- 1Noguchi Memorial Institute for Medical Research, University of Ghana, Accra, Ghana
- 2Medical Research Council Unit The Gambia (MRCG) at the London School of Hygiene and Tropical Medicine (LSHTM), Banjul, Gambia
- 3Institute for Environment and Sanitation Studies, University of Ghana, Accra, Ghana
- 4Department of Microbial Pathogens and Immunology, Rush University Medical Center, Chicago, IL, United States
- 5Department of Biochemistry, Cell and Molecular Biology, University of Ghana, Accra, Ghana
Tuberculosis (TB), caused by Mycobacterium tuberculosis complex (MTBC), remains a global health burden, claiming millions of lives annually. Despite the availability of a vaccine (the Bacillus Calmette-Guérin; BCG), diagnostics (smear microscopy and DNA-based diagnostics) and drugs for treatment, challenges like drug resistance, lower diagnostic sensitivity such as microscopy, instrumentation and high-level operational expertise requirement and poor vaccine efficacy pose a greater hinderance to TB monitoring and control. The inability of the BCG vaccine to protect against TB other than disseminated disease in neonates call for innovative approaches to provide improved interventions. This review highlights the potential of multi-omics as a powerful emerging tool offering a holistic insight into the intricate interplay between pathogens and the host immune response. It discusses the potential of multi-omics for discovering biomarkers as targets for rational drug design, production of more effective vaccines and as stable targets for production of universally applicable rapid diagnostics for early and accurate TB detection as well as monitoring treatment.
Background
Tuberculosis (TB) remains a significant global health burden, affecting about 7.5 million people while claiming approximately 1.3 million lives in 2022 alone (1). The World Health Organization (WHO) End TB Strategy aims to reduce TB incidence by 90% and deaths by 95% by 2035 (2). Efforts including prevention of infection through vaccination, rapid diagnosis and treatment of diagnosed cases, toward the attainment of these goals have met some challenges. Whereas the complex interplay of host-pathogen interactions affects the usefulness of the only WHO approved Bacillus Calmette-Guérin (BCG) vaccine other than protection of neonates against disseminated TB, there is limited impact of current diagnostic tools (including low sensitivity and specificity of sputum-smear microscopy, long turnaround time for sputum cultures and high instrumentation need and/or expertise requirement for DNA-based diagnostics coupled with their inability to be used for monitoring treatment). Moreso, the emergence of drug-resistant (DR) strains of the TB pathogen, threatens to make TB an untreatable disease. To overcome these obstacles, a paradigm shift toward innovative approaches is crucial. Multi-omics, the integration of multiple biological datasets (genomics, transcriptomics, proteomics, metabolomics, etc.), offers a promising approach for discovery of novel biomarkers for vaccine development, enhanced TB diagnostics (including treatment monitoring) and rationale drug development.
Tuberculosis, caused by the Mycobacterium tuberculosis complex (MTBC) is a group of closely related acid-fast bacilli, primarily affects the lungs but can spread to other organs. Despite the availability of effective treatment, TB remains the leading cause of death from a single infectious agent until the outbreak of COVID-19 (1).
BCG vaccine, the only licensed TB vaccine, has shown variable efficacy in preventing TB, especially in adults (3). This variability has been attributed to several factors, including waning immunity over time, regional variations in circulating MTBC genotypes, and prior exposure to environmental mycobacteria (4, 5). The failure of BCG vaccine to provide consistent protection has contributed to exacerbating the global TB burden. This is particularly true in settings of high-incidence of TB, which hinders progress toward TB elimination goals (1, 2). This lack of complete protection by the only existing vaccine has necessitated the continuous reliance on diagnostics and complex treatment regimens, which are challenged by drug resistance and limited resources (1). Treatment of uncomplicated TB takes up to six months and this extended treatment duration affects compliance leading to defaulting and its associated development of drug resistance (6). The emergence of drug resistant (DR) TB, particularly multidrug-resistant (MDR-TB) and extensively drug-resistant (XDR-TB) strains, poses a significant threat to global TB control efforts (7). Treatment of DR-TB with the current drugs is expensive, with associated adverse effects and relatively low treatment success resulting in poor treatment adherence and high mortality rates (8). To accelerate attaining the End TB Strategy by 2035, the need for new potent TB drugs to shorten the treatment course is warranted. The introduction of DNA-based diagnostics including the GeneXpert and line-probe assays at diagnostics and referral laboratories have led to simultaneous increased in accuracy of TB diagnostics and detection of drug resistant cases, compared to the use sputum smear microscopy and culture. Nevertheless, culture remains the choice tool for monitoring treatment albeit limited reduced sensitivity, specificity and long turnaround time (9, 10). On the contrary, the tuberculin skin test (TST) and interferon-gamma release assays (IGRAs) detect latent TB infection (LTBI) but cannot distinguish between active TB disease and LTBI (11). There is an urgent need for accurate and rapid diagnostic tests to enable early detection of active TB and prompt initiation of appropriate treatment as well as monitor treatment.
