- 1Department of Health Management, Atlantic Veterinary College, University of Prince Edward Island, Charlottetown, PE, Canada
- 2Department of Pathology and Microbiology, Atlantic Veterinary College, University of Prince Edward Island, Charlottetown, PE, Canada
- 3Mathematics and Computer Science Division, Argonne National Laboratory, Chicago, IL, USA
- 4Diagnostic Services, Atlantic Veterinary College, University of Prince Edward Island, Charlottetown, PE, Canada
The objectives of this study are to determine the occurrence of antimicrobial resistance (AMR) genes using whole-genome sequence (WGS) of Streptococcus uberis (S. uberis) and Streptococcus dysgalactiae (S. dysgalactiae) isolates, recovered from dairy cows in the Canadian Maritime Provinces. A secondary objective included the exploration of the association between phenotypic AMR and the genomic characteristics (genome size, guanine–cytosine content, and occurrence of unique gene sequences). Initially, 91 isolates were sequenced, and of these isolates, 89 were assembled. Furthermore, 16 isolates were excluded due to larger than expected genomic sizes (>2.3 bp × 1,000 bp). In the final analysis, 73 were used with complete WGS and minimum inhibitory concentration records, which were part of the previous phenotypic AMR study, representing 18 dairy herds from the Maritime region of Canada (1). A total of 23 unique AMR gene sequences were found in the bacterial genomes, with a mean number of 8.1 (minimum: 5; maximum: 13) per genome. Overall, there were 10 AMR genes [ANT(6), TEM-127, TEM-163, TEM-89, TEM-95, Linb, Lnub, Ermb, Ermc, and TetS] present only in S. uberis genomes and 2 genes unique (EF-TU and TEM-71) to the S. dysgalactiae genomes; 11 AMR genes [APH(3′), TEM-1, TEM-136, TEM-157, TEM-47, TetM, bl2b, gyrA, parE, phoP, and rpoB] were found in both bacterial species. Two-way tabulations showed association between the phenotypic susceptibility to lincosamides and the presence of linB (P = 0.002) and lnuB (P < 0.001) genes and the between the presence of tetM (P = 0.015) and tetS (P = 0.064) genes and phenotypic resistance to tetracyclines only for the S. uberis isolates. The logistic model showed that the odds of resistance (to any of the phenotypically tested antimicrobials) was 4.35 times higher when there were >11 AMR genes present in the genome, compared with <7 AMR genes (P < 0.001). The odds of resistance was lower for S. dysgalactiae than S. uberis (P = 0.031). When the within-herd somatic cell count was >250,000 cells/mL, a trend toward higher odds of resistance compared with the baseline category of <150,000 cells/mL was observed. When the isolate corresponded to a post-mastitis sample, there were lower odds of resistance when compared with non-clinical isolates (P = 0.01). The results of this study showed the strength of associations between phenotypic AMR resistance of both mastitis pathogens and their genotypic resistome and other epidemiological characteristics.
Introduction
Bovine mastitis continues to be a priority in the dairy cattle industry, as it is one of the leading causes of reduced profitability, through decreased milk production and increased treatment costs (2). Mastitis is caused by a wide variety of pathogens, with different pathogenic characteristics that damage the mammary gland in a variety of ways (3, 4). Multiple control strategies have been implemented, depending on the epidemiology of the pathogens involved in the intramammary infections (IMIs) (5, 6).
Among all the microbial species causing bovine mastitis, Streptococcus spp. are part of a larger group of organisms associated with IMI (7) and have been linked with both clinical and subclinical mastitis (6). Streptococcus uberis (S. uberis) and Streptococcus dysgalactiae (S. dysgalactiae) have been classified as Gram-positive, catalase-negative cocci (PNC) microorganisms (8) and are frequently isolated in IMI on Canadian dairy farms (9). S. uberis has been reported as having a heterogeneous genotype pattern (6) and associated with environmental transmission sources (10), such as water, soil, plant matter, and bedding material (11). In contrast, S. dysgalactiae can spread from infected cows to healthy herd mates (12) or directly from the cow’s environment (13, 14).
Antimicrobial resistance (AMR) has been reported among different mastitis pathogens to various degrees in both short- and long-term studies (15). Phenotypic in vitro susceptibility is the ability of an antimicrobial to inhibit the growth of a bacterium in an assay at a concentration above clinical cutoff. However, this indicates high probability of treatment in vivo. Recently, patterns of phenotypic antimicrobial in vitro susceptibility have been reported for isolates of S. uberis and S. dysgalactiae (1). These results are our group’s (AMR of the Atlantic Veterinary College) previous work in the area. In that study, isolates of S. uberis displayed lower susceptibility and higher minimum inhibitory concentration (MIC) than S. dysgalactiae isolates (1). Similarly, in a separate study, multidrug resistance was more common among S. uberis isolates than for isolates of S. dysgalactiae (16).
The advent of whole-genome sequencing (WGSq) allows the detection of antimicrobial drug resistance determinants and virulence factors genes in mastitis pathogens. Whole-genome sequencing and comparison also allow the investigation of the transmission of bacterial genetic material among host populations with high detail (17, 18). In recent years, there have been several studies using WGS comparisons (the presence of particular sequences) for bovine mastitis pathogens, such as Escherichia coli (E. coli) (19) and Staphylococcus aureus (S. aureus) strains (genotyping) (20) and for both S. uberis and S. dysgalactiae (gene content and comparative genomics) (21, 22). However, there is scarce literature related to the understanding of the AMR genetic potential among bovine mastitis streptococcal species and its relationship with specific epidemiological characteristics such as somatic cell count (SCC) and days in milk (DIM) of the cow.
The primary objectives of this research were to determine the occurrence of unique AMR genes found in S. uberis and S. dysgalactiae isolates collected from dairy cows in the Maritime Provinces of Canada using WGS analysis and to explore the relationship between phenotypic susceptibility and the presence of AMR resistance genes and epidemiological characteristics. In addition, the secondary objective was to explore the association between phenotypic AMR and the genomic characteristics, such as genome size, guanine–cytosine (GC) content, and number of unique AMR gene sequences, of the isolates.
Materials and Methods
Bacterial Collection
Streptococcus uberis and S. dysgalactiae isolates were obtained from the Mastitis Pathogen Culture Collection of the Canadian Bovine Mastitis and Milk Quality Research Network (CBMQRN) consisting of 16,500 isolates, recovered from 91 commercial dairy herds located in 6 Canadian provinces (23). From this national cohort, 98 isolates included in the Cameron et al.’s (1) study were initially considered for WGS, based on their resistant and pan susceptibility characteristics. Seven isolates were excluded, from the initial number due to low DNA quality. Consequently, 91 isolates of S. uberis (n = 66) and S. dysgalactiae (n = 25) were subject to WGS.
Bacterial Culture
One colony of each isolate was selected and cultured overnight with incubation (6–12 h) at 37°C in 5 mL of Trypticase soy broth (Bacto™ Tryptic Soy Broth, Becton Dickinson, Mississauga, ON, Canada). The grown-up colonies were collected for DNA extraction. A total of 500 µL of each bacterial culture was then removed and added to an equal volume of glycerol freezing solution (65% glycerol, 0.1 M MgSO4, 0.025 M Tris–HCl, pH 8.0). Samples were stored at −80°C until needed.
Determination of Antimicrobial Susceptibility
The antimicrobial susceptibility of each pure subculture was determined by the Sensititre microdilution plate system, containing serial dilutions of eight antimicrobials (ampicillin, ceftiofur, cephalothin, erythromycin, penicillin, penicillin–novobiocin, pirlimycin, and tetracycline). The mastitis plates were read automatically, as described in the study by Cameron et al. (1). The MIC and the susceptibility status were interpreted according to the Clinical Laboratory and Standards Institute guidelines for each of the antimicrobials (CLSI 2013).
