- 1School of Public Health, Lanzhou University, Lanzhou, China
- 2Key Laboratory of Restoration Ecology for Cold Regions in Qinghai, Xining, China
- 3Department of Applied Biology, College of Biotechnology and Bioengineering, Zhejiang University of Technology, Hangzhou, China
- 4Key Laboratory of Health Aquaculture and Product Processing in Dongting Lake Area of Hunan Province, Zoology Key Laboratory of Hunan Higher Education, Hunan University of Arts and Science, Changde, China
- 5Central South Inventory and Planning Institute of National Forestry and Grassland Administration, Changsha, China
- 6Key Laboratory of Adaptation and Evolution of Plateau Biota, Northwest Institute of Plateau Biology, Chinese Academy of Sciences, Xining, China
The rumen microbiota plays an important role in animal functional attributes. These microbes are indispensable for the normal physiological development of the rumen, and may also convert the plant polysaccharides from grass into available milk and meat, making it highly valuable to humans. Exploring the microbial composition and metabolites of rumen across developmental stages is important for understanding ruminant nutrition and metabolism. However, relatively few reports have investigated the microbiome and metabolites across developmental stages in ruminants. Using 16S rRNA gene sequnecing, metabolomics and high-performance liquid chromatography techniques, we compared the rumen microbiota, metabolites and short chain fatty acids (SCFAs) between lambs and sub-adult Tibetan sheep (Ovis aries) from Qinghai-Tibetan Plateau. Bacteroidetes and Spirochaetae were enriched in sub-adult sheep, while Firmicutes and Tenericutes were more abundant in young individuals. The sub-adult individuals had higher alpha diversity values than those in young sheep. Metabolomics analysis showed that the content of essential amino acids and related gene functional pathways in rumen were different between the lambs and sub-adult population. L-Leucine that participates in valine, leucine and isoleucine biosynthesis was more abundant in the lambs, while phenylethylamine that takes part in phenylalanine metabolism was more enriched in the sub-adults. Both rumen microbial community structures and metabolite profiles were impacted by age, but rumen SCFA concentration was relatively stable between different age stages. Some specific microbes (e.g., Clostridium and Ruminococcaceae) were positively associated with L-Leucine but negatively correlated with phenylethylamine, implying that rumen microbes may play different roles for metabolite production at different ages. Mantel test analysis showed that rumen microbiota was significantly correlated with metabolomics and SCFA profiles. Our results indicates the close relationship between microbial composition and metabolites, and also reveal different nutritional requirement for different ages in ruminants, thus having important significance for regulating animal nutrition and metabolism by microbiome intervention.
Introduction
Ruminants have a close relationship with their symbiotic microorganisms in the digestive tract. These microbes include bacteria, archaea, fungi, viruses, and protozoa (1), which provides many ecosystem functional services on hosts. A typical example is the rumen of ruminants, and the microbial communities in this organ are able to convert those indigestible structural plant polysaccharides from grass into available compounds for animals to help hosts acquire enough energy and promote animal growth (2). This ecological process is very important to human beings because it can convert solar energy stored in plant materials into available food, such as meat and milk. Thus, understanding the composition and function of rumen microbiome is pivotal for improving the productivity of ruminant digestive system.
During the infancy of ruminants, the rumen is not yet developed and lack fermentation function for grass when animals are still suckling milk. One important reason is that the rumen wall papillae, as a region for nutritional absorption, is not mature (3). Some reports have revealed the rapid colonization of aerobic and facultative anaerobic microorganisms at birth (4). With the maturation of animals, foreign anaerobic microorganisms from animal's parents and their surrounding environment gradually replace those aerobic and facultative anaerobic taxa and keep a constant level at between 6 and 8 weeks of age (5). For example, the anaerobic cellulolytic bacteria (Ruminococcus and Fibrobacter) in the bovine had a low abundance in the newborn (1–3 days) individuals, while had higher abundance in the adult sheep and maintained a relatively constant level (5). When animal reaches sub-adult or adulthood stages, these rumen microbes ferment food, especially those indigestible plant cellulose to produce short chain fatty acids (SCFAs), microbial cell protein, ammonia and other unknown metabolites (1). Although rumen bacterial communities have been investigated from birth to adulthood, there is still lack of information on microbial functional taxa and metabolites. More importantly, the relationship between rumen microbiota and metabolome remains largely unknown.
Metabolomics is one of latest popular “omics” science field based on high-throughput methods, such as liquid chromatography and mass spectrometry (LC/MS), which can extract many metabolites from samples and uncover the metabolic phenotypes in humans (6), animals (7), plants (8), and microbes (9). Metabolomics has opened new insights in animal nutrition research, and has revealed some metabolic pathways and metabolic biomarkers in ruminants in response to food and environmental stress. For example, the goats with high-grain feeding had increased levels of several toxic and inflammatory compounds, such as tryptamine, endotoxin, tyramine, and histamine (7). In addition, LC-MS analysis unraveled that 13 potential metabolic biomarkers associated with carbohydrate, amino acid and lipid metabolism were enriched in dairy cows under controlled heat stress, indicating the metabolic effects of environmental stress on ruminants (10). However, relative few reports have described metabolomics features and related metabolic pathways under different age stages in ruminants.
Tibetan sheep (Ovis aries) are one of the most widely distributed and numerous livestock on the Qinghai-Tibetan Plateau, as their population size reaches more than 50 million (11). They provide abundant milk, meat and income for local Tibetan herdsman by converting the plant polysaccharides into available products. Several reports have uncovered the microbiota composition and gene functions in the digestive tract of ruminants on the Qinghai-Tibetan Plateau (12), but there is still paucity of information on the rumen microbiome and metabolites in the Tibetan sheep. In this study, we performed microbiome, metabolome and short chain fatty acid (SCFA) analysis for rumen contents in lambs and sub-adult Tibetan sheep. We mainly addressed the following questions. (1) Whether the microbiomes of Tibetan sheep were different between the young and sub-adult Tibetan sheep? (2) Which rumen metabolites (including SCFAs) and functional pathways were associated with age? (3) Was there a correlation between microbiome and metabolome or SCFAs?
Materials and Methods
Animal Feeding and Sampling
Rumen contents were collected between February and July from the Haibei Demonstration Zone of Plateau Modern Ecological Animal Husbandry (100.9518°E, 36.9181°N). After the sheep were anesthetized using diethylether and dissected, we obtained a total of 12 rumen samples from 1-month-old (the lamb group, abbreviated as ES; N = 6) and 6-month-old Tibetan sheep (sub-adult group, abbreviated as SS; N = 6) in the same cohort. Three portions (~5 g per portion) of the contents from the anterior, middle and posterior parts of the rumen were taken and mixed well-before sample collection. These Tibetan sheep were all males. The sub-adult individuals were grazed on pasture (The main grass included Kobresia humilis, Oxytropis ochrocephala, Poa sp.) on the Qinghai-Tibet Plateau, and supplied commercial feed#8876 (Yongxing Ecological Agriculture and Animal Husbandry Development Co., Ltd. in Mengyuan County) at dusk. The main nutritional components of this commercial feed include crude protein ≥ 16%, crude fat ≥ 3%, crude fiber ≤ 8.0%, and crude ash ≤ 9.0%. The daily feed intake of sub-adult individuals was 4.75 ± 0.32 kg. The lamb individuals were mainly fed milk, and also ate a small amount of the grass (~0.72 ± 0.0.07 kg) and commercial feed (~0.54 ± 0.0.12 kg) mentioned above. Drinking water was freely available to these Tibetan sheep. The body weight of lamb and sub-adult groups was 15.75 ± 5.90 and 26.35 ± 4.01 kg, respectively. After collection, the ruminal contents were immediately divided into three parts on ice for the following microbiome, metabolome and short chain fatty acid (SCFA) analysis, and were temporarily kept in −20°C portable refrigerator in field. Finally, all samples were transferred to our lab within 24 h and stored at −40°C refrigerator.
The experimental protocols in current study were allowed by the Animal Welfare and Ethics Committee of Lanzhou University. The related experimental methods and procedures strictly followed the guidelines of the aforementioned institutions.
Microbiome Sequencing and Bioinformatic Analysis
The Soil Ezup DNA extraction kit (Sangon Biotech, China) was applied to extract total microbial DNA from the ruminal contents according to the product manual. Thereafter, Qubit 2.0 Fluorometer (Invitrogen, Carlsbad, CA) was used to detect the concentration of DNA samples. The V3-V4 regions of 16S rRNA gene were amplified using the primer pairs 341F(CCTACGGRRBGCASCAGKVRVGAAT) and 806R (GGACTACNVGGGTWTCTAATCC) (13). PCR amplification was performed in duplicate. The detailed procedures of PCR amplification and MiSeq (Illumina, San Diego, CA, USA) sequencing have been recorded previously (13).
