- 1International Centre of Insect Physiology and Ecology, Nairobi, Kenya
- 2Kenyatta University, Nairobi, Kenya
- 3Department of Zoology and Entomology, University of Pretoria, Pretoria, South Africa
Dengue virus (DENV) transmission risk is influenced by the bionomic traits of the key vector, Aedes aegypti. We investigated patterns of abundance, survival, and human blood-feeding of Ae. aegypti populations in two environments in Kenya: peri-urban Rabai (coastal Region, dengue-endemic) and rural Kerio Valley (Rift Valley Region, no reported dengue outbreak). In both environments, Ae. aegypti survival (estimated by parity), was inversely correlated with vector abundance, and this was influenced by weather conditions, notably temperature and relative humidity. In Rabai, Ae. aegypti mostly fed on humans (human blood index=51%), a pattern that corroborates with dengue cases in the coastal region. Aedes aegypti additionally, exhibited opportunistic feeding (livestock, rodents, reptiles, birds), suggesting the risk of human exposure to zoonotic pathogens via spillover transmission events aided by the vector. Abundance and human blood-feeding rates were consistently lower in Kerio Valley likely related to the degree of urbanization. Remarkably, the periods of high human feeding in Rabai coincided with high vector survival rates, a trend that could potentially drive intense DENV transmission at certain times of the year. We found a genetic influence of Ae. aegypti on the degree of anthropophagy but this could be influenced by potential seasonal shifts in human feeding. The findings of this study have implications both for DENV transmission risk and vector control strategies, but also in modeling which should integrate vector bionomic factors beyond vector abundance.
Introduction
Dengue has re-emerged as one of the most important infectious arboviral diseases across the globe and it is exerting a toll not only on human health but also on the economic development of affected countries (1). The disease is caused by four distinct dengue virus (DENV) serotypes namely Dengue 1, 2, 3 and 4, which are transmitted to humans primarily through the bite of an infected Aedes aegypti mosquito (2, 3). According to the WHO, about half of the world’s population is currently at risk of dengue infection, especially in tropical and subtropical areas. Globally, dengue cases have increased >10-fold between 2000 and 2019 (500,000 to 5.2 million), indicating expanding epidemiology of the disease. Reported deaths increased four-fold during the same period (~1000 in 2000 to ~4000 in 2015) (1). The global trend is unparalleled in the eastern African region where frequent dengue outbreaks continue to occur in Kenya and in neighboring countries like Tanzania, Somalia, Djibouti, Eritrea, Sudan and Ethiopia (1, 4–8). For instance, in Kenya the public health impact of dengue has continued to rise sharply, with successive recent outbreaks (between 2011-2022) resulting in hundreds of thousands of human cases and multiple fatalities (9). These frequent outbreaks and the co-circulation of all four DENV serotypes (10) are an indication of the significant burden posed by dengue.
The geographic spread of dengue occurs in part against a backdrop of limited understanding of the transmission dynamics, and ecology, including the bionomic role of geographic populations of the known vectors. Convergence in the basic components of the disease cycle: the virus, vector, and susceptible human hosts; in a permissive environment, provides the necessary ingredients that facilitate local arboviral pathogen emergence (11, 12). Yet, amidst the geographic spread, dengue risk patterns at local scales can be substantially heterogeneous (1, 13, 14). A better understanding of the complex interactions among these factors is required to predict the risk of dengue spread (15), but knowledge on vector populations and adaptation under changing habitat conditions are critical to achieving this.
Epidemic dengue cycles occurring in urban/peri-urban environments are increasing in eastern Africa and are driven primarily by the adaptation and expansion of vector range (e.g., Ae. aegypti) (16). Tracking changes in relevant biological parameters that define vectorial capacity can improve the prediction and response to infections or outbreaks in a timely manner (17, 18). Key among these is human blood-feeding preference (including multiple feeding) and survival (longevity) of the vector which controls opportunities for infection and transmission intensity (19–21). Besides density, measuring these vector traits in natural mosquito populations could be useful in risk assessment and to guide the implementation of vector control strategies. Mosquito longevity is a limiting factor for the transmission of arboviral pathogens, as pathogens must undergo a period of development (extrinsic incubation period - EIP) within the vector before it becomes transmissible via the salivary glands. EIP is temperature-sensitive with mean values of 15 and 6.6 days estimated for DENV at 25°C and 30°C, respectively (22). Few studies especially in Africa have described components of vectorial capacity (VC) for Ae. aegypti mostly relating to human blood meal patterns (13, 21, 23). Also, survivorship of natural populations of Ae. aegypti is seldom estimated (20, 24). Additionally, little information currently exists regarding possible differences in vector survival, as well as human blood-feeding among the two known ecotypes: the sylvan Ae. aegypti formosus and the domestic Ae. aegypti aegypti. Variations in virus vectoring abilities and geographic distribution between the forms have been described (21, 25).
Weather parameters (e.g., temperature, rainfall, humidity) modulate various biological and behavioural processes of mosquito vectors. These and other non-climatic variables such as human mobility and urbanization have been implicated as important drivers of dengue spread and outbreak occurrence (15). How these factors interact to influence bionomic traits and local transmission has received little attention.
To improve our understanding of local-scale variation in dengue occurrence, this study investigated the survival and blood-feeding patterns of wild Ae. aegypti in two ecosystems that vary in dengue outbreak occurrences: peri-urban (coastal Rabai) and rural (Rift Valley Kerio Valley) in Kenya. Also, we examined the relationship between Ae. aegypti age structure and abundance and assessed whether the genetic variability in the vector corelates with the extent of human blood-feeding.