Nucleic Acid Extraction
The DNA extraction was performed using the QIAamp DNA Mini Kit (Qiagen Inc., Canada). A total of 1.5 mL of suspended bacterial culture was pipetted into a 1.5-mL microcentrifuge tube and then pelleted by centrifugation for 10 min at 5,000 × g. The bacterial pellet was suspended in 180 µL of lysozyme (20 mg/mL in 20 mM Tris–HCl, pH 8.0; 2 mM EDTA; 1.2% Triton) and incubated at 37°C for 45 min. Then 20 µL of ProtK (Qiagen kit) and 200 µL of buffer AL (Qiagen Kit) were added to each tube and mixed by vortexing. Samples were incubated at 56°C for 1 h. After that, 4 µL of RNase A (100 mg/mL) was added, mixed by vortexing for 15 s, and incubated at 70°C for 60 min. This step was followed by adding 200 µL of ethanol (95–100%) and pulse vortexing for 15 s. Samples were added to the QIAamp mini spin column, and the method was continued according to kit instructions. All samples were eluted with 30 µL of elution buffer from the kit.
DNA Quantification and DNA Sequencing
From each sample, 2 µL was used to determine the 260:280 ratios for DNA quality and to estimate the DNA concentration using the NanoDrop 1000 spectrophotometer (Thermo Scientific, Wilmington, DE, USA). At least 500 ng of DNA for each sample was added to a 96-well plate and sent for sequencing.
Whole-Genome Sequencing
Samples for WGS were processed at the Genome British Columbia Genome Sciences Centre. A PCRFree genome library construction from 500 ng of genomic DNA in 62.5 μL of elution buffer (i.e., a concentration of 8 ng/mL) was used. Different qualitative and quantitative control steps were taken for samples at various stages of the library construction (i.e., initial receipt, post-shearing, post-library construction), and these steps were as follows: QCed for quality and quantity with Caliper LabChip GX for DNA samples using the High Sensitivity Assay (PerkinElmer, Inc., USA), Agilent DNA 1000 series II assay, Quant-iT dsDNA HS Assay Kit using Qubit fluorometer (Invitrogen), and KAPA qPCR. A paired-end 125 bp sequencing was performed on the Illumina HiSeq 2500 platform, using V4 chemistry according to manufacturer recommendations. The average coverage was 367×. The overall coverage range was 232×–534×, with the exception of two samples that only got 65×, and therefore, those samples were discarded.
Bioinformatics and AMR Genes Search
The sequencing center provided libraries in binary alignment files (BAM) against the provided reference genomes for each pathogen species: S. uberis 0140J (21) and S. dysgalactiae subsp. dysgalactiae ATCC 27957 (22). Only Illumina chastity-passed reads were included in the alignment. However, these alignments were not used in this analysis. Instead, the files were transformed back to FASTA format with SAMtools (version 1.3) (24). These genomes were de novo assembled using the auto strategy option. This assembly option integrates algorithms for base calling correction (BayesHammer), assembly (Velvet, Spades, IDBA), and scoring (ARAST) giving three separate assemblies and indicating the best assembly (25). Furthermore, the genomes were annotated using the Rast tool kit found in the Pathosystems Resource Integration Center (PATRIC) (PATRIC 3.2.96), as part of the all-bacteria Bioinformatics Resource Center available online (25). The genomes were visualized with the genome browser option, powered by JBrowse (development version), available also in the PATRIC resource (25). Furthermore, these genomes were interrogated for AMR genes, using the specialty genes service of PATRIC 3.2.96 with the AMR filter. This specialty genes filtered data type, including reference sequences of the Comprehensive Antibiotic Resistance Database (CARD) (26) and the Antibiotic Resistance Genes Database (ARDB) (27), which are integrated in the PATRIC tool (http://patricbrc.org). The sequence queries for the specialty genes search were performed with 80% coverage and 80% identity value, including both literature and BLASTP options, described in the PATRIC resource (25).
Other characteristics of the genomes retrieved from the analysis included GC content and genome size. The information regarding AMR genes obtained from the PATRIC tool was downloaded in a tab-delimited format from PATRIC and merged with the phenotypic resistance data used in the study by Cameron et al. (1). This information was processed and analyzed in Stata 13 statistical/data analysis software (28).
Statistical Analysis
Genomic and Antibiotic Resistance Characteristics
Descriptive statistics for the genomic information, such as genome size and GC content, were calculated, stratified by susceptibility phenotype and species (S. dysgalactiae and S. uberis). Only genomes with sizes <2,300,000 bp (lower than the 75% percentile) were considered for further analysis, since genomes greater than this value could be a result of mixed culture for our population of isolates. The occurrence of identified AMR resistance genes sequences by bacterial species was determined. The number of unique AMR gene sequences per genome was calculated and categorized into three different levels as follows: (1) <7 AMR genes, (2) ≥7–<11 AMR genes, and (3) ≥11 AMR genes. The average within-herd SCC was included as a host factor and categorized as in the study by Cameron et al. (1) into three categories: (1) ≤150,000 cells/mL, (2) 151,000–250,000 cells/mL, and (3) 251,000–400,000 cells/mL. The milk sample type from which the isolate was cultured and placed in one of three categories as follows: clinically healthy lactational milk sample (all isolates recovered in lactation and pre-dry-off sampling) from cows with visually normal milk, post-calving milk sample (collected between 0 and 14 DIM), and post-clinical mastitis milk sample (collected between 2 and 5 weeks after a mastitis event). In addition, the presence of unique AMR genes relevant to each antimicrobial class, among the MIC values, was determined.
Binary variables for each of the three classes of antimicrobials (β-lactam, macrolide, and tetracycline) were constructed based on the presence/absence of AMR gene(s) for that specific class. The occurrence of class-specific AMR genes were compared between phenotypically susceptible versus intermediate/resistant isolates for each of the eight antimicrobials antimicrobial using Fisher’s exact test. Unconditional associations for variable selection between the phenotypic AMR (the outcome variable) and the main epidemiologic variables reported by Cameron et al. (1) were assessed. A statistical significance of P ≤ 0.20 was used for the unconditional associations, and further P ≤ 0.05 statistical significance criteria were used in the model building process, described below. However, variables with an overall P ≤ 0.10 for all categories were retained for informative purposes and shown in the final model. For informative purposes, we left associations in the tables with P < 0.10, but we used a P < 0.20 for the selection of the variables.
Two separate models were calculated to assess the association between the outcome variable with the number of unique AMR genes sequences (three-level categorical predictor as described above), herd SCCs (three-level categorical predictor as described above), bacterial species (S. uberis and S. dysgalactiae), and sample type (clinically healthy lactational, post-calving, and post-clinical mastitis milk samples). Isolate and herd were included as random effects. The first model consisted of a two-level logistic model, using isolate and herd as random effects to account for clustering. Moreover, the cow-level clustering was not considered, since a low number of cows contributed to more than one isolate. The second model consisted of a simple logistic regression, to explore the effect of the phenotypic resistance and the same predictor variables, but without the effect of clustering. Interaction was assessed during the model building process, introducing and withdrawing some specific variables to see the change of the magnitude of the coefficients. Furthermore, potential confounding variables were reintroduced and retained if the coefficient varied more than 20%. The model assumptions were examined through residual analysis, and consideration of influential observations and likelihood ratio tests were used to compare both models.