Microbiome data analysis was mainly performed using QIIME Pipeline-Version 1.9.0 (http://qiime.org/scripts/index.html) from our Microbiome and Bioinformatics Platform in School of Public Health, Lanzhou University. The detailed analysis methods and procedures were described in our previous reports with slight modification (14–17). In brief, the original paired-end reads produced by MiSeq sequencer were assembled using the FLASH-1.2.8 software (18). Those sequences containing N, with base length < 200 bp, or average sequencing quality score < 30 were filtered. After removing chimeras, the remaining sequences were clustered into operational taxonomic units (OTUs) using VSEARCH-1.9.6 at 97% sequence similarity (19). Those OTUs with only one sequence across all samples were excluded. The longest reads for each OTU was picked as the representative sequence. These representative reads were further aligned against the Silva 123 database using PyNAST (20). Taxonomic arrangement of these representative OTUs was based on the Ribosomal Database Project (RDP) classifier with 80% threshold (21) at different categorical level. Those taxa that were not classified as “bacteria” were removed in OTU table.
To minimize the impact of sequencing depth for different samples, each sample was rarefied to 25,131 sequences. Alpha diversity values, including phylogenetic diversity and observed OTUs, were calculated. Beta diversity indices, including unweighted and weighted UniFrac distance matrices (both consider phylogentic relationship between microbes), were calculated using QIIME Pipeline. Unweighted UniFrac distance matrice is based on the absence or presence of OTUs, while weighted UniFrac is dependent on the relative abundance of OTUs (22). Principal coordinates analysis (PCoA) plots based on the above distance matrices were visualization using Origin 2018 (Originlab, Northampton, USA).
Metabolomic Measurement
One hundred mg ruminal contents were transferred into 5 mL centrifuge tubes, and then 500 μ L ddH2O (4°C) were added into the tubes. The mixture was thoroughly vortex-mixed for 60 s. Thereafter, 1,000 ul of methanol (pre-cooled at −20°C) was added into the samples, and the mixed liquids were shaken for 30 s. Thereafter, we further placed the tubes into an ultrasound machine at room temperature for 10 min, and then stew for 30 min on the ice. The samples were centrifuged for 10 min at 14, 000 rpm 4°C, and then 1.2 mL supernatant was transferred into a new centrifuge tube. Samples were further blow-dried by vacuum concentration. Thereafter, samples were dissolved using 400 μl methanol aqueous solution (1:1, 4°C), and underwent 0.22 μm membrane for filtration. For the quality control (QC) samples, 20 μL of prepared samples were extracted and mixed. These QC samples were used to monitor deviations of the analytical results from these pool mixtures. Finally, samples were ready for LC - MS (Waters, Milford, MA, USA) detection. More detailed methods for LC-MS procedures have been described in the previous report (23).
The original data obtained was converted into mzXML format using Proteowizard software (v3.0.8789). Then the metabolomic data underwent peaks identification, filtration and alignment using the XCMS package in R (v3.3.2). In order to compare data of different magnitude, peak area was normalized for further statistical analysis (24).
Short Chain Fatty Acid Measurement
Short chain fatty acid (SCFA) profiles of ruminal content samples were measured using an Agilent 1100 series high-performance liquid chromatography (HPLC) system (Agilent Technologies, Santa Clara, CA, USA). We measured the concentration of acetate, propionate, butyrate, isobutyrate, valerate, isovalerate, and hexanoate using an Alltech IOA-2000 organic acid column. The detailed procedures for measuring SCFAs have been described previously (25).
Statistical Analysis
To test the differences of the alpha diversity indices (phylogenetic diversity and observed OTUs) of ruminal microbiomes, Mann–Whitney U-tests were used. Permutational multivariate analysis of variance (PERMANOVA) analysis based on the unweighted and weighted UniFrac distance matrices was used to evaluate whether the community structures were significantly distinct between groups using the procedure “Adonis” in the R “vegan” package. In order to identify significant taxonomic differences of rumen microbiota between groups, we used LEfSe analysis at phylum, genus and OTU level. This method considers both biological and statistical significance of data. Linear discriminant analysis (LDA) effect size (LDA > 2) was applied to assess the magnitude scale of the effect of differentially abundant taxa. This analysis was performed at Galaxy module of LEfSe (http://huttenhower.sph.harvard.edu/galaxy/).
For metabolomic data, the detailed analysis methods were described in Li et al. (23). Briefly, the Soft Independent Modeling of the Class Analogy (SIMCA)-P (Version 11.0) was used to perform the principal component analysis (PCA) and partial least squares-discriminant analysis (PLS-DA). Those variables with VIP (Variable Importance in the Projection) > 1 that play major roles were chosen for the following analysis. One-way ANOVA was used to identify the significantly different metabolites (P < 0.05) between the groups ES and SS. The identification of these compounds was based on their MS/MS spectra, online databases and literature. Subsequently, the related metabolic pathways of the above different metabolites were analyzed based on Hypergeometric test using the online software MetaboAnalyst (www.metaboanalyst.ca). The impacting factor plots of KEEG (Kyoto Encyclopedia of Genes and Genomes) based on those different metabolites were visualized.
One-way analysis of variance (one-way ANOVA) with Tukey's post-hoc test in SPSS13.0 was used to analyze the differences of SCFA concentration of sheep ruminal contents between young and sub-adult groups. The spearman correlation analysis between rumen bacterial genera and SCFAs, differential metabolites was also performed using SPSS13.0. Heatmap2 was used to visualize the correlation results. In addition, mantel test was used to detect the relationship between rumen microbiota and metabolic profile or SCFAs using the “mantel” procedure in the R vegan package.
Nucleotide Sequence Accession Numbers
All the original 16S rRNA gene data in this study were submitted to the European Nucleotide Archive, and were available by accession NO. PRJEB36249 (http://www.ebi.ac.uk/ena/data/view/PRJEB36249).
Results
Overall Rumen Microbiota Composition
We obtained 698,333 original paired-end reads across all samples (Mean = 58,194, SD = 5,550) from MiSeq sequencer. A total of 800 unique OTUs were detected in the Tibetan sheep rumen across all samples using VSEARCH clustering. By taxonomic classification, we found that the rumen microbiota was dominated by Firmicutes (mean relative abundance = 56.57%), Bacteroidetes (39.46%), Proteobacteria (1.88%) and Verrucomicrobia (1.24%), followed by other rare phyla (mean relative abundance <1%), such as Spirochaetae, Actinobacteria, Tenericutes and Saccharibacteria. At genus level, five most abundant genera were Ruminococcaceae_UCG-005 (10.22%), Bacteroides (8.18%), Rikenellaceae_RC9_gut_group (7.58%), two unknown genus from Lachnospiraceae (7.42%) and Ruminococcaceae_UCG-010 (5.77%). The rumen microbial composition of each sample was visualized in Figure 1.