Multi-omics approaches offer unmatched prospects to unravel the complex molecular mechanisms underlying TB pathogenesis, drug resistance, and host response (12). By integrating data from different biological levels, multi-omics can provide a comprehensive picture of the molecular landscape of TB, identifying novel biomarkers with diagnostic, prognostic, and therapeutic potentials (13). Multi-omics can identify host and/or pathogen biomarkers that differentiate between active TB and LTBI, predict risk of disease progression and essential bacteria genes and/or enzymes, or detect DR-TB associated markers early in the course of infection. These biomarkers could serve as targets for developing rapid, non-invasive diagnostics for rational drug design and, development of new vaccines (14). Several studies have demonstrated the potential of multi-omics in TB biomarker discovery. For instance, metabolomics identification of host metabolites associated with active TB and treatment response (15, 16). Transcriptomic discovery of gene expression signatures associated with DR-TB (17) and proteomic identification of peptides for potential TB diagnosis and monitoring (18, 19). Multi-omics hold immense potential for revolutionizing TB control. Multiple omics datasets can be integrated with machine learning to enhance biomarker discovery by providing a more comprehensive understanding of the complex molecular interactions in TB to identify subtle patterns and correlations that may not be apparent through traditional statistical methods (14). By identifying novel biomarkers and shedding light on the molecular mechanisms underlying TB pathogenesis, multi-omics can pave the way for development of potent vaccine, better diagnostics, personalized treatment strategies, and ultimately, more effective interventions to combat this global health threat.
The power of multi-omics
Traditional research on TB often focuses on single “omes,” such as the genome or proteome, providing a limited window into complex biological systems. Multi-omics on the other hand takes a synergistic approach by integrating data from various “omes” including genomics (study of the complete genetic makeup of an organism), transcriptomics (analysis of total expressed genes (mainly mRNA) by the organism under specified conditions), proteomics (characterization of total protein abundance and modifications) and metabolomics (study of metabolites, the small molecules produced by cellular processes). By analyzing data on an organism under specified conditions from these diverse platforms, researchers gain comprehensive understanding of the organism under investigation which wouldn’t be possible by analyzing individual omics data sets in isolation (14, 20, 21). As shown below (Figure 1), data integration from multi-omics platforms can lead to better understanding of pathogenesis and host-pathogen interactions at different stages of TB infection/disease progression. While identifying genes, peptides and/or metabolites that play key functions at these stages. Such identified genes, peptides and/or metabolites can serve as potential biomarkers for new improved diagnostics, rational drug design and/or development of potent vaccines for control of TB.
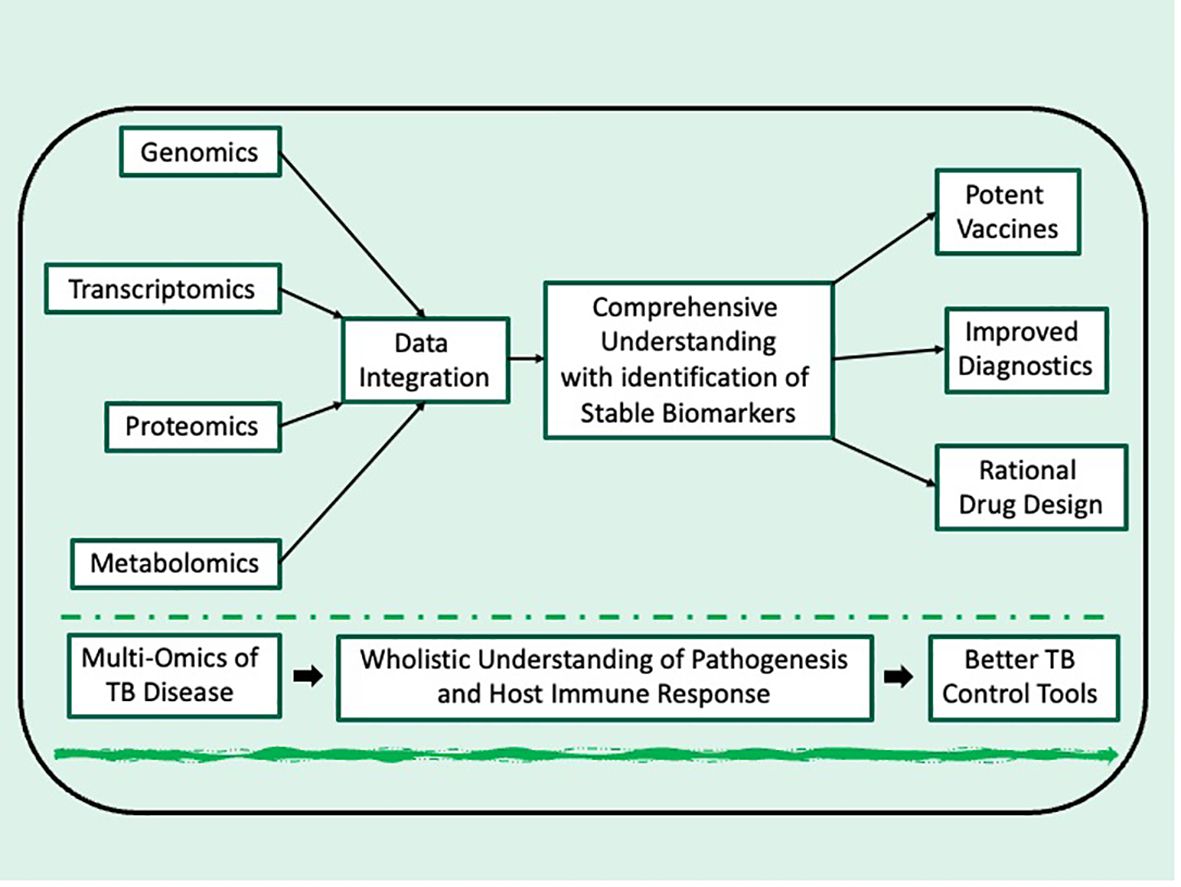
Figure 1 Schematic depiction of integrating multi-omics for TB research toward development of improved control tools.
