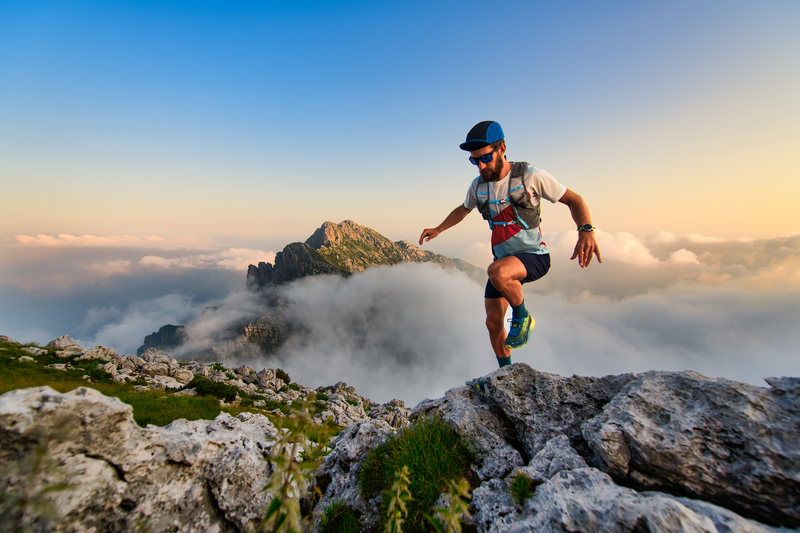
95% of researchers rate our articles as excellent or good
Learn more about the work of our research integrity team to safeguard the quality of each article we publish.
Find out more
ORIGINAL RESEARCH article
Front. Trop. Dis. , 17 May 2024
Sec. Vaccines for Tropical Diseases
Volume 5 - 2024 | https://doi.org/10.3389/fitd.2024.1393570
Background: Soil- and water-transmitted helminths are a major concern in the developing world due to their high prevalence. More than a quarter of the population were estimated to be infected with helminths in these endemic zones.
Research design: An in silico approach was used to design a vaccine construct against the Taenia genus utilizing the proteomic information and evaluation of the construct using immune-informatics.
Results: Our study identified 451 conserved proteins in Taenia spp. using the existing proteome; out of these, 141 were found to be expressed in cysticerci. These proteins were screened for antigenic epitopes and a multi-subunit vaccine was constructed. The constructed vaccine was assessed for its efficacy in mounting the appropriate immune response. Our constructed vaccine showed stability and optimal performance against the TLR 4 receptor, which is reported to be upregulated in Taenia infections in hosts.
Conclusion: Immune-informatics tools help design vaccines for neglected diseases such as those attributed to helminths, which are known to cause widespread morbidity. Our vaccine construct holds tremendous potential in conferring protection against all Taenia spp. of clinical relevance to human.
Helminths that cause infections in humans are traditionally classified as platyhelminths (meaning flatworms) and nematodes (roundworms). Platyhelminths are further classified into cestodes (tapeworms) and trematodes (flukes). They are relatively large (>1 mm) in size and have well-developed organ systems. Helminths are distributed widely across continents, and its infection rate is higher and alarming among poor countries. According to one estimate, more than a quarter of the world's population is facing the threat of helminthic infections, causing substantial disease burden and associated disabilities (1). Among the helminths infecting humans, Taenia spp. (T. solium and T. asiatica) are the prominent ones. According to a recent World Health Organization (WHO) estimate, a total of 2.56–8.30 million individuals have symptomatic or asymptomatic neurocysticercosis (NCC) globally (2). Even though a large proportion of the population have been infected and high morbidity is associated with this infection, it falls under the neglected tropical disease (NTD) classification of the WHO list due to the lack of interest shown towards this disease from local authorities and pharmaceutical companies. It is mainly prevalent in economically poor regions across the globe, such as in sub-Saharan Africa, Latin America, and Asia. Taenia spp. infection is widely recognized as endemic (3–6).
T. solium and T. asiatica are the primary Taenia spp. that infect humans, whereas T. saginata infects cattle or may infect immunocompromised humans. T. solium is primarily endemic in various countries across the world, while T. asiatica is more indigenous in Asian countries such as Thailand, India, China, and Indonesia (7–10). Adult tapeworms of T. solium and T. asiatica species reside in the human intestine for several years and cause taeniasis. Among these two, T. solium infection is more detrimental to the host as it may cause NCC. NCC is one of the leading causes of acquired epilepsy in tropical regions (11, 12). The foodborne pathogen was the leading cause of death in 2015, resulting in a considerable total of 2.8 million disability-adjusted life-years (DALYs) (13). The infection occurs due to the consumption of raw infected pork, and an infected person can show a diverse range of symptoms such as diarrhea, loss of appetite, weight loss, and nausea (14, 15). Taeniasis can cause severe adverse effects in children, causing malnutrition, weight loss, intellectual disability, and stunted physical growth (14, 16). However, the cystic form of T. solium causes a more deadly infection where the cyst invades the host’s different body parts like muscles, eye, liver, and brain and causes cysticercosis (3, 17, 18). Cysticercosis could have a more severe impact on the patient’s health by causing epileptic seizures, paralysis, mood swings, depression, and death in some rare cases (15, 19–22).
The WHO has been carrying out mass antihelminthic treatment using drugs like praziquantel, albendazole, or niclosamide in endemic countries to reduce the infection incidence and prevalence (22, 23). However, mass drug administration cannot be a long-term solution to the problem. Because of the rampant use of anthelminthic drugs, several incidences of drug-resistant helminths have been reported, which provides a warning and raises awareness for future treatment regimens for helminths (24–26). To completely eradicate these parasites, it requires continuous administration of drugs for a prolonged period of time at several intervals, which is economically expensive and logistically cumbersome. Thus, there is an urgent need for a human vaccine against taenia infections to completely eradicate them.
To date, only a T. solium oncospheral protein-based vaccine (TSOL18) is available for pig cysticercosis, which was reported to have 90% efficacy and used in endemic regions to passively control the spread of these parasites in the human population (27–29). Nevertheless, the vaccination cycle faces significant obstacles due to the poor economic circumstances and limited knowledge of rural farmers, impeding the overarching objective of vaccination efforts. A single person infected with taeniasis sheds 10,000–50,000 eggs daily by excretion of proglottids in fecal matter, which can re-establish the infectious cycle in humans and pigs. While there is an urgent need to emphasize improved sanitation, hygiene practices, proper cooking of pork, and public health interventions to reduce the individual risk of Taenia infections, vaccination proves to be an effective strategy to break the ongoing reinfection cycle and confer protection to populations at risk.
A single vaccine providing complete protection to humans from all Taenia spp. infections will be a better strategy. However, attracting good funding for the development of an NTD-associated vaccine is a huge challenge. In this research work, we utilized the potential of immune-informatics along with proteomics and designed a multi-epitope vaccine by applying subtractive proteomics in vaccine target mining followed by the reverse vaccinology method. This approach has been utilized before for designing vaccines for several other pathogens like Schistosoma spp., Ascaris spp., and filarial worms (30–33). We investigated the complete proteome of Taenia spp. to identify highly conserved proteins expressed during infection using an in silico approach and then confirmed the expression of these proteins in T. solium cysticercosis infection at the cysticerci stage. After predicting the B- and T-lymphocyte epitopes, we separated non-toxic, highly antigenic, and non-allergic peptide epitopes from non-antigenic, toxic, and allergic peptides for vaccine construction. The manufactured vaccine was modeled and characterized for different biological parameters such as physicochemical properties. We also verified the quality of the modeled protein and interaction with host innate immune receptors.
T. solium cysts were isolated from naturally infected pork; cyst wall (CW) protein and crude lysate (CL) were prepared according to previously published protocols (34). Briefly, isolated viable cysts from naturally infected swine were washed with chilled phosphate buffer saline (PBS) (Sigma-P4417; supplemented with 1% antibiotic–antimycotic; Gibco-15240062). The CW was separated from the cysts under aseptic conditions. The isolated whole cysts and collected CW were crushed in a pestle and mortar under liquid nitrogen separately for the preparation of CL and CW. The obtained powder for CL and CW was then resuspended in chilled PBS supplemented with protease inhibitor (Abcam-GR3281688-1), sonicated, and ultra-centrifuged to settle down the insoluble fractions. Supernatants were collected for CL and CW, aliquoted, and stored at −80°C until further used.