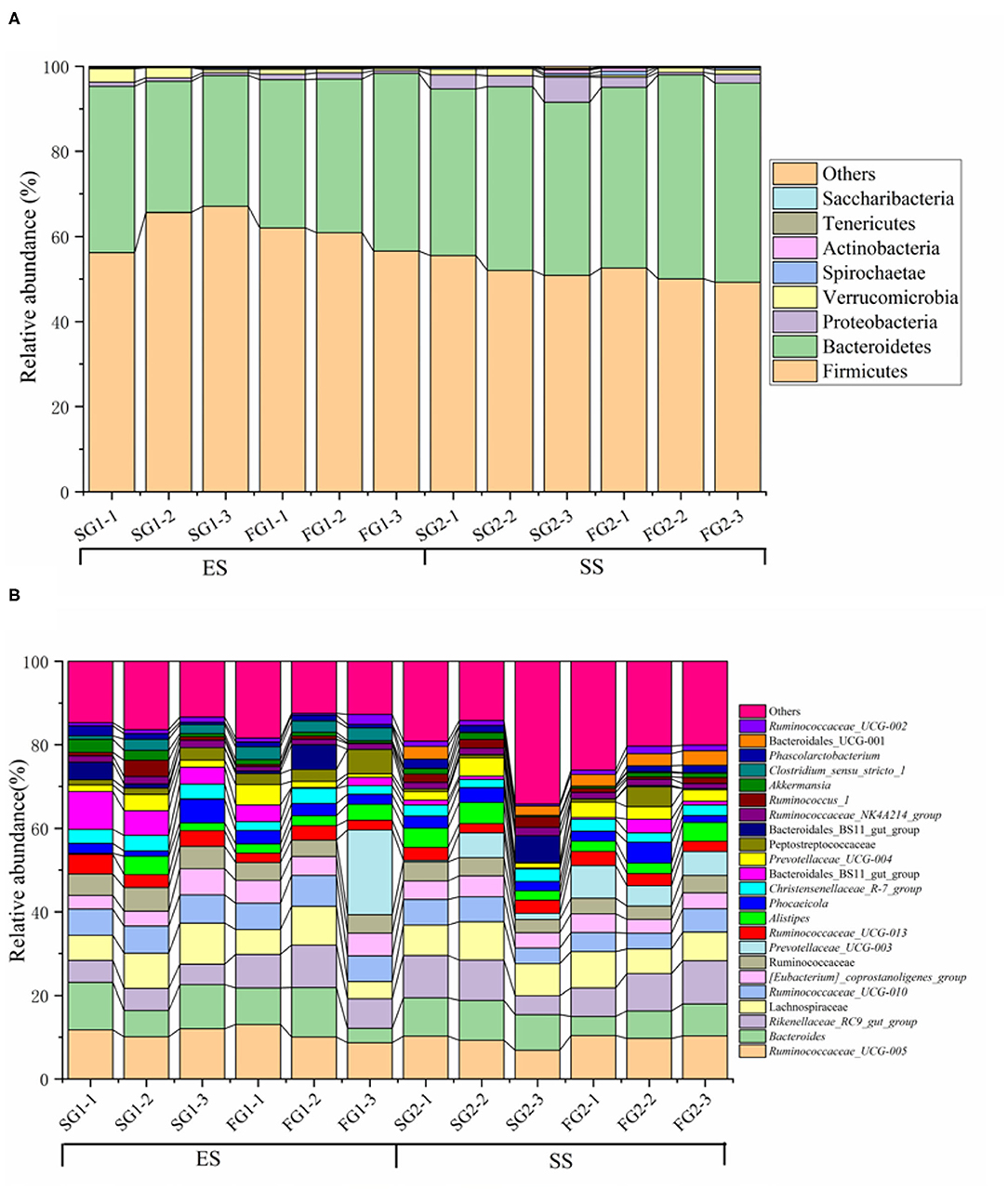
Figure 1. The rumen microbiome composition at phylum (A) and genus (B) level. Only those with mean relative abundance more than 0.1% (for phyla) or 1% (for genera) are shown.
The Difference of Rumen Microbial Composition and Diversity Between Young and Sub-adult Tibetan Sheep
Using LefSe analysis, we compared the significant different bacteria taxa between developmental stages of Tibetan sheep at phylum, genus and OTU level (Figure 2). We found that Bacteroidetes and Spirochaetae were more enriched in the sub-adult individuals, while Firmicutes and Tenericutes were more abundant in the young sheep. At genus level, a total of 40 genera were significantly different between the young and sub-adult sheep. Among these genera, the ES (or lamb) group showed a higher abundance for 18 genera, such as Clostridium_sensu_stricto_1, Turicibacter and Ruminococcaceae_UCG_010. The SS (or sub-adult) group was enriched by 22 genera, including Escherichia_Shigella, Prevotellaceae_UCG_001, Succinivibrio and Treponema_2. At OTU level, a total of 27 OTUs were more abundant in the ES group, these OTUs were mainly affiliated with Clostridium_sensu_stricto_1, Ruminococcaceae, Barnesiella, Akkermansia and Eubacterium. In contrast, 26 OTUs showed a higher abundance in the SS group, these OTUs mainly belonged to Lachnospiraceae, Succinivibrio, Rikenellaceae_RC9_gut_group, Ruminococcaceae, Eubacterium and Bacteroidales. In addition, we compared the alpha diversity values between the ES and SS groups. The rumen microbiota of sub-adult sheep had higher phylogenetic diversity and observed OTUs (both P < 0.05, Figure 3) compared with those in young individuals. For beta diversity, PCoA analysis showed that the young sheep had clear structural separation of microbial communities based on the unweighted or weighted UniFrac dissimilarities (Figure 4). PERMANOVA analysis confirmed that these two groups had significantly different community structures (R 2 = 0.294, P = 0.002 for unweighed UniFrac dissimilarity; R2 = 0.312, P = 0.008 for weighed UniFrac dissimilarity).
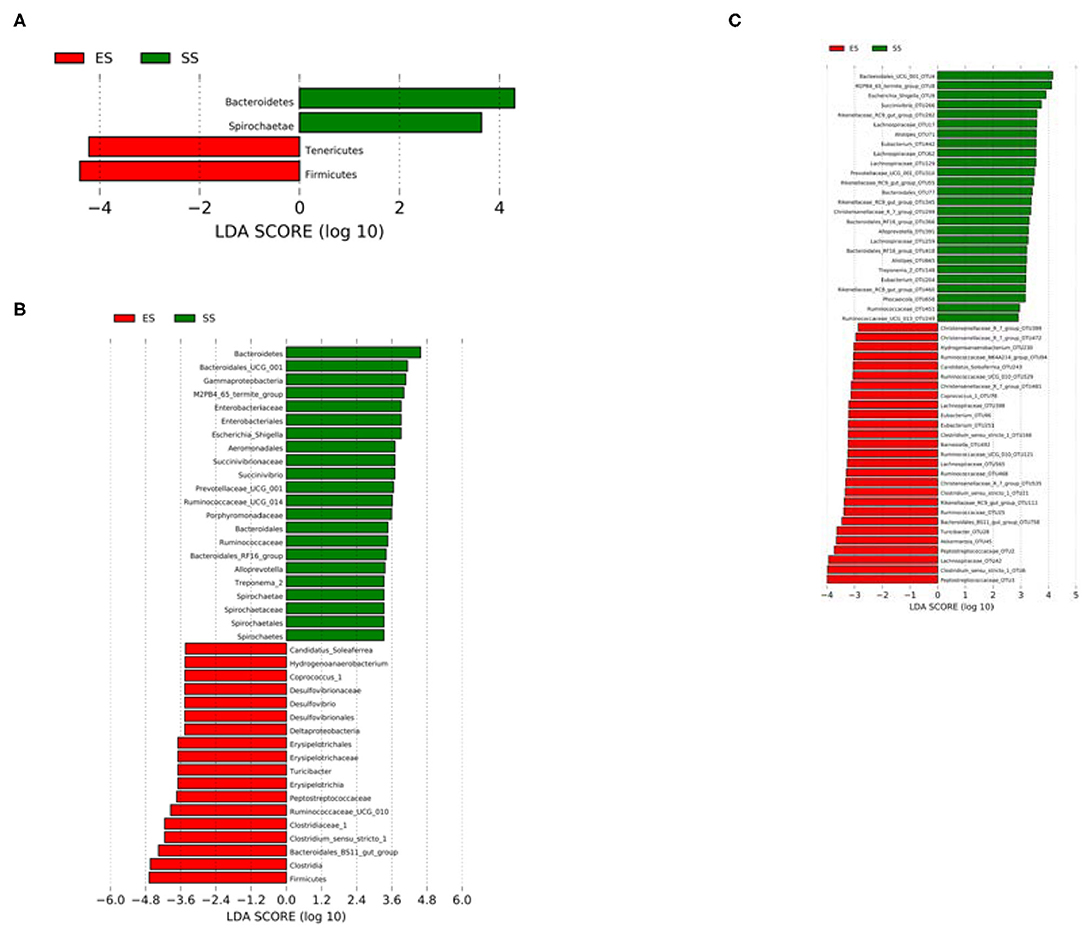
Figure 2. Differences in the rumne microbiota between juvenile and sub-adult Tibetan sheep. Linear discriminant analysis (LDA) effect size (LEfSe) results show that bacterial phyla (A)/genera (B)/OTUs (C) were significantly different in abundance between the two age stages.