Improved TB diagnostics
Early and accurate TB diagnosis is crucial for prompt treatment and transmission prevention. Multi-omics approaches can facilitate the development of rapid, and reliable diagnostic tools (high specificity and sensitivity) by discovering of novel host and pathogen biomarkers that are associated with TB infection. This can be done by 1)non-targeted omics comparison of whole transcriptomes, proteomes and metabolomes of target biological specimen such as serum, urine and/or stool of confirmed TB patients to those from healthy individuals 2) non-targeted omics comparison of whole genome, transcriptomes, proteomes and metabolomes from MTBC spanning all the identified phylogenetic lineages and other non-MTBC mycobacteria, zooming unto markers present in each phylogenetic lineage but absent in all non-MTBC mycobacteria (13, 22–25). These biomarkers can be used to develop non-invasive rapid diagnostic tests that do not only rely on sputum but other biological specimen such as stool, urine and/or blood (26–28). Such diagnostics should not require high expertise and/or instrumentation and should be applicable at point of care especially for TB patients coinfected with HIV and children who are mostly associated with difficulty in producing good quality sputum which negatively affect sputum-based diagnostics (29, 30). Once such diagnostics are developed, they would have to be evaluated in different geographical regions of the world because of the unique phylogeography of the MTBC to ensure their universal specificity. This evaluation must be supported by targeted multi-omics profiling of the indicator biomarkers.
Rational TB drug production
The emergence of DR-MTBC strains necessitates the discovery of novel therapeutics. Multi-omics strategies can contribute significantly to this critical endeavor through rapid identification of new druggable targets and evaluating efficacy and/or safety of administered drug candidates and determining the mode of action of drug candidates to hasten development approval (31). Transcriptomics, proteomics and metabolomics have been used to identify essential MTBC genes and proteins that are critical for the pathogens survival and/or virulence (32–37). Such identified markers can be targeted through rational drug design such BacPROTACs to disrupt critical MTBC pathways (38, 39), hindering its growth or replication. For example, after identification of MTBC KasA as potential drug target (40–42), several studies reviewed previously by Rudraraju et al. have used diverse omics approaches to screen for KasA inhibitors as potential candidates for drug development (43). The metabolic perturbations induced by drug candidates in MTBC can provide insights into their potential effectiveness, safety and mode of action (44), hence guiding the prioritization of leads with the most promising activity against the pathogen but safe for the host and potentially reducing the risk of resistance development.
TB vaccine development
Effective vaccines are crucial for preventing TB infection and/or disease. Multi-omics strategies are aiding in the development of improved vaccines by helping to understand host immune responses and analyzing the response to trial and approved vaccines. Transcriptomics and proteomics have been utilized in identifying immune signatures (e.g., antibodies, T-cells) crucial for protective immunity against MTBC infection (45–47). Such discoveries can inform selection of potential vaccine antigens capable of eliciting desired immunological responses, which could be accessed using a desired omics platform such as transcriptomics and/or proteomics (48–50). For example, transcriptomics have been used to identify T-cell responses associated with protection against TB in animal models, paving the way for the development of vaccines targeting these specific T-cell populations (51, 52). On the other hand, metabolomics was used to predict trained immunity in response to BCG vaccination (53). By analyzing the immune response to vaccines under trials, researchers can identify potential limitations and refine vaccine design to enhance their efficacy and overcome existing challenges. Since conventional immunologic assays are time consuming and very expensive (54–56), employing multi-omics approaches can efficiently deliver much better outcome at a shorter turnaround time and at relatively reduced cost (57). For example, multi-omics have been used to identify the core vaccine targets for Campylobacter jenuni (58) and in another to reveal attenuated inflammatory responses induced by COVID-19 vaccination (59). In TB research however, multi-omics was used to identify the epigenetic cellular states associated with trained immunity in response to BCG vaccination (60). With the failure of the BCG vaccine to protect against TB other than disseminated TB in neonates, it is imperative that current and future TB vaccine developments capitalize on efficiency of mult-omics to hasten the process as well as produce adjuvants, vaccine candidates and vaccine formulations that are more potent than the BCG vaccine.