Results
A total of 89 genomes were assembled and annotated through PATRIC pipelines. The assembly metrics are presented in Table S1 in Supplementary Material for all the genomes, along with the accession numbers in the PATRIC resource. Sixteen isolates were removed from the analysis as genomic size was >2,300,000 bp, suggesting non-pure samples. A total of 73 isolates with complete WGS, representing 18 dairy herds from the Maritime region of Canada, were included. The isolates were distributed among Maritime Provinces as follows: 37% of the isolates were collected in Prince Edward Island (PE), 44% in Nova Scotia, and 19% in New Brunswick. From this population of isolates, 76% were S. uberis and 24% were S. dysgalactiae.
Table 1 shows the descriptive statistics of the genomic characteristics for both bacterial species stratified by phenotypic resistant status. The overall frequency of phenotypic resistance (intermediate susceptibility or resistance) of the isolates, to one or more of the eight antimicrobials tested was 22%. The proportion of S. uberis demonstrating phenotypic AMR was 25%, and the proportion of S. dysgalactiae demonstrating phenotypic AMR was 11%.
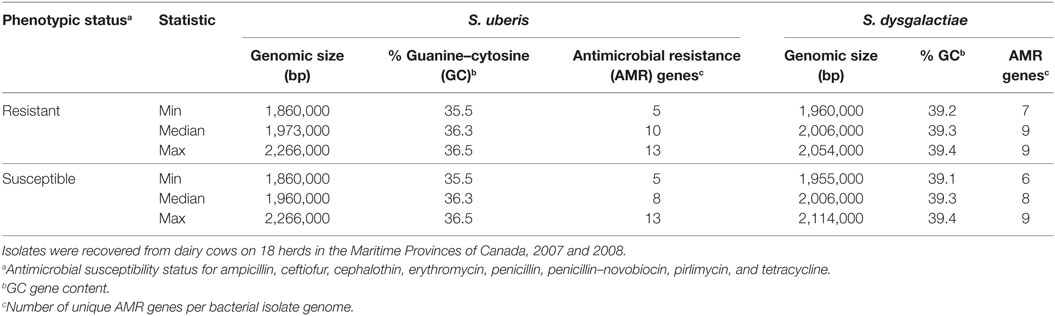
Table 1. Summary of the genomic characteristics of Streptococcus uberis (n = 56) and Streptococcus dysgalactiae (n = 17) isolates by phenotypic antimicrobial susceptibility status.
A total of 23 unique AMR genes were found in the bacterial genomes. There were 10 AMR genes sequences found exclusively in S. uberis genomes and 2 unique gene sequences in the S. dysgalactiae genomes, with the remaining 11 genes shared between both bacterial species (Figure 1). Table 2 shows the occurrence of these genes by bacterial species, the mean identity value, a description of the gene, and the mechanism of resistance. The referred AMR mechanisms were extracted from the Antibiotic Resistance Ontology (26).
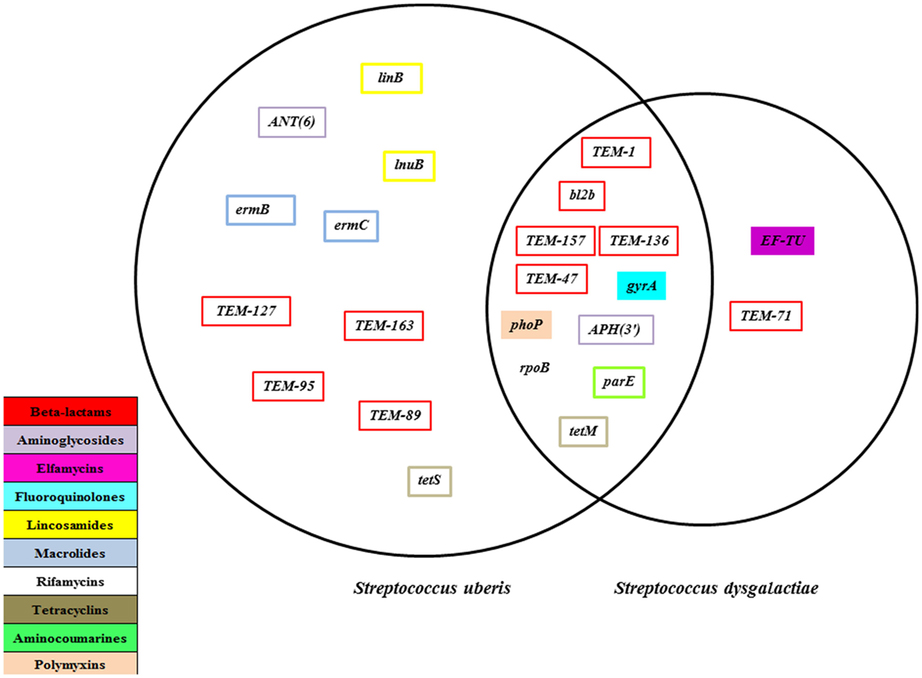
Figure 1. Antimicrobial resistance genes present for Streptococcus uberis (n = 64) and Streptococcus dysgalactiae (n = 25) isolates recovered from dairy herds from the Maritime province of Canada, 2007 and 2008.
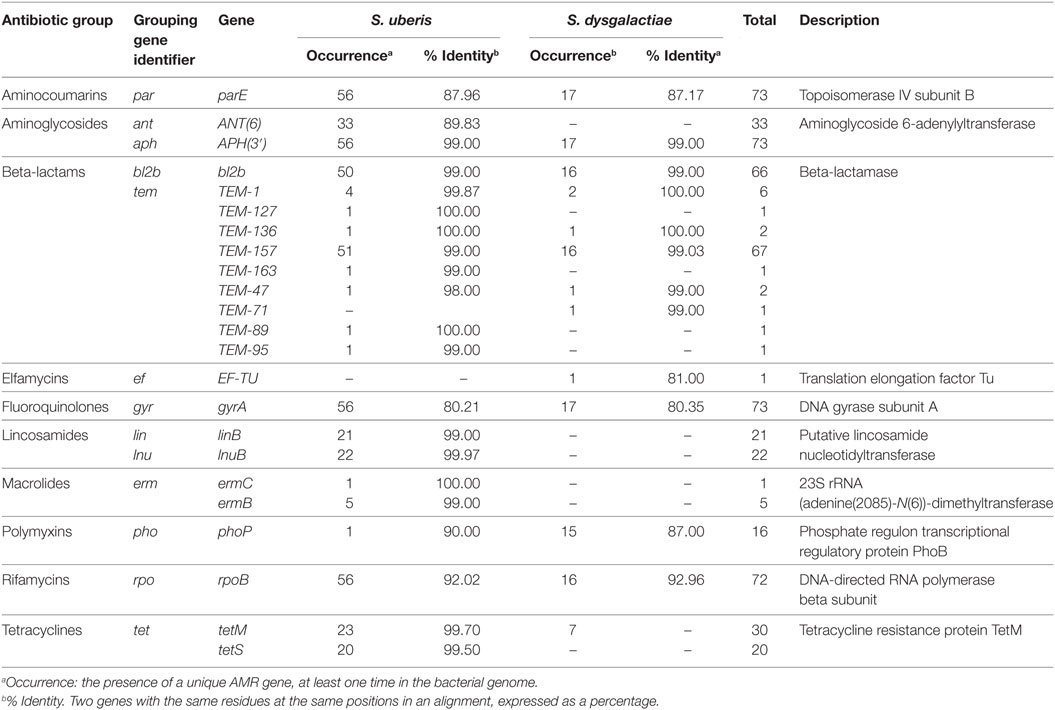
Table 2. Occurrence of antimicrobial resistance (AMR) genes and mean identity values identified in the genomes of 56 isolates of Streptococcus uberis and 17 isolates of Streptococcus dysgalactiae recovered from dairy cows on 18 herds in the Maritime Provinces of Canada, 2007 and 2008.