Materials and methods
Ethical approval
The study received approval from the Scientific Ethics Review Unit (SERU) of the Kenya Medical Research Institute (Protocol NO. SSC 2787). Additionally, consent was sought from household heads to set up traps around their homesteads.
Study sites
Host seeking female Ae. aegypti collected outdoors around residential areas in two environments- peri-urban environment (human density ~600/km2) in Rabai and rural environment (human density ~ 45/km2) in Kerio Valley (KV) were used in this study. Rabai (Kilifi County) is located northeast and ~25 km from Mombasa City in the dengue-endemic coastal Kenya (26) while KV with a history of YF outbreak (27) (no reported dengue outbreak) is in the Rift Valley Region (Figure 1). The larval habitats of Ae. aegypti differ in both areas, with KV providing a rural woodland setting with numerous tree holes for mosquito breeding as opposed to Rabai, a peri-urban setting providing common water storage containers for mosquitoes to breed in addition to outdoor breeding in plant axils and water receptacles.
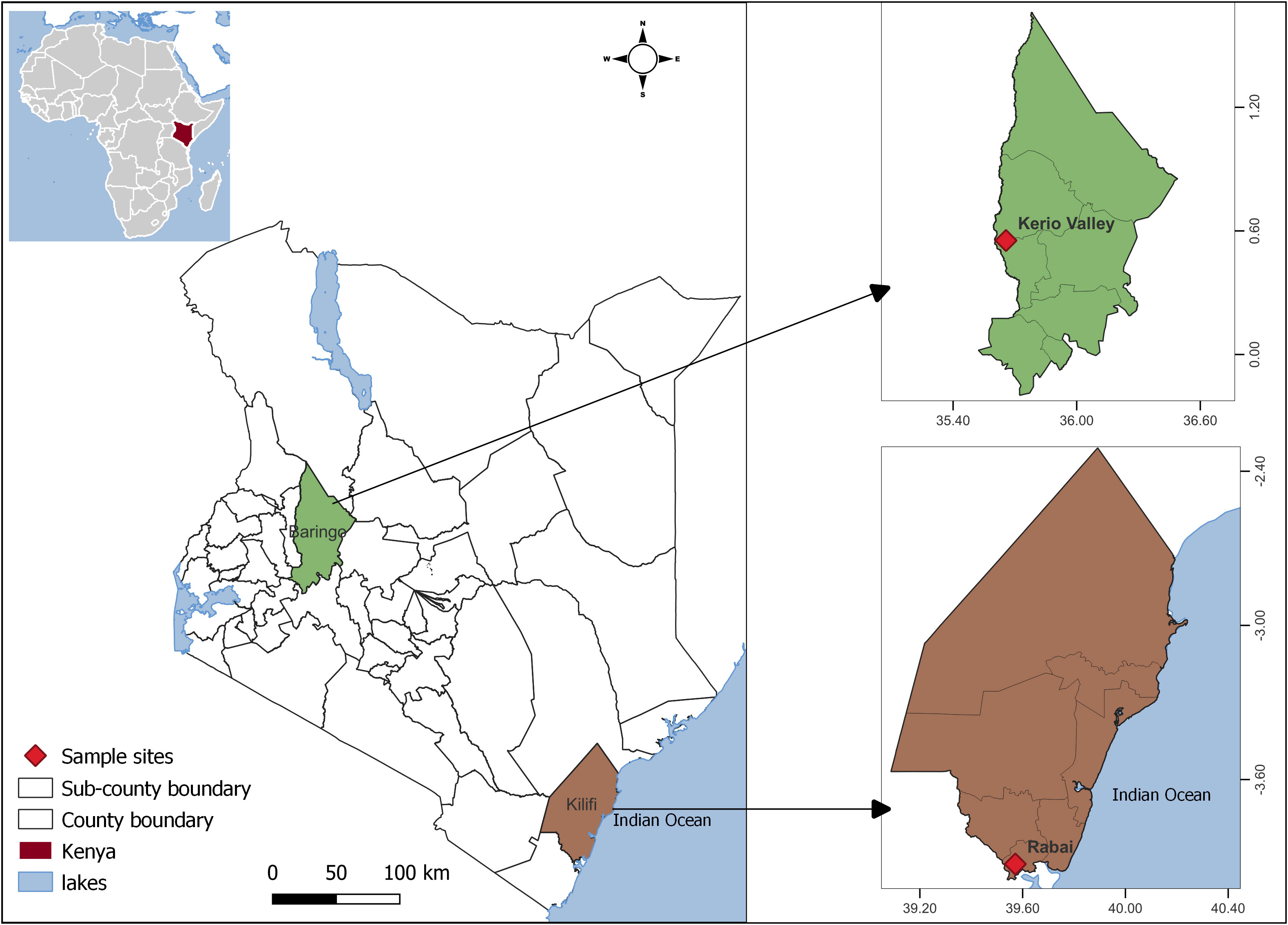
Figure 1 Map of Kenya showing location of the study sites. The map was designed using ArcMap 10.2.2. with the ocean and lakes base layer derived from Natural Earth (http://www.naturalearthdata.com/, a free GIS data source). The locations were collected using a GPS gadget (garmin etrex 20, https://buy.garmin.com/en-US/US/p/518046), and the county boundaries for Kenya derived from AfricaOpendata (https://africaopendata.org/dataset/kenya-counties-shapefile, license Creative Commons).