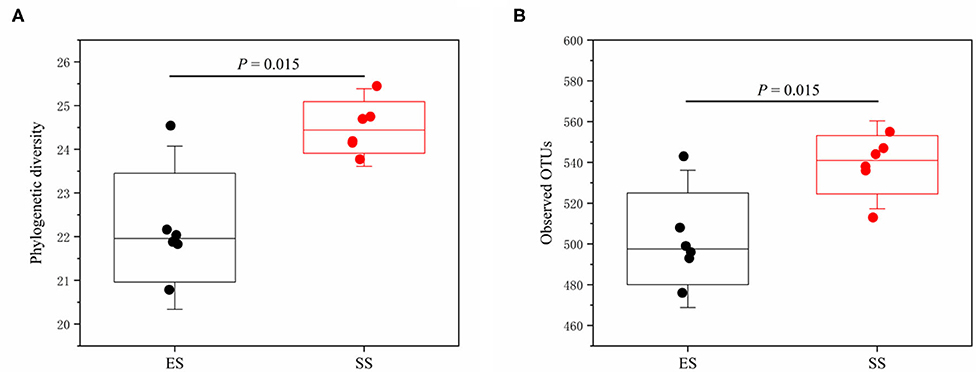
Figure 3. The comparisons of phylogenetic diversity (A) and observed OTUs (B) of the rumen microbiota between juvenile and sub-adult Tibetan sheep. Mann–Whitney U-test was used to test the differences between groups. Significant difference is indicated by P < 0.05.
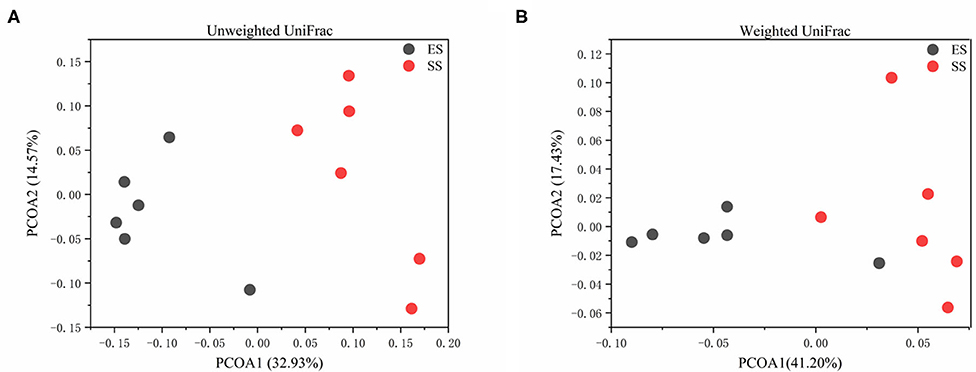
Figure 4. The principal coordinate analysis of rumen microbiota based on unweighted (A) or weighted UniFrac (B) dissimilarities between juvenile and sub-adult Tibetan sheep.
The Difference of Rumen Metabolomics Profiles Between Young and Sub-adult Sheep
Metabolic biomarker can reveal the significantly different metabolites between young and sub-adult Tibetan sheep. A total of 32 biomarkers were identified between the two groups (Figure 5A). Among these metabolites, 11 metabolite biomarkers were enriched in the lambs, these biomarkers included barceloneic acid A, terephthalic acid, 3-Hydroxy-3-Methylglutaric acid, TyrMe-TyrMe-OH, fumaric acid, L-Leucine, 5,6-Dihydrouracil, galactitol, glycochenodeoxycholic acid, (2E)-hexenal and 9(S)-HpOTrE. In contrast, 21 metabolite biomarkers were more abundant in the sub-adult individuals, these metabolites consisted of 4-Pyridoxic acid, biotin, prednisolone, nicotinic acid, lithochol-11-Enic acid, 4-Hydroxy-L-Proline, bicyclo prostaglandin E1, 2-Hydroxyhexadecanoic acid, 6 beta-PGI1, curvulinic acid, hexadecanedioic acid, tridecanedioic acid, 3,4-Dimethylbenzoic acid, phenylethylamine, 13,14-Dihydro-15-Keto prostaglandin D1, dibutyl phthalate, tetradecanedioic acid, 1,11-Undecanedicarboxylic acid, sequiterpene Lactone 326, 5-Oxo-D-Proline, and adipic acid. In addition, there are complex co-occurrence and co-exclusion patterns in these metabolic biomarkers. For example, 4-Hydroxy-L-Proline was positively correlated with curvulinic acid, while 4-Hydroxy-L-Proline was negatively associated with L-Leucine (Figure 5B).
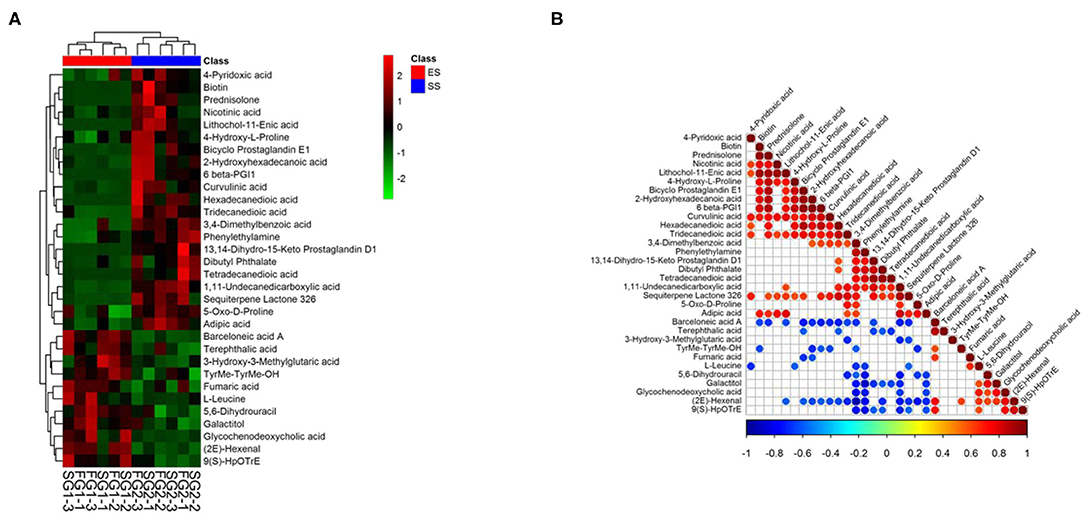
Figure 5. Heatmap showing the significantly different metabolites (VIP > 1.5, A) and their co-occurrence patterns (B).
According to the PCA or PLS-DA plots, we found that the young sheep had significantly different metabolomic profiles with sub-adult individuals regardless of ion mode (positive or negative, Figure 6). Based on KEEG pathways analysis, we found that the metabolic biomarkers were involved in 15 functional pathways, such as valine, leucine and isoleucine biosynthesis, phenylalanine metabolism and vitamin B6 metabolis. Among these pathways, the two pathways, including valine, leucine and isoleucine biosynthesis and phenylalanine metabolism, showed the most striking difference between the young and sub-adult sheep (Figure 7). Specifically, L-Leucine that participates in valine, leucine and isoleucine biosynthesis was more abundant in the young sheep. In contrast, phenylethylamine that takes part in phenylalanine metabolism was more enriched in the sub-adult sheep.
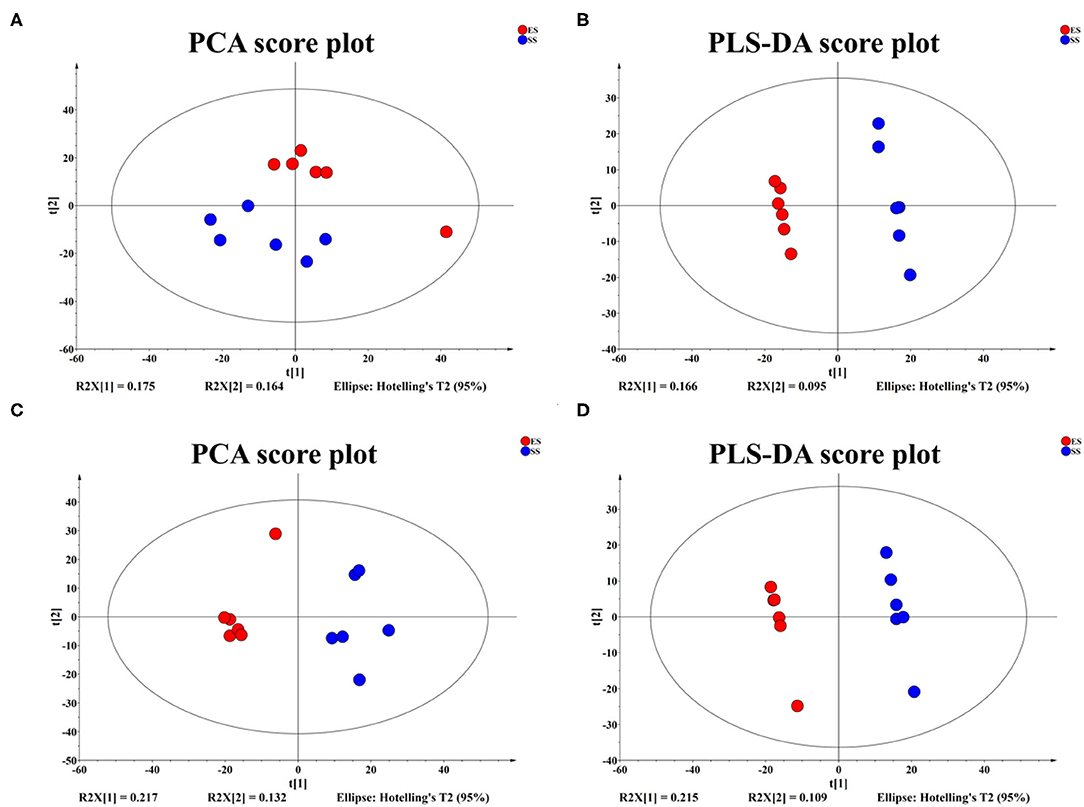
Figure 6. PCA and PLS-DA score plots with positive (A,B) and negative (C,D) ion modes showing the difference of metabolic profiles between juvenile and sub-adult Tibetan sheep.
