- 1Department of Global Health, University of Washington, Seattle, WA, United States
- 2The DeWorm3 Project, University of Washington, Seattle, WA, United States
- 3The Wellcome Trust Research Laboratory, Division of Gastrointestinal Sciences, Christian Medical College, Vellore, India
- 4Institut de Recherche Clinique du Bénin, Abomey-Calavi, Benin
- 5Department of Ophthalmology, Blantyre Institute for Community Outreach (BICO), Lions Sight First Eye Hospital, Blantyre, Malawi
- 6Kamuzu University of Health Sciences, Blantyre, Malawi
- 7Centre de Recherche pour la Lutte Contre les Maladies Infectieuses Tropicales (CReMIT/TIDRC), Université d’Abomey-Calavi, Abomey-Calavi, Benin
- 8Departments of Medicine, Pediatrics, & Epidemiology, University of Washington, Seattle, WA, United States
Introduction: Large community-based public health programs, like mass drug administration (MDA), require coordination across many stakeholders. We used social network analysis (SNA) to systematically identify the network of stakeholders who influence delivery of school-based and community-wide MDA for soil-transmitted helminths (STH) in Benin, India, and Malawi and determine how network dynamics may impact implementation and scale-up across these delivery platforms.
Methods: This study was embedded within the implementation science research of the DeWorm3 Project, a hybrid clinical trial in Benin, India, & Malawi testing the feasibility of STH transmission interruption via community-wide MDA. Sites developed lists of stakeholders engaged in both MDA programs and indicated stakeholders’ attitudes towards the intervention and influence over intervention delivery. We developed digital sociograms for both MDA networks by site, comparing baseline vs. endline. We descriptively compared changes over time in stakeholder attitudes and influence and key SNA measures, including centrality, centralization, and density.
Results: Across sites, we identified an expansive network of stakeholders involved in delivery of school-based (N= 139, 63, 58 vs. N=139, 162, 63) and community-wide MDA programs (N=52, 137, 54 vs. N=54, 136, 60) at baseline vs. endline in Benin, India, and Malawi, respectively. At both timepoints, a majority (>70%) of stakeholders held positive attitudes towards both programs. For both programs, stakeholders with the highest degree centrality scores (i.e., the most connected individuals) were those responsible for implementation such as community drug distributors or school teachers, while those with the highest betweenness centrality scores (i.e. those who controlled resource or information flow across networks) were responsible for policy-making & program leadership (e.g., NTD Program Managers). Low density scores indicated networks had poor overall connectedness due to minimal connectivity across administrative levels, while low centralization scores reflected stable networks where no single individual exhibited high control over resource flow.
Conclusion: During stages of innovation, redesign, or scale-up, analyzing the network of policymakers and implementers provides an opportunity to optimize effectiveness and efficiency of public health programs. Study findings provide useful insight for NTD policymakers and implementers in STH-endemic countries aiming to successfully interrupt STH transmission by transitioning from school-based to community-wide MDA.
Introduction
Approximately 1.5 billion people globally are infected with soil-transmitted helminths (STHs), a group of intestinal worms predominantly affecting poverty-stricken populations in low- and middle-income countries (LMICs) (1). Chronic or high-intensity STH infection can lead to various nutritional, cognitive, or physical morbidities, especially amongst children (1). Current World Health Organization (WHO) STH guidelines thus recommend morbidity control via school-based mass drug administration (MDA) of deworming medicines primarily targeting pre-school age and school-age children (2). School-based deworming is a cost-effective intervention for reducing STH-related outcomes (3–6), reaching more than 600 million children annually in 2019 (7). However, mathematical models suggest that untreated individuals, including adults not targeted in school-based programs, continue to serve as important reservoirs of reinfection in many settings (8–10). Emerging evidence suggests that expanding deworming to community members of all ages via a community-wide MDA platform has the potential to effectively interrupt the transmission of STH, preventing both pediatric morbidity and community reinfection (5, 11).
Prior to launching any new intervention, such as community-wide MDA for STH, it is essential to understand the networks of individuals who impact intervention delivery and subsequent scale-up through their unique roles in program delivery. Stakeholder networks create pathways for the diffusion of attitudes, knowledge, behaviors, and resources (12, 13). During periods of fundamental innovation, redesign, or scale-up of a health program, analyzing the social network of policymakers, implementers, and other relevant stakeholders provides an opportunity to optimize effectiveness and efficiency of public health programs, particularly those with limited resources (12, 14). For example, networks that rely on single or few individuals to funnel information or provide supervision can experience intervention delivery bottlenecks through slow, ineffective, or harmful resource diffusion, especially if the individual is not well-connected to the rest of the network. In contrast, highly-connected and optimally-sized networks with numerous influential stakeholders can hasten diffusion and facilitate effective intervention uptake, especially if stakeholders with a positive attitude towards the intervention can be empowered to serve as champions to build trust with recipient populations.
In this study, we describe and analyze networks of stakeholders for school-based and community-wide MDA for STH in order to identify individuals who influence program delivery and to gain a better understanding of how network dynamics may impact program implementation and scale-up, particularly of a new community-wide MDA program for STH transmission interruption. We describe how networks change over time to understand the fluidity of intervention network structures and assess whether increased intervention exposure may impact intervention acceptability and delivery.
Materials and methods
Study design and setting
Our study is embedded within the DeWorm3 Project, a multi-country hybrid implementation-effectiveness community cluster randomized clinical trial in Benin (Comé commune), India (Timiri and Jawadhu Hills blocks in Vellore and Thiruvanamalai districts, Tamil Nadu state), and Malawi (Mangochi district) testing the feasibility of interrupting STH transmission (15). Over three years (2017-2020), all eligible community members in intervention clusters received bi-annual community-wide MDA, while those in control clusters received bi-annual or annual school-based MDA in accordance with national STH guidelines (15). Embedded in the clinical trial are a series of implementation science research aims to evaluate the factors, internal and external to the intervention context, that impact successful implementation of community-wide MDA for STH i.e., achieving high deworming coverage (16). As part of DeWorm3 implementation science research, we conducted stakeholder mapping to systematically identify and analyze the network of stakeholders involved in MDA delivery (16).
Data collection
All data were collected by site implementation science research teams, which underwent a standardized training during study planning. Across sites, study participants primarily included government officials from the Ministry of Health (MOH) and the Ministry of Education (MOE), as well as other community-based or non-governmental organizations involved in the delivery of school-based MDA for STH or community-wide MDA programs for other neglected tropical diseases (NTDs) highly-prevalent in the country. At trial baseline and endline, the Benin and Malawi study teams facilitated stakeholder mapping workshops that included at least one representative from each aforementioned institution. In India, geographic dispersion of participants across administrative levels required adapting data collection to utilize a series of key informant interviews (KIIs) with snowball sampling as the single workshop format was not feasible.
At baseline, participants developed a list of stakeholders at each administrative level who support delivery of school-based and, separately, community-wide MDA. Across sites, a stakeholder was typically an individual (e.g., NTD Program Director), although, for large cadres of individuals, a stakeholder could also be a discrete group of individuals (e.g., teachers). The following descriptive information was collected for each identified stakeholder: name, organization or Ministry, job title, and administrative level (National to community/village). Participants then designated stakeholder attributes, including attitude towards MDA (positive, neutral, or negative) and influence over successful MDA implementation (high, medium, or low). The research team specified attributes for participants who were included in the map or during interviewing as in India. Attitude and implementation influence were assessed using a consensus approach where, after collaborative discussion, a majority of participants or site research team members agreed on each rating (17). After building these stakeholder identification lists, participants or researchers then developed hand-drawn sociogram graphs (i.e., network diagrams that include individual stakeholders with connecting lines indicating the relationship between two given individuals). Relationships between stakeholders were categorized as: supervisory (i.e., one stakeholder had direct authority of another), financial (i.e., one stakeholder provided financial support to another), technical assistance (i.e., one stakeholder provided targeted implementation support to another, excluding financial assistance), or formal communication (i.e., the two stakeholders did not hold any official relationship, yet formally communicate during MDA implementation). At each site, two stakeholder lists and two maps were developed separately for each MDA delivery platform (school-based and community-wide MDA).
