Northwest A&F University, Yangling, China
Dampness-heat diarrhea (DHD), a common syndrome in Chinese dairy farms, is mainly resulted from digestive system disorders, and accompanied with metabolic disorders in some cases. However, the underlying mechanisms in the intestinal microbiome and plasma metabolome in calves with DHD remain unclear. In order to investigate the pathogenesis of DHD in calves, multi-omics techniques including the 16S rDNA gene sequencing and metabolomics were used to analyze gut microbial compositions and plasma metabolic changes in calves. The results indicated that DHD had a significant effect on the intestinal microbial compositions in calves, which was confirmed by changes in microbial population and distribution. A total of 14 genera were changed, including Escherichia-Shigella, Bacteroides, and Fournierella, in calves with DHD (P < 0.05). Functional analysis based on the Kyoto Encyclopedia of Genes and Genomes (KEGG) annotations indicated that 11 metabolic functions (level 2) were significantly enriched in DHD cases. The untargeted metabolomics analysis showed that 440 metabolites including bilineurin, phosphatidylcholine, and glutamate were significantly different between two groups (VIP > 1 and P < 0.05), and they were related to 67 signal pathways. Eight signal pathways including alpha-linolenic acid, linoleic acid, and glycerophospholipid metabolism were significantly enriched (P < 0.05), which may be potential biomarkers of plasma in calves with DHD. Further, 107 pairs of intestinal microbiota-plasma metabolite correlations were determined, e.g., Escherichia-Shigella was significantly associated with changes of sulfamethazine, butyrylcarnitine, and 14 other metabolites, which reflected that metabolic activity was influenced by the microbiome. These microbiota-metabolite pairs might have a relationship with DHD in calves. In conclusion, the findings revealed that DHD had effect on intestinal microbial compositions and plasma metabolome in calves, and the altered metabolic pathways and microorganisms might serve as diagnostic markers and potential therapeutic targets for DHD in calves.
Introduction
Diarrhea, the most common disease in calves, is one of the major causes of economic loss in cattle herds worldwide (1, 2). Neonatal calves are susceptible to bovine coronavirus, Escherichia coli, bovine rotavirus, and Cryptosporidium parvum, which can cause diarrhea in calf (3–5). In addition, some non-infectious factors including colostrum management, calf housing, and hygiene are inducer of diarrhea in calf (6, 7). The rational use of traditional Chinese medicine (TCM) to treat this disease has certain advantages (8). Different types of diarrhea require different prescriptions based on the theory of TCM. According to the clinical symptoms, diarrhea can be divided into dampness-heat diarrhea (DHD), spleen deficiency diarrhea, and kidney deficiency diarrhea (9–11). DHD is one of the most common syndromes in Chinese cattle herds. According to traditional Chinese veterinary medicine (TCVM), DHD is usually caused by the exogenous heat and dampness toxin pathogens invading the intestine (12). The main clinical symptoms of DHD in calves are hyperthermia, sticky and loose stools with blood and mucus, red tongue, and thick greasy tongue-coating (9, 13). The mechanism of DHD has not been fully elucidated. This study provides new insights on understanding the pathogenesis and rational treatment of DHD in calves.
The alteration of gut microbial composition is associated with many diseases (14). Diarrheal diseases often alter the richness and diversity of intestinal flora (15, 16). Therefore, the microbiota is an important factor in the maintenance of health and the development of disease (17). The 16S rDNA gene amplicon sequencing, a powerful tool for routine microbial identification, can help researchers to identify, categorize, and understand the complex interactions between the host, pathogen, and microbiome (18, 19). Studies have confirmed that the increase of Firmicutes and Bacteroidetes proportion is an important manifestation of diarrhea, and diarrhea could be alleviated when this abnormal proportion was adjusted to be normal (20, 21). Gegenqinlian decoction, a classical TCM prescription, regulated the balance of intestinal mucosa flora in mice with diarrhea induced by high temperature and humidity (22). Therefore, it might be a helpful strategy for elucidation of the pathogenic mechanism of DHD to study of gut microbial composition in calves.
Qualitative and quantitative analysis of low molecular weight metabolites in biological samples can reflect the influence of diseases on metabolic in body (23, 24). In recent years, a great deal of research has been conducted on the metabolomics in a lot of diseases (25–28). The 16S rRNA gene sequencing with untargeted metabolomics can present much better perspectives on physiological and metabolic mechanisms involved in the pathogenesis of DHD. Some metabolites in plasma such as chenodeoxycholic acid and creatinine were found to the biomarkers for colorectal cancer diagnosis and prognosis (29). Metabolomics was utilized to study the molecular mechanisms of Atractylodis Rhizoma in the treatment of spleen deficiency (30). Therefore, we hypothesized that the changes of metabolic and intestinal microbiological might be related to DHD in calves. In this study, the difference in fecal microbiome and plasma metabolic profile between DHD calves and healthy calves was detected by 16S ribosomal DNA gene sequencing and metabolomics, and the results revealed that DHD had effect on intestinal microbial compositions and plasma metabolome, and the altered metabolic pathways and microorganisms might serve as diagnostic markers and potential therapeutic targets for DHD in calves.
Materials and Methods
Experimental Animals
The experiment was carried out in a dairy farm in north-western China. The calves in this study were similar in genetic background and age, and all of enrolled animals were housed and fed under same conditions. A total of 6 DHD calves and 6 healthy calves were enrolled in this study. The standards of dampness-heat diarrhea in the literature are mostly determined by primary and secondary symptoms (9, 10, 13). The inclusion criteria for DHD cases were required two main symptoms and secondary symptoms, or three main symptoms (Table 1). The specific clinical syndrome differentiation was carried out by the author and a Chinese veterinary expert. According to the diagnostic criteria, we screened 6 eligible calves from 129 diarrhea cases as DHD group and six healthy calves as the control group. The enrolled calves were not given any probiotics or antibiotics prior to sample collection. All animal procedures were carried out in accordance with the Guidelines on Laboratory Animal Ethics Commission of the Lanzhou Institute of Husbandry and Pharmaceutical Sciences of CAAS (SYXK [Gan] 2019-0002).
Fecal Sample Collection and DNA Extraction
Fresh fecal samples were collected by inserting an anal swab into the anus. The anal swabs were deposited into a sterile sampling tube and sent to the laboratory within 2 h. The fecal samples were immediately frozen in liquid nitrogen until the microbial DNA was extracted.
Microbial DNA was extracted from each fecal sample using the HiPure Fecal DNA Kit (Magen, Guangzhou, China) according to kit instructions. The DNA purity was determined using a Nanodrop microspectrophotometer (NanoDrop 2000, Thermo Fisher Scientific, America) and DNA integrity was investigated using the agarose gel electrophoresis.
Plasma Sample Collection and Metabolite Extraction
Blood was collected from the jugular vein of the calves and stored in an anticoagulant tube containing ethylenediaminetetraacetic acid (EDTA), and was temporarily stored in an incubator at 4°C and sent to the laboratory within 2 h. The plasma was separated after centrifugation at 3,000 rpm, and 4°C for 15 min, and then frozen at −80°C until metabolite extraction.
Metabolites were extracted separately from plasma. After addition of 300 μL of methanol and 20 μL internal references in 100 μL plasma, the samples were mixed briefly and sonicated for 5 min in an ice-water bath. Following incubation at −20°C for 2 h, the mixture was centrifuged at 13,000 rpm at 4°C for 15 min. The supernatants were transferred to liquid chromatography-mass spectrometry (LC-MS) vials and stored at −80°C until the UHPLC-QE Orbitrap/MS analysis (Agilent, America).