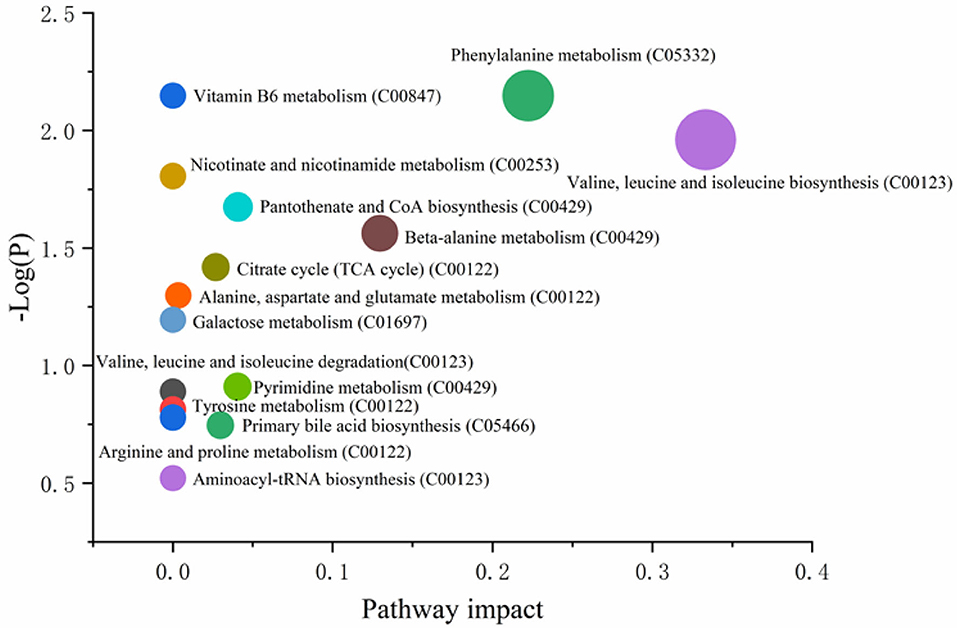
Figure 7. Impacting factor plot of KEEG pathways based on differential metabolites showing the distinct metabolic pathways.
The Difference of Rumen SCFAs Between Young and Sub-adult Sheep
The concentration of acetate, propionate, butyrate, isobutyrate, valerate, isovalerate, hexanoate and total SCFAs were measured in the rumen contents of Tibetan sheep. Acetate, propionate and butyrate were the three most abundant SCFAs in the Tibetan sheep. However, we found that these SCFAs showed no significant difference between the young and sub-adult sheep (P > 0.05, Figure 8).
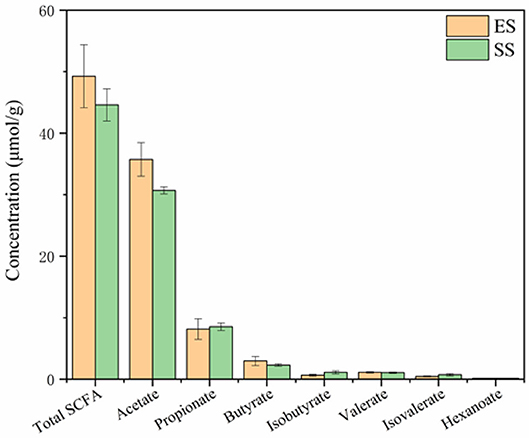
Figure 8. The comparison of short chain fatty acids (SCFAs) in rumen between juvenile and sub-adult Tibetan sheep. One-way ANOVA with Tukey's post-hoc test was used to detect the difference. There are no significant difference between the two age stages (P > 0.05).
The Relationship Between Rumen Microbiota, Metabolomic Profiles and SCFAs
Some rumen microbes were associated with metabolites (Figure 9). For example, Akkermansia showed positive correlations with 3-Hydroxy-3-Methylglutaric acid, while was negatively associated with isobutyrate and isovalerate. Ruminococcus_1 was positively associated with bicyclo prostaglandin E1 and 4-Hydroxy-L-Proline, while showed negative correlations with fumaric acid. Clostridium_sensu_stricto_1 was positively correlated with (2E)-Hexenal, 3-Hydroxy-3-Methylglutaric acid, glycochenodeoxycholic acid, 5,6-Dihydrouracil, 9(S)-HpOTrE, barceloneic acid A, galactitol, and L-Leucine, while this genus showed negative correlations with 16 metabolites (e.g., 1,11-Undecanedicarboxylic acid and phenylethylamine).
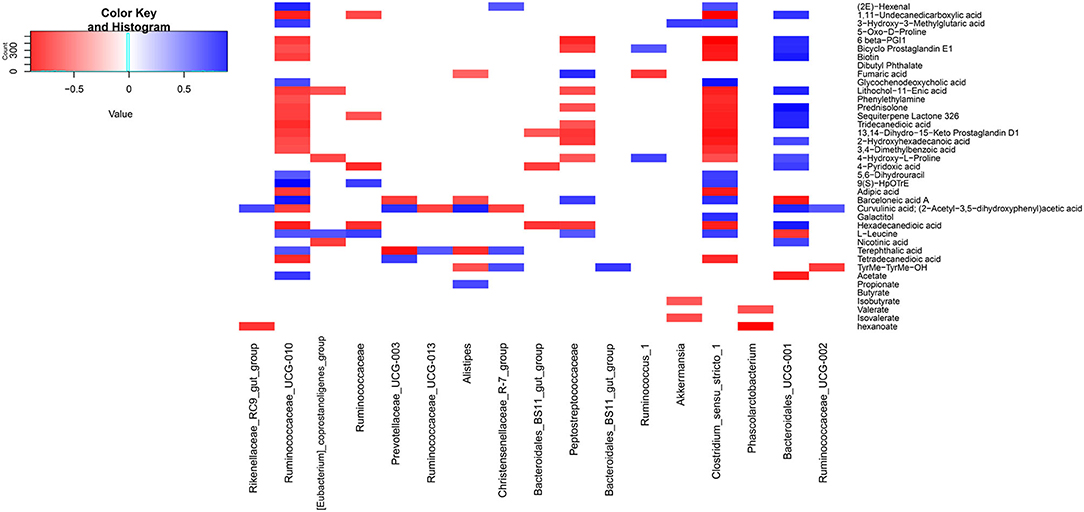
Figure 9. Heatmap plot of spearman correlations between main genera and metabolite biomarkers. Only those correlations with P < 0.05 are shown.
Mantel test was also used to detect the relationship between rumen microbiota (using weighted UniFrac distance) and metabolomic or SCFA profiles. We found that rumen microbial communities were significantly correlated with metabolomic profiles (positive ion mode, r = 0.296, P = 0.028; negative ion mode, r = 0.343, P = 0.019). In addition, SCFA profiles also showed significant associations with rumen microbiota (r = 0.315, P = 0.029).