The CL and CW fractions were resolved on 10% SDS-PAGE and stained with Coomassie brilliant blue, and the protein bands for CW and CL were cut using a clean scalpel and small pieces were collected in a freshly prepared 50 mM ammonium bicarbonate (ABC) solution until the gel turned colorless. Protein bands were washed with 50% acetonitrile (ACN) in 50 mM ABC to shrink the volume of gel pieces. The dried gel fragments were treated with 10 mM dithiothreitol (DTT) for 15 min at 56°C for reduction, followed by alkylation with 55 mM iodoacetamide (IAA) in the dark at room temperature for 20 min. Before putting the gel pieces in trypsin solution, they were washed with 50% ACN in 50 mM ABC solution and later incubated in trypsin solution overnight at 37°C. Finally, the digested protein peptides were extracted in a small volume of 50% ACN along with 1% trifluoroacetic acid (TFA) solution twice by sonicating and centrifuging. The peptides obtained were dehydrated using a speed vacuum and combined with α-cyano-4-hydroxycinnamic acid matrix (0.5 µL) before subjecting them to matrix-assisted laser desorption ionization (MALDI)-TOF/TOF-MS/MS analysis using Bruker Daltonics equipment. Bio-Tools 3.0 software facilitated a combined MS and MS/MS search for peptide determination, utilizing the “MASCOT” search engine (v.2.1; Matrix Science, UK), which uses the specific limiting factors, such as taxonomy: T. solium (12,467 sequences); trypsin (enzyme), carbamidomethyl (fixed alterations), oxidation (M), and Glu > pyro-Glu (N-terminal glutamine) (variable alterations), missed cleavage, 1; MS/MS mass tolerance (0.7 Da); parent ion mass tolerance (20 ppm); and MS/MS peak filtering, monoisotopic, and M+ H+. Analyzed proteins meeting Mascot’s threshold score (p < 0.05), appearing as top hits in the search report, and showing similarities with more than two peptides, were considered to be positively identified. Functional annotation of these proteins was conducted using Blast2GO to categorize them into cellular, biological, and molecular functions. The resulting GO terms were then verified manually against the UniProtKB database (https://www.uniprot.org/), and KEGG pathway analysis (https://www.genome.jp/kegg/) was performed to confirm the putative enzymes. Subsequently, the G: profiler tool accessible on the https://parasite.wormbase.org/index.html was utilized to carry out gene enrichment analysis.
The whole proteome of T. asiatica and T. solium was downloaded from the WormBase ParaSite (https://parasite.wormbase.org/index.html) server in FASTA format. The BLAST NCBI online server was used to sort the conserved proteins between these species by employing ≥95% identity and e-value < 1e−04 stringent conditions. Proteins that showed ≥50% homology (and e-value > 0.01) with the human proteome were removed from further analysis.
The in silico identified conserved proteins were filtered for proteins expressed during the infection. For this, our previously published proteome of cyst fluid (CF) and excretory–secretory proteins (ESPs) (35, 36) along with the proteome of CL and CW from MALDI was screened to confirm the presence of in silico identified conserved proteins. This step is crucial for the successful removal of all the proteins that had not been expressed at their infective stage.
The expressed proteins were functionally sorted based on their possible subcellular location such as secretory and membrane. The fate of a protein is greatly determined by the signal peptide they express, with some being directed for secretion and transport to the cell membrane. The Signal P 5.0 (https://services.healthtech.dtu.dk/services/SignalP-5.0/) online server was used to determine the presence or absence of signal peptide (37). The presence of transmembrane helix was predicted using the TMHMM 2.0 (https://services.healthtech.dtu.dk/services/TMHMM-2.0/) server and Phobius (https://phobius.sbc.su.se/), which further confirmed their membrane localization property (38) With the DeepLoc (https://services.healthtech.dtu.dk/services/DeepLoc-2.0/) server, subcellular locations such as mitochondrial, endoplasmic reticulum, and the nuclear membrane of the proteins were predicted (39). Non-classically secreted proteins were predicted using Outcyte (http://www.outcyte.com/) and SecretomeP (https://services.healthtech.dtu.dk/services/SecretomeP-2.0/) (40).
All the secretory and membrane-bound proteins were screened for B-lymphocyte peptide epitopes utilizing the IEDB-B-cell 2.0 (http://tools.iedb.org/main/bcell/) prediction tool, which works on Random Forest Algorithms. A threshold of 0.8 was set to ensure the specificity of the predicted peptides and reduce the false-positive predictions (41).
The NetCTL 2.1 server (https://services.healthtech.dtu.dk/services/NetCTL-1.2/) was employed for the determination of T-lymphocyte peptide epitopes on all the membrane-bound and secreted proteins identified. Cytotoxic T cells interact with the MHCI molecule binding peptides present on immune cells (33).
To evaluate any associated risk of the vaccine, allergic proteins were separated from the non-allergic proteins. The AllerCatPro 2.0 server (https://allercatpro.bii.a-star.edu.sg/help.html) screens for proteins capable of eliciting IgE-mediated reaction (42). It uses a known dataset of 4,180 allergic proteins obtained from different sources. The obtained results showed weak and strong evidence to allergenicity. All the allergen proteins were removed from further analysis.
The Th1 peptide epitopes were identified as IFN-γ-inducing epitopes; it helps to activate the CD4 T cells and generate a pro-inflammatory cytokine response that could mount robust immune response against invading pathogen. The IFNepitope prediction tool (https://webs.iiitd.edu.in/raghava/ifnepitope/predict.php) works using a hybrid approach (SVM and MERCI-based prediction) (43). The IFN-inducing positive epitopes were further evaluated for their antigenicity using the ANTIGENpro server (https://scratch.proteomics.ics.uci.edu/) that works on a set of five machine algorithms (32); this further refined our selection of epitopes.
The final predicted small B- and T-lymphocyte epitopes were connected using GPGPG and AAY linkers, respectively, to compose a multi-epitope chimera vaccine (31). Owing to their small size, these peptides cannot provoke a robust immune reaction. To enhance their immunogenicity, these peptides should be joined together. These linkers maintain the conformation and functional domains of predicted epitopes in the structured model.
The “Expasy ProtParam” online server (https://web.expasy.org/protparam/) was used to study the biophysical properties of the constructed vaccine (44). It calculated different properties of the query protein involving theoretical pI (isoelectric point), molecular weight, aliphatic index, instability index, and GRAVY index. The aliphatic index measures the proportionate magnitude of aliphatic side chains of the aliphatic amino acids (Ala, Val, Ile, and Leu) while the instability index detects the stability of the protein experimentally. GRAVY indicates the hydrophobicity of the proteins that vary from −2 to +2.
The planar conformation of the final vaccine construct was obtained by accessing the PSIPRED tool (http://bioinf.cs.ucl.ac.uk/psipred/). The PSIPRED protein structure prediction server consists of three methods, namely, PSIPRED, MEMSAT2, and GenTHREADER, that collectively work together to predict protein structure, utilizing its amino acid sequence only (45). The I-TASSER (Iterative Threading ASSEmbly Refinement) protein structure and function prediction server (http://zhanglab.ccmb.med.umich.edu/I-TASSER) was exploited to acquire tertiary structures of the final construct. Using multiple threading alignment approaches, I-TASSER recognizes the structural templates from the PDB. Then, complete structural models are created through a process of redundant simulations that assemble fragments constantly (46).
The obtained model was further calibrated using 3Drefine (https://3drefine.mu.hekademeia.org/) and Galaxy refine servers (https://galaxy.seoklab.org/index.html) to strengthen the local attributes of the model. The 3Drefine web server utilizes a repetitive method consisting of two steps of minimization: improved H-bond network and minimized energy by employing a combined physical and knowledge-based force field (47). In contrast, Galaxy Refine initially reassembles the side chains on the basis of the highest-probability rotamers, which then stretch to the surface in a layer-wise fashion (48). The different parameters such as local quality, overall quality score, and Ramachandran plot of the final refined model were measured using the ProSA web (https://prosa.services.came.sbg.ac.at/) and SAVES 6.0 servers (https://saves.mbi.ucla.edu/) (49).