The distribution of MIC values for each of the eight antimicrobials by AMR gene and bacterial species is presented in Table S2 in Supplementary Material. S. uberis presented higher MICs values than S. dysgalactiae for ampicillin, ceftiofur, cephalothin, and penicillin, with some variation when TEM genes were present. Also a similar result was observed for pirlimycin and tetracycline, when the respective genes were present. Table S3 in Supplementary Material and Table 3 depict the two-way tabulations between phenotypic susceptibility for each antimicrobial and bacterial species and between the absence/presence of specific AMR genes relevant to each antimicrobial type. Moderate to strong associations were observed in S. uberis isolates for ampicillin, ceftiofur, lincosamides, and tetracycline antimicrobials. Moreover, S. dysgalactiae showed only moderate associations for cephalothin, penicillin-novobiocin, and tetracycline. The number of total observations for each combination of genes and antimicrobials varied because some specific genomes did not have the presence of specific genes. There were no significant associations between the phenotypic and genotypic variables among the β-lactam antimicrobials.
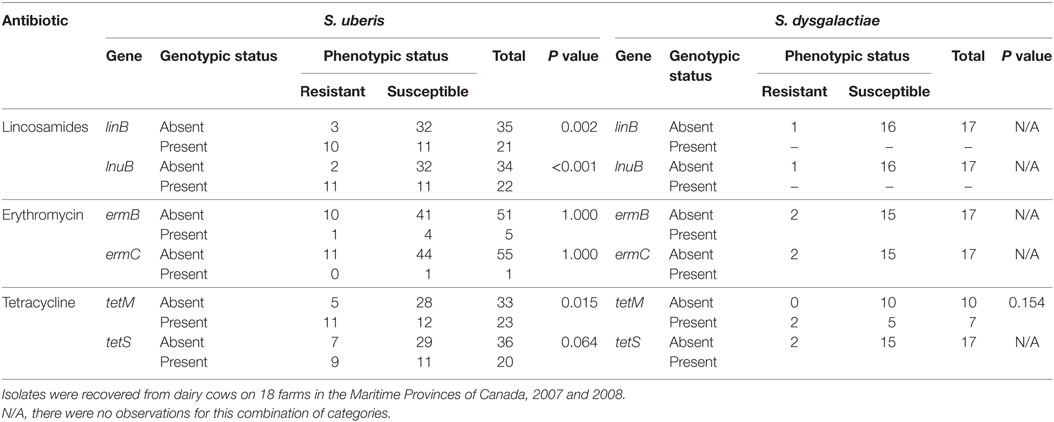
Table 3. Two-way tabulations used for Fischer’s exact test of the phenotypic susceptibility (resistant/susceptible) against three antimicrobials (pirlimycin, erythromycin, and tetracycline) for isolates of Streptococcus uberis (n = 56) and Streptococcus dysgalactiae (n = 17) and the absence/presence of antimicrobial resistance genes categories.
The random effects were not significant when accounting for the predictors in the model; therefore, a simple logistic regression model was used. The final simple logistic model was built with 584 observations (8 antibiotics per isolate) and 73 isolates from 17 herds (Table 4). One herd was not included in neither of the two models, because it had only one isolate with complete information. The number of unique AMR gene sequences was significant (P < 0.001), and the odds of resistance was 4.34 times greater when there were ≥11 AMR genes present in the genome, compared with genomes with <7 AMR genes. The log odds of resistance was lower for S. dysgalactiae than for S. uberis (P = 0.031). Herds with SCC was >250,000 cells/mL and showed a trend toward higher odds of resistance (P = 0.055) compared with the baseline category of <150,000 cells/mL; however, the overall effect of SCC was non-significant (P = 0.159). In addition, the sample type categories were significant (P = 0.0362). When the isolate was recovered from a post-mastitis sample, the resistance was lower when compared with isolates recovered in non-clinical lactating quarters (P = 0.010).
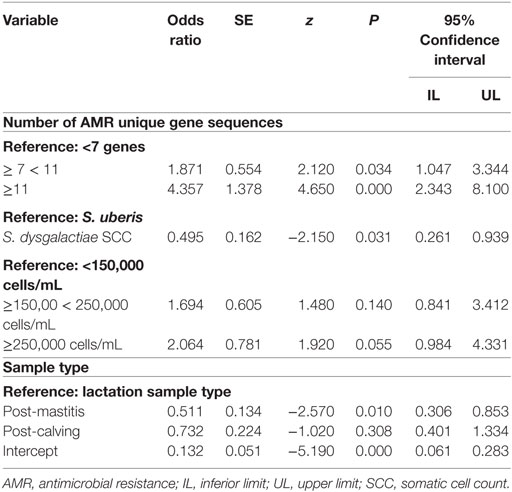
Table 4. Logistic model of AMR against 8 antimicrobials for isolates of Streptococcus uberis (n = 56) and Streptococcus dysgalactiae (n = 17) recovered from dairy cows on 17 farms in the Maritime provinces of Canada (2007 and 2008) adjusted by genomic characteristics, antibiotic tested, and AMR gene presence.
Discussion
The combination of phenotypic data (MIC) and WGS information is a novel approach for the field of udder health studies and provides insight into the AMR potential of two major mastitis pathogens in Canadian dairy farms. The occurrence of AMR genes in the whole genomes of S. uberis and S. dysgalactiae isolates was investigated in relation to phenotypic resistance and with specific epidemiological characteristics. Among both bacterial species, 23 unique AMR genes were detected, distributed differently between S. uberis and S. dysgalactiae isolates, and conferring potential resistance to different antibiotic groups.
AMR Genes
The detection of AMR genes obtained through the PATRIC resource (29) was exhaustive since this tool integrates the two main databases of known AMR genes (ARDB and CARD) (26, 27) with standardized genome functional annotation and comparisons pipelines. In general, the average identity value was high for the AMR genes relevant to specific antibiotics used for mastitis treatment: beta-lactams (99.36%), tetracyclines (99.60%), lincosamides (95.15%), and macrolides (96.48%). These high identities could be suggesting that these genes were likely functional. However, the activation of the two-component regulatory system (including the membrane sensor protein and the cytoplasmic response regulator) is necessary to produce gene expression and to trigger inducible genotypes (30). More studies related with the expression of frequently found that genes are needed to further understand the relationship between the phenotype and the genotype of both pathogens.
Aminocoumarin and Aminoglycosides Resistance Genes
The presence of the parE, APH(3′), and ANT(6) genes has been described in other Gram-positive microorganisms such as S. aureus (31, 32). The parE prevents aminocoumarin antimicrobials from inhibiting DNA synthesis (33). Moreover, APH(3′) and ANT(6) genes inactivate kanamycin and neomycin and are located in transposons in Gram-positive and Gram-negative bacteria (32, 34, 35). In the current study, parE and APH(3′) genes were present in both bacterial species with high identity values. In contrast, the ANT(6) gene was exclusively present in the S. uberis genomes with high identity values. It has been suggested that homologous sequences with more than 40% of identity are likely to share function between them (36). These genes could have been potentially obtained from the dissemination of transposons and plasmids (32) between species cohabiting in the farm environment, especially for S. uberis that behaves more as an opportunistic environmental pathogen and is less adapted to the bovine host (11).