Discussion
Most of previous reports on ruminants only focused on rumen microbiome, but lacked functional and metabolic information. Here, we investigated the relationship among age, rumen microbiome, metabolomics and SCFA profiles. Our data indicate that age significantly influence the rumen microbiome and metabolomic profiles, but had no impacts on SCFA concentrations. In addition, we found that rumen microbial communities were significantly correlated with metabolomic profiles and SCFAs. These results have important significance for understanding nutrition and metabolism of ruminants on the Qinghai Tibetan Plateau.
The Gut Microbiota in Sub-adult Tibetan Sheep Was More Diverse and Stable Than That in Lambs
The rumen microbial diversity in the sub-adult Tibetan sheep was more diverse than that in the young individuals. This finding is consistent with those of previous studies (5, 26), which showed that the rumen microbial diversity increase with age before adulthood. When ruminants reach close to adulthood, the rumen microbial diversity is relatively stable (5). Compared with the lambs, the higher alpha diversity in the sub-adult sheep may help hosts degrade those indigestible plant polysaccharides and improve rumen performance. It has been demonstrated that gut microbial diversity was positively correlated with cellulolytic activity (25), and also promoted dietary fiber intake (27). Thus, the sub-adult individuals need to better utilize the nutrition from plant-based food by increasing bacterial species.
In addition, the community structures of rumen microbiota were also significantly divergent between the young and sub-adult Tibetan sheep. These results were consistent with those in previous studies (5, 26), which indicated that the microbial community structures were significantly different across ages. The dietary difference between the two age stages may be one important factor impacting the rumen microbial structures. In this study, the sub-adult individuals were mainly fed grass, which consists of plant cellulose. While the young individuals mainly sucked milk, which consists of animal protein. Plant cellulose and animal protein may lead to different microbiota structures (28, 29). In addition, age is also an important impacting factor for the microbial structure. The effects of age on rumen bacterial communities have been demonstrated in Jiao et al. (26) and Jami et al. (5). However, our results can not conclude that whether diet or age was more important in shaping rumen microbiota structures, because these two factors are confounding. Future research should control one factor in order to study the effect of single factor on rumen microbial communities.
Our data showed that Bacteroidetes were more enriched in the sub-adult individuals, while Firmicutes were more abundant in the young sheep. This finding was partly consistent with that in Jami et al. (5), who found that Bacteroidetes and Firmicutes showed an increase trend in relative abundance with age in ruminants. At genus level, Treponema, Ruminococcus and several unknown genera from Ruminococcaceae and Spirochaetaceae were enriched in the sub-adult Tibetan sheep. Some members of these bacterial taxa were involved in the degradation of plant cellulose, hemicellulose, and chitin (30). At OTU level, those microbes belonging to Barnesiella, Akkermansia and Eubacterium were enriched in the young sheep. Some members of Barnesiella have also been isolated from human feces (31), and are associated with host immune response. For example, the strain Barnesiella intestinihominis may activate anti-cancer immune response of spleen T cell, and thus inhibit tumor growth and improve chemotherapy effect (32). Eubacterium, especially Eubacterium hallii, may increase fecal butyrate concentrations and modify bile acid metabolism. More importantly, it can improve the insulin sensitivity in diabetic mice, thus contributing potential benefits to animal health (33). Akkermansia is mucin-degrading bacteria, and may protect hosts against pathogen invasion. The abundance of this genus in gut microbiome is positively correlated with health and metabolic ability of hosts (34, 35), thus may be developed as potential probiotics in future.
Rumen Essential Amino Acids and Related Gene Functional Pathways Were Different Between Age Stages, but SCFA Concentration Was Relatively Stable
Our data showed that the metabolomics profiles were significantly different between the young and sub-adult Tibetan sheep based on PCA or PLS-DA analysis, indicating that age is likely to influence the metabolic profiles of animals. These results were consistent with those in Li et al. (25), who also found that age shaped the metabolome of ruminant animals (36). In addition to age, food is also an important impacting factor for the metabolomic difference. Notably, a total of 32 metabolic biomarkers had significantly difference between the two age stages. Among these metabolites, L-Leucine was an essential amino acid and were enriched in the young Tibetan sheep. The animals were unable to synthesize the L-Leucine themselves, and the milk for the young individuals is possibly the main source. In addition to the nutritional value, L-Leucine can also improve the intestinal development (37) and inhibit excessive accumulation of intestinal fat (38), thus contributing to a lot of benefits for the young Tibetan sheep. By KEEG analysis, we found that the functional pathways, valine, leucine and isoleucine biosynthesis, were more abundant in the young individuals, suggesting that the importance and requirement of L- Leucine for the lambs. In contrast, phenylethylamine that takes part in phenylalanine metabolism was more enriched in the sub-adult sheep. Phenylethylamine is also one of the essential amino acids for animals. Most of them are able to oxidized to tyrosine by the phenylethylamine hydroxylase, and together with tyrosine, they may synthesize important neurotransmitters and hormones, which are involved in glucose metabolism and fat metabolism (39). To sum up, metabolomics results demonstrated that the nutritional requirement of essential amino acids were different for the young and sub-adult Tibetan sheep.
Several organic acid were more abundant in the young sheep, such as barceloneic acid A, terephthalic acid, 3-Hydroxy-3-Methylglutaric acid, fumaric acid, and glycochenodeoxycholic acid. These organic acids probably play important roles in host development and metabolism. For example, it has been reported that terephthalic acid was tightly correlated with the ruminal development of lambs (40). Glycochenodeoxycholic acid participates in bile acid metabolism, and its absence in human bile was an indication of cholestasis (41). By contrast, the rumen of sub-adult Tibetan sheep had more content in 11 organic acids, such as 4-Pyridoxic acid, nicotinic acid, lithochol-11-Enic acid, 2-Hydroxyhexadecanoic acid, curvulinic acid, hexadecanedioic acid, tridecanedioic acid, 3,4-Dimethylbenzoic acid, tetradecanedioic acid, 1,11-Undecanedicarboxylic acid, and adipic acid. Among these organic acids, some can improve animal growth and milk yield. For example, nicotinic acid may improve utilization of feed protein and also milk production (42–44). However, the functional roles of these organic acids in rumen need to be further studied. In addition, we found that the co-occurrence patterns of some metabolic products. Whether these metabolites are produced directly by same microorganisms, or indirectly by different microbes, also needs to be explored in future.
SCFAs are the end metabolic products of plant cellulose and other dietary polysaccharides via rumen microbial fermentation. These metabolites contributes to energy and nutrition of ruminants. For example, butyrate can supply ~60–70% energy for normal epithelial cells (45). Besides, these SCFAs may also influence animal physiology, including the synthesis of macromolecular substances in vivo. For instance, acetate can been transported to peripheral tissues, and then to liver for participating in cholesterol synthesis (46). Propionate is mainly assimilated by liver, and is involved in gluconeogenesis and protein synthesis (47). In addition, SCFAs also have antimicrobial and anti-inflammatory properties. For example, it has been demonstrated that butyrate, propionate and hexanoate can reduce Staphylococcus aureus internalization into bovine mammary epithelial cells and regulate antimicrobial peptide gene expression (48, 49). Our results showed that the concentration of various SCFAs was relatively stable in different age stages, indicating that SCFAs were not impacted by age although gut microbiota changed.
Rumen Microbiota Was Associated With Metabolomics and SCFAs
Our results showed that rumen microbiota structure was associated with metabolic profiles. This result was congruent with that in goat (7), fish (50), human (51), gorillas (52), and mice (53), suggesting that a tight link between microbiome and metabolome independent of host species. In addition, a weak correlation was also found between rumen microbiota and SCFA profiles, and this finding was consistent with that in our previous study on pika gut microbiome (25). Spearman correlation analysis revealed the potential relationship between rumen microbiota and metabolome or SCFAs. Because some rumen microbes always co-occur with metabolites, this result has important significance for regulating animal nutrition and health by microbiome intervention. However, it seems that we can not conclude which specific microbes responsible for certain metabolites due to the complex interaction among microorganisms, such as resource competition (54) and cross-feeding (55). Thus, future research should isolate these bacterial strains and study the relationship between specific bacteria and metabolites.
Conclusion
In conclusion, we found that both rumen microbial community structures and metabolite profiles of Tibetan sheep were distinct at different ages, but rumen SCFA concentration was relatively stable between the two age stages. Notably, metabolomics analysis showed that the nutritional requirement of essential amino acids in rumen were different between the lambs and sub-adult Tibetan sheep. We found L-Leucine that participates in valine, leucine and isoleucine biosynthesis was more abundant in the lambs, while phenylethylamine that takes part in phenylalanine metabolism was enriched in the sub-adults. In addition, rumen microbita was associated with metabolomics and SCFAs, indicating the close relationship between microbial composition and metabolites. These results have important significance for regulating animal nutrition and health by microbiome intervention.