16S RDNA Gene Sequencing Analysis
The 16S rDNA V3-V4 region was amplified using a specific primer with barcode 341F: CCTACGGGNGGCWGCAG; 806R: GGACTACHVGGGTATCTAAT (31). The prokaryotic 16S fragment was amplified by a relevant PCR reagent (Toyobo, Japan), and the PCR conditions consisted of an initial denaturation at 94°C for 2 min, followed by 30 cycles at 98°C for 10 s, 62°C for 30 s, and 68°C for 30 s and a final extension at 68°C for 5 min. PCR reactions were performed in triplicate.
The amplification material was extracted from a 2% agarose gel and purified using the AxyPrep DNA gel extraction kit (Axygen Biosciences, Union City, CA, USA) according to the manufacturer's instructions, and quantified using the ABI Step-OnePlus Real-Time PCR System (Life Technologies, Foster City, USA). The purified amplicons were polymerized on the Illumina platform with equimolar and paired-end sequence (PE250) according to the standard protocol. The original reads were stored in the NCBI Sequence Read Archive database (PRJNA713974).
DNA library sequencing was performed by Genedenovo Biotechnology Co., Ltd (Guangzhou, China) on the Illumina HiseqTM 2500. To obtain the effective tags, the raw tags of sequences were spliced and filtered. Detailed steps are described in the Supplementary Material. The effective tags were clustered into operational taxonomic units (OTUs) with >97% similarity using UPARSE (32). The representative sequences were classified by a naive Bayesian model with an RDP classifier (version 2.2) based on the SILVA database (version 132) with a confidence threshold of 0.8. The abundance statistics of taxonomy were visualized using KRONA (version 2.6) (33–35). The beta-diversity was assessed by calculating the weighted unifrac distances matrix and Bray-Curtis distance matrix dissimilarity, which was visualized through the use of principal coordinate analysis (PCoA). Species comparison among groups of various classification levels was calculated by the Wilcoxon rank test (P < 0.05). Tax4Fun functional prediction was used to infer the Kyoto Encyclopedia of Genes and Genomes (KEGG) pathway analysis of the OTUs. Analysis of function difference between groups was calculated by the Wilcoxon rank test.
Metabolomics Analysis
The extracted metabolites were detected and quantitated with a liquid chromatography-tandem mass spectrometry (LC-MS/MS). Details of LC-MS/MS analysis were available in the Supplementary Material.
A data matrix containing retention time (RT), mass/charge ratio (M/Z), and peak strength was generated by preprocessing the MS raw data using the profile analysis software. Principal component analysis (PCA) and orthogonal partial least squares discriminant analysis (OPLS-DA) algorithms were used to compare the metabolite distribution using R package models (http://www.r-project.org/). The variable importance in projection (VIP) values of OPLS-DA and the P-values of the t-test were used to screen the metabolites with significant differences between the two groups (36). Those with a P < 0.05 on the t-test and VIP > 1 were preliminarily determined as the different metabolites between two groups. Pathway analysis and enrichment analysis of the metabolites were carried out by locating them to the associated pathway. The calculated P-value was corrected by false discovery rate (FDR), and FDR ≤ 0.05 as the threshold. The pathway satisfied this condition was defined as the pathway of significant enrichment in differential metabolites.
Correlation Analysis of Fecal Microbiota and Plasma Metabolites
Bidirectional orthogonal projections to latent structures (O2PLS) analysis were used to integrate the microbiota and metabolomic data (37, 38). The significantly different metabolites and microbes were calculated by the Pearson statistical method, and the final correlation and the network map between them were obtained (39).
Results
Differences in Bacterial Communities Between DHD Calves and Healthy Calves
The fecal microbiome diversity of calves with DHD was investigated by 16S rDNA gene sequencing. The similarity of sequences contained in the same OTUs was ≥97%. The total number of effective tags and OTUs is shown in Table 2, OTUs was over 1,000 and effective tags was between 14,338 and 42,083 in samples. The Venn diagram (Figure 1A) shows the common and unique OTUs, 568 was the common OTUs, 373 OTUs in the control group, and 326 OTUs in DHD group. In order to characterize the similarity and difference in community abundance and composition, the beta diversity of microbiota was measured by PCoA. The result of the weighted Unifrac distance was ANOSIM R = 0.907, P = 0.002 (Figure 1B), and the Bray-Curtis analysis was ANOSIM R = 0.876, P = 0.004 (Figure 1C), both analyses indicated that the microflora of DHD group was significantly different from that of the control group.
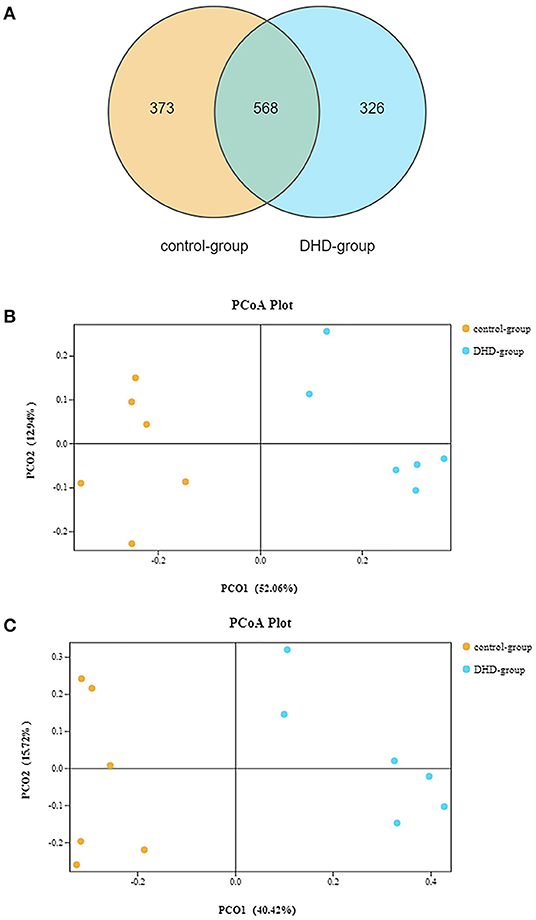
Figure 1. Gut microbial diversity in DHD and healthy calves. (A) Venn diagram showing the number of common or unique OTUs. (B) PCA by the weighted Unifrac of beta diversity of the OTU levels. (C) PCA by Bray-Curtis analysis of beta diversity at the OTU levels.
Alterations in Intestinal Microbiota Composition in Calves With DHD
The relative abundance of the top 10 bacteria at the genus level is shown in Figure 2A, which indicates that the bacterial composition of feces in healthy and DHD calves is different, and Escherichia-Shigella (44.84%) was the predominant genus in the diseased calves. In healthy calves, the prevalent genera were Bacteroides (20.72%), Fournierella (7.38%), and Escherichia-Shigella (5.50%). The Wilcoxon rank sum test was used to compare the fecal bacterial community at the genus level, and 14 genera were significantly different between the two groups (P < 0.05). In these discriminatory genera, the abundance of Escherichia-Shigella and Enterococcus were increased significantly, and the abundance of Bacteroides, Fournierella, Subdoligranulum, Lachnoclostridium, and other 8 genera were decreased significantly in DHD calves (Figure 2B).