At study endline, a second round of workshops or KIIs were conducted where participants were provided the baseline lists of stakeholders and their relationships and asked to update the baseline data. Updates included adding any new stakeholders or relationships that developed over the past three years of community-wide MDA implementation, removing any stakeholders due to changes in the NTD or STH ecosystem during trial implementation (e.g., job transfers), and updating attitudes towards the intervention. For stakeholders identified at baseline and any newly added stakeholders, participants were asked to describe the stakeholder attitude towards the intervention at endline (positive, neutral, or negative) as well as influence over intervention scale-up (high, medium, or low). Influence over intervention scale-up was only collected for community-wide MDA given that school-based MDA is currently standard of care. When a stakeholder left their position and was replaced by another individual in the same role, the role remained on the map as part of the MDA program and any turnover was descriptively assessed.
Data analysis
We conducted a series of descriptive analyses, calculating the proportion of stakeholders by administrative level, level of influence over MDA implementation at baseline and scale-up at endline, and attitudes towards the intervention. We utilized social network analysis (SNA) to examine MDA stakeholder characteristics and evaluate the structures of relational connections within intervention networks (14, 18). SNA utilizes network and graph theory to examine structures of relationships between a group of individuals by evaluating the positions of and relationship between stakeholders (14, 18). In SNA, each identified stakeholder (i.e., individual or group) represents a ‘node’ in the network, and each relationship is described as an ‘edge’. Overall, SNA has the potential to improve program implementation by proactively identifying strengths and weaknesses in program coordination or information dissemination (14) has been used in several implementation studies to monitor intervention delivery (19), measure the impact of network-strengthening intervention strategies on implementation quality (19) and increase collaboration between previously disconnected network members to improve program outcomes (20). In this study, we utilized SNA to assess stakeholders’ unique influence on implementation delivery and scale – directly through their position within the network or indirectly via their interaction with other network members (14, 21). Although several studies have underscored the utility of stakeholder mapping and SNA within NTDs as it relates to describing transmission of infection, mapping treatment diffusion, and identifying the impact of social relationships on coverage and compliance (22–25), to our knowledge, this is the first application of SNA to evaluate the ecosystem of an NTD program in regards to intervention delivery.
We conducted several nodal (i.e., node-level) analyses, including degree centrality and betweenness centrality (Table 1) to assess the connectivity of individual stakeholders within the network (13, 18). At the network-level, we quantified density and centralization scores (both ranging from 0-1) to evaluate overall network connectedness (i.e., the extent of the connections of all stakeholders in a network) and fragility (i.e., how much the stability of the network centered around the connections of one or few stakeholders), respectively (Table 1) (13, 18). These network-level analyses are especially useful for understanding intervention scalability – for example, more decentralized networks suggest an intervention may be easier to scale, as the flow and resources are not bound to one or few stakeholders and are more equally shared across the intervention network (14, 21). For each MDA delivery platform, we describe similarities and differences in these measures across networks from baseline to endline, by site.
We digitized the hand-drawn maps into sociograms to visually display nodal attributes and edges and describe overall network structure. We visually identified any structural holes, which are defined by a cluster of nodes that lack connections to other nodes or node clusters and are thus isolated from the rest of the network (14, 18). All analyses were conducted using RStudio V.1.4.1717 using the igraph package (26, 27).
Results
Stakeholder network for community-wide MDA
Network composition
Stakeholders in the community-wide MDA networks included a variety of stakeholders at various administrative levels. At the national & regional levels, these included Ministry personnel who define policies and provide program leadership as well as various partners, including pharmaceutical companies, and multilateral and non-governmental organizations that support program planning, implementation, and evaluation. The sub-regional & health center levels included MDA program managers who design and oversee program planning & implementation and health facility workers who support program management, manage local supply chains, monitor MDA for adverse events, and supervise community drug distributors (CDDs) and community health workers [CHWs, known as health service assistants (HSAs) in Malawi and accredited social health activists (ASHAs) in India]. Lastly, the community level stakeholders included CDDs and CHWs who conduct community sensitization and administer deworming drugs as well as village leaders and community-based organizations and community groups such as women’s associations who mobilize community members.
Across sites, a total of 243 and 288 unique stakeholders were identified in the community-wide MDA networks at baseline and endline, respectively (Figure 1). Across sites, the largest number of stakeholders were at the health center/block and community levels. Overall, we observed a minimal difference in the number of stakeholders (N¾6) and number of unique relationships over time (N¾50), indicating that the networks remained fairly stable over the study period.
India identified the largest number of community-wide MDA stakeholders at both time points, (137 and 136, respectively), with Benin identifying 52 and 54 unique stakeholders and Malawi identifying 54 and 60 unique stakeholders at baseline and endline, respectively (Figure 1). Changes in the number of stakeholders were primarily driven by the introduction of new individuals into the network, either as replacement or as new additions. Over a three-year period, the changes in the number of stakeholders from baseline to endline were six in Malawi, two in Benin, and only one in India. The number of relationships in the community-wide MDA networks from baseline to endline remained relatively stable in Benin (150 vs. 152) while it decreased in India (483 vs. 433, decrease of 50 relationships) and Malawi (148 vs, 108, decrease of 40 relationships).
Attitude towards community-wide MDA intervention
We observed a slight decrease or no change in the proportion of stakeholders who had positive attitudes towards community-wide MDA at baseline (82%, 97%, and 72%), as compared to endline (77%, 96%, and 72%), in Benin, India, and Malawi, respectively. Although India and Malawi did not have any stakeholders with a negative attitude towards community wide-MDA at either time point, in Benin, approximately 10% of stakeholders, namely community-level implementers who support service delivery, held a negative attitude towards the intervention at both baseline and endline.
Influence on community-wide MDA intervention
At baseline, a majority of stakeholders in Benin and India (62% and 72%, respectively) were identified as having high influence over successful launch of community-wide MDA, as compared to 17% in Malawi. At endline, the proportion of stakeholders that were identified as having high influence of intervention scale-up were highest in Benin and Malawi (62% and 51%, respectively) as compared to India (10%). Across sites, most of these influential stakeholders were at the health center or community levels.
Across the three sites, the most connected stakeholders (i.e., with the highest degree centrality scores) were at the community and district and sub-district levels, including CDDs and CHWs, village leaders, community groups, and local leadership of NTD and community health programs. Stakeholders with the most control over the flow of information and resources across the networks (i.e., highest betweenness centrality scores) included national- and district-level MOH program personnel and governance leadership (e.g., district health officers or mayors within the trial implementation area), as well as DeWorm3 site staff. Across sites, we observed low density scores (¾0.06) for community-wide MDA networks over a three-year time period (Figure 2). Centralization scores also remained relatively moderate or low across sites over time, ranging from 0.23 to 0.09 for all networks (Figure 2).