Mosquito surveys and weather parameters
Aedes aegypti mosquitoes were trapped using CO2-baited BG-Sentinel traps supplemented with chemical attractants (28) at three-time points: KV (November 2019) and Rabai (August-September 2019; February 2020). Each trapping covered a period of 2-3 weeks. Being a day-active mosquito, traps were set outdoors around homesteads at 06:30 h and retrieved at 18:00 h on the same day. Sampling was intended to encompass a large spatial area per site. Collected samples were immobilised using triethylamine, preserved in liquid nitrogen in the field and transported to the laboratory at icipe. Ae. aegypti among the specimens were identified morphologically using taxonomic keys and then stored at -80°C.
The weather-related parameters during the trapping periods were as follows: August-September 2019 (mean daily temperature=25.4°C; mean daily rainfall=0.58mm; relative humidity=75.9%) and February 2020 (mean daily temperature=27.4°C; mean daily rainfall=0.30mm; relative humidity=80.3%) in Rabai and November 2019 (mean daily temperature=19.9°C; mean daily rainfall=3.76mm; relative humidity=72.4%) in KV. These were derived from sourced weather data for each study site using the GPS location data. Daily weather data including relative humidity, precipitation, and temperature during the trapping period was extracted from NASA (National Aeronautics and Space Administration) POWER (Prediction of Worldwide Energy Resource) at the NASA Langley Research Center - https://power.larc.nasa.gov/. This is a satellite sensed weather dataset derived from satellite imagery, ground observations and assimilation models. The platform provides global gridded daily meteorological data from 1981 to near real time at a spatial resolution of 0.5°. Satellite-sensed weather data was used in place of the ground observed weather data due to large spatial gaps in weather station coverage in the study areas. Studies have proven that there is a strong correlation between the NASA data and ground station data (29, 30).
Parity rate, daily survival, and longevity estimation
Adult female mosquitoes were dissected for parity (31), which estimates vector survival in natural populations. Daily survival rates were estimated from parity data for each sampling period as described previously (32) using the formula: Pn = M, where P is the daily survival rate, M the parity rate and n representing the gonotrophic cycle i.e., the number of days between adult female blood meal and first oviposition. Published data on parity for Ae. aegypti range from 3-4 days for populations outside of Africa (33–36) and this reduces with dry conditions. For tropical Africa with much higher temperatures, we assumed a value of n=3. Mosquito longevity (life expectancy in days) was derived using the formula: 1/(-lnP) where P is the estimated daily survival rate (37).
Analysis of blood meals
Blood-fed specimens were individually dissected by separating the abdomen containing engorged blood from the head/thorax. The head/thorax was preserved for each mosquito and subjected to DNA extraction and molecular speciation (described below). Genomic DNA was extracted from the abdomen using the ISOLATE II Genomic DNA Kit (Bioline, Meridian Bioscience, Germany) as per the manufacturer’s instructions. DNA was amplified by targeting a 500 bp fragment of the 12S mitochondrial rRNA gene using the primers 12S3F [5’-GGGATTAGATACCCCACTATGC-3’] and 12S5R [5’-TGCTTACCATGTTACGACTT-3’] (38) as described previously (18, 26). PCRs in a 10 μl reaction volume comprised 2 μl 2x MyTaq Mix (Bioline, Germany), 10 μM of each primer, 0.2 U of Mytaq DNA polymerase and 1 μl of the template DNA (~20 ng). Thermal cycling conditions were 95°C for 3 min followed by 40 cycles at 95°C for 20 s, 59°C for 30 s and 72°C for 30 s and 72°C for 7 min. Amplicons were resolved on 1.2% agarose gel electrophoresis against a 100bp DNA HyperLadder (Bioline, Meridian Bioscience, Tennessee, USA). The PCR products were purified using the SureClean Plus kit (Bioline, Meridian Bioscience) and outsourced to Macrogen Europe BV (Amsterdam, The Netherlands) for Sanger sequencing using the forward primer. DNA sequences were compared using the BLAST algorithm and the GenBank database (http://blast.ncbi.nlm.nih.gov/Blast.cgi). Species level identification was determined when sequences exhibited ≥ 98% identity spanning at least 300 bp as described previously (18, 26).
Discrimination of Ae. aegypti genetic forms among blood-fed specimens
The Ae. aegypti genetic forms, domestic Ae. aegypti aegypti (Aaa) and forest Ae. aegypti formosus (Aaf) cannot be reliably identified morphologically, requiring genetic approaches. To achieve this, genomic DNA was extracted from the head/thorax of each blood fed specimen as described above. PCR was then performed targeting an 860 bp barcode region of mitochondrial Cytochrome C Oxidase subunit 1 (CO1) gene. DNA was amplified using the primers CO1-22-F 5’-TGTAATTGTAACAGCTCATGCA-3’ and CO1-22-R 5’-AATGATCATAGAAGGGCTGGAC-3’ (39). This marker has shown utility in resolving differences between Aaa and Aaf (13) The PCR was performed using MyTaq HS Mix kit (Bioline, Germany), the reaction volume comprised of 5μl of 2xMytaq HS mix polymerase, 10 M of each primer and a template DNA of 2 μl using the thermal cycling conditions of 95°C for 2 min followed by 40 cycles at 95°C for 30 s, 60°C for 30 s and 72°C for 45 s and 72°C for 7 min. Amplicons were confirmed by gel electrophoresis and the PCR products similarly purified and sequenced as explained earlier; however, in both the forward and reverse direction.