Challenges of multi-omics applications in TB and future directions
Despite the significant progress made in utilizing multi-omics for TB research, several challenges remain including data complexity, standardization of data and sharing, translation of scientific findings to clinical applications and policy (61–63).
Firstly, different omics technologies (genomics, transcriptomics, proteomics, metabolomics, etc.) produce data with varying formats, scales, and noise levels. Integrating these heterogeneous data types into a coherent framework is technically challenging requiring sophisticated bioinformatics tools and expertise. This necessitates the development of user-friendly analytical tools and the training of researchers in advanced data analysis techniques to unlock the full potential of multi-omics data. However, transitioning from wet lab basic research assays to learning computer programming languages is not an easy feat. There are several commercially available omics analytics platforms but the subscription fees are quite expensive and not sustainable for less endowed TB research teams (64, 65). Perhaps the best way around this is to train young and upcoming scientists to take up courses in bioinformatics, computer engineering and/or computer science or employ already experienced bioinformaticians to beef up the data handling aspect of TB labs. However, this endeavor is very expensive and remains critical challenge for most TB research groups especially those in resource limited regions of the world who are also the most burden with TB. Nevertheless, collaboration of such TB labs with well-resourced international TB research groups who have competent multi-omics expertise would be a step in the right direction to assist the struggling labs analyzing their multi-omics data as well as build omics capacity for the future (66). It also important to note the omics capacity building activities of regional institutions and research collaborative networks such as the AfCDC (African Center for Disease Control), H3Africa (Human Heredity and Health in Africa), WANETAM (West Africa Network of Excellence for Tuberculosis, Aids and Malaria), PANDORA-ID-Net (Pan-African Network For Rapid Research, Response, Relief and Preparedness for Infectious Disease Epidemics) and PANGenS (Pan-Africa Network for Genomic Surveillance of Poverty Related Disease and Emerging Pathogens) in Sub-Saharan Africa which is one of the high TB burdened WHO regions are commendable and can be emulated by other resource limited regions (67–70).
Another multi omics challenge is the lack of standardized protocols for sample handling and data generation across different laboratories which can lead introduction of inconsistent variabilities and/or biases into the resulting data, making it difficult to compare and integrate datasets from different sources. However, high quality and consistent data are crucial for reliable multi-omics analyses. Therefore, establishing standardized protocols for data collection, analysis, and interpretation is crucial for facilitating collaborative research and maximizing the impact of multi-omics approaches. To overcome this hurdle open-access data and protocol sharing would be essential. That notwithstanding, there is the need to consider institutional, local and international propriety laws and regulations to protect vulnerable groups and ensure team, national and collaborative interests while making data available to the research community and policy makers. Once these regulations are established, essential data sharing platforms can be built to enable researchers to build upon existing findings and accelerate progress in TB research.
Lastly, albeit multi-omics holds immense promise for biomarker discovery, translating these findings into clinically useful diagnostics and therapeutics require further research and development efforts. These include conducting large-scale validation studies, addressing regulatory hurdles, and ensuring the affordability and accessibility of new diagnostics and drugs, while competing with existing interventions especially in resource-limited settings (61–63).
Conclusion
Multi-omics techniques are revolutionizing TB research, offering a powerful approach to identify novel biomarkers for rational drug design, vaccine development, and rapid diagnostics. By integrating data from various “omes,” researchers can gain a wholistic understanding of the complex interplay between the MTBC and the host immune response, paving the way for the development of more effective tools to combat this global health canker. Addressing the existing challenges with expertise and fostering fruitful collaborations hold the key to unlocking the full potential of multi-omics and translating these discoveries into tangible benefits for improving TB diagnosis, treatment, and ultimately, global health outcomes.
Data availability statement
The raw data supporting the conclusions of this article will be made available by the authors, without undue reservation.
Author contributions
IO: Conceptualization, Data curation, Investigation, Validation, Visualization, Writing – original draft, Writing – review & editing. SA: Data curation, Validation, Writing – review & editing. PA: Data curation, Validation, Writing – review & editing. AA: Data curation, Validation, Writing – review & editing.
Funding
The author(s) declare that no financial support was received for the research, authorship, and/or publication of this article.
Acknowledgments
IDO is a Postdoctoral Fellow of the West African Network of Excellence for Tuberculosis, HIV/AIDs and Malaria (WANETAM) which is funded by the EDCTP-3.
Conflict of interest
The authors declare that the research was conducted in the absence of any commercial or financial relationships that could be construed as a potential conflict of interest.
Publisher’s note
All claims expressed in this article are solely those of the authors and do not necessarily represent those of their affiliated organizations, or those of the publisher, the editors and the reviewers. Any product that may be evaluated in this article, or claim that may be made by its manufacturer, is not guaranteed or endorsed by the publisher.
References
2. World Health Organization. The End Strategy TB (2015). Available online at: https://apps.who.int/iris/bitstream/handle/10665/331326/WHO-HTM-TB-2015.19-eng.pdf?sequence=1&isAllowed=y.