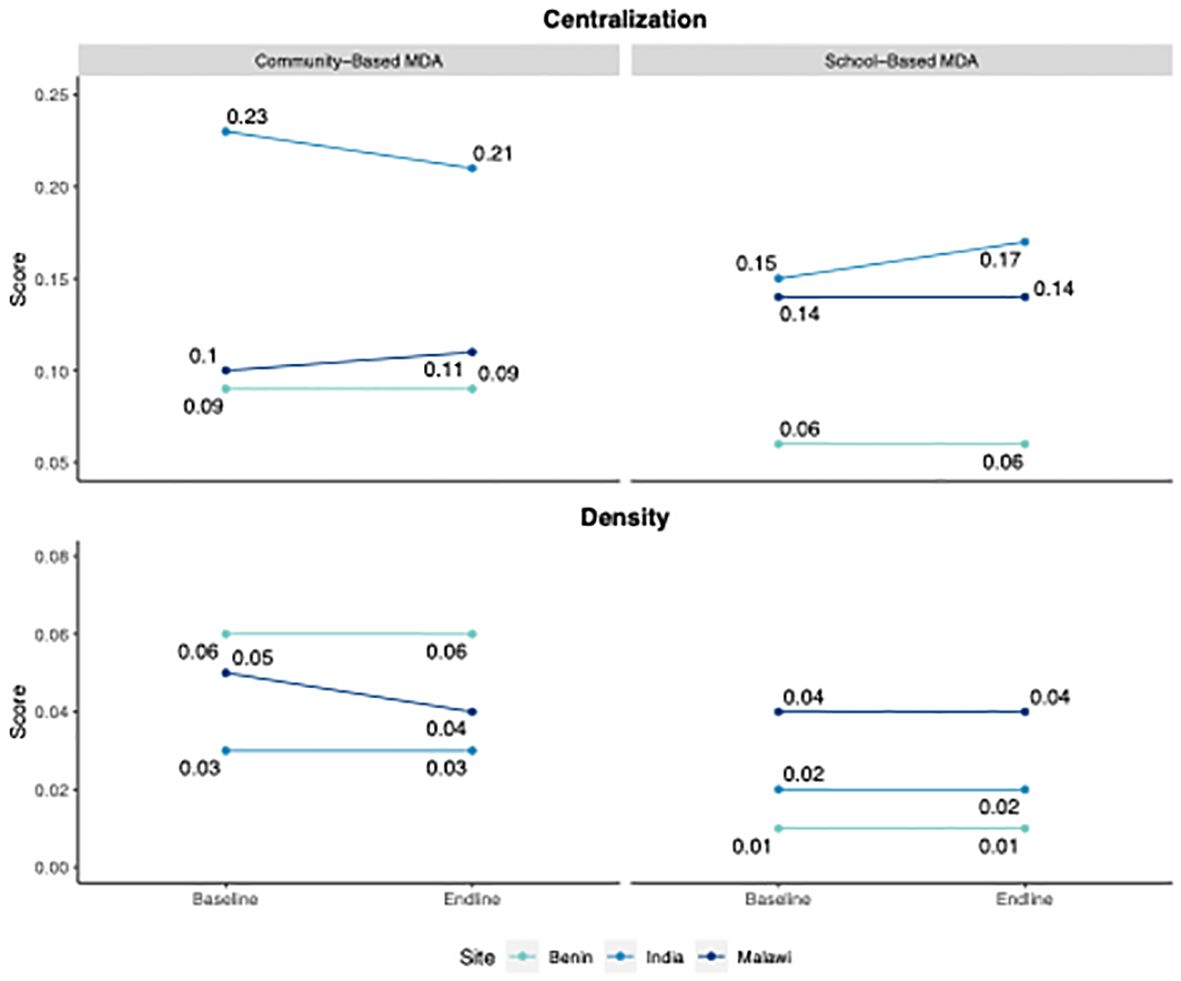
Figure 2. Community-wide and school-based MDA network centralization and density scores for Deworm3 sites, baseline vs. endline.
Community-wide MDA network sociograms
We visualized six baseline (Figures 3A–C) and endline (Figures 4A–C) community-wide MDA sociograms. These sociograms visually display three different network structures for each site. In Benin, we observe “random” shaped sociograms, with a mixture of relationship types, indicated by the various arrow colors between stakeholders. India’s sociograms follow a divergent “y-shaped” structure, representative of the separation of the two geographically-distinct trial implementation areas, with a majority of supervisory relationships (i.e., black arrows). The Malawi sociograms appear to have a linear structure with a variety of relationship types. Although we did not observe any structural holes in the baseline networks, two holes (shaded in gray) appeared in the India endline sociogram due to the removal of one national-level stakeholder, which demonstrate the impact of stakeholder loss on overall network connectivity (Figure 4B).
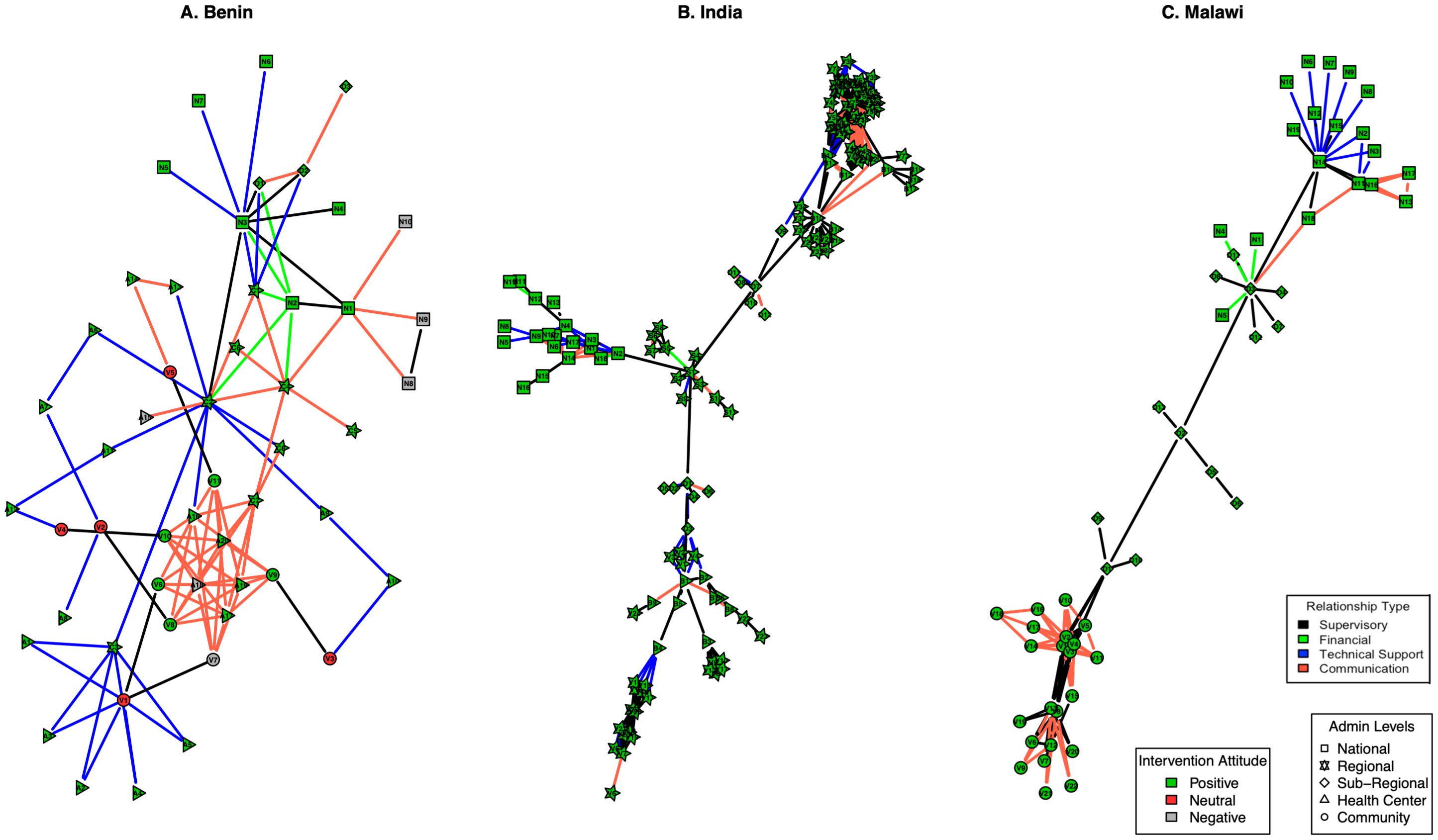
Figure 3. Baseline community-wide MDA sociograms, by site [(A) = Benin, (B) = India, (C) = Malawi]. Relationship type (edges connecting nodes): Black = supervisory, green = Financial, Blue = technical support, Red = communication. Intervention attitude (color of node): Green = positive, Red = negative, Gray = neutral. Regional levels per site: Benin = department, India = state, Malawi = district. Sub-regional levels per site: Benin = commune, India & Malawi = district. Each node is identified by a unique nodal ID necessary for analysis and visualization.