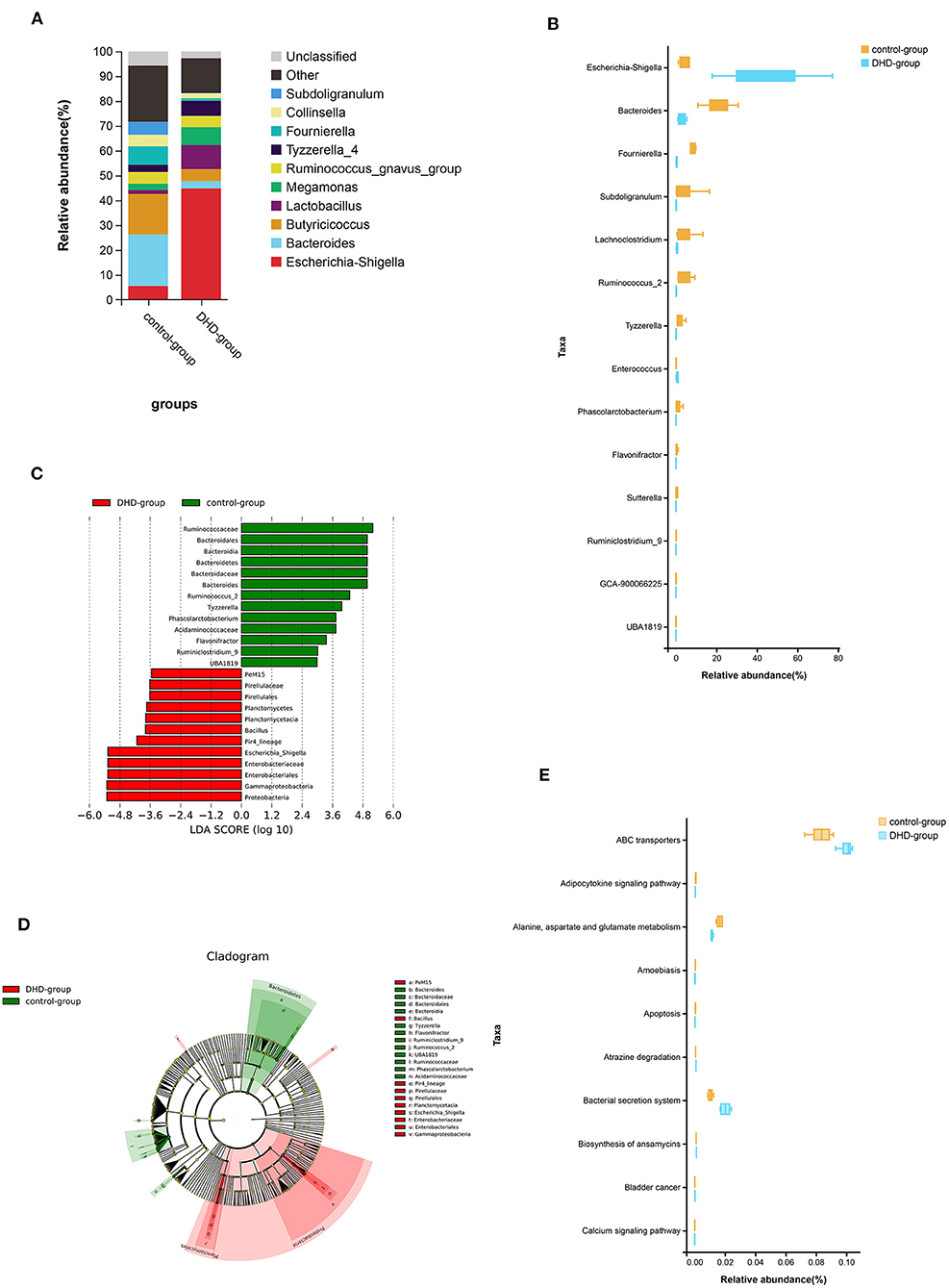
Figure 2. Fecal microbial abundance and diversity in DHD calves and healthy calves. (A) Taxonomic distributions of bacteria at the genus level (top 10) between DHD and healthy calves. (B) Box plot of the generic bacteria with significantly different between these two groups. Data for each group are shown as relative abundances. The Wilcoxon rank-sum test was used for statistical analysis. (C) The cladogram obtained by linear discriminant analysis effect size (LEfSe) analysis showed the phylogenetic distribution of the microflora of DHD calves and control calves from phylum to genus. (D) LDA score histograms used to identify bacterial genera (LDA score > 2) differed significantly between DHD calves and control calves. (E) Box plot of the top 10 significantly different KEGG pathways that were predicted between these two groups.
Linear discriminant analysis effect size (LEfSe) was used to further determine whether there was a differential enrichment of specific bacterial groups in calves. A total of 25 discriminatory taxa were clearly displayed in the linear discriminant analysis (LDA) score cutoff of 2.0 (Figure 2C). The Pir4_lineage, Bacillus, and Escherichia_Shigella were significantly over-represented in feces of DHD calves, whereas Flavonifractor, Tyzzerella, Ruminiclostridium_9, Phascolarctobacterium, UBA1819, Bacteroides, and Ruminococcus_2 were the crucial microorganisms in healthy calves. A cladogram of the taxonomic hierarchy of fecal microbiota from phylum to genus illustrated a remarkable difference in the phylogenetic distribution of the microbiota between DHD calves and healthy calves (Figure 2D).
Tax4Fun was used to predict KEGG pathways in 16S rDNA sequencing result, and 284 pathways were predicted, most of which were related to metabolism (Supplementary Table 1), and 170 pathways were significantly different between DHD calves and healthy calves (P < 0.05). The top 10 pathways including adipocytokine and calcium, alanine, aspartate and glutamate metabolism, and apoptosis were shown in Figure 2E.
Correlation Network Analysis
Correlation network analysis was carried out at the genus level to determine whether DHD was associated with changes in the related structures and putative interactions of the intestinal microbiota. The correlation network diagrams of the top 50 genera with the richest abundant in DHD animals and healthy animals were represented in Figures 3A,B. The network had more edges (95 vs. 81) and higher mean degree (3.725 vs. 3) in the control group than those in the DHD group, which showed that the interaction structures of genus in the two groups were different.
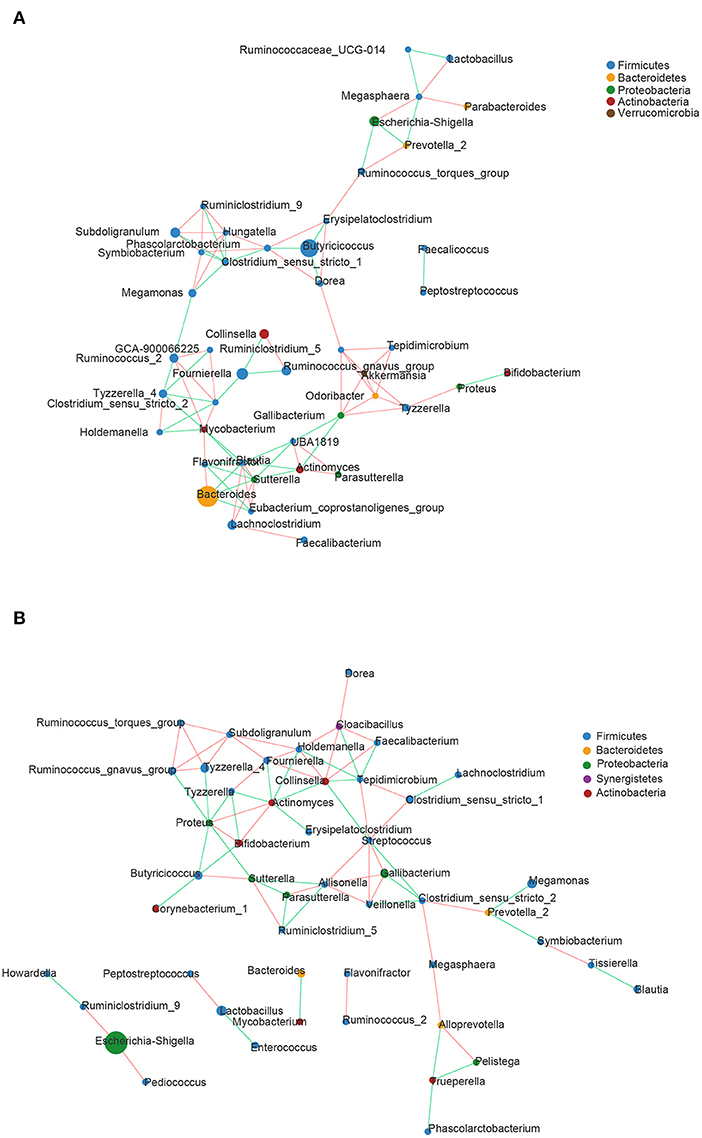
Figure 3. Correlation network diagrams of the 50 richest abundant genera of (A) healthy and (B) DHD calves. The lines between nodes represent the Spearman correlation and the color intensity represents the correlation coefficient (red, positive; green, negative). The color of the genera was based on phylum affiliation, and the size indicates average relative abundance.