3. Fine PEM. Variation in protection by BCG: implications of and for heterologous immunity. Lancet. (1995) 346:1339–45. doi: 10.1016/S0140-6736(95)92348-9
4. Brandt L, Cunha JF, Olsen AW, Chilima B, Hirsch P, Appelberg R, et al. Failure of the Mycobacterium bovis BCG vaccine: Some species of environmental mycobacteria block multiplication of BCG and induction of protective immunity to tuberculosis. Infect Immun. (2002) 70:672–8. doi: 10.1128/IAI.70.2.672-678.2002
5. Mangtani P, Abubakar I, Ariti C, Beynon R, Pimpin L, Fine PEMM, et al. Protection by BCG vaccine against tuberculosis: A systematic review of randomized controlled trials. Clin Infect Dis. (2014) 58:470–80. doi: 10.1093/cid/cit790
6. Ajema D, Shibru T, Endalew T, Gebeyehu S. Level of and associated factors for non-adherence to anti-tuberculosis treatment among tuberculosis patients in Gamo Gofa zone, southern Ethiopia: cross-sectional study. BMC Public Health. (2020) 20:1–9. doi: 10.1186/s12889-020-09827-7
7. Dookie N, Rambaran S, Padayatchi N, Mahomed S, Naidoo K. Evolution of drug resistance in Mycobacterium tuberculosis: A review on the molecular determinants of resistance and implications for personalized care. J Antimicrob Chemother. (2018) 73:1138–51. doi: 10.1093/jac/dkx506
8. Baluku JB, Nakazibwe B, Naloka J, Nabwana M, Mwanja S, Mulwana R, et al. Treatment outcomes of drug resistant tuberculosis patients with multiple poor prognostic indicators in Uganda: A countrywide 5-year retrospective study. J Clin Tuberc Other Mycobact Dis. (2021) 23:100221. doi: 10.1016/j.jctube.2021.100221
9. Lawn SD, Nicol MP. Xpert® MTB/RIF assay: Development, evaluation and implementation of a new rapid molecular diagnostic for tuberculosis and rifampicin resistance. Future Microbiol. (2011) 6:1067–82. doi: 10.2217/fmb.11.84
10. Stadler JAM. Updated WHO definitions for tuberculosis outcomes: Simplified, unified and future-proofed. Afr J Thorac Crit Care Med. (2022) 28:48–9. doi: 10.7196/AJTCCM.2022.v28i2.224
11. Pai M, Denkinger CM, Kik SV, Rangaka MX, Zwerling A, Oxlade O, et al. Gamma interferon release assays for detection of Mycobacterium tuberculosis infection. Clin Microbiol Rev. (2014) 27:3–20. doi: 10.1128/CMR.00034-13
12. Khan MM, Ernst O, Manes NP, Oyler BL, Fraser IDC, Goodlett DR, et al. Multi-omics strategies uncover host-pathogen interactions. ACS Infect Dis. (2019) 5:493–505. doi: 10.1021/ACSINFECDIS.9B00080/ASSET/IMAGES/MEDIUM/ID-2019-00080Q_0002.GIF
13. Ahamad N, Gupta S, Parashar D. Using omics to study leprosy, tuberculosis, and other mycobacterial diseases. Front Cell Infect Microbiol. (2022) 12:792617. doi: 10.3389/fcimb.2022.792617
14. Guha P, Dutta S, Murti K, Karan Charan J, Pandey K, Ravichandiran V, et al. The integration of omics: A promising approach to personalized tuberculosis treatment. Med Omi. (2024), 100033. doi: 10.1016/j.meomic.2024.100033
15. Weiner J, Parida SK, Maertzdorf J, Black GF, Repsilber D, Telaar A, et al. Biomarkers of inflammation, immunosuppression and stress with active disease are revealed by metabolomic profiling of tuberculosis patients. PLoS One. (2012) 7. doi: 10.1371/journal.pone.0040221
16. Chen JXJ, Han YS, Zhang SQ, Li Z, Chen JXJ, Yi WJ, et al. Novel therapeutic evaluation biomarkers of lipid metabolism targets in uncomplicated pulmonary tuberculosis patients. Signal Transduction Targeting Ther. (2021) 6. doi: 10.1038/s41392-020-00427-w
17. Madamarandawala P, Rajapakse S, Gunasena B, Madegedara D, Magana-Arachchi D. A host blood transcriptional signature differentiates multi-drug/rifampin-resistant tuberculosis (MDR/RR-TB) from drug susceptible tuberculosis: a pilot study. Mol Biol Rep. (2023) 50:3935–43. doi: 10.1007/S11033-023-08307-6/METRICS
18. DiNardo AR, Gandhi T, Heyckendorf J, Grimm SL, Rajapakshe K, Nishiguchi T, et al. Gene expression signatures identify biologically and clinically distinct tuberculosis endotypes. Eur Respir J. (2022) 60. doi: 10.1183/13993003.02263-2021
19. Parwati I, Pitaloka DAE, Chaidir L. Transcriptional biomarkers for treatment monitoring of pulmonary drug-resistant tuberculosis: protocol for a prospective observational study in Indonesia. Trop Med Infect Dis. (2022) 7. doi: 10.3390/tropicalmed7110326