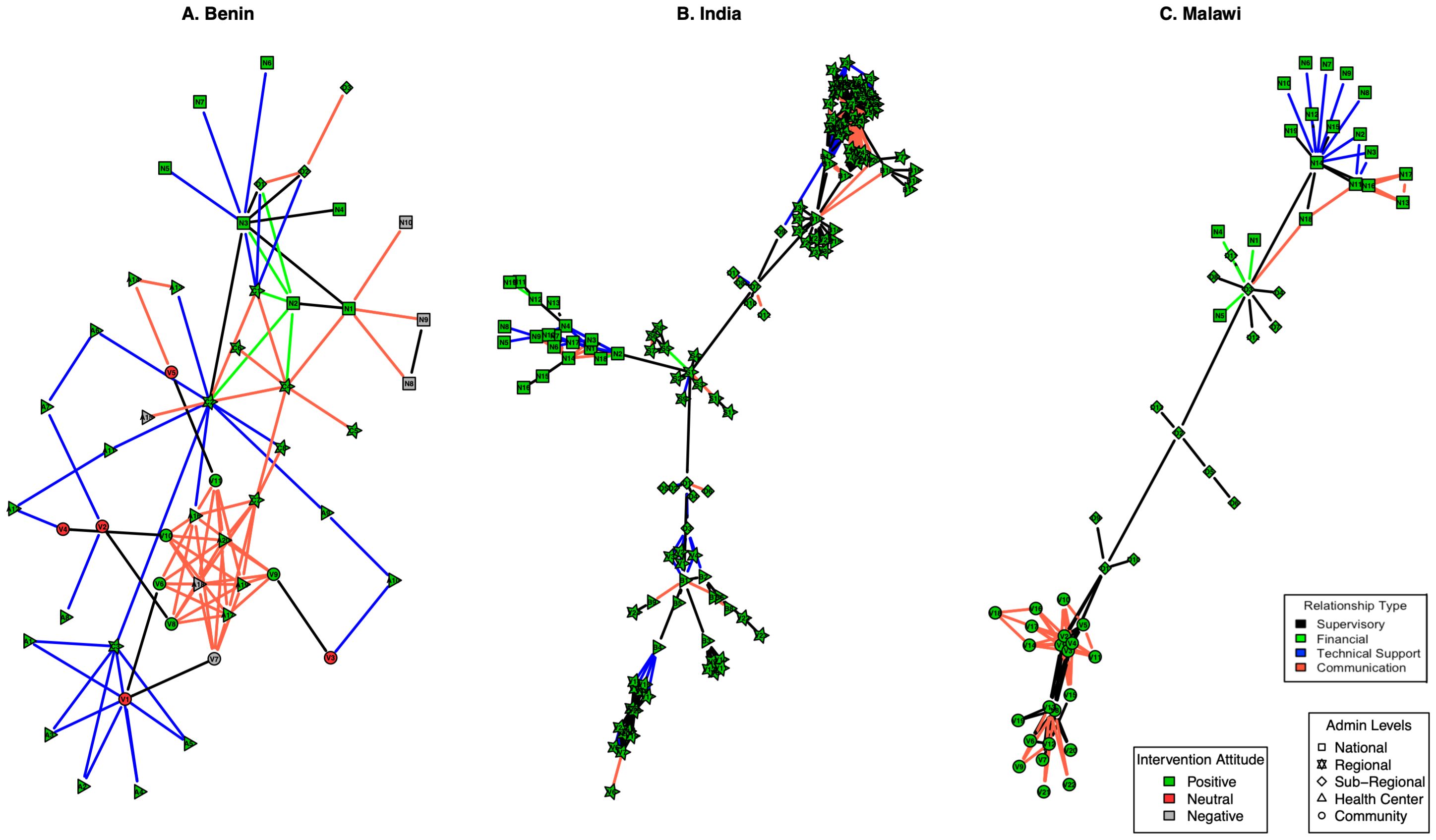
Figure 4. Endline community-wide MDA sociograms, by site [(A) = Benin, (B) =India, (C) = Malawi]. Relationship type (edges connecting nodes): Black = supervisory, green = Financial, Blue = technical support, Red = communication. Intervention attitude (color of node): Green = positive, Red = negative, Gray = neutral. Regional levels per site: Benin = department, India = state, Malawi = district. Sub-regional levels per site: Benin = commune, India & Malawi = district. Each node is identified by a unique nodal ID necessary for analysis and visualization.
Stakeholder networks for school-based MDA
Network composition
Most stakeholders in the school-based MDA networks were also included in the community-wide MDA networks. Additional stakeholders unique to the school-MDA networks included: national & regional level stakeholders such as MOE and MOH (except for India where MOH is common to both school and community-wide MDA) personnel who define policies and provide program management for school-based health programs; sub-regional & health center level stakeholders, such as school deworming program managers who design and oversee program planning & implementation; and community-level stakeholders, including school principals who oversee deworming days, health workers that monitor for adverse events, parent and teacher associations who support mobilization of students and non-enrolled children, teachers and CHWs who administer deworming to students at schools and non-enrolled children at child health centers (i.e., anganwadi centers in India).
Across sites, the school-based MDA networks were much larger than the community-wide MDA networks, with a total of 360 and 364 unique stakeholders identified at baseline and endline, respectively (Figure 5). Similar to the community-MDA networks, most stakeholders in the school-based MDA networks were at the health center and community levels. The networks remained fairly stable over the three-year period, as we observed a minimal difference in the number of stakeholders and relationships over time. Comparing baseline and endline, India had the largest school-based network at both time points (163 and 162, respectively), followed by Benin (139 at both time points), and Malawi (58 and 63, respectively). In Benin and Malawi, the number of relationships also remained relatively stable in the networks from baseline to endline (165 vs. 166 relationships in Benin and 128 vs. 132 in Malawi), as compared to India, which had a decrease of 42 relationships (513 vs. 471).
Attitude towards school- based MDA intervention
Nearly all stakeholders (93%, 98%, and 88% in Benin, India, and Malawi, respectively) had positive attitudes towards school-based MDA at baseline, though these proportions slightly reduced or remained the same at endline (93%, 99%, and 75%, respectively) across the sites.
Influence on school-based MDA intervention
A majority of stakeholders were identified as having high influence over implementation of school-based MDA at baseline in Benin, India (92% and 72%, respectively), as compared to Malawi (21%).
Across the three sites, the most connected stakeholders (i.e., with the highest degree centrality scores) were school teachers and anganwadi workers, as well as health center workers and village leaders (e.g., village heads and religious leaders). Stakeholders with the most control over the flow of information and resources across the networks (i.e., highest betweenness centrality scores) included national- and regional-level STH and school deworming program leadership, district-level educational health program personnel, health facility workers, and school principals, and village leaders. Density scores for school-based MDA networks (Figure 2) were low across sites over time (all ¾0.04). Centralization scores also remained low across sites over time, ranging from 0.15-0.06 for school-based MDA networks (Figure 2).
School-based MDA network sociograms
Sociograms for baseline and endline school-based MDA are presented in Figures 6A–C, 7A–C. Visually, each school-based MDA sociogram is similar to the relevant community-wide MDA sociogram: we observed a random structure in Benin with a mixture of relationship types, a “y-shaped” structure in India with a majority of supervisory relationships, and a linear structure in Malawi with a mixture of relationship types. The Benin baseline sociogram (Figure 6A) exhibits two structural holes (shaded in gray), both including health center- and community- level stakeholders. Although there was no change in the number of stakeholders from baseline to endline in Benin, one of the holes in the endline sociogram disappeared due to the addition of new relationships at endline at the health center level. The Malawi baseline (Figure 6C) and endline (Figure 7C) sociograms included two structural holes including stakehoders at the national and village levels that persisted in the endline sociogram.