The invading pathogens generate humoral immune response by interacting with the B-cell receptor on the B lymphocytes. The ElliPro server was utilized for the detection of discontinuous B-cell epitopes within the finalized and refined tertiary model structure (http://tools.iedb.org/ellipro/). It gives the ellipsoid score to each residue in the structure, also known as the Protrusion Index (PI) value, and defines the most probable regions that work as confirmational B-cell epitopes. The amino acid residues with greater PI scores are linked to higher solvent accessibility, and they predicted discontinuous B-cell epitopes better (50).
Proteasomal cleavage peptides were determined by using the Proteasomal Cleavage Prediction tool of the IEDB server (http://tools.iedb.org/netchop/) and the Proteasome Cleavage Prediction Server of the Immunomedicine group (http://imed.med.ucm.es/Tools/pcps/). The threshold value was kept at 0.5 in both servers. These tools are based on neural networks for predicting T-cell epitopes (51, 52).
During T. solium infection, various Toll-like receptors (TLR2, TLR3, TLR4, and TLR7) are known to be expressed (53, 54). We also performed the docking studies to validate the direct interactions between innate immune receptor TLR4 and constructed vaccines by employing the ClusPro 2.0 server (https://cluspro.org/login.php?redir=/home.php) (55). We retrieved the 3D structure of TLR4 in PDB format from the PDB database (“https://www.rcsb.org/”), with an accession number of 4G8A. This server involves a three-step computational process. Firstly, it performs docking by exploring multiple conformations through extensive sampling. Subsequently, it employs root mean square deviation (RMSD) to cluster the 1,000 lowest-energy structures, which helps to identify the potential models. Finally, the selected structures undergo refinement through energy minimization to increase their accuracy and stability (56). To determine the overall energy and different interactions between the docked complex, we used the HADDOCK 2.4 server (https://wenmr.science.uu.nl/haddock2.4/submit/1) and the LigPlot+ software (https://www.ebi.ac.uk/thornton-srv/software/LigPlus/), respectively (57).
Molecular dynamics (MD) simulations were executed with the help of GROMACS (GROningen Machine for Chemical Simulations) 2022.3 version. The PDB file of the vaccine construct, TLR4, and vaccine-TLR4 docked structures were introduced into MD simulation. The system was plunged into a cubic enclosure employing the TIP4P water model and OPLS-AA force field. Sodium and chloride ions were administered for neutralization purposes. Furthermore, energy reduction and solvent equilibration were carried out via NVT (temperature) and NPT (pressure) run for 50,000 nsteps. Finally, MD simulations for 5 crore nsteps (100 ns) was conducted to create the desired files, i.e., RMSD, root mean square fluctuation (RMSF), H-bonding, and radius of gyration.
To confirm the immune reaction induced by the modeled vaccine construct, we carried out an immune simulation investigation using the C-ImmSim (10.0) server (https://kraken.iac.rm.cnr.it/C-IMMSIM/index.php) (58). It estimates different parameters for both cellular and humoral immune response such as titers of different immunoglobulins (IgM, IgG1, and IgG2), the T-lymphocyte population (Th1 and Th2), and the cytokines released. For simulation, we opted for three injection volumes, each comprising 10 µL, administered at intervals of 0, 84, and 164 days. The modeling process involved increments of 1,000 steps (58).
In silico cloning was performed to design a viable plasmid construct containing the peptide vaccine within an expression vector for potential vaccine production. Initially, the amino acid sequence of the vaccine was reverse-translated into nucleotides using the reverse translation tool available on the Sequence Manipulation Suite server (https://www.bioinformatics.org/sms2/). Next, codon optimization was performed utilizing the Java Codon Adaptation Tool (JCat) (https://www.jcat.de/) to improve the expression efficiency of the vaccine design (59). This optimization process yields two key outputs: the Codon Adaptation Index (CAI) and the % GC content. Optimal expression of the vaccine requires the CAI value to fall within the range of 0.2 to 1.0, while the % GC content should ideally range between 30% and 70% (59, 60). Following optimization, the nucleotide sequence was then in silico cloned into the pET28b vector, thus preparing it for the expression within the bacterial Escherichia coli k12 system. This is required to design a proficient cloning approach for the prospective vaccine candidate.
All the work was performed after approval from the Institute Ethics Committee, Animal Ethics Committee (IIT/IAEC/2023/003), and Institute Biosafety Committee of IIT Mandi.
The CBB-stained proteins of cyst isolated proteins (CL and CW) showed multiple bands with molecular weight varying from 10 to 250 kDa (Figure 1A). MALDI data analysis was carried out using the MASCOT server. We found 189 proteins in CL and 402 proteins in CW. The molecular weight of the proteins identified in CL varies from 20 to 160 kDa, whereas CW protein’s molecular weight varies from 20 to 250 kDa. The calculated theoretical pI of both CL and CW proteins lies between 2 and 15 (Figure 1B).
Figure 1 Protein separation on SDS gel and protein analysis. (A) Polyacrylamide Gel stained with Silver Nitrate showing the isolated proteins in band patterns. (B) Different Physicochemical Parameters of Proteins identified during the study. (i and iv) – Molecular Weight ranges in CL and CW respectively. (ii and v) – GRAVY Indices of proteins found in CL and CW respectively. (iii and iv) – Isoelectric Points of the proteins found in CL and CW respectively. [CL, Crude Lysate; CF, Cyst Fluid; CW, Cyst Wall; ESP, Excretory Secretory Proteins and MW, Molecular Weight Marker].
T. solium has 12,481 protein sequences while T. asiatica has a slightly higher number of proteins, 13,161. Both species had 1,393 conserved proteins with ≥95% identify and <1e−04 e-value in their proteome. After removing 942 proteins with shared homology to humans, we proceeded with 451 non-homologous proteins.
To confirm the expression of these adult tapeworm proteins during infection, 451 non-homologous proteins were screened. We screened T. solium MALDI data for CL and CW and LC-MS/MS data of CF of ESP. We found that 141 proteins were expressed by the cysticerci (Supplementary Table S1). This step helped us to identify proteins of relevance during infection as they are present at the cystic (pathologic) stage and to eliminate the rest of the proteins from further analysis.
The subcellular locations of expressed proteins were predicted using various tools, and we confirmed the presence of 22 membrane-bound and 74 secretory proteins. We predicted and confirmed the localization of membrane proteins using Phobius, TMHMM 2.0, and DeepLoc and sorted 22 membrane proteins, while two other software, Outcyte and SecretomeP 2.0, along with DeepLoc confirmed the secretory nature of 74 expressed proteins. Proteins that were not predicted as membrane-bound or secretory (45) were discarded from further analysis.
The analysis focused on highly conserved membrane and excretory proteins to identify the B- and T-lymphocyte peptide epitopes using IEDB-B-cell 2.0 and NetCTL 2.1 prediction tools, respectively. The seven membrane proteins showed both B- and T-cell epitopes above the 0.8 threshold value, while 17 excretory proteins had B- and T-cell epitopes above the 0.7 threshold value.
To ensure the safety of vaccine for human use, it is essential that a vaccine candidate does not cause any adverse reactions. Based on the allergenicity potential, i.e., eliciting IgE-mediated response in the host, three membrane and six secretory proteins were removed. Finally, 4 membrane and 11 secretory non-allergic proteins were selected for further analysis.
The predicted T-cell epitopes were also analyzed for their potential to activate the IFN-γ signaling pathway, which could also stimulate B cells for antibody production and help in parasite elimination. Two membrane (TsM_000707200 and TsM_000606600) and four secretory (TsM_000558000, TsM_000774000, TsM_000828800, and TsM_000836300) proteins were highly antigenic proteins with IFN-γ-inducing epitopes and were studied further.
A total of 18 peptides predicted to be B- and T-lymphocyte epitopes, with positive IFN-γ-inducing properties (Table 1), were connected using GPGPG and AAY linkers, respectively, to make a stable multi-chimeric protein vaccine. These epitopes are conserved in Taenia spp. (Figure 2). At the N-terminal, an adjuvant (β-defensin) was attached to an EAAAK linker to elevate the immunogenicity of the resultant vaccine as it can recruit more immune cells at the site of vaccination. The built vaccine construct consisted of 344 amino acid residues, the sequence for which is given below:
Table 1 The list of predicted epitopes used to construct a multi-epitope vaccine against the Taenia spp. infections.
Figure 2 The aligned conserved peptides found in Taenia solium (TsM) and Taenia asiatica (TASK) with epitope predictions in high statistical confidence range used to construct the multi-epitope vaccine. β-defensin is attached to the N-terminal with the help of an EAAAK linker to design the final product.