β-Lactams Resistance Genes
One of the most frequent findings in this study was the presence of coding regions for β-lactamase enzymes (bl2b, TEM-1, TEM-127, TEM-136, TEM-157, TEM-163, TEM-47, TEM-71, TEM-89, and TEM-95). In the present study, as presented in Table 2, the TEM variants were more frequent among the S. uberis isolates than in the S. dysgalactiae. In addition, they have been reported as the most common mechanism of β-lactam resistance in clinically important Gram-negative bacteria (37, 38) such as Klebsiella pneumoniae and E. coli (39, 40). The presence of encoding regions for β-lactamases in this population of isolates, which are catalase-negative Gram-positive cocci, is a novel finding. However, further molecular confirmation of the presence of these encoding regions and gene expression are needed.
Elfamycin, Fluoroquinolones, Polymyxin, and Rifamycin Resistance Genes
The presence of elfamycin, fluoroquinolones, polymyxin, and rifamycin resistance genes are presented in Table 2. Elfamycin resistance through the presence of the elongation factor Tu (EF-TU) has been described in species of Staphylococcus, Lactobacillus, and Bacillus (41). However, there is scarce literature regarding elfamycin resistance in streptococci. Also, the EF-TU has been used as a genetic marker to perform phylogenetic analysis among streptococcal species (42). The presence of the DNA gyrase (gyrA) with mutations have been reported in fluoroquinolone-resistant isolates of Streptococcus pneumoniae (43) and Streptococcus pyogenes (44). The existence of gyrA in both S. uberis and S. dysgalactiae genomes indicates that it can be expressed as a housekeeping gene regardless the selective pressure. Fluoroquinolones are antimicrobials of high importance in human medicine but rarely used in dairy herds for bovine mastitis treatment (45).
The RNA polymerase beta subunit (rpoB) mutations can produce rifamycin resistance in E. coli, Mycobacterium tuberculosis, and other microorganisms (46, 47). The high occurrence of this gene in S. uberis shown in Table 2 entails a potential public health risk (i.e., rifamycins are used for human tuberculosis).
Lincosamides, Macrolides, and Tetracyclines Resistance Genes
The resistance to lincosamides through the presence of linB and lnuB genes has been reported among the streptococci (48–50). In our study, both lincosamide resistance genes were found in the S. uberis isolates, but none were identified among the isolates of S. dysgalactiae as presented in Table 2. The association between the presence of these two genes and the phenotypic status can suggest potential resistance to other lincosamides, including pirlimycin.
The ribosomal methylase, encoded by the ermC, ermB, and ermR genes, has been identified as the main determinant of macrolide–lincosamide–streptogramin (MLS) resistance in streptococci, which can be laterally transferred as plasmids between bacteria (48, 51, 52). In the present study, the macrolide–lincosamides resistant genes, such as ermB and ermC, were present only in the S. uberis genomes, similar to the results of Haenni et al. (53), where S. uberis isolates carried the genes linB and linD, implying potential erythromycin and lincomycin phenotypic resistance.
Ribosomal protection proteins genes tetM and tetS have been described as tetracycline resistance mobile elements in Gram-positive and Gram-negative isolates (53–55). In our study, the tetM was present in both bacterial species, and tetS was only present in S. uberis. The association between the presence of tetM and phenotypic status presented in Table 3 in addition to the high-identity mean value (99.5%) could suggest that this gene was expressed within S. uberis isolates.
Both streptococci in this study have been classified in the pyogenic group and occupy diverse ecological niches, with pathogenic and commensal characteristics (56). Also, these two species have been classified as low GC Gram-positive bacteria, also known as firmicutes (57). These bacterial genomes can exhibit niche adaptation characteristics, for example, nucleotide usage trends and GC nucleotide distribution (56, 58).
The genome size could potentially influence the phenotypic and genotypic resistance of a pathogen, and it was worth to be explored in the present study. The comparison of genome sizes can be potentially affected by the presence or absence of AMR genes, since those that provide resistance due to point mutations in housekeeping genes could confound this comparison. The genome size has been hypothesized to be associated with the ability of a particular bacterium to develop antibiotic resistance (59). Nonetheless, in the present study, there were no differences in the genome sizes, or GC content, between susceptible and resistant isolates. According to the hypothesis proposed by Projan (59), organisms with larger genomes are more adaptable to environmental changes, and the differences between S. uberis and S. dysgalactiae isolates can be explained from the proposed “dual nature” (contagious/environmental) of S. dysgalactiae (60, 61). In contrast, S. uberis has been described as an opportunistic environmental pathogen (11) with lower potential for cow to cow transmission than the S. dysgalactiae. The limited survival ability of S. uberis in the environment requires constant reintroduction (56). Moreover, the presence of a single strain type in different cows within a herd can be derived from a predominant environmental strain (11).
The GC content is a bacterial taxonomic marker and can vary between 17 and 75% in bacterial species (62). This variation has been attributed to mutation patterns or selective processes and also environmental adaptation (63–65). Moreover, the GC content has been used for distinguishing genes based on their method of transfer, and it is important to characterize AMR genes and pathogenicity islands originated from the exposure to mobile genetic elements (66). The percentage of genomic GC content has been reported to be associated with genome size and the environmental adaptation of the pathogen (67). However, the GC percentage could be influenced by the total GC content originated from successful AMR mobile elements transfers with variable GC content. This might be a potential indicator of the resistome of the microorganism and its probable origin, which needs further investigation.
Genotypic and Phenotypic Characteristics
Despite the fact that this was mainly a population of susceptible isolates, the presence of β-lactamase enzymes (TEM-157 and bl2b) of plasmid origin (68) was relatively frequent with high MIC values for some specific β-lactams antimicrobials, especially for S. uberis isolates. Interestingly, these β-lactamase enzymes were only previously reported in Gram-negative microorganisms (69). A similar scenario was observed for the MLS antimicrobials (lincosamides and macrolides) where the ermB, linB, and lnuB genes were more frequent among the isolates with higher MIC values. In addition, for both bacterial species, isolates exhibiting tetM and tetS had higher MICs values. The presence of both genes has been previously reported in bovine and human Streptococcus spp. isolates (53, 70). In general, associations between the phenotypic and genotypic characteristics were observed for a limited number of antibiotics for both pathogens (Table 3; Table S3 in Supplementary Material; P < 0.10). However, the majority of isolates showed no association between the phenotypic and genotypic characteristics, for some specific antimicrobials. These results can be explained from different approaches, proposed by Gao et al. (71). The first consideration is that gene expression may or may not be present, depending on the existence of a promoter or inducer (71). The second consideration is the possibility that some of the genes need point mutations to be functional and not because of the presence of the gene by itself (71). The third possibility relates to low statistical power in the present study resulting from the small sample size and low number of observations, among combinations of binary predictor variables, representing the phenotypic and genotypic characteristics.
The analysis of genomic variables with phenotypic characteristics is a novel approach to help researchers understand the indicators of host environment adaptation and AMR resistance frequency in dairy herds. The potential resistome is represented here as the number of unique AMR genes and can be related directly with the capacity of developing phenotypic expression; nonetheless, there is scarce literature related to this finding in bovine mastitis pathogens. The expression level of the AMR genes is a key determinant of phenotypic resistance (72), and needs also to be studied, to develop reliable genome-based AMR prediction tools.