Metabolic Variation Analysis
Metabolite identification results showed that there were 8,145 and 7,074 peaks in positive and negative ion modes, respectively. PCA analysis indicated that the metabolites in plasma from tested calves were clearly separated in the positive and negative mode (Figures 4A,B). Potential metabolic biomarkers were screened using the multivariate model OPLS-DA (VIP > 1 and P < 0.05), and 440 potential metabolic biomarkers (Supplementary Table 2) were different between DHD calves and healthy calves.
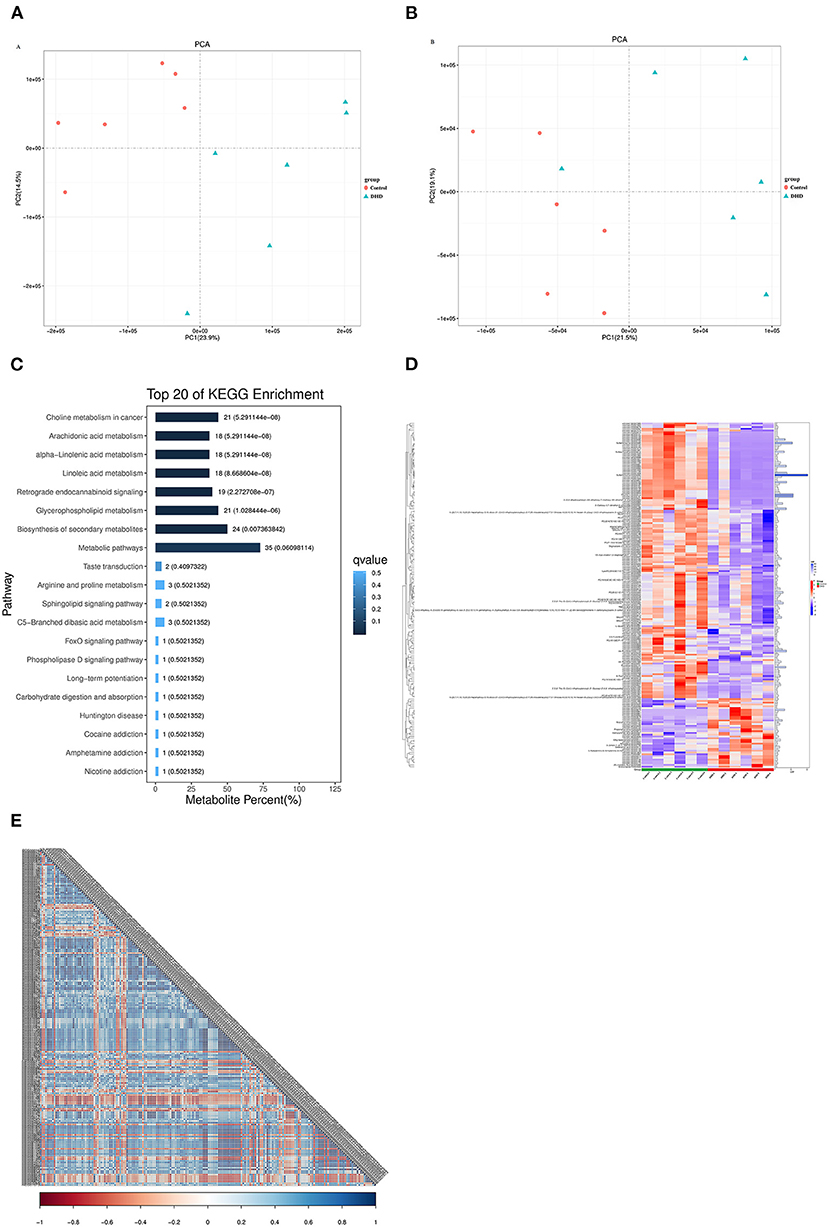
Figure 4. Plasma metabolic profiles in DHD and control calves. PCA score of plasma metabolite analysis between DHD calves and healthy calves in (A) positive ion mode and (B) negative ion mode. (C) Results of the KEGG pathway enrichment analysis of significantly different metabolites (top 20). The horizontal axis represents the percentage of the number of metabolites in this pathway to the total number of significantly different metabolites, and the value on the histogram is the number of differential metabolites in this pathway and the Q value. (D) Significant differences in metabolites between DHD calves and control calves are shown in the hierarchical clustering and heat map on the left (columns, individual; rows, specific metabolite). The histogram on the right represents the VIP score for each metabolite derived from the OPLS-DA model. (E) Correlation coefficient matrix thermograph illustrating the functional correlation between the significantly altered metabolites in plasma. The correlation coefficient is expressed by color, blue is positive correlation and red is negative correlation. The darker the color, the stronger the correlation.
To compare the main biochemical metabolic pathways and signal transduction pathways involved in differential metabolites between DHD calves and healthy calves, the enrichment analysis of pathways on these metabolites was evaluated by KEGG. The significantly different metabolites were enriched into 67 pathways, 8 pathways of them were significantly changed in DHD calves, including metabolism of arachidonic acid, alpha-linolenic acid, linoleic acid, and glycerophospholipid (P < 0.05, Figure 4C).
In present study, the metabolites with VIP > 2 were considered to be significantly different, and 194 metabolites were identified (Supplementary Table 3). The tendencies in variation of the 194 metabolites were depicted in a heat map (Figure 4D), and 39 metabolites were increased and 155 metabolites were decreased in DHD calves (P < 0.05). Most of different metabolites was Lipids and lipid-like molecules, and glycerophospholipids such as PC [16:0/20:2(11Z,14Z)], PC [20:2(11Z,14Z)/14:0], and PC [22:4(7Z,10Z,13Z,16Z)/16:0] were down-regulated in DHD calves, and some fatty acyls such as ethyl dodecanoate and butyrylcarnitine presented at higher levels in DHD calves. In addition, the correlation coefficient matrix thermograph showed an obvious correlation in these 194 metabolites (Figure 4E).
Correlation Analysis of Intestinal Microbiota and Plasma Metabolites
In order to investigate the functional correlation between 14 microbial communities at the genus level and 194 metabolites with significant differences, a correlation matrix was calculated using Spearman's correlation coefficient. As shown in Figure 5, 107 pairs of microbiota-metabolite were determined, including 102 positive correlations and 5 negative correlations (| cor | ≥ 0.75 and P < 0.01). Specially, Ruminococcus_2, Phascolarctobacterium, Flavonifractor, and UBA1819 were significantly associated with 33, 15, 17, and 10 plasma metabolites, respectively. In addition, Fournierella was positively correlated with PC [18:3(6Z,9Z,12Z)/18:1(11Z)] and an unknown metabolite. Subdoligranulum was negatively correlated with aminomalote and positively correlated with LysoPC [20:4(8Z,11Z,14Z,17Z)]. Tyzzerella and Enterococcus were positively correlated with 3 metabolites and negatively correlated with 1 metabolite, respectively. The details of these significant microbiota-metabolite correlations were shown in Supplementary Table 4. Furthermore, the Ruminococcus_2 node was the largest in fecal flora and the dominant bacteria in the network diagram. There were 33 metabolites with significant positive correlation with Ruminococcus_2, including saccharin, sulfamethazine and 2-carboxy-5,7-dimethyl-4-octanolide. In the significantly different metabolites, 2-carboxy-5,7-dimethyl-4-octanolide nodes were the largest in the network diagram, and with a positive correlation to Ruminococcus_2, Flavonifractor, Ruminiclostridium_9 and UBA1819 (Figure 5B). These data suggested that there is a significant taxonomic disturbance in the fecal microbiota in calves with DHD, which might lead to changes in metabolism.