MRVLYLLFSFLFIFLMPLPGVFGGIGDPVTCLKSGAICHPVFCPRRYKQIGTCGLPGTKCCKKPEAAAKETDVILMCFAAYSEKWTAEVAAYRALAELSKFAAYFLVAFPTDYAAYEPYYNEPGFEKITNPRASAHYNEMAAYVPSLRTWRRGMLIRAAYDPAQADAYTLFIQNRGPGPGSAQAYQLAYDESIGPGPGQKGSNDGTGPGPGKENAKLTQPPDGPGPGDVMERKASFPDGPGPGLEQYEDYLGPGPGRYSFSLTGPGPGKFNSELIDESSISGPGPGKRYNDKLELEGPGPGRVLQPSEIKGPGPGQFPEVYVPTVFGPGPGGQEDYDRLRPLSY.
The Expasy ProtParam server was utilized to estimate various biophysical properties of the construct, revealing a molecular weight of 37 kDa with a theoretical pI value of 5.64. The high molecular weight of the vaccine increases its recognition by the immune system. The low instability index of 32.22 (as per literature, it should be less than 40) confirmed its stability, whereas a relatively high aliphatic index, 62.76, marked its thermal stability. The constructed vaccine had a negative GRAVY score of −0.403, suggesting its hydrophilic nature. The observed half-life of the developed vaccine was 30 h for mammalian cells, >20 h for Saccharomyces cerevisiae (in vivo), and >10 h for Escherichia coli. These properties confirmed that the designed vaccine structure is stable.
The probable 2D structures of the constructed model was measured by PSIPRED, and it consisted of 30% α-helix, 20% β-sheet, and 50% coils (Figure 3). The proteins were folded into stable and conformational 3D structure to perform differential functions. I-TASSER gave the top five models with different parameters, and the first model had the best parameters as compared to the other four based on C-score and RMSD value, which showed the closest resemblance to the experimental structure. The C-score (which ranges from −5 to 2) refers to the confidence that measures the quality of the predicted models by I-TASSER. The top-ranked model had a −3.48 C-score and a 15.1 ± 3.5 Å RMSD score and was further refined to reduce the overall energy and stabilize the overall structure.
Figure 3 A graphical representation of the predicted secondary structure elements from the PSIPRED view tool, showing α-helix, β-sheet, and coils with percentages of 30%, 20%, and 50%, respectively.
The obtained 3D structure of the constructed vaccine was further refined firstly using the 3Drefine server and then the Galaxy Refine server. It gave the top five refined models, which were further validated by measuring different parameters such as overall quality measured with Z-score, local quality, and Ramachandran plot analysis (Figure 4). The overall quality of the best model vaccine construct was estimated to be about −7.49. This also showed its 3D resemblance with experimentally proven x-ray crystal structures (Figure 4B). The dark green line in local quality confirmed the overall negative energy of the 3D structure, which further confirmed the stability of the 3D structure of the designed vaccine (Figure 4C). In the Ramachandran plot, we found that 94.9% of amino acids lie in the favored and additionally favored region collectively, and only 4.7% of amino acids were in the disallowed region, which confirmed that the formed 2D structures such as α-helix, β-sheets, and turns were stable and were not having any steric hinderance (Figure 4D). Vaccine construct has also been found to be stable and reliable as per the 3D/1D ratio and ERRAT score (Supplementary Figure 1).
Figure 4 The final validated structure of the designed vaccine. (A) The refined 3D structure of the vaccine construct obtained from the I-TASSER server, visualized with PyMOL software. (B, C) Structural validation through the ProSA web tool showing a Z-score of −7.49 showing a great overall quality of the structure. (D) Ramachandran plot analysis by the SAVES 6.0 server unveils that 75.6% of amino acids resides in the most favored region, 19.7% falls under the allowed region, whereas 4.7% amino acids lie in the disallowed region, respectively.
The B-cell receptors are present on B lymphocytes and interact with conformational B-cell epitopes on intact antigenic proteins. The ElliPro server located B-cell epitopes on the constructed model and found 68 residues with a score above the 0.7 threshold value (Supplementary Table S2), and collectively, these residues in a three-dimensional protein structure served as B-cell epitopes (Figures 5A, B).
Figure 5 Predicted B-cell epitopes via the ElliPro server and proteasomal cleavage sites. (A) B-cell epitopes are represented in the form of a graph where threshold value was kept 0.5. The X-axis depicts the amino acid position, whereas the Y-axis depicts the score. (B) Discontinuous B-cell epitopes (yellow) in a 3D representation. (C) NetChop prediction of proteasomal cleavage sites predicted through the IEDB server. Here, threshold was 0.5 and the green color represents the positive predictions.
To confirm the activation of cytotoxic T lymphocytes with our vaccine design, we performed proteasomal processing. A total of 104 proteasomal and 130 immunoproteasomal cleavage sites were identified during analysis with the Proteasome Cleavage Prediction server. The Proteasomal Cleavage Prediction tool of the IEDB server detected a total of 121 cleavage sites (Figure 5C).
The innate immune receptors such as pattern recognition receptors (PRRs) primarily detect the invading pathogens and initiate the immune response against them to eradicate. The TLRs are the major PRRs that are present on the immune cells to recognize the pathogen-associated molecular patterns (PAMPs) present on the pathogens. In patients with NCC, TLR4 expression was reported to be upregulated, and it plays an important role in the epileptogenesis via inflammation in response to the immune activation initiated to clear parasites from the body (54). The biological importance of the modeled vaccine was validated by its stable interactions with TLR4. The ClusPro server was employed to determine interactions; it gave the 10 top interacting models with variable levels of binding energy. The best model with the lowest binding energy and that made several stable interactions like hydrogen bonds, hydrophobic bonds, and salt bridges between the vaccine and TLR4 was visualized with the Ligplot+ server (Figure 6). Next, the HADDOCK server was employed to select the best-docked complex based on RMSD and energy information (Supplementary Table S3). HADDOCK clustered 154 structures in 13 clusters, which signifies 77% of water-refined models. The docked structure was visualized with PyMOL, whereas Ligplot was utilized to identify residual interactions. HADDOCK score and energy graph (electrostatic energy and van der Waals energy) details are also provided in Supplementary Figure 2.
Figure 6 (A) The interaction between TLR4 (Yellow) and vaccine (Red) construct having the lowest binding energy confirmed the biological importance of the constructed vaccine. The docked structure is visualized through PyMOL software (B) Ligplot+ confirming the various types of interactions in the complex. Dark green represents the hydrogen bonds, and dark red and blue bars represent the hydrophobic interactions.
MD simulations were carried out using the GROMACS server for 100 ns to estimate the stability between the constructed vaccine and TLR4. It calculated the RMSD, radius of gyration (Rg), and number of hydrogen bonds for the docked complex. The RMSD plot of the docked structure has a value of 5 ± 0.05 Å, indicating a stable complex (Figure 7A). The stability of the structure is consistent throughout the 100-ns simulation. The Rg value, which represents the density of the structure, was approximately 3.12 ± 0.05 for the initial 20 ns and then reduced to 3.08 ± 0.05 and remained almost constant (Figure 7B). Strong hydrogen bonding further reinforced the compactness of the structure (Figure 7C). Overall, the complex assembled between the vaccine and the receptor demonstrates robust stability and interplay. This implies that the vaccine possesses the capability to bind to the receptor with potent interactions, leading to the elicitation of a substantial immune response.
Figure 7 (A) The RMSD plot obtained after performing MD simulations shows the stability of the docked complex. The RMSD values are fluctuating during the initial nanoseconds but becomes stable after 10 ns. (B) The plotted radius of gyration demonstrates the compactness of the vaccine–receptor complex. The Rg value is obtained in nm and determines the average distance between the docked complex and their center of mass. (C) The H-bond plot showing the number of hydrogen bonds formed between the receptor and designed vaccine across the simulation period.
Enhanced secondary and tertiary immune responses were observed in an in silico injection scenario. There was an elevated concentration of combined IgM+IgG, IgG1+IgG2, IgG1, and IgM antibodies (Figure 8) with increased B-cell population (especially the memory B cell), T-helper, and cytotoxic cells. Molecules related to robust immune response, particularly TGF-β, IL-2, and IFN-γ, were also elevated, whereas no allergic response was detected (Supplementary Figure 3). These observations indicate that this vaccine structure is capable of generating a strong immune response, characterized by a sustained humoral reaction. Additionally, it is anticipated to be free from any allergic responses.