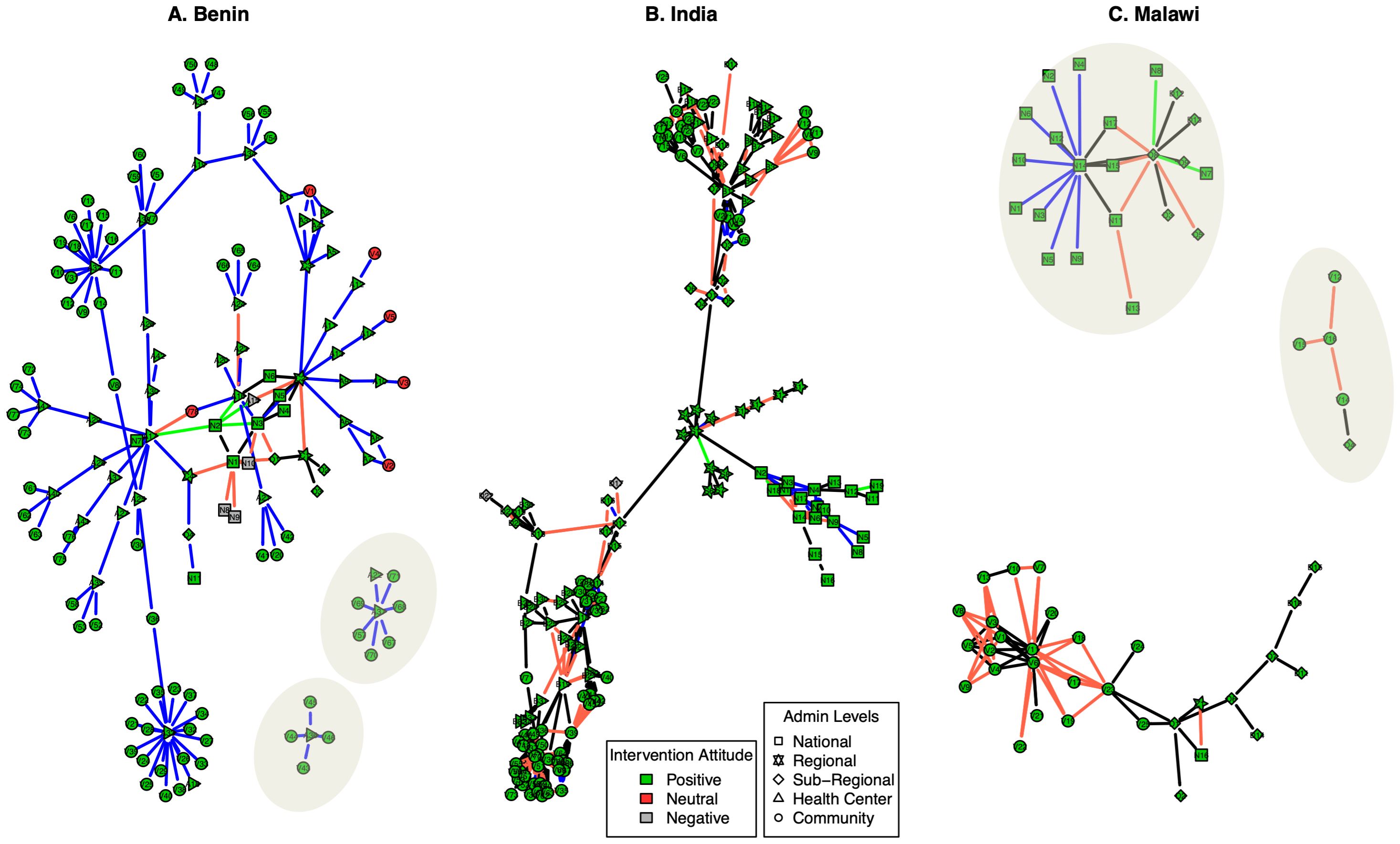
Figure 6. Baseline school-based MDA sociograms, by site [(A) = Benin, (B) = India, (C) = Malawi]. Relationship type (edges connecting nodes): Black = supervisory, green = Financial, Blue = technical support, Red = communication. Intervention attitude (color of node): Green = positive, Red = negative, Gray = neutral. Regional levels per site: Benin = department, India = state, Malawi = district. Sub-regional levels per site: Benin = commune, India & Malawi = district. Each node is identified by a unique nodal ID necessary for analysis and visualization.
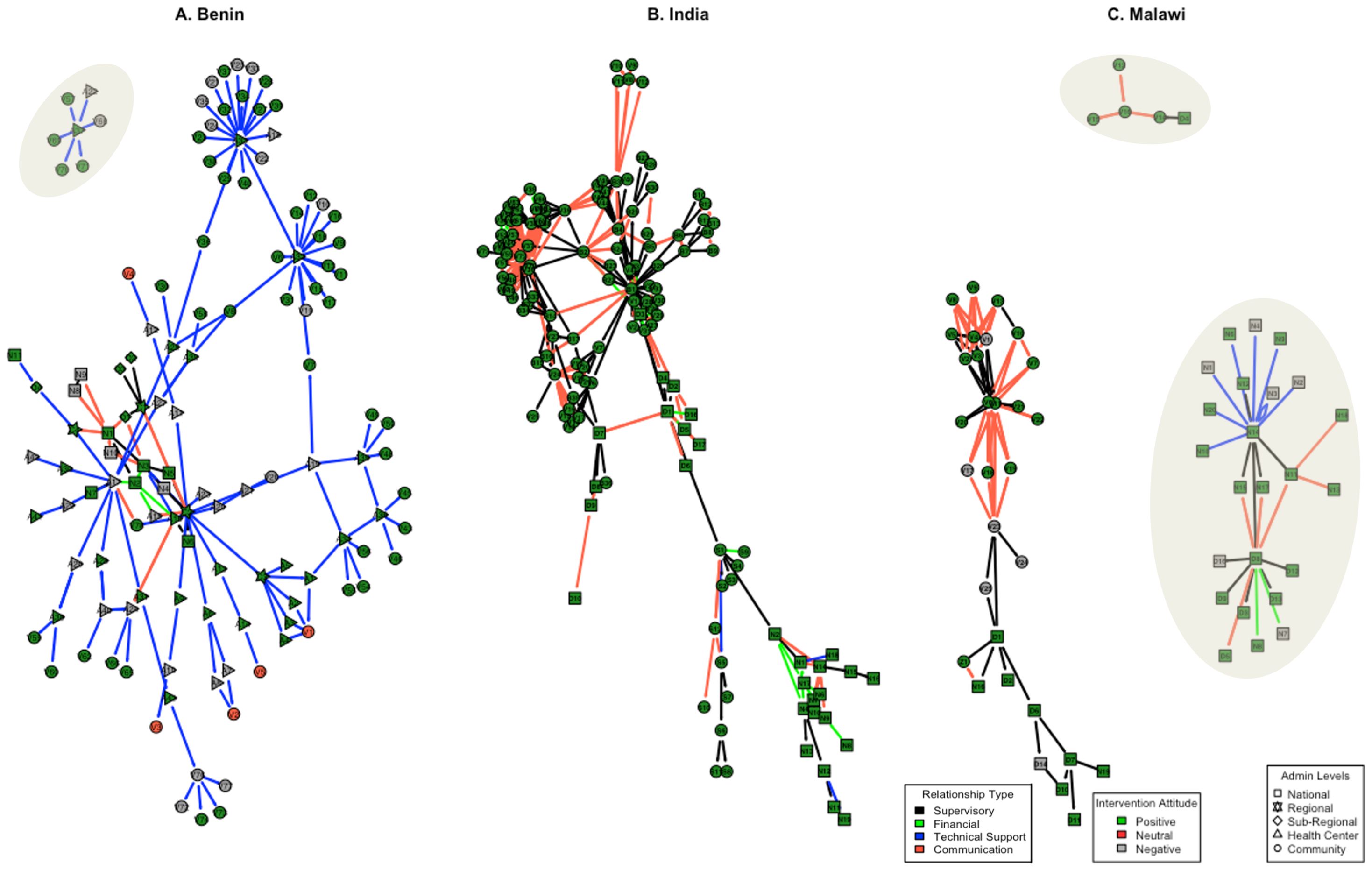
Figure 7. Endline school-based MDA sociograms, by site [(A) = Benin, (B) = India, (C) = Malawi]. Relationship type (edges connecting nodes): Black = supervisory, green = Financial, Blue = technical support, Red = communication. Intervention attitude (color of node): Green = positive, Red = negative, Gray = neutral. Regional levels per site: Benin = department, India = state, Malawi = district. Sub-regional levels per site: Benin = commune, India & Malawi = district. Each node is identified by a unique nodal ID necessary for analysis and visualization.
Discussion
An understanding of stakeholder networks is useful for optimizing the launch and scale-up of large, complex public health programs such as MDA. In this study, we applied SNA methods to comprehensively describe and evaluate the dynamics of an expansive network of stakeholders involved in the delivery of school-based and community-wide MDA programs in DeWorm3 trial sites in Benin, India, and Malawi. Our findings offer valuable insights for policymakers and implementers in STH-endemic countries considering a potential policy transition from school-based to community-wide MDA. Identifying and understanding individual influencers and resource brokers as well as network strengths and weaknesses can enhance the planning, implementation, and monitoring of MDA programs by pinpointing critical relationships and dynamics that may impede or facilitate effective program delivery. These insights can further support policymakers and implementers to develop robust operational guidelines and strategies that enhance multisectoral communication, optimize resource allocation, strengthen capacity building efforts, and enhance community acceptability and uptake. Such efforts are crucial for ensuring the success and sustainability of MDA programs in achieving high coverage, and ultimately, STH transmission interruption. We further detail how understanding key network characteristics, including network size, connectivity, and fragility, as well as stakeholder characteristics, including intervention attitude and influence, may be helpful in supporting important decision-making processes for key NTD policymakers and implementers.