20. Fu J, Zhu F, Xu C, Li Y. Metabolomics meets systems immunology. EMBO Rep. (2023) 24:1–18. doi: 10.15252/embr.202255747
21. Wan L, Hu P, Zhang L, Wang ZX, Fleming J, Ni B, et al. Omics analysis of Mycobacterium tuberculosis isolates uncovers Rv3094c, an ethionamide metabolism-associated gene. Commun Biol. (2023) 6:1–14. doi: 10.1038/s42003-023-04433-w
22. Gupta RS, Lo B, Son J. Phylogenomics and comparative genomic studies robustly support division of the genus Mycobacterium into an emended genus Mycobacterium and four novel genera. Front Microbiol. (2018) 9:67/FULL. doi: 10.3389/FMICB.2018.00067/FULL
23. Otchere ID, Coscollá M, Sánchez-Busó L, Asante-Poku A, Brites D, Loiseau C, et al. Comparative genomics of Mycobacterium africanum Lineage 5 and Lineage 6 from Ghana suggests distinct ecological niches. Sci Rep. (2018) 8:11269. doi: 10.1038/s41598-018-29620-2
24. Briffotaux J, Liu S, Gicquel B. Genome-wide transcriptional responses of mycobacterium to antibiotics. Front Microbiol. (2019) 10:249. doi: 10.3389/fmicb.2019.00249
25. Morey-León G, Andrade-Molina D, Fernández-Cadena JC, Berná L. Comparative genomics of drug-resistant strains of Mycobacterium tuberculosis in Ecuador. BMC Genomics. (2022) 23:1–16. doi: 10.1186/s12864-022-09042-1
26. Lacourse SM, Pavlinac PB, Cranmer LM, Njuguna IN, Mugo C, Gatimu J, et al. Stool Xpert Mtb/rif and urine lipoarabinomannan for the diagnosis of tuberculosis in hospitalized Hiv-infected children. Aids. (2018) 32:69–78. doi: 10.1097/QAD.0000000000001662
27. Laura M-P, Raman M, Lazro FN, Yoanis BPA, Buai TC, Eltigani O, et al. Xpert-Ultra assay on stool and urine to improve tuberculosis diagnosis in children: the Médecins Sans Frontières experience in Guinea-Bissau and South Sudan. Open Forum Infect Dis. (2024) 11:5–6. doi: 10.1093/ofid/ofae221
28. Carratala-Castro L, Ssengooba W, Kay A, Acácio S, Ehrlich J, DiNardo AR, et al. A stool based qPCR for the diagnosis of TB in children and people living with HIV in Uganda, Eswatini and Mozambique (Stool4TB): a protocol for a multicenter diagnostic evaluation. BMC Infect Dis. (2024) 24:1–9. doi: 10.1186/s12879-023-08708-9
29. Hamada Y, Getahun H, Tadesse BT, Ford N. HIV-associated tuberculosis. Int J STD AIDS. (2021) 32:780. doi: 10.1177/0956462421992257
30. Powell L, Denoeud-Ndam L, Herrera N, Masaba R, Tchounga B, Siamba S, et al. HIV matters when diagnosing TB in young children: an ancillary analysis in children enrolled in the INPUT stepped wedge cluster randomized study. BMC Infect Dis. (2023) 23:1–10. doi: 10.1186/S12879-023-08216-W/TABLES/5
31. Go A, Cantillon D, Wildner LM, Waddell SJ. Multi-omics technologies applied to tuberculosis drug discovery. Appl Sci. (2020) 10. doi: 10.3390/app10134629
32. De Carvalho LPS, Fischer SM, Marrero J, Nathan C, Ehrt S, Rhee KY. Metabolomics of mycobacterium tuberculosis reveals compartmentalized co-catabolism of carbon substrates. Chem Biol. (2010) 17:1122–31. doi: 10.1016/j.chembiol.2010.08.009
33. Målen H, De Souza GA, Pathak S, Søfteland T, Wiker HG. Comparison of membrane proteins of Mycobacterium tuberculosis H37Rv and H37Ra strains. BMC Microbiol. (2011) 11. doi: 10.1186/1471-2180-11-18
34. Albrethsen J, Agner J, Piersma SR, Højrup P, Pham TV, Weldingh K, et al. Proteomic profiling of Mycobacterium tuberculosis identifies nutrient-starvation-responsive toxin-antitoxin systems. Mol Cell Proteomics. (2013) 12:1180–91. doi: 10.1074/mcp.M112.018846
35. Rienksma RA, Suarez-diez M, Mollenkopf H, Dolganov GM, Dorhoi A, Schoolnik GK, et al. Comprehensive insights into transcriptional adaptation of intracellular mycobacteria by microbe-enriched dual RNA sequencing. BMC Genomics. (2015) 16:1–15. doi: 10.1186/s12864-014-1197-2
36. Dutta NK, Klinkenberg LG, Vazquez MJ, Segura-Carro D, Colmenarejo G, Ramon F, et al. Inhibiting the stringent response blocks Mycobacterium tuberculosis entry into quiescence and reduces persistence. Sci Adv. (2019) 5:1–13. doi: 10.1126/sciadv.aav2104
37. Serafini A, Tan L, Horswell S, Howell S, Greenwood DJ, Hunt DM, et al. Mycobacterium tuberculosis requires glyoxylate shunt and reverse methylcitrate cycle for lactate and pyruvate metabolism. Mol Microbiol. (2019) 112:1284–307. doi: 10.1111/mmi.14362