Figure 8 C-ImmSimm-based prediction of immune response after administration of the constructed B-cell vaccine for 350 days post-vaccination by the designed vaccine. (A) Vaccine injection leads to elevated immunoglobulin production, indicating immune response. (B) B-lymphocyte counts following the vaccine injection give an overview of the immune reaction evoked by the vaccine. (C) T-helper lymphocyte count increased at the time of vaccine injection, though the cell count decreases as the concentration of vaccine decreases. (D) The evolution of T-cytotoxic lymphocytes with each vaccine injection, marking their potential role in immune response generation. (E) Total TH cell population is markedly increased at every injection. However, the change in TH memory cell count over time depicts their increased population exclusively after the third injection. (F) Cytokine concentration that evolved during vaccine injections shows the presence of humoral immune response.
The protein sequence of the final modeled vaccine was reverse translated to DNA and then engineered for enhanced expression in the K12 (E. coli) strain. This process resulted in an enhanced CAI value of the initial sequence from 0.577 to 1.0 and GC% content from 62.421% to 54.494%. The final DNA sequence was composed of 6,401-bp nucleotides, and it was inserted between the HindIII and NotI site within the pET28a vector through in silico cloning (Figure 9).
Figure 9 In silico cloning of optimized nucleotide sequence of the constructed vaccine in pET28a+ vector plasmid (constructed in Snapgene 6.0 software), expressed between NotI and HindIII restriction sites. The nucleotide sequence of the vaccine is indicated by the red color in the vector plasmid.
The immune-informatics approach offers a fast and reliable platform to identify targets and construct vaccine in a resource-efficient and cost-effective manner (61–63). Multi-subunit vaccines offer greater flexibility in vaccine design, allowing for the incorporation of multiple antigens or epitopes from different strains or variants of a pathogen. This flexibility enables the development of vaccines that provide broad protection against diverse strains or even multiple pathogens simultaneously. This approach has shown success in designing suitable vaccine candidates against viral, bacterial, and helminthic infections (64–67). In our previous study, we have identified 57 unique T. solium proteins from the whole proteome using the immune-informatics approach alone and chosen two highly antigenic proteins to build a multi-epitope vaccine (68). However, in this investigation, we aimed to identify the conserved proteins expressed by the Taenia genus (of human importance) using bioinformatics. Taenia spp. share similarity in genome sequences, and it is reflected in their shared physical morphology; for instance, both T. solium and T. asiatica adult tapeworms have four suckers, with the former having a rostellum containing two rows of hooks and the latter having rudimentary hooklets in a wart-like formation (69). Because of the semblance in the morphology, sometimes parasitologists are not able to morphologically differentiate T. solium infection from T. asiatica infection, and vice versa, and this requires testing at the molecular level (70–73). There have been reports highlighting cysticercosis infection caused by T. solium that might actually have been caused by T. asiatica; this required a detailed study for better understanding (10, 74–76). Given the present circumstance of widespread infection of taeniasis or cysticercosis, species identification adds another challenge to the existing problem. Hence, it is prudent to vaccinate against both species. The shared genome provided valuable advantage, as it contained conserved proteins that formed the basis of our study. The proteome of both Taenia spp. was analyzed to find conserved proteins for our vaccine construct. To identify the conserved protein of importance in infection, we identified T. solium cyst stage-specific proteins by performing MALDI on T. solium CL and CW proteins and also retrieved the proteome data of CF and ESP from our previous published data (36).
The shortlisted proteins underwent a screening process as outlined above and were assessed for their efficacy and safety for human use. Since a vaccine should not result in unwanted response in host, the predicted peptide epitopes were evaluated for their allergenicity and toxicity (77–79). Next, we linked the non-allergic, non-toxic B-cell and T-cell epitopes of highly antigenic proteins using GPGPG and AAY linkers, respectively, as a single small peptide epitope cannot generate a strong immune response in the host body. The GPGPG linker was specifically used for B-cell epitopes as this promotes the breaking of junctional immunogenicity, which leads to the restoration of immunogenicity of the individual epitopes. MHC-I epitopes were joined by the AAY linker, as this linker provides the cleavage site for the proteasomes in mammalian cells. Therefore, epitopes joined using the AAY linker are separated effectively within the cells, thereby reducing the junctional immunogenicity. The AAY linker also increases the immunogenicity of the multi-epitope vaccine.
The large antigenic protein can successfully be recognized by the host immune system and activate the immune reactions (80, 81). Furthermore, secondary and tertiary structures of the build primary sequence of the vaccine were determined using the PSIPRED tool and the I-TASSER server, and these predicted structures were further validated by measuring different parameters like local and overall quality score. The final refined model was used for docking studies to confirm the biological importance of the resultant vaccine, and the low free binding energy of the complex validated the stable interactions between the TLR4 and vaccine with the help of tools like Cluspro, HADDOCK, and Ligplot+. Moreover, we performed MD simulations that demonstrate that the designed vaccine candidate can interact with the receptor with great stability and compactness. Innate immune response plays a crucial role in combating helminthic/extracellular infections, and TLRs serve as the key receptors, which are expressed by the immune cells to recognize the pathogens. The C-ImmSimm server was exploited to validate the immunoreactivity of the vaccine candidate. Immune simulation unveiled the increased level of immunoglobulins such as IgM and IgGs (IgG1 and IgG2) after a periodic exposure to antigens. Increased expression of cytokine level like TGF-β, IL-2, and IFN-γ represents good humoral response along with elevated B- and T-cell populations. We also performed in silico molecular cloning of our vaccine construct for subsequent downstream processes. Docking studies of the constructed vaccine with TLR validated the in vivo application of the vaccine. However, it needs experimental validation to confirm the protection efficiency of the constructed vaccine. Despite the significant advances in the bioinformatics-based vaccine design that are very accurate and effective, immune-informatics has its limitations. One of the major shortcomings of immune-informatics is the difficulty in selecting an appropriate animal model to validate the findings of potential vaccine candidates. Additionally, this approach can easily identify linear and non-linear epitopes. Yet, some of the predicted epitopes may remain hidden deep inside the target protein, making them challenging in terms of recognition by antibodies under in vivo conditions (82). The precision of the immunoinformatic approach is constrained by the proficiency and consistency of the algorithms. Therefore, in vitro and in vivo studies are required to validate the true efficacy of the designed vaccine for Taenia.
Soil/water-transmitted helminths are considered as neglected tropical diseases by the WHO due to their widespread prevalence in the developing countries of the world. Taenia spp. imposes a significant threat to the human race due to the unavailability of readily available diagnostic tools and vaccines for effectively preventing infections in populations at risk. In this study, we utilized bioinformatics and proteomics tools to identify a potential protein vaccine candidate for human use. We constructed a multi-epitope chimera vaccine by linking together conserved epitopes expressed by T. solium and T. asiatica and evaluated it across a range of parameters. Our study yielded a vaccine construct of optimal performance. We anticipate that our modeled vaccine will demonstrate promising efficacy against Taenia spp.
The original contributions presented in the study are included in the article/Supplementary Material. Further inquiries can be directed to the corresponding authors.
The animal study was approved by Institute Ethics Committee, Animal Ethics Committee (IIT/IAEC/2023/003). The study was conducted in accordance with the local legislation and institutional requirements.
SS: Data curation, Formal analysis, Investigation, Methodology, Validation, Writing – original draft. US: Data curation, Formal analysis, Investigation, Methodology, Validation, Writing – original draft. RK: Data curation, Formal analysis, Investigation, Software, Writing – original draft. AC: Formal analysis, Writing – original draft. SR: Data curation, Formal analysis, Methodology, Writing – original draft. AK: Formal analysis, Investigation, Methodology, Writing – original draft. NA: Conceptualization, Validation, Writing – original draft, Writing – review & editing. AP: Conceptualization, Funding acquisition, Investigation, Project administration, Resources, Supervision, Validation, Visualization, Writing – original draft, Writing – review & editing.
The author(s) declare financial support was received for the research, authorship, and/or publication of this article. AP is supported by research grants from Indian Council of Medical Research 92/03/2021-Omics/TF/MS and IIT Mandi iHub and HCI Foundation grant number IT MANDI iHub/RD/2023/0010.