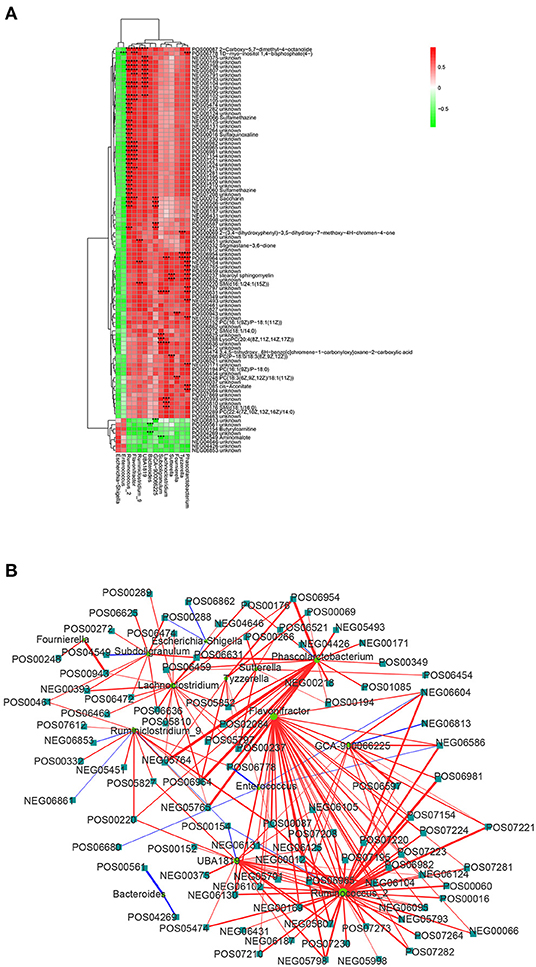
Figure 5. Relationship between fecal microorganisms and plasma metabolites. (A) Heat map summarizing the correlation between significantly different fecal microbiota and significantly altered metabolites in plasma (red, positive correlation; green, negative correlation). ***Indicates the significant microbiota-metabolite correlations (|cor| > 0.75 and P < 0.01). (B) Network diagram of significant microbiota-metabolite correlations. The circle represents the altered bacteria, and the rectangle represents the altered metabolites.
Discussion
Calf diarrhea is a growing concern worldwide because it has caused serious economic loss in cattle industry. Although DHD is a common type of diarrhea in calves, the mechanisms of this disease have not been fully elucidated. Previous studies revealed that intestinal microflora play a key role in maintaining metabolic balance (40). Most of metabolites in the blood were used to as biomarkers for diarrhea-related diseases (41). Thus, to study the fecal microbiota composition and plasma metabolomics characteristics of DHD calves may help to understand the pathogenesis of DHD and develop appropriate intervention strategies. This study is the first to characterize the fecal microbiome and plasma metabolites in calves with DHD by integrating 16S rDNA gene sequencing and LC–MS-based metabolomics approaches. The results revealed that the intestinal microbiota composition and plasma metabolic phenotype of DHD calves were significantly different from those of healthy calves.
As a complex ecosystem of the body, the intestinal microbiota is maintained a dynamic balance in healthy host. Many of disease were due to the disturbance of the intestinal microbiota (42, 43). In present study, 16S rDNA gene sequencing was performed to investigate the alteration of fecal microbiota in DHD. There were significant differences in the species abundance and composition of fecal microbes between DHD calves and healthy calves, which suggested that DHD may be related to the significant changes of the intestinal microbiota composition. Compared with healthy calves, the proportion of Bacteroidetes in calves with DHD was significantly down-regulated, but Proteobacteria was significantly up-regulated. Proteobacteria is the most abundant bacterial phylum in the intestinal microbiota of DHD calves, and it can produce lipopolysaccharide (LPS), which in turn induces inflammation (44). The ratio of Firmicutes to Bacteroidetes is widely accepted to play an important role in maintaining normal intestinal homeostasis, and the dysbiosis of this ratio can lead to some diseases (45, 46). In some diarrhea-related diseases, Bacteroidetes were lower, and Firmicutes were higher (47, 48). The Firmicutes/Bacteroidetes ratio was also significantly increased in calves with DHD, which reflected the disorder of the gut microbiota. At the genus level, Escherichia-Shigella and Enterococcus were remarkably higher in DHD calves. Escherichia-Shigella was the leading agent in the causes of diarrhea (49, 50). Increased Enterococcus has also been associated with some diarrhea-related diseases (51). We also detected a significant decrease in Bacteroides, Subdoligranulum, Ruminococcus_2, and Sutterella in fecal samples. Bacteroides have been found to be negatively correlated with colonic proinflammatory cytokines such as IL-6, IL-1β, and TNF-α (52), and Sutterella was negatively associated with inflammation (53). Thus, we speculated that an increased inflammatory response occurred in calves with DHD. Lachnoclostridium is associated with the production of short-chain fatty acids in the gut (54), and its significant decrease in DHD calves may be related to the significant changes in unsaturated fatty acid metabolism pathways. Subdoligranulum has also been found to be significantly decreased in a lot of intestinal diseases (55, 56). In this study, the correlation network analysis showed that DHD was associated with changes in the relevant structure and possible interaction structure of the intestinal microbiota. A similar disruption of the intestinal microbiota interaction network has been observed in other diseases presenting with diarrhea (57, 58). However, further studies are needed to determine the real role of intestinal microbiota interaction networks in the development of DHD.
Plasma metabolic profiles of DHD and healthy calves were significantly different. A total of 194 metabolites in plasma were identified as significantly different between the two groups (VIP > 2 and P < 0.05), which could serve as potential biomarkers of DHD in calves. Meanwhile, eight metabolic pathways were found to be associated with these differential metabolites, including pathways of glycerophospholipids, arachidonic acid, linoleic acid, and alpha-linolenic acid. Some of these metabolic pathways have also been shown to be the major disordered pathways in serum of animals with DHD (9, 59). Glycerophospholipids, the major components of cell membranes, alleviated diarrhea-related diseases (49, 60). Arachidonic acid, an important unsaturated fatty acid, is associated with many diseases including DHD (9, 61, 62). Linoleic acid is a necessary fatty acid that has been reported to be helpful in relieving diarrhea (63). It was reported that the increasing intake of essential fatty acids such as linoleic acid and alpha-linolenic acid improved immune status (64). Although some metabolites may be related to the pathogenesis of DHD, these metabolites still need to be quantitatively analyzed through targeted metabolomics, and the role of these metabolites in DHD pathogenesis should also be determined in future study.
Recently, a large number of studies have shown that metabolic changes and intestinal microbiota disorders were parallel during the disease progression (65, 66). Dysbiosis of the gut microbiota was accompanied with the alteration of plasma metabolome in calves with DHD. Spearman correlation analysis was performed to explore the correlation between the changes in fecal microorganisms and the plasma metabolites, and the results suggested that intestinal flora disturbance is related to metabolic phenotypic changes. Escherichia-Shigella was significantly correlated with metabolites involved in metabolism of arachidonic acid and alpha-linolenic acid, such as phosphatidylcholine and lecithin. Fournierella and Ruminiclostridium_9 were significantly correlated with L-glutamate and sphingomyelin which are related to arginine, and proline metabolism, and the sphingolipid signaling pathway. These metabolites can also be produced by gut microbiota. In diarrhea cases, the disturbances in the metabolism of amino acids and glucose, and disorders of intestinal microecology were often observed (16, 67, 68). These studies also implied that the fecal microbes in DHD calves are closely related to the metabolic phenotype of the host.