Cleaned sequences were aligned and maximum likelihood (ML) trees constructed with nodal support for the different groupings evaluated through 1000 bootstrap replications utilizing the Tamura 3-parameter with gamma distribution and proportion of invariant sites as best-fit model of sequence evolution. The haplotypes generated in this study were deposited in GenBank under accession numbers OP920609 - OP920647.
Statistical analyses
The parity rate calculated as the percentage of parous mosquitoes to the total number dissected was established for each trapping period and comparisons were made by Pearson chi-squared tests. The 95% confidence intervals (CIs) for the parity rates were estimated using binom.confint
function. The human blood index (HBI) expressed as the proportion of blood-feeding on humans of the total number of engorged mosquitoes with successful host identification, was compared by trapping periods or mitochondrial COI lineages using Pearson chi-squared tests. All analyses were performed at α=0.05 level of significance using R v. 4.21.
Results
Ae. aegypti survival rate varies by site and study periods
A total of 981 Ae. aegypti (non-blood fed) were successfully scored for parity from KV and Rabai across the three sampling periods recording an overall parous rate of 74.9% (735/981; 95%CI 72.1-77.5%). Parous rates in Rabai were 67.7% (212/313; 95%CI 62.4-72.7%) in August-September 2019 and 77.8% (277/356; 95%CI 73.2-81.8%) in February 2020, and 78.8% (246/312; 95%CI 74.0-83.0%) in KV in November 2019. Parous rates differed significantly only between the collection periods in Rabai (χ2 = 8.10, df = 1, p=0.004) (Table 1). In Rabai, estimated daily survival rates ranged from 0.87 in August-September 2019 to 0.91 in February 2020. A value of 0.92 was estimated for KV in November 2019. Overall, estimated longevity ranged from 7.7 to 12.7 days across the periods (Table 1).
Ae. aegypti human feeding rates vary by area and survey period
Of the 53-blood fed Ae. aegypti analysed from both areas, blood meal sources were successfully profiled in 47 (88.7%) of which 44 (94%) were from Rabai and 3 (6%) from KV. Nine hosts were represented in individual blood meals with the highest proportion from humans (24/47; Figure 2A). This was followed by blood meals from domestic dog (8/47), African giant pouched rat (4/47), lizard (3/47), and minor representation of domestic cat (2/47), goat (2/47), bat (1/47), tortoise (1/47) and mongoose (1/47). The hosts commonly utilised in both areas were humans, domestic dog and lizard (Figure 2A). The three engorged specimens from KV had each fed on mongoose, goat and African giant pouched rat. Collectively, the HBI was 51% (24/47), although when disaggregated by trapping period, the HBI varied from 0 (0/3) in KV, 0.33 (6/18) in Rabai August-September 2019, to 0.69 (18/26) in Rabai February-2020. The HBI was significantly different between the study periods in Rabai (χ2 = 4.18, df = 1, p = 0.04).
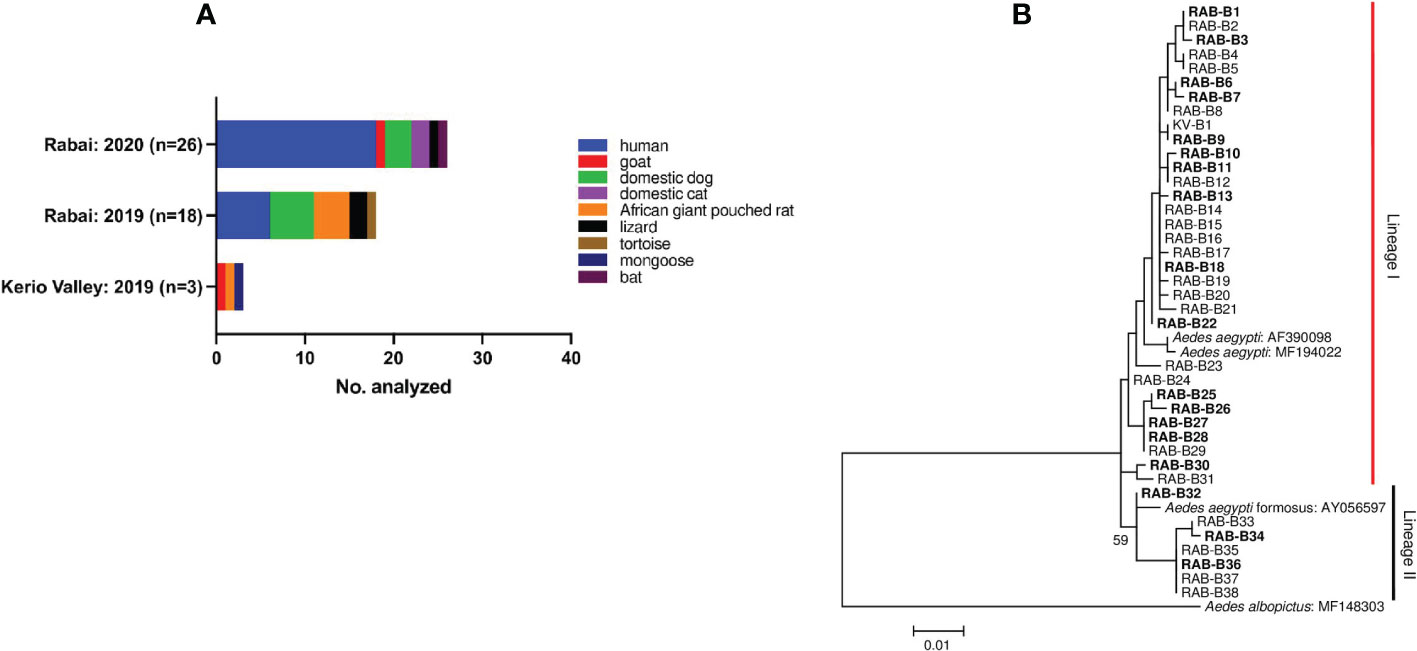
Figure 2 (A) Aedes aegypti-host feeding associations and (B) Maximum-likelihood tree derived from cox1 sequences of blood-fed specimens using a Tamura 3-parameter model with gamma distribution and proportion of invariant sites (746 nt). Bootstrap values are shown above relevant nodes. Sequence of Ae. albopictus indicated as outgroup. The scale-bar indicates the number of substitutions per site. Taxon abbreviations represent sampling sites with numbers corresponding to specific sequence samples: RAB, Rabai; KV, Kerio Valley. Sequences in bold font are samples that fed on humans. Sequences were submitted to GenBank with accession numbers OP920609 - OP920647.