In the multivariable model, there was a proportional increase of the log odds of the phenotypic resistance with the number of unique AMR genes. Having more than 11 AMR genes showed increased odds of being phenotypically resistant, when compared to the baseline category. Despite there were variable associations between phenotypic and genotypic characteristics for specific genes and antimicrobials, the overall effect of the number of unique genes showed a significant association with phenotypic resistance. This finding was similar to a recent study, where high agreement between the gene identification in whole genomes of five different bacterial species and their AMR phenotypic characteristics was reported (73). Another study showed high degree of correlation between phenotypic resistance and the presence of one or more relevant resistance genes to a particular antimicrobial in Campylobacter species isolates (74). Moreover, the variables such as bacterial species (S. uberis compared with S. dysgalactiae), different SCC categories (<150,000 cells/mL, ≥150,000 < 250,000 cells/mL, ≥250,000 cells/mL), and sample type (lactation, post-mastitis, and post-calving) had similar effects over the phenotypic resistance as shown in the study by Cameron et al. (1). The isolates from post-mastitis or post-calving milk samples were more likely to be exposed to antimicrobials derived from mastitis treatment and/or from dry period treatments.
Conclusion
This study showed the distribution of the potential resistome for S. uberis and S. dysgalactiae isolates through WGS analysis. The presence of specific AMR genes showed a trend toward higher MICs values especially for β-lactams and tetracycline antimicrobials. However, there were no statistically significant associations between the phenotypic and genotypic characteristics for β-lactams and macrolides. On the contrary, there was association between the genotypic and phenotypic characteristics, relevant to lincosamides and tetracycline antimicrobials. The results of this research showed the association between phenotypic resistance and the number of unique AMR gene sequences. There were differences in the phenotypic and genotypic resistance between bacterial species. Interestingly, these results showed that subclinical isolates can harbor AMR genes as well and should be considered as potential propagators of the AMR resistance in dairy herds. Some specific AMR genes found in these genomes could be potentially originated from horizontal transfer of mobile elements. In general, more studies are needed to explore the potential sources of these AMR genes, especially in this pyogenic group of pathogens.
Author Contributions
JV was the primary author of this manuscript and was responsible for the bioinformatics and data analysis with the participation of MC, FX, and JS. The rest of the authors were involved in the design, analysis, and interpretation of these results. All the authors reviewed critically the manuscript.
Conflict of Interest Statement
None of the authors of this manuscript have financial or personal relationship with organizations or other people that could influence the content of this study and represent potential conflict of interest.
The reviewer, GM, and handling editor declared their shared affiliation, and the handling editor states that the process nevertheless met the standards of a fair and objective review.
Acknowledgments
Preliminary results of this research were accepted as an abstract at the National Mastitis Council 56th Annual Meeting, St. Pete Beach, FL, USA, January 28–31, 2017. The bacterial isolates used in this study were obtained from the CBMQRN pathogen and data collections. The authors would like to acknowledge to Dr. Leslie Alfaro (Genome BC) for assistance in providing information about the sequencing process and to Dr. Alice R. Wattam (Biocomplexity Institute, Virginia Tech University), Dr. James Davis (University of Chicago), Gordon D. Pusch (Fellowship for Interpretation of Genomes), and Maulik Shukla (Biocomplexity Institute, Virginia Tech University) from the PATRIC-RAST team, for their generous advice in the PATRIC resource and bioinformatics.
Supplementary Material
The Supplementary Material for this article can be found online at http://journal.frontiersin.org/article/10.3389/fvets.2017.00063/full#supplementary-material.
References
1. Cameron M, Saab M, Heider L, McClure JT, Rodriguez-Lecompte JC, Sanchez J. Antimicrobial susceptibility patterns of environmental Streptococci recovered from bovine milk samples in the maritime provinces of Canada. Front Vet Sci (2016) 3:79. doi: 10.3389/fvets.2016.00079
2. Gröhn YT, González RN, Wilson DJ, Hertl JA, Bennett G, Schulte H, et al. Effect of pathogen-specific clinical mastitis on herd life in two New York State dairy herds. Prev Vet Med (2005) 71:105–25. doi:10.1016/j.prevetmed.2005.06.002
3. Barkema HW, Schukken YH, Lam TJGM, Beiboer ML, Wilmink H, Benedictus G, et al. Incidence of clinical mastitis in dairy herds grouped in three categories by bulk milk somatic cell counts. J Dairy Sci (1998) 81:411–9. doi:10.3168/jds.S0022-0302(98)75591-2
4. Gröhn YT, Wilson DJ, González RN, Hertl JA, Schulte H, Bennett G, et al. Effect of pathogen-specific clinical mastitis on milk yield in dairy cows. J Dairy Sci (2004) 87:3358–74. doi:10.3168/jds.S0022-0302(04)73472-4
5. Blowey R, Edmondson P. Mastitis Control in Dairy Herds (Electronic Resource). Wallingford: CABI (2010).
6. Lundberg A, Nyman A, Unnerstad HE, Waller KP. Prevalence of bacterial genotypes and outcome of bovine clinical mastitis due to Streptococcus dysgalactiae and Streptococcus uberis. Acta Vet Scand (2014) 56:80. doi:10.1186/s13028-014-0080-0
7. Wyder AB, Boss R, Naskova J, Kaufmann T, Steiner A, Graber HU. Streptococcus spp. and related bacteria: their identification and their pathogenic potential for chronic mastitis – a molecular approach. Res Vet Sci (2011) 91:349–57. doi:10.1016/j.rvsc.2010.09.006
8. Raemy A, Meylan M, Casati S, Gaia V, Berchtold B, Boss R, et al. Phenotypic and genotypic identification of streptococci and related bacteria isolated from bovine intramammary infections. Acta Vet Scand (2013) 55:53. doi:10.1186/1751-0147-55-53
9. Olde Riekerink RGM, Barkema HW, Kelton DF, Scholl DT. Incidence rate of clinical mastitis on Canadian dairy farms. J Dairy Sci (2008) 91:1366–77. doi:10.3168/jds.2007-0757