Network size
Across sites, the largest number of stakeholders were at the health center/block and community levels, which reflect the nature of MDA programs where a significant number of implementers are necessary for drug administration. Additionally, across sites, school-based MDA networks were larger than community-wide MDA networks. This may be because school-based deworming programs are well-established in each country, which makes it easier to identify individuals involved in the delivery platform. The cross-ministerial collaboration (i.e., MOH and MOE) necessary for planning and delivering school-based MDA naturally requires more stakeholders than an intervention primarily implemented by one predominant Ministry (i.e., MOH), such as community-wide MDA. Thus, if countries choose a hybrid implementation approach that requires both delivery platforms, they should consider how this collaboration, while essential, can become politically and logistically complicated. This finding aligns with DeWorm3 qualitative research, where policy stakeholders across sites identified the overburdening of existing health workers and weak intragovernmental coordination as key barriers to the successful launch of community-wide MDA for STH (28). The complexities of this approach further validate the importance of identifying and galvanizing network connectors prior to intervention launch.
Although we observed a minimal change in the total number of stakeholders over time, the addition or removal of even one highly influential stakeholder could have significant impacts on overall network structure. The aforementioned DeWorm3 qualitative study with policy stakeholders highlighted that a key facilitator for launching community-wide MDA for STH was leveraging existing infrastructure from other NTD programs, which requires a robust network with effective communication across administrative levels (28). Thus, these observations are useful for building connections to bridge any existing structural holes. Identifying these critical actors is essential for developing strategic engagement strategies that can help retain these actors in the network, as they can help maintain the institutional memory and efficient disbursement of key resources that supports high-performing implementation (29).
Network connectivity & fragility
Stakeholder-level analyses suggest that individuals with the highest connectivity (i.e., high degree centrality) were not the same as those with greatest control over the flow of information and resources (i.e., high betweenness centrality); more highly-connected stakeholders were clustered at community and health center levels while those who controlled resource flow were clustered at national and regional levels. These patterns reflect the ‘pyramid-shaped’ structure of stakeholders involved in MDA implementation; the larger number of implementers at lower levels provide more opportunity for connection due to the integrated nature of MDA delivery that requires close collaboration and communication, while the less-populated upper levels, including policymakers and program managers, serve as gatekeepers of information and resource flow. Interestingly, several of the most connected stakeholders were included in both the school-based MDA and community-wide MDA sociograms (e.g., district-level MDA program managers, health facility workers, and village leaders), indicating potential opportunities for leveraging human resource capacity of single individuals and their expertise to support the transition from school-based to community-wide deworming programs. Understanding which stakeholders play critical roles in diffusing innovations in a network is critical for developing engagement strategies to complement the launch of future policy updates (30) or disseminating key sensitization messages to help maximize coverage and uptake rates at scale (14). A mixed-methods DeWorm3 implementation science study identified that strategic engagement with local leaders and health workers was central to building trust in community-wide MDA, a key determinant in achieving high coverage (31). Inversely, insufficient engagement of these actors could likely result in a more-fragmented network, negatively impacting the cohesion of stakeholders upholding policy and implementation landscape (32).
For both platforms, we consistently observed low density and centralization scores in each of the intervention networks. Low density is indicative of a network with minimal overall connectivity, despite the presence of a few individuals with particularly high connectivity. Visual maps demonstrate how connections between administrative levels are dependent on one or a few stakeholders (e.g. low centralization), which could result in inefficient diffusion of information or resources and implementation bottlenecks (33). Low network centralization indicates a more balanced distribution of information or resources without dominance by one or few highly-connected individuals, which is particularly advantageous for intervention scale-up (13, 33, 34). Additionally, low centralization may also provide an opportunity for network actors with high degree of betweenness centrality who serve as key dissemination agents to expand their influence and facilitate network strengthening by integrating new relationships or providing opportunities for knowledge exchange (29, 32). For example, actors with high degree centrality could use their strong connectivity to bring together personnel working across various ministries or organizations to enhance collaboration, while those with high betweenness centrality could help promote complementary cross-ministerial strategies that leverage institutional knowledge and expertise. These network characteristics could be useful for assessing health system readiness or capacity for effective implementation at scale (35, 36).
Lastly, we observed structural holes in several of the baseline and endline networks, which are readily explained by the three-year difference in study timelines. Although we did not investigate the relationship between the change in perceived attitude towards the intervention and the existence of structural holes, structural hole theory suggests that bridging these holes can bring alternative perspectives into a group with homogenous opinions (13, 14). The mapping conducted at different time points revealed connection gaps across administrative levels. This insight can support decision makers to identify critical positions that need to be filled to promote effective program implementation by further improving the flow of information or resources across sub-networks (13). Ultimately, understanding structural holes can offer practical programmatic support. For example, programs aiming to strengthen intervention delivery can introduce “connection brokers” that can be strategically engaged to bridge communication or resource flow gaps and enhance overall network cohesion (37–39).
Intervention influence and attitude
Across all administrative levels and all sites, a majority of stakeholders held positive attitudes towards both MDA platforms at both time points, with a slight decrease at study endline. While we did not qualitatively assess drivers of the decrease in positive attitude from baseline, it is possible that over time stakeholders recognized the additional workload necessary for expanding MDA from schools to the entire community (9, 40). These perspectives have been investigated in baseline qualitative research with policy makers (9) and will be further assessed in endline qualitative research with implementers. Given that all sites have either ongoing or prior MDA programs for other NTDs, it is possible that the ubiquity of positive attitudes towards both MDA platforms may be an indication of stakeholder confidence in implementation capacity based on experience with other NTD programs. This information is useful for assessing the policy transition climate, as stakeholders may be more accepting of a change in standard-of-care STH programming (i.e., transition to community-wide MDA) when the change is aligned with other familiar community delivery models. For instance, each DeWorm3 study site has previously operated community-wide MDA programs for other NTDs, such as lymphatic filariasis, which administered the same deworming medication (albendazole) used in STH MDA programs. Across sites, other DeWorm3 implementation science research found that community members’ familiarity with the treatment drug and previous positive experiences with other community health programs positively influenced their perception towards and willingness to participate in community-wide MDA (31).
Interestingly, there was extensive heterogeneity across sites in the proportion of stakeholders influencing successful MDA implementation and scale-up across administrative levels for both platforms. In all sites, a majority of stakeholders with high influence were at the local administrative units. Recent implementation readiness findings from these settings indicate that there is heterogeneity in readiness and capacity to deliver community-wide MDA, with stakeholders at the community levels who are the primary implementation MDA workforce exhibiting particularly low readiness regarding human resource availability (41). Thus, the high influence of these local stakeholders suggests that it is important to prioritize their readiness to transition from school-based to community-wide MDA prior to intervention launch. During DeWorm3 qualitative research, policy makers identified inadequate supervision of local implementers, such as health workers and community drug distributors, as a key barrier to effectively launching community-wide MDA (28). Interestingly, for both interventions, significantly fewer proportions of stakeholders were identified as having high influence over implementation in Malawi, as compared to Benin and India. Despite the standardization in training, it is plausible that the inter-site heterogeneity may be an indication of differential interpretation of the influence indicator; rather than a unique distinction in Malawi regarding where influence lies.
This study had several notable strengths. Data were collected systematically across multiple geographies using standardized methods. As a result, these findings provide STH policymakers and program implementers an extensive assessment of the current implementation environment. However, this study also has several limitations. First, this study utilized a sociocentric approach to data collection that aimed to identify a complete network relying on group consensus rather than self-report from a specific individual who identifies others in their network (i.e., an egocentric approach). While an egocentric approach may have more accurately captured stakeholder relationships and attitudes, this approach had low feasibility due to the large size of the networks. Thus, it is possible that some stakeholders are missing from the networks. Suggested strategies for strengthening the data collection approach include hosting several rounds of workshops with different key informants, extending the timeline for data collection, or embedding ongoing stakeholder identification within other research activities, such as qualitative data collection. Future research should assess more objective approaches to collecting data regarding individual attitudes towards an intervention and develop robust methods for data validation. Additionally, although we were unable to empirically investigate the relationship between network dynamics and intervention delivery within the scope of this network analysis, we highlighted associations between our findings with other DeWorm3 implementation research uncovering key barriers and facilitators for successful delivery of community-wide MDA for STH within study sites. Future research could incorporate mixed-methods social network analysis (42–44) to provide deeper insights into how network dynamics impede or foster successful intervention delivery. Despite these limitations, our findings demonstrate the utility of studying intervention networks to prepare for potential scale-up of a new policy.
Overall, this study sends important signals to key decision makers, namely around the importance of strategic intervention landscape assessments that can help forecast necessary investments in key influential stakeholders who serve as structural links (e.g., the most connected or those who hold positive attitudes) to optimize intervention delivery, uptake, and subsequent impact.