Human-biting Ae. aegypti represented among mitochondrial COI lineages
Next, we addressed whether human blood feeding rates varied between the Ae. aegypti genetic forms. Phylogenetic analysis was performed on sequences of 37 blood-fed Ae. aegypti (successful of the 47 processed) from both study areas (KV=1, Rabai=36). Two mitochondrial lineages were recovered in the maximum likelihood phylogenetic tree. Most of the specimens (n=30) clustered in lineage 1 containing the domestic form (Ae. aegypti aegypti — GenBank Accession no: AF390098 and MF194022) (Figure 2B). Lineage II grouped with the feral form (Ae. aegypti formosus—GenBank Accession no. AY056597) and had few samples (n=7). Estimated HBI for lineage I was 0.53 (16/30) and 0.43 (3/7) for lineage II and the rates did not differ significantly (p=0.94). The one engorged specimen from KV represented in lineage I, had fed on rodent.
Mosquito survival is inversely related to trap densities
A summary of the parity rates, mean Ae. aegypti densities and prevailing weather conditions (mean temperature, relative humidity and rainfall) for each of the trapping periods are presented in Table 2. Mean daily Ae. aegypti catches/trap were 2.9, 18.7 and 8.8 in KV (November 2019), Rabai in August-September 2019 and February 2020, respectively, and correlate inversely with respective parity rates during these periods. When data were stratified by trapping periods in coastal Rabai, we found increased mosquito survival with an increase in mean temperature (25.4°C to 27.4°C) and relative humidity (75.9 to 80.3) from August-September 2019 to February 2020 (Table 2). Notably, both Ae. aegypti HBI and survival were higher in February 2020 than in August-September 2019 in coastal Rabai. Also, HBI values increased as rainfall decreased between the two trapping periods in Rabai with a similar inverse relationship observed between survival and rainfall.

Table 2 Summary of Ae. aegypti bionomic estimates in relation to weather variables during the trapping periods.
Discussion
Like other mosquito-borne pathogens, the transmission efficiency of DENV is dependent on critical components of vectorial capacity such as mosquito density, survival and human feeding preference. Here, we document how these factors could interact with each other to influence dengue dynamics influenced by weather factors. We found variation in vector parameters as a function of trapping period, a pattern that appears to mirror the observed seasonal variation in transmission risk in the Kenyan coast (40, 41).
Mosquito abundance increases the likelihood of vector-human contact and is an important parameter in climate-based models to predict dengue dynamics (42). While vector presence is a prerequisite for DENV transmission, increasingly, vector density could be a poor predictor of DENV infection risk in humans. For instance, an area with the lowest Ae. aegypti density in Vietnam displayed the highest incidence of dengue while the converse effect was true in another province (43). A similar inverse relationship between Ae. aegypti abundance and dengue endemicity was observed in Mexico (44). Also, higher abundance of Ae. aegypti in the Kenyan city of Kisumu than Mombasa, could not explain the lack of outbreaks in the former, yet recurrent in the latter (14). In our study, high vector survival rate was associated with reduced trap catches, a proxy for mosquito abundance. The strong association between mosquito age and dengue endemicity (44) or onset and offset of DENV transmission season (24), could indicate that vector demographic structure is a better predictor of dengue risk than density.
The estimated survival rates for Ae. aegypti from this study are consistent with the range of data for this species reported in the literature (parous rates: 27-92%) (24, 35, 45). Parity assumes age-independent survival (46), although Hugo et al. (24), found no difference employing age dependent and independent models in the estimation of mosquito age. The samples analyzed in our study were representative of captures over several days within a spatial scale for each trapping period potentially increasing the reliability of the estimates as previously suggested (46). Of note, parity estimates could be affected by the mosquito population recruitment whether growing, stable, or declining (47) which we did not assess at the different study sites.
The blood meal profiles for Ae. aegypti included humans but also a diverse range of animals. We observed a moderate HBI (0.51) in Rabai, a value which is comparable or even higher than the previously reported estimate (of 0.4) in coastal Kenya (13). In this study, Ae. aegypti is observed to exhibit zoophilic characteristics, an observation that contradicts the primarily anthropophilic behavior (HBI > 0.87-1) of this species in other dengue endemic areas out of Africa (21, 48, 49). The difference in Ae. aegypti HBI could be related to the origin of the samples (indoor vs outdoor) and habitat (urban vs rural/sylvatic). Lower blood fed specimens were encountered in KV (n=3), none of which had fed on humans. Thus, the difference in Ae. aegypti blood feeding between Rabai and KV could relate to the degree of urbanization i.e., human population density, which is an important risk parameter of dengue risk (2, 14). The observation of a more anthropophilic population in Rabai compared to KV may also point to fundamental differences in the behavior of Ae. aegypti populations in areas/regions of differing dengue endemicity in Kenya which, requires further elucidation. While the blood meal data provides insights into the trophic habit of this species, this inference could be affected by the relatively low number of engorged specimens analyzed (n=53). Our trapping methodology mainly targeted host-seeking females generally biased against blood-feeding cohorts whose catches can be improved by incorporating resting collections (50).