10. Abureema S, Smooker P, Malmo J, Deighton M. Molecular epidemiology of recurrent clinical mastitis due to Streptococcus uberis: evidence of both an environmental source and recurring infection with the same strain. J Dairy Sci (2014) 97:285–90. doi:10.3168/jds.2013-7074
11. Zadoks RN, Tikofsky LL, Boor KJ. Ribotyping of Streptococcus uberis from a dairy’s environment, bovine feces and milk. Vet Microbiol (2005) 109:257–65. doi:10.1016/j.vetmic.2005.05.008
12. Barkema HW, Schukken YH, Lam TJGM, Beiboer ML, Benedictus G, Brand A. Management practices associated with the incidence rate of clinical mastitis. J Dairy Sci (1999) 82:1643–54. doi:10.3168/jds.S0022-0302(99)75393-2
13. Todhunter DA, Smith KL, Hogan JS. Environmental streptococcal intramammary infections of the bovine mammary gland. J Dairy Sci (1995) 78:2366–74. doi:10.3168/jds.S0022-0302(95)76864-3
14. Calvinho LF, Almeida RA, Oliver SP. Potential virulence factors of Streptococcus dysgalactiae associated with bovine mastitis. Vet Microbiol (1998) 61:93–110. doi:10.1016/S0378-1135(98)00172-2
15. Oliver SP, Murinda SE. Antimicrobial resistance of mastitis pathogens. Vet Clin North Am Food Anim Pract (2012) 28:165–85. doi:10.1016/j.cvfa.2012.03.005
16. Minst K, Märtlbauer E, Miller T, Meyer C. Short communication: Streptococcus species isolated from mastitis milk samples in Germany and their resistance to antimicrobial agents. J Dairy Sci (2012) 95:6957–62. doi:10.3168/jds.2012-5852
17. Punina NV, Makridakis NM, Remnev MA, Topunov AF. Whole-genome sequencing targets drug-resistant bacterial infections. Hum Genomics (2015) 9:19. doi:10.1186/s40246-015-0037-z
18. Woolhouse M, Ward M, van Bunnik B, Farrar J. Antimicrobial resistance in humans, livestock and the wider environment. Phil Trans R Soc Lond B Biol Sci (2015) 370:20140083. doi:10.1098/rstb.2014.0083
19. Blum S, Sela N, Heller ED, Sela S, Leitner G. Genome analysis of bovine-mastitis-associated Escherichia coli O32:H37 strain P4. J Bacteriol (2012) 194:3732. doi:10.1128/JB.00535-12
20. Silva DM, da Silva MP, Vidigal PMP, Barcelos RM, Klein RC, Aguilar AP, et al. Draft genome sequences of Staphylococcus aureus strains isolated from subclinical bovine mastitis in Brazil. Genome Announc (2016) 4:e01594–15. doi:10.1128/genomeA.01594-15
21. Hossain M, Egan SA, Coffey T, Ward PN, Wilson R, Leigh JA, et al. Virulence related sequences; insights provided by comparative genomics of Streptococcus uberis of differing virulence. BMC Genomics (2015) 16:334. doi:10.1186/s12864-015-1512-6
22. Suzuki H, Lefebure T, Hubisz MJ, Pavinski Bitar P, Lang P, Siepel A, et al. Comparative genomic analysis of the Streptococcus dysgalactiae species group: gene content, molecular adaptation, and promoter evolution. Genome Biol Evol (2011) 3:168–85. doi:10.1093/gbe/evr006
23. Reyher KK, Dufour S, Barkema HW, Des Côteaux L, DeVries TJ, Dohoo IR, et al. The National Cohort of dairy farms – a data collection platform for mastitis research in Canada. J Dairy Sci (2011) 94:1616–26. doi:10.3168/jds.2010-3180
24. Li H, Handsaker B, Wysoker A, Fennell T, Ruan J, Homer N, et al. The sequence alignment/map format and SAMtools. Bioinformatics (2009) 25:2078–9. doi:10.1093/bioinformatics/btp352
25. Wattam AR, Davis JJ, Assaf R, Boisvert S, Brettin T, Bun C, et al. Improvements to PATRIC, the all-bacterial Bioinformatics Database and Analysis Resource Center. Nucleic Acids Res (2017) 45:D535–42. doi:10.1093/nar/gkw1017
26. McArthur AG, Waglechner N, Nizam F, Yan A, Azad MA, Baylay AJ, et al. The comprehensive antibiotic resistance database. Antimicrob Agents Chemother (2013) 57:3348–57. doi:10.1128/AAC.00419-13
27. Liu B, Pop M. ARDB – antibiotic resistance genes database. Nucleic Acids Res (2009) 37:D443–7. doi:10.1093/nar/gkn656
29. Wattam AR, Abraham D, Dalay O, Disz TL, Driscoll T, Gabbard JL, et al. PATRIC, the bacterial bioinformatics database and analysis resource. Nucleic Acids Res (2013) 42:D581–91. doi:10.1093/nar/gkt1099
30. Depardieu F, Podglajen I, Leclercq R, Collatz E, Courvalin P. Modes and modulations of antibiotic resistance gene expression. Clin Microbiol Rev (2007) 20:79–114. doi:10.1128/CMR.00015-06
31. Fujimoto-Nakamura M, Ito H, Oyamada Y, Nishino T, Yamagishi J. Accumulation of mutations in both gyrB and parE genes is associated with high-level resistance to novobiocin in Staphylococcus aureus. Antimicrob Agents Chemother (2005) 49:3810–5. doi:10.1128/AAC.49.9.3810-3815.2005
32. Ramirez MS, Tolmasky ME. Aminoglycoside modifying enzymes. Drug Resist Updat (2010) 13:151–71. doi:10.1016/j.drup.2010.08.003
33. Schmutz E, Mühlenweg A, Li S-M, Heide L. Resistance genes of aminocoumarin producers: two type II topoisomerase genes confer resistance against coumermycin A1 and clorobiocin. Antimicrob Agents Chemother (2003) 47:869–77. doi:10.1128/AAC.47.3.869-877.2003
34. Shaw KJ, Rather PN, Hare RS, Miller GH. Molecular genetics of aminoglycoside resistance genes and familial relationships of the aminoglycoside-modifying enzymes. Microbiol Rev (1993) 57:138–63.
35. Wright GD, Thompson PR. Aminoglycoside phosphotransferases: proteins, structure, and mechanism. Front Biosci (1999) 4:D9–21. doi:10.2741/A408
36. Pearson WR. An introduction to sequence similarity (“homology”) searching. Curr Protoc Bioinformatics (2013) 3:27:3.1:3.1.1–3.1.7. doi:10.1002/0471250953.bi0301s42
37. Bush K, Jacoby GA. Updated functional classification of β-lactamases. Antimicrob Agents Chemother (2010) 54:969–76. doi:10.1128/AAC.01009-09
38. Kong K-F, Schneper L, Mathee K. Beta-lactam antibiotics: from antibiosis to resistance and bacteriology. APMIS (2010) 118:1–36. doi:10.1111/j.1600-0463.2009.02563.x
39. Medeiros AA. Evolution and dissemination of β-lactamases accelerated by generations of β-lactam antibiotics. Clin Infect Dis (1997) 24:S19–45. doi:10.1093/clinids/24.Supplement_1.S19
40. Jeong SH, Bae IK, Lee JH, Sohn SG, Kang GH, Jeon GJ, et al. Molecular characterization of extended-spectrum beta-lactamases produced by clinical isolates of Klebsiella pneumoniae and Escherichia coli from a Korean nationwide survey. J Clin Microbiol (2004) 42:2902–6. doi:10.1128/JCM.42.7.2902-2906.2004
41. Miele A, Goldstein BP, Bandera M, Jarvis C, Resconi A, Williams RJ. Differential susceptibilities of enterococcal species to elfamycin antibiotics. J Clin Microbiol (1994) 32:2016–8.