Conclusion
Our findings provide useful insight to policymakers and implementers in STH-endemic countries considering a transition from school-based to community-wide MDA. Specifically, stakeholder maps and SNA approaches provide the opportunity to identify and quantify the influence of key stakeholders across various administrative levels responsible for MDA planning and delivery, including those who serve as key connectors and brokers of resources or those who can serve as champions for launching and scaling community-wide MDA for STH. At the network level, these approaches identified key strengths (e.g., the networks were not overly dependent on any single individual) and relevant weaknesses (e.g., identifying structural holes where there is limited communication and resource flow across key entities in the network) that are critical for program planning and monitoring. Our findings also demonstrate strategic opportunities to purposefully activate MDA stakeholders to champion different roles based on their position or function within the intervention network. Cultivating these strengths could help the entire network optimally operate in order to drive effective implementation and sustainable impact, especially for countries aiming to evolve from STH control to elimination strategies by transitioning from implementing school-based MDA to community-wide MDA.
Data availability statement
The raw data supporting the conclusions of this article will be made available by the authors, without undue reservation.
Ethics statement
The IS research component of the DeWorm3 Project has been reviewed and approved by the Institut de Recherche Clinique au Bénin (IRCB) through the National Ethics Committee for Health Research (002-2017/CNERS-MS) from the Ministry of Health in Benin, The London School of Hygiene and Tropical Medicine (12013), The College of Medicine Research Ethics Committee (P.04/17/2161) in Malawi, Christian Medical College in Vellore, and KEM Hospital Research Centre Ethics Committee (1718 and 1719). The study was also approved by The Human Subjects Division at the University of Washington (STUDY00000180).
Author contributions
M-CG-C: Project administration, Validation, Conceptualization, Formal analysis, Methodology, Software, Visualization, Writing – original draft, Writing – review & editing. KA: Conceptualization, Data curation, Investigation, Methodology, Supervision, Validation, Writing – review & editing. EA: Conceptualization, Data curation, Investigation, Methodology, Supervision, Validation, Writing – review & editing. CC: Data curation, Investigation, Methodology, Supervision, Validation, Writing – review & editing. MS: Data curation, Methodology, Validation, Writing – review & editing. AT: Data curation, Methodology, Validation, Writing – review & editing. PH: Data curation, Methodology, Writing – review & editing. CT: Data curation, Methodology, Validation, Writing – review & editing. FC: Data curation, Methodology, Validation, Writing – review & editing. PN: Data curation, Methodology, Writing – review & editing. JS: Data curation, Methodology, Project administration, Supervision, Writing – review & editing. JJ: Data curation, Methodology, Writing – review & editing. KK: Validation, Writing – review & editing, Conceptualization, Data curation, Investigation, Methodology, Project administration, Supervision. MI: Conceptualization, Data curation, Investigation, Methodology, Project administration, Supervision, Validation, Writing – review & editing. SA: Conceptualization, Data curation, Investigation, Methodology, Project administration, Supervision, Validation, Writing – review & editing. BW: Conceptualization, Methodology, Supervision, Validation, Writing – review & editing. JW: Conceptualization, Funding acquisition, Methodology, Supervision, Validation, Writing – review & editing. AM: Conceptualization, Data curation, Funding acquisition, Investigation, Methodology, Project administration, Supervision, Validation, Writing – original draft, Writing – review & editing.
Funding
The author(s) declare financial support was received for the research, authorship, and/or publication of this article. The DeWorm3 Project was funded by a grant from the Bill & Melinda Gates Foundation (OPP1129535, PI: JW). The funders had no role in study design, data collection and analysis, decision to publish, or preparation of the manuscript.
Acknowledgments
The authors wish to thank all of the study participants, communities and community leaders, national NTD program staff, and local, regional, and national partners (Ministry of Health and Family Welfare, Delhi and the Directorate of Public Health, Chennai in India and Programme National de lutte contre les Maladies Transmissibles du Ministère de la Santé du Bénin) who have participated in or supported the implementation of the DeWorm3 study. We also would like to acknowledge the work of all members of the DeWorm3 study teams and affiliated institutions.
Conflict of interest
The authors declare that the research was conducted in the absence of any commercial or financial relationships that could be construed as a potential conflict of interest.
Publisher’s note
All claims expressed in this article are solely those of the authors and do not necessarily represent those of their affiliated organizations, or those of the publisher, the editors and the reviewers. Any product that may be evaluated in this article, or claim that may be made by its manufacturer, is not guaranteed or endorsed by the publisher.
References
1. Hotez PJ, Bundy DAP, Beegle K, Brooker S, Drake L, de Silva N, et al. Helminth infections: soil-transmitted helminth infections and schistosomiasis. Disease control priorities in developing countries. 2nd edition. (2006), 467–82.
2. World Health Organization. Preventive chemotherapy to control soil-transmitted helminth infections in at-risk population groups. (2017).
3. Brooker S, Kabatereine NB, Fleming F, Devlin N. Cost and cost-effectiveness of nationwide school-based helminth control in Uganda: intra-country variation and effects of scaling-up. Health Policy Plan. (2008) 23:24–35. doi: 10.1093/heapol/czm041
4. Leslie J, Garba A, Oliva EB, Barkire A, Tinni AA, Djibo A, et al. Schistosomiais and soil-transmitted helminth control in Niger: cost effectiveness of school based and community distributed mass drug administration. PloS Negl Trop Dis. (2011) 5:e1326. doi: 10.1371/journal.pntd.0001326
5. Brooker SJ, Mwandawiro CS, Halliday KE, Njenga SM, McHaro C, Gichuki PM, et al. Interrupting transmission of soiltransmitted helminths: A study protocol for cluster randomised trials evaluating alternative treatment strategies and delivery systems in Kenya. BMJ Open. (2015) 5:1–12. doi: 10.1136/bmjopen-2015-008950
6. delos Trinos JP, Ng-Nguyen D, Coffeng LE, Dyer CE, Clarke N, Traub R, et al. Cost and cost-effectiveness analysis of mass drug administration compared to school-based targeted preventive chemotherapy for hookworm control in Dak Lak province, Vietnam. Lancet Regional Health–Western Pacific. (2023) 41. doi: 10.1016/j.lanwpc.2023.100913
7. World Health Organization. Schistosomiasis and soil-transmitted helminthiases: number of people treated in 2019. Weekly Epidemiological Rec. (2020) 95.
8. Truscott JE, Hollingsworth TD, Brooker SJ, Anderson RM. Can chemotherapy alone eliminate the transmission of soil transmitted helminths? Parasit Vectors. (2014) 7:1–8. doi: 10.1186/1756-3305-7-266
9. Anderson RM, Truscott JE, Pullan RL, Brooker SJ, Hollingsworth TD. How effective is school-based deworming for the community-wide control of soil-transmitted helminths? PloS Negl Trop Dis. (2013) 7:e2027. doi: 10.1371/journal.pntd.0002027
10. Anderson R, Truscott J, Hollingsworth TD. The coverage and frequency of mass drug administration required to eliminate persistent transmission of soil-transmitted helminths. Philos Trans R Soc B Biol Sci. (2014) 369(1645):20130435. doi: 10.1098/rstb.2013.0435
11. Brooker SJ, Nikolay B, Balabanova D, Pullan RL. Global feasibility assessment of interrupting the transmission of soil-transmitted helminths: A statistical modelling study. Lancet Infect Dis. (2015) 15:941–50. doi: 10.1016/S1473-3099(15)70042-3
12. Perkins J, Subramanian S, Christakis NA. Social networks and health: A systematic review of sociocentric network studies in low- and middle-income countries. Soc Sci Med. (2017) 125:60–78. doi: 10.1016/j.socscimed.2014.08.019
14. Valente TW, Palinkas LA, Czaja S, Chu KH, Brown CH. Social network analysis for program implementation. PloS One. (2015) 10(6):e0131712. doi: 10.1371/journal.pone.0131712
15. Ásbjörnsdóttir KH, Ajjampur SSR, Anderson RM, Bailey R, Gardiner I, Halliday KE, et al. Assessing the feasibility of interrupting the transmission of soil-transmitted helminths through mass drug administration: The DeWorm3 cluster randomized trial protocol. PloS Negl Trop Dis. (2018) 12:1–16. doi: 10.1371/journal.pntd.0006166
16. Means AR, Ajjampur SSR, Bailey R, Galactionova K, Gwayi-Chore MC, Halliday K, et al. Evaluating the sustainability, scalability, and replicability of an STH transmission interruption intervention: The DeWorm3 implementation science protocol. PloS Negl Trop Dis. (2018) 12:1–13. doi: 10.1371/journal.pntd.0005988
17. Harvey N, Holmes CA. Nominal group technique: an effective method for obtaining group consensus. Int J Nurs Pract. (2012) 18:188–94. doi: 10.1111/j.1440-172X.2012.02017.x
18. Yang S, Keller FB, Zheng L. Social Network Analysis: Methods and Examples. Thousand Oaks, California: SAGE Publications (2017).