Genetic differences underlie the preference for human over other vertebrate hosts between Aaa and Aaf (51). We asked whether this can be verified through correlational studies of the genetics and host meal sources of individually analysed wild blood-fed specimens. Our data show that the mtCOI lineage that grouped with the domestic form Aaa was more associated with a higher human feeding rate (0.53 (16/30)) compared to the lineage having the feral form (0.43 (3/7) (Figure 2B). We suspect that the lack of a significant difference in the human feeding rates between the lineages may have been masked by tropic shifts between the trapping periods observed at the coast. Our findings point to sympatric occurrence of Ae. aegypti ecotypes, however, with possible higher abundance of the domestic than feral form in coastal Kenya as previously suggested (13, 52). The potential consequence of seasonality in their occurrence, biting patterns and effect on transmission risk merit additional studies. Feeding on diverse hosts including humans by both lineages representing the forms increases the risk of transmission to humans of diverse vector-borne pathogens (e.g., dengue, chikungunya, and Zika viruses) that are known to have animal reservoirs (53). The contribution of multiple human feeding, a common trait in Ae. aegypti (19, 54) could not be determined based on the single marker used in our present study. PCR followed by amplicon sequencing analysis underestimates the resolution of multiple host meals from individually engorged mosquitoes compared to next-generation sequencing approaches (55, 56).
Coastal Kenya has been particularly prone to multiple dengue outbreaks in the last decade (3, 57) with annual upsurges experienced after the short and long rains typically between February and June (40, 41). While rainfall may increase mosquito abundance and perhaps biting rates, the reasons for this outbreak occurrence patterns are not fully understood. Focusing on our coastal data, we analyzed trends in vector indices and weather-related variables during the two sampling periods of August-September 2019 and February 2020. The data showed that higher vector survival coincided with higher human feeding rates (i.e., HBI) in February 2020. Both values were consistently lower in August-September 2019 (Parity=77% vs 67% and HBI=69% vs 29% for February 2020 and September 2019, respectively). High human feeding rates have been shown to correlate with heightened period of dengue risk (33,54]. February 2020 are generally dry/cool periods which have been found to correlate positively with enhanced vector survival rates and dengue transmission as observed in Vietnam (24). Higher vector survival was associated with both increases in mean temperature and relative humidity, during this period compared to August-September 2019, suggesting them as influential factors affecting mosquito age (or longevity) consistent with previous findings (58). It is noteworthy that temperature increases including a 1°C rise can accelerate a reduction in the extrinsic incubation period (EIP) and thereby, increase pathogen transmission potential (59). Our results suggest that intense DENV transmission and perhaps onset of outbreaks at specific times of the year may be punctuated by enhanced Ae. aegypti vectorial capacity linked to increased vector survival and human feeding in concert with suitable weather conditions. A convergence of these biotic and abiotic attributes could be critical in reinitiating the seasonal pattern of dengue virus transmission in coastal Kenya. The low temperature conditions, low human biting may have dampened the estimated dengue risk in KV despite evidence of high survival rates. It is also noteworthy that we assumed a uniform gonotrophic cycle (3 days) in our estimates of daily survival and longevity and this value has been observed to vary as a function of temperature and seasons (35, 60). Thus, further studies should consider this likely discrepancy which could influence interpretation of the findings.
Conclusion
We conclude that, Ae. aegypti exhibits local variation in important components of vectorial capacity (VC)- abundance, survival, and human blood feeding. In coastal Rabai, vector density was inversely related to mosquito age which together with human feeding are the most sensitive VC parameters (20, 26). Convergence of high survival and propensity to feed on humans as noted in coastal Rabai, could drive intense DENV transmission or onset of outbreaks at certain times of the year; however, in concert with suitable weather conditions. Genetic factors likely underpin the degree of anthropophagy in Ae. aegypti but may be influenced by potential seasonal shift in human feeding rates. Despite high representation of humans in the trophic habit, Ae. aegypti exhibited opportunistic feeding, a trend which increases human exposure to risk of zoonotic pathogens. Modeling transmission is increasingly seen as an important component of disease risk prediction. For dengue, quantifying transmission risk needs to integrate vector bionomic factors beyond abundance together with climatic parameters in simulation studies for reliability of any developed models (15, 61).
Data availability statement
The original contributions presented in the study are included in the article/supplementary material. Further inquiries can be directed to the corresponding author.
Ethics statement
The study received approval from the Scientific Ethics Review Unit (SERU) of the Kenya 116 Medical Research Institute (Protocol NO. SSC 2787). Additionally, consent was sought from household heads to set up traps around their homesteads.
Author contributions
Conceptualization, RS and DT. Investigation and Methodology, WK, RS, GR, DT. Data analyses, WK, DT. Validation, RS, DT. Supervision, RS, NM, DT. Funding acquisition, RS, BT, DT. Writing—original draft, DT. Writing—review and editing, WK, RS, GR, SA, NM, BT, DT. All authors contributed to the article and approved the submitted version.