SS and RK are supported by PhD studentship from the Indian Institute of Technology Mandi. SR and AK acknowledge the Department of Biotechnology, Government of India and Council for Scientific and Industrial Training, New Delhi, respectively, for financial support. AP acknowledges financial support from Ramalingaswami Fellowship, Department of Biotechnology, Government of India, New Delhi.
The authors declare that the research was conducted in the absence of any commercial or financial relationships that could be construed as a potential conflict of interest.
The author(s) declared that they were an editorial board member of Frontiers, at the time of submission. This had no impact on the peer review process and the final decision.
All claims expressed in this article are solely those of the authors and do not necessarily represent those of their affiliated organizations, or those of the publisher, the editors and the reviewers. Any product that may be evaluated in this article, or claim that may be made by its manufacturer, is not guaranteed or endorsed by the publisher.
This manuscript is a part of M. Tech thesis of US, submitted to IIT Mandi.
The Supplementary Material for this article can be found online at: https://www.frontiersin.org/articles/10.3389/fitd.2024.1393570/full#supplementary-material
1. Jourdan PM, Lamberton PHL, Fenwick A, Addiss DG. Soil-transmitted helminth infections. Lancet. (2018) 391:252–65. doi: 10.1016/S0140-6736(17)31930-X
2. WHO. WHO guideline on management of T.solium Neurocysticercosis. (2021). Geneva: World Health Organization; 2021. Licence: CC BY-NC-SA 3.0 IGO.
3. Prasad A, Prasad KN, Yadav A, Gupta RK, Pradhan S, Jha S, et al. Lymphocyte transformation test: a new method for diagnosis of neurocysticercosis. Diagn Microbiol Infect Dis. (2008) 61:198–202. doi: 10.1016/j.diagmicrobio.2007.12.016
4. Coyle CM, Mahanty S, Zunt JR, Wallin MT, Cantey PT, White AC Jr., et al. Neurocysticercosis: neglected but not forgotten. PloS Negl Trop Dis. (2012) 6:e1500. doi: 10.1371/journal.pntd.0001500
5. Laranjo-Gonzalez M, Devleesschauwer B, Trevisan C, Allepuz A, Sotiraki S, Abraham A, et al. Epidemiology of taeniosis/cysticercosis in Europe, a systematic review: Western Europe. Parasit Vectors. (2017) 10:349. doi: 10.1186/s13071-017-2280-8
6. Zammarchi L, Strohmeyer M, Bartalesi F, Bruno E, Munoz J, Buonfrate D, et al. Epidemiology and management of cysticercosis and Taenia solium taeniasis in Europe, systematic review 1990-2011. PloS One. (2013) 8:e69537. doi: 10.1371/journal.pone.0069537
7. Anantaphruti MT, Yamazaki H, Nakao M, Waikagul J, Watthanakulpanich D, Nuamtanong S, et al. Occurrence of Taenia solium, T. saginata and T. asiatica, Thailand. Emerg Infect Dis. (2007) 13:1413–6. doi: 10.3201/eid1309.061148
8. Flisser A. State of the art of Taenia solium as compared to Taenia asiatica. Korean J Parasitol. (2013) 51:43–9. doi: 10.3347/kjp.2013.51.1.43
9. Li T, Openshaw JJ, Chen X, Medina AC, Felt SA, Zhou H, et al. High prevalence of Taenia solium taeniasis and cysticercosis in Tibetan schoolchildren in western Sichuan, China: a cross-sectional study. Lancet. (2017) 390:S89. doi: 10.1016/S0140-6736(17)33227-0
10. Singh SK, Prasad KN, Singh AK, Gupta KK, Chauhan RS, Singh A, et al. Identification of species and genetic variation in Taenia isolates from human and swine of North India. Parasitol Res. (2016) 115:3689–93. doi: 10.1007/s00436-016-5186-z
11. Arora N, Tripathi S, Sao R, Mondal P, Mishra A, Prasad A. Molecular neuro-pathomechanism of neurocysticercosis: how host genetic factors influence disease susceptibility. Mol Neurobiol. (2018) 55:1019–25. doi: 10.1007/s12035-016-0373-6
12. Carpio A. Neurocysticercosis and epilepsy. Novel aspects on cysticercosis and neurocysticercosis. IntechOpen (2013). doi: 10.5772/52389
13. Havelaar AH, Kirk MD, Torgerson PR, Gibb HJ, Hald T, Lake RJ, et al. World health organization global estimates and regional comparisons of the burden of foodborne disease in 2010. PloS Med. (2015) 12:e1001923. doi: 10.1371/journal.pmed.1001923
14. Gonzales I, Rivera JT, Garcia HH, Cysticercosis Working Group in P. Pathogenesis of Taenia solium taeniasis and cysticercosis. Parasite Immunol. (2016) 38:136–46. doi: 10.1111/pim.12307
15. Prasad KN, Prasad A, Gupta RK, Pandey CM, Singh U. Prevalence and associated risk factors of Taenia solium taeniasis in a rural pig farming community of north India. Trans R Soc Trop Med Hyg. (2007) 101:1241–7. doi: 10.1016/j.trstmh.2007.04.019
16. Prasad KN, Prasad A, Verma A, Singh AK. Human cysticercosis and Indian scenario-a review. J Biosci. (2008) 33:571–82. doi: 10.1007/s12038-008-0075-y
17. Debacq G, Moyano LM, Garcia HH, Boumediene F, Marin B, Ngoungou EB, et al. Systematic review and meta-analysis estimating association of cysticercosis and neurocysticercosis with epilepsy. PloS Negl Trop Dis. (2017) 11:e0005153. doi: 10.1371/journal.pntd.0005153
18. Garcia HH, Gonzalez AE, Evans CA, Gilman RH. Cysticercosis Working Group in P. Taenia solium cysticercosis. Lancet. (2003) 362:547–56. doi: 10.1016/S0140-6736(03)14117-7
19. Verastegui MR, Mejia A, Clark T, Gavidia CM, Mamani J, Ccopa F, et al. Novel rat model for neurocysticercosis using Taenia solium. Am J Pathol. (2015) 185:2259–68. doi: 10.1016/j.ajpath.2015.04.015
20. Prasad KN, Prasad A, Gupta RK, Nath K, Pradhan S, Tripathi M, et al. Neurocysticercosis in patients with active epilepsy from the pig farming community of Lucknow district, north India. Trans R Soc Trop Med Hyg. (2009) 103:144–50. doi: 10.1016/j.trstmh.2008.07.015
21. Arora N, Kaur R, Anjum F, Tripathi S, Mishra A, Kumar R, et al. Neglected agent eminent disease: linking human helminthic infection, inflammation, and Malignancy. Front Cell Infect Microbiol. (2019) 9:402. doi: 10.3389/fcimb.2019.00402
22. Werkman M, Wright JE, Truscott JE, Oswald WE, Halliday KE, Papaiakovou M, et al. The impact of community-wide, mass drug administration on aggregation of soil-transmitted helminth infection in human host populations. Parasit Vectors. (2020) 13:290. doi: 10.1186/s13071-020-04149-4
23. Webster JP, Molyneux DH, Hotez PJ, Fenwick A. The contribution of mass drug administration to global health: past, present and future. Philos Trans R Soc Lond B Biol Sci. (2014) 369:20130434. doi: 10.1098/rstb.2013.0434
24. Bagheri H, Simiand E, Montastruc JL, Magnaval JF. Adverse drug reactions to anthelmintics. Ann Pharmacother. (2004) 38:383–8. doi: 10.1345/aph.1D325
25. Cárdenas G, Carrillo-Mezo R, Jung H, Sciutto E, Hernandez JL, Fleury A, et al. Subarachnoidal Neurocysticercosis non-responsive to cysticidal drugs- a case series. BMC Neurol. (2010) 10:1–5. doi: 10.1186/1471-2377-10-16
26. Geerts SGB. Drug resistance in human helminths- current situation and lessons from livestock. Clin Microbiol Rev. (2000) 13:207–22. doi: 10.1128/CMR.13.2.207