19. Palinkas LA, Holloway IW, Rice E, Fuentes D, Wu Q, Chamberlain P. Social networks and implementation of evidence-based practices in public youth-serving systems: a mixed-methods study. Implementation Science. (2011) 6:1–11. doi: 10.1186/1748-5908-6-113
20. Valente TW, Fujimoto K, Palmer P, Tanjasiri SP. A network assessment of community-based participatory research: linking communities and universities to reduce cancer disparities. Am J Public Health. (2010) 100:1319–25. doi: 10.2105/AJPH.2009.171116
21. Blanchet K, James P. How to do (or not to do) … a social network analysis in health systems research. Health Policy Plan. (2012) 27(5):438–46. doi: 10.1093/heapol/czr055
22. Chami GF, Ahnert SE, Kabatereine NB, Tukahebwa EM. Social network fragmentation and community health. Proc Natl Acad Sci. (2017) 114(36):E7425–31. doi: 10.1073/pnas.1700166114
23. Chami GF, Kontoleon AA, Bulte E, Fenwick A, Kabatereine NB, Tukahebwa EM, et al. Diffusion of treatment in social networks and mass drug administration. Nat Commun. (2017) 8(1):1929. doi: 10.1038/s41467-017-01499-z
24. Chami GF, Molyneux DH, Kontoleon AA, Dunne DW. Exploring network theory for mass drug administration. Trends Parasitol. (2013) 29:370–9. doi: 10.1016/j.pt.2013.04.005
25. Chami GF, Kontoleon AA, Bulte E, Fenwick A, Kabatereine NB, Tukahebwa EM, et al. Social Science & Medicine Community-directed mass drug administration is undermined by status seeking in friendship networks and inadequate trust in health advice networks. Soc Sci Med. (2017) 183:37–47. doi: 10.1016/j.socscimed.2017.04.009
26. Csardi G, Nepusz T. The igraph software package for complex network research. InterJournal: Vol. Complex Systems. (2006). p. 1695.
27. Csardi G, Nepusz T. The igraph software for complex network research. InterJournal Complex Syst. (2006) 1695.
28. Roll A, Saxena M, Orlan E, Titus A, Juvekar SK, Gwayi-Chore MC, et al. Policy stakeholder perspectives on barriers and facilitators to launching a community-wide mass drug administration program for soil-transmitted helminths. Glob Health Res Policy. (2022) 7:1–14. doi: 10.1186/s41256-022-00281-z
29. Hagel C, Weidemann F, Gauch S, Edwards S, Tinnemann P. Analysing published global Ebola virus disease research using social network analysis. PloS Negl Trop Dis. (2017) 11:e0005747. doi: 10.1371/journal.pntd.0005747
30. Lomas J, Enkin M, Anderson GM, Hannah WJ, Vayda E, Singer J. Opinion leaders vs audit and feedback to implement practice guidelines: delivery after previous cesarean section. Jama. (1991) 265(17):2202–7.
31. Saxena M, Roll A, Walson JL, Pearman E, Legge H, Nindi P, et al. Our desire is to make this village intestinal worm free”: Identifying determinants of high coverage of community-wide mass drug administration for soil transmitted helminths in Benin, India, and Malawi. PloS Negl Trop Dis. (2024) 18:e0011819. doi: 10.1371/journal.pntd.0011819
32. Vanderelst D. Social Network Analysis as a tool for research policy. PloS Negl Trop Dis. (2015) 9:e0004266. doi: 10.1371/journal.pntd.0004266
33. Himelboim I, Smith MA, Rainie L, Shneiderman B, Espina C. Classifying Twitter topic-networks using social network analysis. Soc Media Soc. (2017) 3:2056305117691545. doi: 10.1177/2056305117691545
34. Blanchet K, James P. How to do (or not to do) … a social network analysis in health systems research. Health Policy Plan. (2012) 27:438–46. doi: 10.1093/heapol/czr055
35. Feinberg ME, Riggs NR, Greenberg MT. Social networks and community prevention coalitions. J Primary Prev. (2005) 26:279–98. doi: 10.1007/s10935-005-5390-4
36. Vardaman JM, Amis JM, Dyson BP, Wright PM, Van de Graaff Randolph R. Interpreting change as controllable: The role of network centrality and self-efficacy. Hum Relations. (2012) 65:835–59. doi: 10.1177/0018726712441642
37. Lei S, Wang C, Zhang T, Liu X. Robustness of open source community multi-project knowledge collaboration network based on structural hole theory. PloS One. (2024) 19:e0292444. doi: 10.1371/journal.pone.0292444
38. Burt RS, Kilduff M, Tasselli S. Social network analysis: Foundations and frontiers on advantage. Annu Rev Psychol. (2013) 64:527–47. doi: 10.1146/annurev-psych-113011-143828
39. Long JC, Cunningham FC, Braithwaite J. Bridges, brokers and boundary spanners in collaborative networks: a systematic review. BMC Health Serv Res. (2013) 13:1–13. doi: 10.1186/1472-6963-13-158
40. Aruldas K, Dawson K, Saxena M, Titus A, Johnson J, Gwayi-Chore MC, et al. Evaluation of opportunities to implement community-wide mass drug administration for interrupting transmission of soil-transmitted helminths infections in India. PloS Negl Trop Dis. (2023) 17:e0011176. doi: 10.1371/journal.pntd.0011176
41. Means AR, Orlan E, Gwayi-Chore MC, Titus A, Kaliappan SP, Togbevi CI, et al. Structural readiness to implement community-wide mass drug administration programs for soil-transmitted helminth elimination: results from a three-country hybrid study. Implement Sci Commun. (2021) 2:1–30. doi: 10.1186/s43058-021-00164-3
42. Kolleck N. Social network analysis in innovation research: using a mixed methods approach to analyze social innovations. Eur J Futures Res. (2013) 1:1–9. doi: 10.1007/s40309-013-0025-2
43. Hollstein B. Mixed methods social networks research: an introduction. Mixed methods social networks research: Design and applications, Vol. 1. New York, NY, USA. (2014). pp. 3–34.
Keywords: soil-transmitted helminths, mass drug administration, network analysis, implementation science, scale-up
Citation: Gwayi-Chore M-C, Aruldas K, Avokpaho E, Chirambo CM, Saxena M, Titus A, Houngbégnon P, Togbevi CI, Chabi F, Nindi P, Simwanza J, Johnson J, Kalua K, Ibikounlé M, Ajjampur SSR, Weiner BJ, Walson JL and Means AR (2024) Key influencers of mass drug administration implementation and scale-up: a social network analysis of soil-transmitted helminth intervention platforms in Benin, India, and Malawi. Front. Trop. Dis 5:1346828. doi: 10.3389/fitd.2024.1346828
Received: 30 November 2023; Accepted: 09 September 2024;
Published: 15 November 2024.
Edited by:
Natalia Hounsome, Brighton and Sussex Medical School, United KingdomReviewed by:
Poppy Lamberton, University of Glasgow, United KingdomCollins Okoyo, Kenya Medical Research Institute (KEMRI), Kenya
Copyright © 2024 Gwayi-Chore, Aruldas, Avokpaho, Chirambo, Saxena, Titus, Houngbégnon, Togbevi, Chabi, Nindi, Simwanza, Johnson, Kalua, Ibikounlé, Ajjampur, Weiner, Walson and Means. This is an open-access article distributed under the terms of the Creative Commons Attribution License (CC BY). The use, distribution or reproduction in other forums is permitted, provided the original author(s) and the copyright owner(s) are credited and that the original publication in this journal is cited, in accordance with accepted academic practice. No use, distribution or reproduction is permitted which does not comply with these terms.
*Correspondence: Marie-Claire Gwayi-Chore, Y2d3YXlpQHV3LmVkdQ==