42. Picard FJ, Ke D, Boudreau DK, Boissinot M, Huletsky A, Richard D, et al. Use of tuf sequences for genus-specific PCR detection and phylogenetic analysis of 28 streptococcal species. J Clin Microbiol (2004) 42:3686–95. doi:10.1128/JCM.42.8.3686-3695.2004
43. Eliopoulos GM. Quinolone resistance mechanisms in pneumococci. Clin Infect Dis (2004) 38:S350–6. doi:10.1086/382709
44. Arai K, Hirakata Y, Yano H, Kanamori H, Endo S, Hirotani A, et al. Emergence of fluoroquinolone-resistant Streptococcus pyogenes in Japan by a point mutation leading to a new amino acid substitution. J Antimicrob Chemother (2010) 66(3):494–8. doi:10.1093/jac/dkq477
45. Saini V, McClure JT, Léger D, Dufour S, Sheldon AG, Scholl DT, et al. Antimicrobial use on Canadian dairy farms. J Dairy Sci (2012) 95:1209–21. doi:10.3168/jds.2011-4527
46. Mariam DH, Mengistu Y, Hoffner SE, Andersson DI. Effect of rpoB mutations conferring rifampin resistance on fitness of Mycobacterium tuberculosis. Antimicrob Agents Chemother (2004) 48:1289–94. doi:10.1128/AAC.48.4.1289-1294.2004
47. Floss HG, Yu T-W. Rifamycin-mode of action, resistance, and biosynthesis. Chem Rev (2005) 105:621–32. doi:10.1021/cr030112j
48. Schmitt-van de Leemput E, Zadoks RN. Genotypic and phenotypic detection of macrolide and lincosamide resistance in Streptococcus uberis. J Dairy Sci (2007) 90:5089–96. doi:10.3168/jds.2007-0101
49. Almuzara M, Bonofiglio L, Cittadini R, Vera Ocampo C, Montilla A, del Castillo M, et al. First case of Streptococcus lutetiensis bacteremia involving a clindamycin-resistant isolate carrying the lnuB gene. J Clin Microbiol (2013) 51:4259–61. doi:10.1128/JCM.01774-13
50. Morar M, Bhullar K, Hughes DW, Junop M, Wright GD. Structure and mechanism of the lincosamide antibiotic adenylyltransferase LinB. Structure (2009) 17:1649–59. doi:10.1016/j.str.2009.10.013
51. Shivakumar AG, Dubnau D. Characterization of a plasmid-specified ribosome methylase associated with macrolide resistance. Nucleic Acids Res (1981) 9:2549–62. doi:10.1093/nar/9.11.2549
52. Mayford M, Weisblum B. The ermC leader peptide: amino acid alterations leading to differential efficiency of induction by macrolide-lincosamide-streptogramin B antibiotics. J Bacteriol (1990) 172:3772–9. doi:10.1128/jb.172.7.3772-3779.1990
53. Haenni M, Saras E, Bertin S, Leblond P, Madec J-Y, Payot S. Diversity and mobility of integrative and conjugative elements in bovine isolates of Streptococcus agalactiae, S. dysgalactiae subsp. dysgalactiae, and S. uberis. Appl Environ Microbiol (2010) 76:7957–65. doi:10.1128/AEM.00805-10
54. Akhtar M, Hirt H, Zurek L. Horizontal transfer of the tetracycline resistance gene tetM mediated by pCF10 among Enterococcus faecalis in the house fly (Musca domestica L.) alimentary canal. Microb Ecol (2009) 58:509–18. doi:10.1007/s00248-009-9533-9
55. Charpentier E, Gerbaud G, Courvalin P. Presence of the Listeria tetracycline resistance gene tet(S) in Enterococcus faecalis. Antimicrob Agents Chemother (1994) 38:2330–5. doi:10.1128/AAC.38.10.2330
56. Ward PN, Holden MT, Leigh JA, Lennard N, Bignell A, Barron A, et al. Evidence for niche adaptation in the genome of the bovine pathogen Streptococcus uberis. BMC Genomics (2009) 10:54. doi:10.1186/1471-2164-10-54
57. Fraser C, Read T, Nelson K. Microbial Genomes (Infectious Disease). 1st ed. Totowa, NJ: Humana Press (2004).
58. Mann S, Chen Y-PP. Bacterial genomic G + C composition-eliciting environmental adaptation. Genomics (2010) 95:7–15. doi:10.1016/j.ygeno.2009.09.002
59. Projan SJ. (Genome) size matters. Antimicrob Agents Chemother (2007) 51:1133–4. doi:10.1128/AAC.01370-06
60. Oliver SP, Gillespie BE, Jayarao BM. Detection of new and persistent Streptococcus uberis and Streptococcus dysgalactiae intramammary infections by polymerase chain reaction-based DNA fingerprinting. FEMS Microbiol Lett (1998) 160:69–73. doi:10.1111/j.1574-6968.1998.tb12892.x
61. Zadoks RN, Middleton JR, McDougall S, Katholm J, Schukken YH. Molecular epidemiology of mastitis pathogens of dairy cattle and comparative relevance to humans. J Mammary Gland Biol Neoplasia (2011) 16:357–72. doi:10.1007/s10911-011-9236-y
62. Nishida H. Comparative analyses of base compositions, DNA sizes, and dinucleotide frequency profiles in archaeal and bacterial chromosomes and plasmids. Int J Evol Biol (2012) 2012:342482. doi:10.1155/2012/342482
63. Foerstner KU, von Mering C, Hooper SD, Bork P. Environments shape the nucleotide composition of genomes. EMBO Rep (2005) 6:1208–13. doi:10.1038/sj.embor.7400538
64. Hildebrand F, Meyer A, Eyre-Walker A. Evidence of selection upon genomic GC-content in bacteria. PLoS Genet (2010) 6:e1001107. doi:10.1371/journal.pgen.1001107
65. Brocchieri L. The GC content of bacterial genomes. J Phylogenetics Evol Biol (2014) 2:e108. doi:10.4172/2329-9002.1000e108
66. Hayek N. Lateral transfer and GC content of bacterial resistance genes. Front Microbiol (2013) 4:41. doi:10.3389/fmicb.2013.00041
67. Lassalle F, Périan S, Bataillon T, Nesme X, Duret L, Daubin V. GC-content evolution in bacterial genomes: the biased gene conversion hypothesis expands. PLoS Genet (2015) 11:e1004941. doi:10.1371/journal.pgen.1004941
68. Liu G, Ling B-D, Zeng Y, Lin L, Xie Y-E, Lei J. Molecular characterization of extended-spectrum beta-lactamases produced by clinical isolates of Enterobacter cloacae from a Teaching Hospital in China. Jpn J Infect Dis (2008) 61:286–9.
69. Li X-Z, Mehrotra M, Ghimire S, Adewoye L. β-Lactam resistance and β-lactamases in bacteria of animal origin. Vet Microbiol (2007) 121:197–214. doi:10.1016/j.vetmic.2007.01.015
70. Ruegg PL, Oliveira L, Jin W, Okwumabua O. Phenotypic antimicrobial susceptibility and occurrence of selected resistance genes in Gram-positive mastitis pathogens isolated from Wisconsin dairy cows. J Dairy Sci (2015) 98:4521–34. doi:10.3168/jds.2014-9137
71. Gao J, Yu F-Q, Luo L-P, He J-Z, Hou R-G, Zhang H-Q, et al. Antibiotic resistance of Streptococcus agalactiae from cows with mastitis. Vet J (2012) 194:423–4. doi:10.1016/j.tvjl.2012.04.020
72. Perry JA, Westman EL, Wright GD. The antibiotic resistome: what’s new? Curr Opin Microbiol (2014) 21:45–50. doi:10.1016/j.mib.2014.09.002
73. Zankari E, Hasman H, Cosentino S, Vestergaard M, Rasmussen S, Lund O, et al. Identification of acquired antimicrobial resistance genes. J Antimicrob Chemother (2012) 67(11):2640–4. doi:10.1093/jac/dks261
Keywords: Streptococcus uberis, Streptococcus dysgalactiae, antimicrobial resistance, bovine mastitis, whole-genome sequence
Citation: Vélez JR, Cameron M, Rodríguez-Lecompte JC, Xia F, Heider LC, Saab M, McClure JT and Sánchez J (2017) Whole-Genome Sequence Analysis of Antimicrobial Resistance Genes in Streptococcus uberis and Streptococcus dysgalactiae Isolates from Canadian Dairy Herds. Front. Vet. Sci. 4:63. doi: 10.3389/fvets.2017.00063
Received: 16 December 2016; Accepted: 19 April 2017;
Published: 22 May 2017
Edited by:
Julio Alvarez, University of Minnesota, USACopyright: © 2017 Vélez, Cameron, Rodríguez-Lecompte, Xia, Heider, Saab, McClure and Sánchez. This is an open-access article distributed under the terms of the Creative Commons Attribution License (CC BY). The use, distribution or reproduction in other forums is permitted, provided the original author(s) or licensor are credited and that the original publication in this journal is cited, in accordance with accepted academic practice. No use, distribution or reproduction is permitted which does not comply with these terms.
*Correspondence: Julián Reyes Vélez, anJleWVzJiN4MDAwNDA7dXBlaS5jYQ==