Funding
This study was conducted under the project Combatting Arthropod Pests for better Health, Food and Climate Resilience (CAP-Africa) funded by the Norwegian Agency for Development Cooperation (Norad) (Grant number: RAF-3058 KEN-18/0005). DT is supported by a Wellcome Trust International Intermediate Fellowship (222005/Z/20/Z). We gratefully acknowledge the financial support for this research by the following organizations and agencies: Swedish International Development Cooperation Agency (Sida), Swiss Agency for Development and Cooperation (SDC), Australian Centre for International Agricultural Research (ACIAR), Federal Democratic Republic of Ethiopia and the Government of the Republic of Kenya. The views expressed herein do not necessarily reflect the official opinion of the donors.
Acknowledgments
We thank community members in the study areas who provided access to their homesteads. We are thankful to Emily Kimathi, icipe, Nairobi, for designing the map of the study sites.
Conflict of interest
The authors declare that the research was conducted in the absence of any commercial or financial relationships that could be construed as a potential conflict of interest.
Publisher’s note
All claims expressed in this article are solely those of the authors and do not necessarily represent those of their affiliated organizations, or those of the publisher, the editors and the reviewers. Any product that may be evaluated in this article, or claim that may be made by its manufacturer, is not guaranteed or endorsed by the publisher.
References
1. World Health Organization. Fact sheets: Dengue and severe dengue. (who.int). (2022). Dengue and severe dengue (who.int).
2. Gubler DJ. Epidemic dengue/dengue hemorrhagic fever as a public health, social and economic problem in the 21st century. Trends Microbiol (2002) 10:100–3. doi: 10.1016/S0966-842X(01)02288-0
3. Sang R, Lutomiah J, Chepkorir E, Tchouassi DP. Evolving dynamics of Aedes-borne diseases in Africa: A cause for concern. Curr Opin Insect Sci (2022) 53:100958. doi: 10.1016/j.cois.2022.100958
4. Malik A, Earhart K, Mohareb E, Saad M, Saeed M, Ageep A, et al. Dengue hemorrhagic fever outbreak in children in port Sudan. J Infect Public Health (2011) 4:1–6. doi: 10.1016/j.jiph.2010.08.001
5. de Laval F, Plumet S, Simon F, Deparis X, Leparc-Goffart I. Dengue surveillance among French military in Africa. Emerg Infect Dis (2012) 18:342–3. doi: 10.3201/eid1802.111333
6. Bosa HK, Montgomery JM, Kimuli I, Lutwama JJ. Dengue fever outbreak in Mogadishu, Somalia 2011: co-circulation of three dengue virus serotypes. Int J Infect Dis (2014) 21:3. doi: 10.1016/j.ijid.2014.03.412
7. Usman A, Ball JD, Rojas DP, Berhane A, Ghebrat Y, Mebrahtu G, et al. Dengue fever outbreaks in Eritrea, 2005–2015. Glob Health Res Policy (2016) 1:17. doi: 10.1186/s41256-016-0016-5
8. Mwanyika GO, Mboera LE, Rugarabamu S, Makange M, Sindato C, Lutwama JJ, et al. Circulation of dengue serotype 1 viruses during the 2019 outbreak in dar es salaam, Tanzania. Pathog Glob Health (2021) 115(7-8):467–75. doi: 10.1080/20477724.2021.1905302
9. Langat SK, Eyase FL, Berry IM, Nyunja A, Bulimo W, Owaka S, et al. Origin and evolution of dengue virus type 2 causing outbreaks in Kenya: Evidence of circulation of two cosmopolitan genotype lineages. Virus Evol (2020) 6:veaa026. doi: 10.1093/ve/veaa026
10. Shah MM, Ndenga BA, Mutuku FM, Vu DM, Grossi-Soyster EN, Okuta V, et al. High dengue burden and circulation of 4 virus serotypes among children with undifferentiated fever, Kenya, 2014–2017. Emerg Infect Dis (2020) 26:2638–50. doi: 10.3201/eid2611.200960
11. Eldridge BF. Strategies for surveillance, prevention, and control of arbovirus diseases in western north America. Am J Trop Med Hyg (1987) 37(3_Part_2):77S–86S. doi: 10.4269/ajtmh.1987.37.77S
12. Torto B, Tchouassi DP. Grand challenges in vector-borne disease control targeting vectors. Front Trop Dis (2021) 1:635356. doi: 10.3389/fitd.2020.635356
13. Agha SB, Tchouassi DP, Turell MJ, Bastos AD, Sang R. Entomological assessment of dengue virus transmission risk in three urban areas of Kenya. PLoS Negl Trop Dis (2019) 13:e0007686. doi: 10.1371/journal.pntd.0007686
14. Agha SB, Tchouassi DP. Urbanisation of Aedes populations and evolution of arboviral disease risk in Africa. Curr Opin Insect Sci (2022) 54:100988. doi: 10.1016/j.cois.2022.100988
15. Tozan Y, Sjödin H, Muñoz ÁG, Rocklöv J. Transmission dynamics of dengue and chikungunya in a changing climate: do we understand the eco-evolutionary response? expert rev. Anti-Infect Ther (2020) 18:1187–93. doi: 10.1080/14787210.2020.1794814