27. Kaur R, Arora N, Rawat SS, Keshri AK, Sharma SR, Mishra A, et al. Vaccine for a neglected tropical disease Taenia solium cysticercosis: fight for eradication against all odds. Expert Rev Vaccines. (2021) 20:1447–58. doi: 10.1080/14760584.2021.1967750
28. Wang YY, Chang XL, Tao ZY, Wang XL, Jiao YM, Chen Y, et al. Optimized codon usage enhances the expression and immunogenicity of DNA vaccine encoding Taenia solium oncosphere TSOL18 gene. Mol Med Rep. (2015) 12:281–8. doi: 10.3892/mmr.2015.3387
29. Zhou BY, Sun JC, Li X, Zhang Y, Luo B, Jiang N, et al. Analysis of Immune Responses in Mice Orally Immunized with Recombinant pMG36e-SP-TSOL18/Lactococcus lactis and pMG36e-TSOL18/Lactococcus lactis Vaccines of Taenia solium. J Immunol Res. (2018) 2018:9262631. doi: 10.1155/2018/9262631
30. Sanches RCO, Tiwari S, Ferreira LCG, Oliveira FM, Lopes MD, Passos MJF, et al. Immunoinformatics design of multi-epitope peptide-based vaccine against Schistosoma mansoni using transmembrane proteins as a target. Front Immunol. (2021) 12:621706. doi: 10.3389/fimmu.2021.621706
31. Kaur R, Arora N, Jamakhani MA, Malik S, Kumar P, Anjum F, et al. Development of multi-epitope chimeric vaccine against Taenia solium by exploring its proteome: an in silico approach. Expert Rev Vaccines. (2020) 19:105–14. doi: 10.1080/14760584.2019.1711057
32. Kaur R, Arora N, Rawat SS, Keshri AK, Singh N, Show SK, et al. Immunoinformatics driven construction of multi-epitope vaccine candidate against Ascaris lumbricoides using its entire immunogenic epitopes. Expert Rev Vaccines. (2021) 20:1637–49. doi: 10.1080/14760584.2021.1974298
33. Shey RA, Ghogomu SM, Esoh KK, Nebangwa ND, Shintouo CM, Nongley NF, et al. In-silico design of a multi-epitope vaccine candidate against onchocerciasis and related filarial diseases. Sci Rep. (2019) 9:4409. doi: 10.1038/s41598-019-40833-x
34. Amit P, Prasad KN, Kumar GR, Shweta T, Sanjeev J, Kumar PV, et al. Immune response to different fractions of Taenia solium cyst fluid antigens in patients with neurocysticercosis. Exp Parasitol. (2011) 127:687–92. doi: 10.1016/j.exppara.2010.11.006
35. Arora N, Kaur R, Rawat SS, Kumar A, Singh AK, Tripathi S, et al. Evaluation of Taenia solium cyst fluid-based enzyme linked immunoelectro transfer blot for Neurocysticercosis diagnosis in urban and highly endemic rural population of North India. Clin Chim Acta. (2020) 508:16–21. doi: 10.1016/j.cca.2020.05.006
36. Arora N, Prasad A. Taenia solium proteins: a beautiful kaleidoscope of pro and anti-inflammatory antigens. Expert Rev Proteomics. (2020) 17:609–22. doi: 10.1080/14789450.2020.1829486
37. Teufel F, Almagro Armenteros JJ, Johansen AR, Gislason MH, Pihl SI, Tsirigos KD, et al. SignalP 6.0 predicts all five types of signal peptides using protein language models. Nat Biotechnol. (2022) 40:1023–5. doi: 10.1038/s41587-021-01156-3
38. Krogh A, Larsson B, von Heijne G, Sonnhammer EL. Predicting transmembrane protein topology with a hidden Markov model: application to complete genomes. J Mol Biol. (2001) 305:567–80. doi: 10.1006/jmbi.2000.4315
39. Almagro Armenteros JJ, Sonderby CK, Sonderby SK, Nielsen H, Winther O. DeepLoc: prediction of protein subcellular localization using deep learning. Bioinformatics. (2017) 33:3387–95. doi: 10.1093/bioinformatics/btx431
40. Zhao L, Poschmann G, Waldera-Lupa D, Rafiee N, Kollmann M, Stuhler K. OutCyte: a novel tool for predicting unconventional protein secretion. Sci Rep. (2019) 9:19448. doi: 10.1038/s41598-019-55351-z
41. Vita R, Mahajan S, Overton JA, Dhanda SK, Martini S, Cantrell JR, et al. The immune epitope database (IEDB): 2018 update. Nucleic Acids Res. (2019) 47:D339–D43. doi: 10.1093/nar/gky1006
42. Maurer-Stroh S, Krutz NL, Kern PS, Gunalan V, Nguyen MN, Limviphuvadh V, et al. AllerCatPro-prediction of protein allergenicity potential from the protein sequence. Bioinformatics. (2019) 35:3020–7. doi: 10.1093/bioinformatics/btz029
43. Dhanda SK, Vir P, Raghava GP. Designing of interferon-gamma inducing MHC class-II binders. Biol Direct. (2013) 8:30. doi: 10.1186/1745-6150-8-30
44. Wilkins MR, Gasteiger E, Bairoch A, Sanchez JC, Williams KL, Appel RD, et al. Protein identification and analysis tools in the ExPASy server. Methods Mol. (1999) 112:531–52. doi: 10.1385/1-59259-584-7:531
45. McGuffin LJ, Bryson K, Jones DT. The PSIPRED protein structure prediction server. Bioinf (Oxford England). (2000) 16:404– 5. doi: 10.1093/bioinformatics/16.4.404
46. Yang J, Zhang Y. I-TASSER server: new development for protein structure and function predictions. Nucleic Acids Res. (2015) 43:W174–81. doi: 10.1093/nar/gkv342
47. Bhattacharya D, Nowotny J, Cao R, Cheng J. 3Drefine: an interactive web server for efficient protein structure refinement. Nucleic Acids Res. (2016) 44:W406–9. doi: 10.1093/nar/gkw336
48. Heo L, Park H, Seok C. GalaxyRefine: Protein structure refinement driven by side-chain repacking. Nucleic Acids Res. (2013) 41:W384–8. doi: 10.1093/nar/gkt458
49. Wiederstein M, Sippl MJ. ProSA-web: interactive web service for the recognition of errors in three-dimensional structures of proteins. Nucleic Acids Res. (2007) 35:W407–10. doi: 10.1093/nar/gkm290
50. Ponomarenko J, Bui HH, Li W, Fusseder N, Bourne PE, Sette A, et al. ElliPro: a new structure-based tool for the prediction of antibody epitopes. BMC Bioinf. (2008) 9:514. doi: 10.1186/1471-2105-9-514
51. Gomez-Perosanz M, Ras-Carmona A, Reche PA. PCPS: A web server to predict proteasomal cleavage sites. Methods Mol Biol. (2020) 2131:399–406. doi: 10.1007/978-1-0716-0389-5_23
52. Diez-Rivero CM, Lafuente EM, Reche PA. Computational analysis and modeling of cleavage by the immunoproteasome and the constitutive proteasome. BMC Bioinf. (2010) 11:1–13. doi: 10.1186/1471-2105-11-479
53. Mishra BB, Mishra PK, Teale JM. Expression and distribution of Toll-like receptors in the brain during murine neurocysticercosis. J Neuroimmunol. (2006) 181:46–56. doi: 10.1016/j.jneuroim.2006.07.019
54. Verma A, Prasad KN, Gupta RK, Singh AK, Nyati KK, Rizwan A, et al. Toll-like receptor 4 polymorphism and its association with symptomatic neurocysticercosis. J Infect Dis. (2010) 202:1219–25. doi: 10.1086/656395
55. Comeau SR, Gatchell DW, Vajda S, Camacho CJ. ClusPro: a fully automated algorithm for protein-protein docking. Nucleic Acids Res. (2004) 32:W96–9. doi: 10.1093/nar/gkh354
56. Kozakov D, Hall DR, Xia B, Porter KA, Padhorny D, Yueh C, et al. The ClusPro web server for protein-protein docking. Nat Protoc. (2017) 12:255–78. doi: 10.1038/nprot.2016.169
57. Wallace AC LR, Thornton JM. LIGPLOT- a program to generate schematic diagrams of protein-ligand interactions. Protein Eng Des Sel. (1995) 8(2):127–34. doi: 10.1093/protein/8.2.127
58. Rapin N, Lund O, Bernaschi M, Castiglione F. Computational immunology meets bioinformatics: the use of prediction tools for molecular binding in the simulation of the immune system. PloS One. (2010) 5:e9862. doi: 10.1371/journal.pone.0009862
59. Grote A, Hiller K, Scheer M, Munch R, Nortemann B, Hempel DC, et al. JCat: a novel tool to adapt codon usage of a target gene to its potential expression host. Nucleic Acids Res. (2005) 33:W526–31. doi: 10.1093/nar/gki376