Our results should be considered in the context of several constraints. First, the animal sample sizes were small, and larger cohorts need to be evaluated in future studies. Second, we did not verify the biomarkers obtained by other methods, and future studies should assess longitudinal microbiota before and during the onset of DHD, and expand analyses such as host responses and transcriptomics to more fully understand the pathogenesis of DHD, with a view of identifying targets for drug development.
Conclusion
DHD disturbed the composition of fecal microbiota and plasma metabolites. The changes in the abundance of fecal microorganisms, especially Escherichia-Shigella, Fournierella, and Ruminococcus_2, and the concentrations of plasma metabolites, especially lecithin, phosphatidylcholine, and choline phosphatid, might affect the progression of DHD by interfering with metabolism of arachidonic acid, alpha-linolenic acid, linoleic acid, and glycerophospholipids. These significantly altered metabolic pathways and microorganisms may serve as diagnostic markers and potential therapeutic targets for DHD in calves. This finding provided a new insight for further investigating the mechanism of this kind of disease in calves.
Data Availability Statement
The datasets presented in this study can be found in online repositories. The names of the repository/repositories and accession number(s) can be found in the article/Supplementary Material.
Ethics Statement
The animal study was reviewed and approved by Laboratory Animal Ethics Commission of Lanzhou Institute of Husbandry and Pharmaceutical Sciences, CAAS.
Author Contributions
JL and XS conceived and designed the work. JL coordinated technical support and funding. ZY wrote the manuscript. ZY, KanZ, KaiZ, GW, LW, JZ, ZQ, and ZG performed the experiments and collected the samples. JL and KaiZ reviewed the manuscript. All authors have read and approved to the published version of the manuscript.
Funding
This work was financially supported by China Agriculture Research System of MOF and MARA, the National key research and development program of China (2017YFE0114400 and 2018YFD0502403), and special Fund of Chinese Central Government for Basic Scientific Research Operations in Commonweal Research Institutes (1610032021002).
Conflict of Interest
The authors declare that the research was conducted in the absence of any commercial or financial relationships that could be construed as a potential conflict of interest.
Publisher's Note
All claims expressed in this article are solely those of the authors and do not necessarily represent those of their affiliated organizations, or those of the publisher, the editors and the reviewers. Any product that may be evaluated in this article, or claim that may be made by its manufacturer, is not guaranteed or endorsed by the publisher.
Acknowledgments
Sincerely thanks to other colleagues in the lab for their help in clinical trial. We are also grateful to Guangzhou Genedenovo Biotechnology Co., Ltd for assisting in MS analysis and bioinformatics analysis.
Supplementary Material
The Supplementary Material for this article can be found online at: https://www.frontiersin.org/articles/10.3389/fvets.2022.703051/full#supplementary-material
References
1. Meganck V, Hoflack G, Piepers S, Opsomer G. Evaluation of a protocol to reduce the incidence of neonatal calf diarrhoea on dairy herds. Prev Vet Med. (2015) 118:64–70. doi: 10.1016/j.prevetmed.2014.11.007
2. Torsein M, Lindberg A, Sandgren CH, Waller KP, Törnquist M, Svensson C. Risk factors for calf mortality in large Swedish dairy herds. Prev Vet Med. (2011) 99:136–47. doi: 10.1016/j.prevetmed.2010.12.001
3. Al Mawly J, Grinberg A, Prattley D, Moffat J, Marshall J, French N. Risk factors for neonatal calf diarrhoea and enteropathogen shedding in New Zealand dairy farms. Vet J. (2015) 203:155–60. doi: 10.1016/j.tvjl.2015.01.010
4. Arsenopoulos K, Theodoridis A, Papadopoulos E. Effect of colostrum quantity and quality on neonatal calf diarrhoea due to Cryptosporidium spp. infection. Comp Immunol Microb. (2017) 53:50–5. doi: 10.1016/j.cimid
5. Cho Y-I, Han J-I, Wang C, Cooper V, Schwartz K, Engelken T, et al. Case–control study of microbiological etiology associated with calf diarrhea. Vet Microbiol. (2013) 166:375–85. doi: 10.1016/j.vetmic
6. Wittek T, Ernstberger M, Muckenhuber M, Flöck M. Effects of wheat protein in milk replacers on abomasal emptying rate in calves. J Anim Physiol Anim Nutr. (2016) 100:264–70. doi: 10.1111/jpn.12363
7. Klein-Jöbstl D, Iwersen M, Drillich M. Farm characteristics and calf management practices on dairy farms with and without diarrhea: a case-control study to investigate risk factors for calf diarrhea. J Dairy Sci. (2014) 97:5110–9. doi: 10.3168/jds.2013-7695
8. Zheng C, Pei T, Huang C, Chen X, Bai Y, Xue J, et al. A novel systems pharmacology platform to dissect action mechanisms of traditional Chinese medicines for bovine viral diarrhea disease. Eur J Pharm Sci. (2016) 94:33–45. doi: 10.1016/j.ejps
9. Hua Y, Ma Q, Zhang X-s, Jia Y, Peng X, Yao W, et al. Pulsatilla decoction can treat the dampness-heat diarrhea rat model by regulating glycerinphospholipid metabolism based lipidomics approach. Front Pharmacol. (2020) 11:197. doi: 10.3389/fphar
10. Xiong R, Li W, Li Y, Zheng K, Zhang T, Gao M, et al. Er Shen Wan extract reduces diarrhea and regulates AQP 4 and NHE 3 in a rat model of spleen-kidney Yang deficiency–induced diarrhea. Biomed Pharmacother. (2018) 98:834–46. doi: 10.1016/j.biopha.2018.01.023
11. Pan X, Xu K, Xiong R, Chen Z, Hu C, Feng J, et al. Therapeutic effect of ershen pill () extract on pi (spleen)-shen (kidney) yang deficiency-induced diarrhea in rat model. Chin J Integr Med. (2019) 25:536–42. doi: 10.1007/s11655-017-2766-4
12. Yao W, Yang C, Wen Y, Zhang W, Zhang X, Ma Q, et al. Treatment effects and mechanisms of Yujin Powder on rat model of large intestine dampness-heat syndrome. J Ethnopharmacol. (2017) 202:265–80. doi: 10.1016/j.jep.2017.03.030
13. Chen X, Wen Y, Wu Z, Zhang B, Hou Z, Xiao J, et al. Development of a traditional Chinese medicine syndrome-specific scale for ulcerative colitis: the large intestine dampness-heat syndrome questionnaire. Evid Based Complementary Altern Med. (2018) 2018:4039019. doi: 10.1155/2018/4039019