Data Availability Statement
All the original 16S rRNA gene data in this study were submitted to the European Nucleotide Archive, and were available by accession no. PRJEB36249.
Ethics Statement
Animal ethics approval for the present project was obtained from the Animal Ethics Committee of Lanzhou University.
Author Contributions
HL and JQ designed this study. JQ carried out the animal experiments and sample collection. HL was responsible for the molecular experiments, data analysis, and wrote the original manuscript. All authors revised and edited the manuscript.
Funding
This work was supported by the National Natural Science Foundation of China (31770459), CAS Light of West China, Youth Innovation Promotion Association CAS, Applied and Basic Research Program of Qinghai Province (2017-ZJ-761), and Thousand People Plan of Qinghai Province and Qinghai Innovation Platform Construction Project (2017-ZJ-Y20).
Conflict of Interest
The authors declare that the research was conducted in the absence of any commercial or financial relationships that could be construed as a potential conflict of interest.
Abbreviations
SCFA, Short chain fatty acid; OTU, Operational taxonomic units; PCoA, Principal coordinates analysis; RDP, Ribosomal Database Project.
References
1. Rey M, Enjalbert F, Combes S, Cauquil L, Bouchez O, Monteils V. Establishment of ruminal bacterial community in dairy calves from birth to weaning is sequential. J Appl Microbiol. (2014) 116:245–57. doi: 10.1111/jam.12405
2. Mackie RI. Mutualistic fermentative digestion in the gastrointestinal tract: diversity and evolution. Integ Compar Biol. (2002) 42:319–26. doi: 10.1093/icb/42.2.319
3. Van Soest P. Nutritional Ecology of the Ruminant, 2nd edn. New York, NY: Cornell University Press (1994).
4. Minato H, Otsuka M, Shirasaka S, Itabashi H, Mitsumori M. Colonization of microorganisms in the rumen of young calves. J Gene Appl Microbiol. (1992) 38:447–56. doi: 10.2323/jgam.38.447
5. Jami E, Israel A, Kotser A, Mizrahi I. Exploring the bovine rumen bacterial community from birth to adulthood. ISME J. (2013) 7:1069. doi: 10.1038/ismej.2013.2
6. Madsen R, Lundstedt T, Trygg J. Chemometrics in metabolomics—a review in human disease diagnosis. Anal Chim Acta. (2010) 659:23–33. doi: 10.1016/j.aca.2009.11.042
7. Mao SY, Huo WJ, Zhu WY. Microbiome–metabolome analysis reveals unhealthy alterations in the composition and metabolism of ruminal microbiota with increasing dietary grain in a goat model. Environ Microbiol. (2016) 18:525–41. doi: 10.1111/1462-2920.12724
8. Mahrous EA, Farag MA. Two dimensional NMR spectroscopic approaches for exploring plant metabolome: a review. J Adv Res. (2015) 6:3–15. doi: 10.1016/j.jare.2014.10.003
9. Yang S, Sadilek M, Lidstrom ME. Streamlined pentafluorophenylpropyl column liquid chromatography–tandem quadrupole mass spectrometry and global 13C-labeled internal standards improve performance for quantitative metabolomics in bacteria. J Chromatograp A. (2010) 1217:7401–10. doi: 10.1016/j.chroma.2010.09.055
10. Tian H, Wang W, Zheng N, Cheng J, Li S, Zhang Y, et al. Identification of diagnostic biomarkers and metabolic pathway shifts of heat-stressed lactating dairy cows. J Proteom. (2015) 125:17–28. doi: 10.1016/j.jprot.2015.04.014
11. Xin G, Long R, Guo X, Irvine J, Ding L, Ding L, et al. Blood mineral status of grazing Tibetan sheep in the Northeast of the Qinghai–Tibetan Plateau. Livest Sci. (2011) 136:102–7. doi: 10.1016/j.livsci.2010.08.007
12. Zhang Z, Xu D, Li W, Hao J, Wang J, Xin Z. Convergent evolution of rumen microbiomes in high-altitude mammals. Curr Biol. (2016) 26:1873–9. doi: 10.1016/j.cub.2016.05.012
13. Huang WC, Jia X, Li J, Li M. Dynamics of microbial community in the bioreactor for bisphenol S removal. Sci Total Environ. (2019) 662:15–21. doi: 10.1016/j.scitotenv.2019.01.173
14. Li H, Li T, Beasley DE, Hedenec P, Xiao Z, Zhang S, et al. Diet diversity is associated with beta but not alpha diversity of pika gut microbiota. Front Microbiol. (2016) 7:1169. doi: 10.3389/fmicb.2016.01169
15. Li H, Qu J, Li T, Li J, Lin Q, Li X. Pika population density is associated with composition and diversity of gut microbiota. Front Microbiol. (2016) 7:758. doi: 10.3389/fmicb.2016.00758
16. Li H, Li T, Yao M, Li J, Zhang S, Wirth S, et al. Pika gut may select for rare but diverse environmental bacteria. Front Microbiol. (2016) 7:1269. doi: 10.3389/fmicb.2016.01269
17. Li H, Zhou R, Zhu J, Huang X, Qu J. Environmental filtering increases with elevation for the assembly of gut microbiota in wild pikas. Microbial Biotechnol. (2019) 12:976–92. doi: 10.1111/1751-7915.13450
18. Magoč T, Salzberg SL. FLASH: fast length adjustment of short reads to improve genome assemblies. Bioinformatics. (2011) 27:2957–63. doi: 10.1093/bioinformatics/btr507
19. Rognes T, Flouri T, Nichols B, Quince C, Mahé F. VSEARCH: a versatile open source tool for metagenomics. PeerJ. (2016) 4:e2584. doi: 10.7717/peerj.2584
20. Caporaso JG, Bittinger K, Bushman FD, DeSantis TZ, Andersen GL, Knight R. PyNAST: a flexible tool for aligning sequences to a template alignment. Bioinformatics. (2009) 26:266–7. doi: 10.1093/bioinformatics/btp636
21. Cole JR, Wang Q, Cardenas E, Fish J, Chai B, Farris RJ, et al. the ribosomal database project: improved alignments and new tools for rRNA analysis. Nucleic Acids Res. (2008) 37:D141–5. doi: 10.1093/nar/gkn879
22. Lozupone C, Knight R. UniFrac: a new phylogenetic method for comparing microbial communities. Appl Environ Microbiol. (2005) 71:8228–35. doi: 10.1128/AEM.71.12.8228-8235.2005
23. Li Y, Chen C, Li Y, Ding Z, Shen J, Wang Y, et al. The identification and evaluation of two different color variations of tea. J Sci Food Agri. (2016) 96:4951–61. doi: 10.1002/jsfa.7897
24. Smith CA, Want EJ, O'Maille G, Abagyan R, Siuzdak G. XCMS: processing mass spectrometry data for metabolite profiling using nonlinear peak alignment, matching, and identification. Analy Chem. (2006) 78:779–87. doi: 10.1021/ac051437y
25. Li H, Qu J, Li T, Wirth S, Zhang Y, Zhao X, et al. Diet simplification selects for high gut microbial diversity and strong fermenting ability in high-altitude pikas. Appl Microbiol Biotechnol. (2018) 102:6739–51. doi: 10.1007/s00253-018-9097-z
26. Jiao J, Huang J, Zhou C. Taxonomic identification of ruminal epithelial bacterial diversity during rumen development in goats. Appl Environ Microbiol. (2015) 81:3502–9. doi: 10.1128/AEM.00203-15
27. Tap J, Furet JP, Bensaada M. Gut microbiota richness promotes its stability upon increased dietary fibre intake in healthy adults. Environ Microbiol. (2015) 17:4954–6. doi: 10.1111/1462-2920.13006
28. Kinross JM, von Roon AC, Holmes E, Darzi A, Nicholson JK. The human gut microbiome: implications for future health care. Curr Gastroenterol Rep. (2008) 10:396–403. doi: 10.1007/s11894-008-0075-y
29. Rampelli S, Schnorr SL, Consolandi C, Turroni S, Severgnini M, Peano C, et al. Metagenome sequencing of the Hadza hunter-gatherer gut microbiota. Curr Biol. (2015) 25:1682–93. doi: 10.1016/j.cub.2015.04.055