38. Morreale FE, Kleine S, Leodolter J, Junker S, Hoi DM, Ovchinnikov S, et al. BacPROTACs mediate targeted protein degradation in bacteria. Cell. (2022) 185:2338–2353.e18. doi: 10.1016/j.cell.2022.05.009
39. Bonjorno AF, Pavan AR, Fernandes GFS, Scarim CB, Castagnolo D, Dos Santos JL. BacPROTACs targeting Clp protease: a promising strategy for anti-mycobacterial drug discovery. Front Chem. (2024) 12:1358539. doi: 10.3389/fchem.2024.1358539
40. Sassetti CM, Boyd DH, Rubin EJ. Genes required for mycobacterial growth defined by high density mutagenesis. Mol Microbiol. (2003) 48:77–84. doi: 10.1046/j.1365-2958.2003.03425.x
41. Griffin JE, Gawronski JD, DeJesus M, Ioerger TR, Akerley BJ, Sassetti CM. High-resolution phenotypic profiling defines genes essential for mycobacterial growth and cholesterol catabolism. PloS Pathog. (2011) 7:1–9. doi: 10.1371/journal.ppat.1002251
42. Dejesus MA, Gerrick ER, Xu W, Park SW, Long JE, Boutte CC, et al. Comprehensive essentiality analysis of the Mycobacterium tuberculosis genome via saturating transposon mutagenesis. MBio. (2017) 8. doi: 10.1128/mBio.02133-16
43. Rudraraju RS, Daher SS, Gallardo-Macias R, Wang X, Neiditch MB, Freundlich JS. Mycobacterium tuberculosis KasA as a drug target: Structure-based inhibitor design. Front Cell Infect Microbiol. (2022) 12:1008213. doi: 10.3389/fcimb.2022.1008213
44. Howell S, Khoury H, Snijders AP, Prosser GA, de Chiara C, Rodenburg A, et al. Glutamate racemase is the primary target of β-chloro-d-alanine in mycobacterium tuberculosis. Antimicrob Agents Chemother. (2016) 60:6091–9. doi: 10.1128/aac.01249-16
45. Fernández-García M, Rey-Stolle F, Boccard J, Reddy VP, García A, Cumming BM, et al. Comprehensive Examination of the Mouse Lung Metabolome following Mycobacterium tuberculosis Infection Using a Multiplatform Mass Spectrometry Approach. J Proteome Res. (2020) 19:2053–70. doi: 10.1021/acs.jproteome.9b00868
46. Krishnan S, Queiroz ATL, Gupta A, Gupte N, Bisson GP, Kumwenda J, et al. Integrative multi-omics reveals serum markers of tuberculosis in advanced HIV. Front Immunol. (2021) 12:676980/FULL. doi: 10.3389/FIMMU.2021.676980/FULL
47. Panchalingam S, Kasivelu G, Jayaraman M, Kumar R, Kalimuthu S, Jeyaraman J. Differential gene expression analysis combined with molecular dynamics simulation study to elucidate the novel potential biomarker involved in pulmonary TB. Microb Pathog. (2023) 182:106266. doi: 10.1016/j.micpath.2023.106266
48. Song L, Wallstrom G, Yu X, Hopper M, Van Duine J, Steel J, et al. Identification of antibody targets for tuberculosis serology using High-Density Nucleic Acid Programmable Protein Arrays. Mol Cell Proteomics. (2017) 16:S277–89. doi: 10.1074/mcp.M116.065953
49. Zhuang L, Ye Z, Li L, Yang L, Gong W. Next-generation TB vaccines: progress, challenges, and prospects. Vaccines. (2023) 11. doi: 10.3390/vaccines11081304
50. Chugh S, Bahal RK, Dhiman R, Singh R. Antigen identification strategies and preclinical evaluation models for advancing tuberculosis vaccine development. NPJ Vaccines. (2024) 9. doi: 10.1038/s41541-024-00834-y
51. Gideon HP, Skinner JA, Baldwin N, Flynn JL, L. PL. Early whole blood transcriptional signatures are associated with severity of lung inflammation in cynomolgus macaques with Mycobacterium tuberculosis infection Hannah. J Immunol. (2016) 197:4817–28. doi: 10.4049/jimmunol.1601138
52. Liu YE, Darrah PA, Zeppa JJ, Kamath M, Laboune F, Douek DC, et al. Blood transcriptional correlates of BCG-induced protection against tuberculosis in rhesus macaques. Cell Rep Med. (2023) 4:101096. doi: 10.1016/j.xcrm.2023.101096
53. Koeken VACM, Qi C, Mourits VP, de Bree LCJ, Moorlag SJCFM, Sonawane V, et al. Plasma metabolome predicts trained immunity responses after antituberculosis BCG vaccination. PloS Biol. (2022) 20:1–18. doi: 10.1371/journal.pbio.3001765
54. Guerra-Maupome M, McGill JL. Characterization of local and circulating bovine γδ T cell responses to respiratory BCG vaccination. Sci Rep. (2019) 9:1–15. doi: 10.1038/s41598-019-52565-z