14. Peng W, Huang J, Yang J, Zhang Z, Yu R, Fayyaz S, et al. Integrated 16S rRNA sequencing, metagenomics, and metabolomics to characterize gut microbial composition, function, and fecal metabolic phenotype in non-obese type 2 diabetic Goto-Kakizaki rats. Front Microbiol. (2020) 10:3141. doi: 10.3389/fmicb.2019.03141
15. Lv W, Liu C, Ye C, Sun J, Tan X, Zhang C, et al. Structural modulation of gut microbiota during alleviation of antibiotic-associated diarrhea with herbal formula. Int J Biol Macromol. (2017) 105:1622–9. doi: 10.1016/j.ijbiomac.2017.02.060
16. Han Z, Li K, Shahzad M, Zhang H, Luo H, Qiu G, et al. Analysis of the intestinal microbial community in healthy and diarrheal perinatal yaks by high-throughput sequencing. Microb Pathogenesis. (2017) 111:60–70. doi: 10.1016/j.micpath.2017.08.025
17. Vitetta L, Vitetta G, Hall S. Immunological tolerance and function: associations between intestinal bacteria, probiotics, prebiotics, and phages. Front. Immunol. (2018) 9:2240. doi: 10.3389/fimmu.2018.02240
18. Hiergeist A, Reischl U, Gessner A. Multicenter quality assessment of 16S ribosomal DNA-sequencing for microbiome analyses reveals high inter-center variability. International J Med Microbiol. (2016) 306:334–42. doi: 10.1016/j.ijmm.2016.03.005
19. Laudadio I, Fulci V, Palone F, Stronati L, Cucchiara S, Carissimi C. Quantitative assessment of shotgun metagenomics and 16S rDNA amplicon sequencing in the study of human gut microbiome. OMICS. (2018) 22:248–54. doi: 10.1089/omi.2018.0013
20. Xu B, Yan Y, Huang J, Yin B, Pan Y, Ma L. Cortex Phellodendri extract's anti-diarrhea effect in mice related to its modification of gut microbiota. Biomed Pharmacother. (2020) 123:109720. doi: 10.1016/j.biopha.2019.109720
21. Wang A, Ling Z, Yang Z, Kiela PR, Wang T, Wang C, et al. Gut microbial dysbiosis may predict diarrhea and fatigue in patients undergoing pelvic cancer radiotherapy: a pilot study. PLoS ONE. (2015) 10:e0126312. doi: 10.1371/journal.pone.0126312
22. Li X, Zhang C, Hui H, Tan Z. Effect of Gegenqinlian decoction on intestinal mucosal flora in mice with diarrhea induced by high temperature and humidity treatment. 3 Biotech. (2021) 11:1–14. doi: 10.1007/s13205-020-02628-0
23. Long NP, Yoon SJ, Anh NH, Nghi TD, Lim DK, Hong YJ, et al. A systematic review on metabolomics-based diagnostic biomarker discovery and validation in pancreatic cancer. Metabolomics. (2018) 14:1–26. doi: 10.1007/s11306-018-1404-2
24. Ke C, Pan C-W, Zhang Y, Zhu X, Zhang Y. Metabolomics facilitates the discovery of metabolic biomarkers and pathways for ischemic stroke: a systematic review. Metabolomics. (2019) 15:1–21. doi: 10.1007/s11306-019-1615-1
25. Iida M, Harada S, Takebayashi T. Application of metabolomics to epidemiological studies of atherosclerosis and cardiovascular disease. J Atheroscler Thromb. (2019) 2019:RV17036. doi: 10.5551/jat.RV17036
26. Liggi S, Griffin JL. Metabolomics applied to diabetes– lessons from human population studies. Int J Biochem Cell B. (2017) 93:136–47. doi: 10.1016/j.biocel.2017.10.011
27. Fearnley LG, Inouye M. Metabolomics in epidemiology: from metabolite concentrations to integrative reaction networks. Int J Epidemiol. (2016) 45:1319–28. doi: 10.1093/ije/dyw046
28. Noorbakhsh H, Yavarmanesh M, Mortazavi SA, Adibi P, Moazzami AA. Metabolomics analysis revealed metabolic changes in patients with diarrhea-predominant irritable bowel syndrome and metabolic responses to a synbiotic yogurt intervention. Eur J Nutr. (2019) 58:3109–19. doi: 10.1007/s00394-018-1855-2
29. Wang ZZ, Cui BB, Zhang F, Yang Y. Development of a correlative strategy to discover colorectal tumor tissue derived metabolite biomarkers in plasma using untargeted metabolomics. Anal Chem. (2019) 91:8b05177. doi: 10.1021/acs.analchem.8b05177
30. Zhang B, Qi X, Cai Q. Metabolomic study of raw and bran-fried Atractylodis Rhizoma on rats with spleen deficiency. J Pharmaceut Biomed. (2020) 182:112927. doi: 10.1016/j.jpba.2019.112927
31. Guo M, Wu F, Hao G, Qi Q, Li R, Li N, et al. Bacillus subtilis improves immunity and disease resistance in rabbits. Front Immunol. (2017) 8:354. doi: 10.3389/fimmu.2017.00354
32. Edgar RC. UPARSE: highly accurate OTU sequences from microbial amplicon reads. Nat Methods. (2013) 10:996–8. doi: 10.1038/nmeth.2604
33. Pruesse E, Quast C, Knittel K, Fuchs BM, Ludwig W, Peplies J, et al. SILVA: a comprehensive online resource for quality checked and aligned ribosomal RNA sequence data compatible with ARB. Nucleic Acids Res. (2007) 35:7188–96. doi: 10.1093/nar/gkm864
34. Wang Q, Garrity GM, Tiedje JM, Cole JR. Naive Bayesian classifier for rapid assignment of rRNA sequences into the new bacterial taxonomy. Appl Environ Microbiol. (2007) 73:5261–7. doi: 10.1128/aem.00062-07
35. Ondov BD, Bergman NH, Phillippy AM. Interactive metagenomic visualization in a Web browser. BMC Bioinformatics. (2011) 12:385. doi: 10.1186/1471-2105-12-385
36. Saccenti E, Hoefsloot HC, Smilde AK, Westerhuis JA, Hendriks MM. Reflections on univariate and multivariate analysis of metabolomics data. Metabolomics. (2014) 10:361–74. doi: 10.1007/s11306-013-0598-6
37. El Bouhaddani S, Uh H-W, Jongbloed G, Hayward C, Klarić L, Kiełbasa SM, et al. Integrating omics datasets with the OmicsPLS package. BMC bioinformatics. (2018) 19:371. doi: 10.1186/s12859-018-2371-3
38. Xiao Y, Xiong T, Peng Z, Liu C, Huang T, Yu H, et al. Correlation between microbiota and flavours in fermentation of Chinese Sichuan Paocai. Food Res Int. (2018) 114:123–32. doi: 10.1016/j.foodres.2018.06.051
39. Shankar V, Homer D, Rigsbee L, Khamis HJ, Michail S, Raymer M, et al. The networks of human gut microbe–metabolite associations are different between health and irritable bowel syndrome. ISME J. (2015) 9:1899–903. doi: 10.1038/ismej.2014.258
40. Wu Z, Chen Y, Zhu D, Zheng Y, Ali KB, Hou K. Advancement of traditional Chinese medicine in regulation of intestinal flora: mechanism-based role in disease management. Recent Pat Anticancer. (2021). doi: 10.2174/1574892816666210929164930. [Epub ahead of print].