30. Dai X, Tian Y, Li J, Su X, Wang X, Zhao S, et al. Metatranscriptomic analyses of plant cell wall polysaccharide degradation by microorganisms in cow rumen. Appl Environ Microbiol. (2014) 81:1375–86. doi: 10.1128/AEM.03682-14
31. Morotomi M, Nagai F, Sakon H, Tanaka R. Dialister succinatiphilus sp. nov and Barnesiella intestinihominis sp nov, isolated from human faeces. Int J Syst Evolu Microbiol. (2008) 58:2716–20. doi: 10.1099/ijs.0.2008/000810-0
32. Daillère R, Vétizou M, Waldschmitt N, Yamazaki T, Isnard C, Poirier-Colame V, et al. Enterococcus hirae and Barnesiella intestinihominis facilitate cyclophosphamide-induced therapeutic immunomodulatory effects. Immunity. (2016) 45:931–43. doi: 10.1016/j.immuni.2016.09.009
33. Udayappan S, Manneras-Holm L, Chaplin-Scott A, Belzer C, Herrema H, Dallinga-Thie GM, et al. Oral treatment with Eubacterium hallii improves insulin sensitivity in db/db mice. NPJ Biofilms Microb. (2016) 2:16009. doi: 10.1038/npjbiofilms.2016.9
34. Schneeberger M, Everard A, Gómez-Valadés AG, Matamoros S, Ramírez S, Delzenne NM, et al. Akkermansia muciniphila inversely correlates with the onset of inflammation, altered adipose tissue metabolism and metabolic disorders during obesity in mice. Sci Rep. (2015) 5:16643. doi: 10.1038/srep16643
35. Plovier H, Everard A, Druart C, Depommier C, Van Hul M, Geurts L, et al. A purified membrane protein from Akkermansia muciniphila or the pasteurized bacterium improves metabolism in obese and diabetic mice. Nat Med. (2017) 23:107. doi: 10.1038/nm.4236
36. Li Z, Wang X, Zhang T, Si H, Nan W, Xu C, et al. The development of microbiota and metabolome in small intestine of sika deer (Cervus nippon) from birth to weaning. Front Microbiol. (2018) 9:4. doi: 10.3389/fmicb.2018.00004
37. Sun Y, Wu Z, Li W, Zhang C, Sun K, Ji Y, et al. Dietary L-leucine supplementation enhances intestinal development in suckling piglets. Amino Acids. (2015) 47:1517–25. doi: 10.1007/s00726-015-1985-2
38. Chen Q, Reimer RA. Dairy protein and leucine alter GLP-1 release and mRNA of genes involved in intestinal lipid metabolism in vitro. Nutrition. (2009) 25:340–9. doi: 10.1016/j.nut.2008.08.012
39. Harper AE. Phenylalanine metabolism. In: Stegink LD, Filer LJ Jr, editors. Aspartame Physiology and Biochemistry. New York, NY: Dekker (1984). p. 77–109.
40. Kunkel H, Tutt FE, Reagor JC, Glimp HA, Robbins JD. Ruminal development of lambs related to rates of gain, anabolic estrogens, antibiotics, hydroxyzine and terephthalic acid. J Animal Sci. (1962) 21:681–7. doi: 10.2527/jas1962.214681x
41. Ijare OB, Bezabeh T, Albiin N, Arnelo U, Bergquist A, Lindberg B, et al. Absence of glycochenodeoxycholic acid (GCDCA) in human bile is an indication of cholestasis: a 1H MRS study. NMR Biomed. (2009) 22:471–9. doi: 10.1002/nbm.1355
42. Erickson PS, Murphy MR, Clark J. Supplementation of dairy cow diets with calcium salts of long-chain fatty acids and nicotinic acid in early lactation. J Dairy Sci. (1992) 75:1078–89. doi: 10.3168/jds.S0022-0302(92)77852-7
43. Drackley JK, LaCount D, Elliott J, Klusmeyer T, Overton T, Clark J, et al. Supplemental fat and nicotinic acid for Holstein cows during an entire lactation. J Dairy Sci. (1998) 81:201–14. doi: 10.3168/jds.S0022-0302(98)75567-5
44. Yuan K, Shaver R, Bertics S, Espineira M, Grummer R. Effect of rumen-protected niacin on lipid metabolism, oxidative stress, and performance of transition dairy cows. J Dairy Sci. (2012) 95:2673–9. doi: 10.3168/jds.2011-5096
45. Scheppach W. Effects of short chain fatty acids on gut morphology and function. Gut. (1994) 35(Suppl. 1):S35–8. doi: 10.1136/gut.35.1_Suppl.S35
46. Wolever T, Brighenti F, Royall D, Jenkins AL, Jenkins DJ. Effect of rectal infusion of short chain fatty acids in human subjects. Am J Gastroenterol. (1989) 84: 1027–33.
47. Wolever TM, Spadafora P, Eshuis H. Interaction between colonic acetate and propionate in humans. Am J Clin Nutri. (1991) 53:681–7. doi: 10.1093/ajcn/53.3.681
48. Ochoa-Zarzosa A, Villarreal-Fernández E, Cano-Camacho H, López-Meza JE. Sodium butyrate inhibits Staphylococcus aureus internalization in bovine mammary epithelial cells and induces the expression of antimicrobial peptide genes. Microb Pathog. (2009) 47:1–7. doi: 10.1016/j.micpath.2009.04.006
49. Alva-Murillo N, Ochoa-Zarzosa A, López-Meza JE. Short chain fatty acids (propionic and hexanoic) decrease Staphylococcus aureus internalization into bovine mammary epithelial cells and modulate antimicrobial peptide expression. Vet Microbiol. (2012) 155:324–31. doi: 10.1016/j.vetmic.2011.08.025
50. Li T, Long M, Li H, Gatesoupe F-J, Zhang X, Zhang Q, et al. Multi-omics analysis reveals a correlation between the host phylogeny, gut microbiota and metabolite profiles in cyprinid fishes. Front Microbiol. (2017) 8:454. doi: 10.3389/fmicb.2017.00454
51. De Filippis F, Pellegrini N, Vannini L, Jeffery IB, La Storia A, Laghi L, et al. High-level adherence to a mediterranean diet beneficially impacts the gut microbiota and associated metabolome. Gut. (2016) 65:1812–21. doi: 10.1136/gutjnl-2015-309957
52. Gomez A, Petrzelkova K, Yeoman CJ, Vlckova K, Mrázek J, Koppova I, et al. Gut microbiome composition and metabolomic profiles of wild western lowland gorillas (Gorilla gorilla gorilla) reflect host ecology. Mol Ecol. (2015) 24:2551–65. doi: 10.1111/mec.13181
53. Lu K, Abo RP, Schlieper KA, Graffam ME, Levine S, Wishnok JS, et al. Arsenic exposure perturbs the gut microbiome and its metabolic profile in mice: an integrated metagenomics and metabolomics analysis. Environ Health Perspect. (2014) 122:284–91. doi: 10.1289/ehp.1307429
54. Mahowald MA, Rey FE, Seedorf H, Turnbaugh PJ, Fulton RS, Wollam A, et al. Characterizing a model human gut microbiota composed of members of its two dominant bacterial phyla. Proc Natl Acad Sci. (2009) 106:5859–64. doi: 10.1073/pnas.0901529106
Keywords: rumen microbiome, metabolomics, SCFA, essential amino acids, age
Citation: Li H, Yu Q, Li T, Shao L, Su M, Zhou H and Qu J (2020) Rumen Microbiome and Metabolome of Tibetan Sheep (Ovis aries) Reflect Animal Age and Nutritional Requirement. Front. Vet. Sci. 7:609. doi: 10.3389/fvets.2020.00609
Received: 14 June 2020; Accepted: 28 July 2020;
Published: 02 September 2020.
Edited by:
Asta Tvarijonaviciute, University of Murcia, SpainReviewed by:
Ingrida Monkeviciene, Lithuanian University of Health Sciences, LithuaniaManuel Gonzalez Ronquillo, Universidad Autónoma del Estado de México, Mexico
Copyright © 2020 Li, Yu, Li, Shao, Su, Zhou and Qu. This is an open-access article distributed under the terms of the Creative Commons Attribution License (CC BY). The use, distribution or reproduction in other forums is permitted, provided the original author(s) and the copyright owner(s) are credited and that the original publication in this journal is cited, in accordance with accepted academic practice. No use, distribution or reproduction is permitted which does not comply with these terms.
*Correspondence: Huan Li, lihuanzky@163.com; Jiapeng Qu, jpqu@nwipb.cas.cn