55. Ahsan H, Ahmad R, Ahsan H, Ahmad R. Multiplex technology for biomarker immunoassays. Innate Immun Heal Dis. (2020). doi: 10.5772/INTECHOPEN.91730
56. Stevens KG, Pukala TL. Conjugating immunoassays to mass spectrometry : Solutions to contemporary challenges in clinical diagnostics. Trends Anal Chem. (2020) 132. doi: 10.1016/j.trac.2020.116064
57. Chu X, Zhang B, Koeken VACM, Gupta MK, Li Y. Multi-omics approaches in immunological research. Front Immunol. (2021) 12:668045. doi: 10.3389/fimmu.2021.668045
58. Cao H, Xu H, Ning C, Xiang L, Ren Q, Zhang T, et al. Multi-omics approach reveals the potential core vaccine targets for the emerging foodborne pathogen campylobacter jejuni. Front Microbiol. (2021) 12:665858. doi: 10.3389/fmicb.2021.665858
59. Drury RE, Camara S, Chelysheva I, Bibi S, Sanders K, Felle S, et al. Multi-omics analysis reveals COVID-19 vaccine induced attenuation of inflammatory responses during breakthrough disease. Nat Commun. (2024) 15. doi: 10.1038/s41467-024-47463-6
60. Moorlag SJCFM, Folkman L, ter Horst R, Krausgruber T, Barreca D, Schuster LC, et al. Multi-omics analysis of innate and adaptive responses to BCG vaccination reveals epigenetic cell states that predict trained immunity. Immunity. (2024) 57:171–87.e14. doi: 10.1016/j.immuni.2023.12.005
61. Seyhan AA. Lost in translation: the valley of death across preclinical and clinical divide – identification of problems and overcoming obstacles. Transl Med Commun. (2019) 4:1–19. doi: 10.1186/s41231-019-0050-7
62. Abu-Odah H, Said NB, Nair SC, Allsop MJ, Currow DC, Salah MS, et al. Identifying barriers and facilitators of translating research evidence into clinical practice: A systematic review of reviews. Health Soc Care Community. (2022) 30:e3265–76. doi: 10.1111/hsc.13898
63. Gagliardi D, Amnatidou E. Translating research into innovation: Lessons from 3 case studies in health partnerships. (2023) Manchester, UK.
64. Omics AI. A curated collection of workflows for processing omics data | Omics AI . Available online at: https://omics.ai/workflows/ (Accessed May 21, 2024).
65. Pythia BioSciences. CDIAM: A multi-omics analysis software platform with adaptability and ease of use . Available online at: https://www.pythiabio.com/post/cdiam-a-multi-omics-analysis-software-platform-with-adaptability-and-ease-of-use (Accessed May 21, 2024).
66. Schumacher A, Rujan T, Hoefkens J. A collaborative approach to develop a multi-omics data analytics platform for translational research. Appl Transl Genomics. (2014) 3:105–8. doi: 10.1016/j.atg.2014.09.010
67. PANDORA. The Pan-African Network For Rapid Research, Response, Relief and Preparedness for Infectious Disease Epidemics (PANDORA-ID-NET) . Available online at: https://pandora.tghn.org/training/ (Accessed May 21, 2024).
69. AfCDC. Digitizing public health genomics in Africa – Africa CDC (2023). Available online at: https://africacdc.org/news-item/digitizing-public-health-genomics-in-africa/ (Accessed May 21, 2024).
Keywords: tuberculosis, Mycobacterium tuberculosis complex (MTBC), drug resistance, diagnostics, Bacillus Calmette-Guérin vaccine, biomarkers, host-pathogen interactions
Citation: Otchere ID, Aboagye SY, Arthur PK and Asante-Poku A (2024) Viewpoint of multi-omics potential in tuberculosis research: identifying biomarkers for biomanufacturing of efficient control tools. Front. Trop. Dis 5:1443248. doi: 10.3389/fitd.2024.1443248
Received: 03 June 2024; Accepted: 12 July 2024;
Published: 31 July 2024.
Edited by:
Emile Rugamika Chimusa, Northumbria University, United KingdomReviewed by:
Bindu Singh, Texas Biomedical Research Institute, United StatesCopyright © 2024 Otchere, Aboagye, Arthur and Asante-Poku. This is an open-access article distributed under the terms of the Creative Commons Attribution License (CC BY). The use, distribution or reproduction in other forums is permitted, provided the original author(s) and the copyright owner(s) are credited and that the original publication in this journal is cited, in accordance with accepted academic practice. No use, distribution or reproduction is permitted which does not comply with these terms.
*Correspondence: Isaac Darko Otchere, aW90Y2hlcmVAbm9ndWNoaS51Zy5lZHUuZ2g=; aWRvdGNoZXJlQG1yYy5nbQ==