41. Beinvogl BC, Manini ML, Camilleri M, Donato LJ, Harmsen WS, Absah I, et al. Markers of bile acid metabolism in pediatric diarrhea predominant irritable bowel syndrome and healthy controls. J Pediatr Gastr Nutr. (2021) 72:859–65. doi: 10.1097/mpg.0000000000003067
42. Meng X, Zhang G, Cao H, Yu D, Fang X, de Vos WM, et al. Gut dysbacteriosis and intestinal disease: mechanism and treatment. J Appl Microbiol. (2020) 129:787–805. doi: 10.1111/jam.14661
43. Zhao E, Zhang W, Geng B, You B, Wang W, Li X. Intestinal dysbacteriosis leads to kidney stone disease. Mol Med Rep. (2021) 23:1–3. doi: 10.3892/mmr.2020.11819
44. Mukhopadhya I, Hansen R, El-Omar EM, Hold GL. IBD—what role do Proteobacteria play? Nat Rev Gastro Hepat. (2012) 9:219–30. doi: 10.1038/nrgastro.2012.14
45. Cui C, Shen C, Jia G, Wang K. Effect of dietary Bacillus subtilis on proportion of Bacteroidetes and Firmicutes in swine intestine and lipid metabolism. Genet Mol Res. (2013) 12:1766–76. doi: 10.4238/2013.May.23.1
46. Stojanov S, Berlec A, Štrukelj B. The influence of probiotics on the Firmicutes/Bacteroidetes ratio in the treatment of obesity and inflammatory bowel disease. Microorganisms. (2020) 8:1715. doi: 10.3390/microorganisms8111715
47. Wang J, Feng W, Zhang S, Chen L, Sheng Y, Tang F, et al. Ameliorative effect of Atractylodes macrocephala essential oil combined with Panax ginseng total saponins on 5-fluorouracil induced diarrhea is associated with gut microbial modulation. J Ethnopharmacol. (2019) 238:111887. doi: 10.1016/j.jep.2019.111887
48. Liu T, Xiong Q, Li L, Hu Y. Intestinal microbiota predicts lung cancer patients at risk of immune-related diarrhea. Immunotherapy. (2019) 11:385–96. doi: 10.2217/imt-2018-0144
49. Akashi T, Muto A, Takahashi Y, Nishiyama H. Enteral formula containing egg yolk lecithin improves diarrhea. J Oleo Sci. (2017) 2017:ess17007. doi: 10.5650/jos.ess17007
50. Qu M, Deng Y, Zhang X, Liu G, Huang Y, Lin C, et al. Etiology of acute diarrhea due to enteropathogenic bacteria in Beijing, China. J Infect. (2012) 65:214–222. doi: 10.1016/j.jinf.2012.04.010
51. Zhou Y, Chen H, He H, Du Y, Hu J, Li Y, et al. Increased Enterococcus faecalis infection is associated with clinically active Crohn disease. Medicine. (2016) 95:e5019. doi: 10.1097/md.0000000000005019
52. Sánchez E, Laparra JM, Sanz Y. Discerning the role of Bacteroides fragilis in celiac disease pathogenesis. Appl Environ Microbiol. (2012) 78:6507–15. doi: 10.1128/aem.00563-12
53. Morgan XC, Kabakchiev B, Waldron L, Tyler AD, Tickle TL, Milgrom R, et al. Associations between host gene expression, the mucosal microbiome, and clinical outcome in the pelvic pouch of patients with inflammatory bowel disease. Genome Biol. (2015) 16:67. doi: 10.1186/s13059-015-0637-x
54. Chen L, Zhou X, Wang Y, Wang D, Ke Y, Zeng X. Propionate and butyrate produced by gut microbiota after probiotic supplementation attenuate lung metastasis of melanoma cells in mice. Mol Nutr Food Res. (2021) 65:e2100096. doi: 10.1002/mnfr.202100096
55. Jang JY, Kim S, Kwon MS, Lee J, Yu DH, Song RH, et al. Rotavirus-mediated alteration of gut microbiota and its correlation with physiological characteristics in neonatal calves. J Microbiol. (2019) 57:113–21. doi: 10.1007/s12275-019-8549-1
56. Xia Y, Wang J, Fang X, Dou T, Han L, Yang C. Combined analysis of metagenomic data revealed consistent changes of gut microbiome structure and function in inflammatory bowel disease. J Appl Microbiol. (2021) 131:3018–31. doi: 10.1111/jam.15154
57. Han C, Dai Y, Liu B, Wang L, Wang J, Zhang J. Diversity analysis of intestinal microflora between healthy and diarrheal neonatal piglets from the same litter in different regions. Anaerobe. (2019) 55:136–41. doi: 10.1016/j.anaerobe.2018.12.001
58. Molist F, Manzanilla EG, Francisco Perez J, Nyachoti CM. Coarse, but not finely ground, dietary fibre increases intestinal Firmicutes:Bacteroidetes ratio and reduces diarrhoea induced by experimental infection in piglets. Br J Nutr. (2012) 108:9–15. doi: 10.1017/s0007114511005216
59. Hua Y-l, Ma Q, Li W, Zhang X-s, Cheng X-h, Jia Y-q, et al. Metabolomics analysis of Pulsatilla decoction on treatment of wetness-heat-induced diarrhea in rats based on UPLC-Q/TOF-MS/MS. Biomed Chromatogr. (2019) 33:e4629. doi: 10.1002/bmc.4629
60. Kalenyak K, Heilmann RM, van de Lest CHA, Brouwers JF, Burgener IA. Comparison of the systemic phospholipid profile in dogs diagnosed with idiopathic inflammatory bowel disease or food-responsive diarrhea before and after treatment. PLoS ONE. (2019) 14:e0215435. doi: 10.1371/journal.pone.0215435
61. Naito Y, Ji X, Tachibana S, Aoki S, Furuya M, Tazura Y, et al. Effects of arachidonic acid intake on inflammatory reactions in dextran sodium sulphate-induced colitis in rats. Br J Nutr. (2015) 114:734–45. doi: 10.1017/s000711451500224x
62. Burns JL, Nakamura MT, Ma DWL. Differentiating the biological effects of linoleic acid from arachidonic acid in health and disease. Prostag Leukotr Ess. (2018) 135:1–4. doi: 10.1016/j.plefa.2018.05.004
63. Faramarzi E, Mandavi R, Mohammad-Zadeh M, Nasirimotlagh B, Sanaie S. Effect of conjugated linoleic acid supplementation on quality of life in rectal cancer patients undergoing preoperative chemoradiotherapy. Pak J Med Sci. (2017) 33:383–8. doi: 10.12669/pjms.332.11925
64. Garcia M, Shin JH, Schlaefli A, Greco LF, Maunsell FP, Thatcher WW, et al. Increasing intake of essential fatty acids from milk replacer benefits performance, immune responses, and health of preweaned Holstein calves. J Dairy Sci. (2015) 98:458–77. doi: 10.3168/jds.2014-8384
65. An G, Zhang Y, Fan L, Chen J, Wei M, Li C, et al. Integrative analysis of vaginal microorganisms and serum metabolomics in rats with estrous cycle disorder induced by long-term heat exposure based on 16S rDNA gene sequencing and LC/MS-based metabolomics. Front Cell Infect Microbiol. (2021) 11:595716. doi: 10.3389/fcimb.2021.595716
66. Vitali B, Cruciani F, Picone G, Parolin C, Donders G, Laghi L. Vaginal microbiome and metabolome highlight specific signatures of bacterial vaginosis. Eur J Clin Micobiol. (2015) 34:2367–76. doi: 10.1007/s10096-015-2490-y
67. Xu S, Wang S. GC-MS and metabolomics analysis of amino acids, glucose and urinary metabolic pathways and characteristics in children with spleen-deficiency diarrhea. Cell Mol Biol. (2020) 66:125–30. doi: 10.14715/cmb/2020.66.5.22
Keywords: dampness-heat diarrhea, gut microbiome, metabolomics, 16S rDNA, pathogenesis
Citation: Yan Z, Zhang K, Zhang K, Wang G, Wang L, Zhang J, Qiu Z, Guo Z, Song X and Li J (2022) Integrated 16S rDNA Gene Sequencing and Untargeted Metabolomics Analyses to Investigate the Gut Microbial Composition and Plasma Metabolic Phenotype in Calves With Dampness-Heat Diarrhea. Front. Vet. Sci. 9:703051. doi: 10.3389/fvets.2022.703051
Received: 14 September 2021; Accepted: 10 January 2022;
Published: 15 February 2022.
Edited by:
Michael D. Flythe, Agricultural Research Service, United States Department of Agriculture (USDA), United StatesReviewed by:
Penka Mladenova Petrova, Bulgarian Academy of Sciences, BulgariaPatricia Louvandini, University of São Paulo, Brazil
Copyright © 2022 Yan, Zhang, Zhang, Wang, Wang, Zhang, Qiu, Guo, Song and Li. This is an open-access article distributed under the terms of the Creative Commons Attribution License (CC BY). The use, distribution or reproduction in other forums is permitted, provided the original author(s) and the copyright owner(s) are credited and that the original publication in this journal is cited, in accordance with accepted academic practice. No use, distribution or reproduction is permitted which does not comply with these terms.
*Correspondence: Jianxi Li, bHpqaWFueGlsJiN4MDAwNDA7MTYzLmNvbQ==; Xiaoping Song, c3hwdGN2bSYjeDAwMDQwOzE2My5jb20=