60. Stothard P. The sequence manipulation suite- JavaScript programs for analyzing and formatting protein and DNA sequences. Biotechniques June. (2000) 28:1102–4. doi: 10.2144/00286ir01
61. Doytchinova ITP, Flower D. Proteomics in vaccinology and immunobiology : an informatics perspective of the immunone. J BioMed Biotecchnol. (2003) 2003(5):267–90. doi: 10.1155/S1110724303209232
62. Tilocca B, Britti D, Urbani A, Roncada P. Computational immune proteomics approach to target COVID-19. J Proteome Res. (2020) 19:4233–41. doi: 10.1021/acs.jproteome.0c00553
63. Yousafi Q, Amin H, Bibi S, Rafi R, Khan MS, Ali H, et al. Subtractive proteomics and immuno-informatics approaches for multi-peptide vaccine prediction against Klebsiella oxytoca and validation through in silico expression. Int J Pept Res Ther. (2021) 27:2685–701. doi: 10.1007/s10989-021-10283-z
64. Miles S, Portela M, Cyrklaff M, Ancarola ME, Frischknecht F, Duran R, et al. Combining proteomics and bioinformatics to explore novel tegumental antigens as vaccine candidates against Echinococcus granulosus infection. J Cell Biochem. (2019) 120:15320–36. doi: 10.1002/jcb.28799
65. Alabbas AB. Integrativesubtractive proteomics, immunoinformatics, docking, and simulation approaches reveal candidate vaccine against Sin Nombre orthohantavirus. Front Immunol. (2022) 13:1022159. doi: 10.3389/fimmu.2022.1022159
66. Soto LF, Romani AC, Jimenez-Avalos G, Silva Y, Ordinola-Ramirez CM, Lopez Lapa RM, et al. Immunoinformatic analysis of the whole proteome for vaccine design: An application to Clostridium perfringens. Front Immunol. (2022) 13:942907. doi: 10.3389/fimmu.2022.942907
67. Tahir ul Qamar M, Ahmad S, Fatima I, Ahmad F, Shahid F, Naz A, et al. Designing multi-epitope vaccine against Staphylococcus aureus by employing subtractive proteomics, reverse vaccinology and immuno-informatics approaches. Comput Biol Med. (2021) 132:104389. doi: 10.1016/j.compbiomed.2021.104389
68. Kaur R, Arora N, Rawat SS, Keshri AK, Singh G, Kumar R, et al. Recognition of immune reactive proteins as a potential multiepitope vaccine candidate of Taenia solium cysticerci through proteomic approach. J Cell Biochem. (2023) 124:1587–602. doi: 10.1002/jcb.30467
69. Nakao M, Lavikainen A, Iwaki T, Haukisalmi V, Konyaev S, Oku Y, et al. Molecular phylogeny of the genus Taenia (Cestoda: Taeniidae): proposals for the resurrection of Hydatigera Lamarck, 1816 and the creation of a new genus Versteria. Int J Parasitol. (2013) 43:427–37. doi: 10.1016/j.ijpara.2012.11.014
70. Hoberg EP. Phylogeny of Taenia: Species definitions and origins of human parasites. Parasitol Int. (2006) 55 Suppl:S23–30. doi: 10.1016/j.parint.2005.11.049
71. Flisser A, Viniegra AE, Aguilar-Vega L, Garza-Rodriguez A, Maravilla P, Avila G. Portrait of human tapeworms. J Parasitol. (2004) 90:914–6. doi: 10.1645/GE-3354CC
72. Sato MO, Sato M, Yanagida T, Waikagul J, Pongvongsa T, Sako Y, et al. Taenia solium, Taenia saginata, Taenia asiatica, their hybrids and other helminthic infections occurring in a neglected tropical diseases’ highly endemic area in Lao PDR. PloS Negl Trop Dis. (2018) 12:e0006260. doi: 10.1371/journal.pntd.0006260
73. Ng-Nguyen D, Stevenson MA, Dorny P, Gabriel S, Vo TV, Nguyen VT, et al. Comparison of a new multiplex real-time PCR with the Kato Katz thick smear and copro-antigen ELISA for the detection and differentiation of Taenia spp. in human stools. PloS Negl Trop Dis. (2017) 11:e0005743. doi: 10.1371/journal.pntd.0005743
74. Eom KS, Rim HJ, Jeon HK. Taenia asiatica: Historical overview of taeniasis and cysticercosis with molecular characterization. Adv Parasitol. (2020) 108:133–73. doi: 10.1016/bs.apar.2019.12.004
75. Galan-PuChades MT, Fuentes MV. Taenia asiatica: the most neglected human Taenia and the possibility of cysticercosis. Korean J Parasitol. (2013) 51:51–4. doi: 10.3347/kjp.2013.51.1.51
76. Galan-PuChades MT FM. Human cysticercosis and larval tropism of Taenia asiatica. Parasitol Today. (2000) 16:174. doi: 10.1016/S0169-4758(99)01630-0
77. Barbosa LC, Garrido SS, Marchetto R. BtoxDB: a comprehensive database of protein structural data on toxin-antitoxin systems. Comput Biol Med. (2015) 58:146–53. doi: 10.1016/j.compbiomed.2015.01.010
78. Negi SS, Schein CH, Ladics GS, Mirsky H, Chang P, Rascle JB, et al. Functional classification of protein toxins as a basis for bioinformatic screening. Sci Rep. (2017) 7:13940. doi: 10.1038/s41598-017-13957-1
79. Ivanciuc O SC, Braun W. Data mining of sequences and 3D structures of allergenic proteins. Bioinformatics. (2002) 18:1358–64. doi: 10.1093/bioinformatics/18.10.1358
80. Shamriz S, Ofoghi H, Moazami N. Effect of linker length and residues on the structure and stability of a fusion protein with malaria vaccine application. Comput Biol Med. (2016) 76:24–9. doi: 10.1016/j.compbiomed.2016.06.015
81. Bin Sayed S, Nain Z, Abdullah F, khan MSA, Haque Z, Rahman SMR, et al. Immunoinformatics-guided designing of peptide vaccine against Lassa virus with dynamic and immune simulation studies. Preprints. (2019), 2019090076. doi: 10.20944/preprints201909.0076.v2
Keywords: helminths, Taenia genus, MALDI, immune-informatics, vaccine design, molecular dynamic simulations, cysticercosis
Citation: Sharma S, Sharan U, Kaur R, Chaudhary A, Rawat SS, Keshri AK, Arora N and Prasad A (2024) An inclusive approach to designing a multi-epitope chimeric vaccine for Taenia infections by integrating proteomics and reverse vaccinology. Front. Trop. Dis 5:1393570. doi: 10.3389/fitd.2024.1393570
Received: 29 February 2024; Accepted: 15 April 2024;
Published: 17 May 2024.
Edited by:
Arghya Das, AIIMS, IndiaReviewed by:
Robert Adamu Shey, University of Buea, CameroonCopyright © 2024 Sharma, Sharan, Kaur, Chaudhary, Rawat, Keshri, Arora and Prasad. This is an open-access article distributed under the terms of the Creative Commons Attribution License (CC BY). The use, distribution or reproduction in other forums is permitted, provided the original author(s) and the copyright owner(s) are credited and that the original publication in this journal is cited, in accordance with accepted academic practice. No use, distribution or reproduction is permitted which does not comply with these terms.
*Correspondence: Naina Arora, bmFpbmFhLmFyb3JhQGdtYWlsLmNvbQ==; Amit Prasad, YW1pdHByYXNhZEBpaXRtYW5kaS5hYy5pbg==
†These authors have contributed equally to this work
Disclaimer: All claims expressed in this article are solely those of the authors and do not necessarily represent those of their affiliated organizations, or those of the publisher, the editors and the reviewers. Any product that may be evaluated in this article or claim that may be made by its manufacturer is not guaranteed or endorsed by the publisher.
Research integrity at Frontiers
Learn more about the work of our research integrity team to safeguard the quality of each article we publish.