16. Kraemer MU, Sinka ME, Duda KA, Mylne AQ, Shearer FM, Barker CM, et al. The global distribution of the arbovirus vectors Aedes aegypti and ae. albopictus. elife (2015) 4:e08347. doi: 10.7554/eLife.08347
17. LaDeau SL, Allan BF, Leisnham PT, Levy MZ. The ecological foundations of transmission potential and vector-borne disease in urban landscapes. Funct Ecol (2015) 29:889–901. doi: 10.1111/1365-2435.12487
18. Tchouassi DP, Torto B, Sang R, Riginos C, Ezenwa VO. Large Herbivore loss has complex effects on mosquito ecology and vector-borne disease risk. Transbound Emerg Dis (2021) 68:2503–13. doi: 10.1111/tbed.13918
19. Harrington LC, Fleisher A, Ruiz-Moreno D, Vermeylen F, Wa CV, Poulson RL, et al. Heterogeneous feeding patterns of the dengue vector, Aedes aegypti, on individual human hosts in rural Thailand. PLoS Negl Trop Dis (2014) 8:e3048. doi: 10.1371/journal.pntd.0003048
20. Lounibos LP, Kramer LD. Invasiveness of aedes aegypti and Aedes albopictus and vectorial capacity for chikungunya virus. J Infect Dis (2016) 214(suppl_5):S453–8. doi: 10.1093/infdis/jiw285
21. Tchouassi DP, Agha SB, Villinger J, Sang R, Torto B. The distinctive bionomics of Aedes aegypti populations in Africa. Curr Opin Insect Sci (2022) 54:100986. doi: 10.1016/j.cois.2022.100986
22. Chan M, Johansson MA. The incubation periods of dengue viruses. PLoS One (2012) 7(11):e50972. doi: 10.1371/journal.pone.0050972
23. Chepkorir E, Venter M, Lutomiah J, Mulwa F, Arum S, Tchouassi DP, et al. The occurrence, diversity and blood feeding patterns of potential vectors of dengue and yellow fever in kacheliba, West pokot county, Kenya. Acta Tropica (2018) 186:50–57. doi: 10.1016/j.actatropica.2018.07.008
24. Hugo LE, Jeffery JA, Trewin BJ, Wockner LF, Thi Yen N, Le NH, et al. Adult survivorship of the dengue mosquito Aedes aegypti varies seasonally in central Vietnam. PLoS Negl Trop Dis (2014) 8:e2669. doi: 10.1371/journal.pntd.0002669
25. Powell JR, Tabachnick WJ. History of domestication and spread of aedes aegypti-a review. Memórias do Instituto Oswaldo Cruz (2013) 108:11–7. doi: 10.1590/0074-0276130395
26. Kamau WW, Sang R, Ogola EO, Rotich G, Getugi C, Agha SB, et al. Survival rate, blood feeding habits and sibling species composition of Aedes simpsoni complex: Implications for arbovirus transmission risk in East Africa. PLoS Negl Trop Dis (2022) 16:e0010171. doi: 10.1371/journal.pntd.0010171
27. Reiter P, Cordellier RO, Ouma JO, Cropp CB, Savage HM, Sanders EJ, et al. First recorded outbreak of yellow fever in Kenya, 1992-93 II. Entomologic Investigations. Am J Trop Med Hyg (1998) 59:650–6. doi: 10.4269/ajtmh.1998.59.650
28. Tchouassi DP, Jacob JW, Ogola EO, Sang R. Torto B.Aedes vector-host olfactory interactions in sylvatic and domestic dengue transmission environments. Proc R Soc B (2019) 286:20192136. doi: 10.1098/rspb.2019.2136
29. White JW, Hoogenboom G, Stackhouse PW Jr., Hoell JM. Evaluation of NASA satellite- and assimilation model-derived long-term daily temperature data over the continental US. Agric For Meteorol (2008) 148:1574–84. doi: 10.1016/j.agrformet.2008.05.017
30. Aboelkhair H, Morsy M, El Afandi G. Assessment of agroclimatology NASA POWER reanalysis datasets for temperature types and relative humidity at 2 m against ground observations over Egypt. Adv Space Res (2019) 64:129–42. doi: 10.1016/j.asr.2019.03.032
31. Detinova TS. Age grouping methods in diptera of medical importance with special reference to some vectors of malaria. Monogr Ser World Health Organ (1962) 47:13–191. doi: 10.2307/3275215
32. Davidson G. Estimation of the survival-rate of anopheline mosquitoes in nature. Nature (1954) 174:792–3. doi: 10.1038/174792a0
33. Pant CP, Yasuno M. Field studies on the gonotrophic cycle of Aedes aegypti in Bangkok, Thailand. J Med Entomol (1973) 10:219–23. doi: 10.1093/jmedent/10.2.219
34. Baak-Baak CM, Ulloa-Garcia A, Cigarroa-Toledo N, Tzuc Dzul JC, Machain-Williams C, Torres-Chable OM, et al. Blood feeding status, gonotrophic cycle and survivorship of Aedes (Stegomyia) aegypti (L.) (Diptera: Culicidae) caught in churches from merida, Yucatan, Mexico. Neotrop. Entomol (2017) 46:622–30. doi: 10.1007/s13744-017-0499-x
35. Garcia-Rejon JE, Ulloa-Garcia A, Cigarroa-Toledo N, Pech-May A, Machain-Williams C, Cetina-Trejo RC, et al. Study of Aedes aegypti population with emphasis on the gonotrophic cycle length and identification of arboviruses: implications for vector management in cemeteries. Rev do Instituto Medicina Trop São Paulo (2018) 60:e44. doi: 10.1590/s1678-9946201860044
36. Casas-Martínez M, Tamayo-Domínguez R, Bond-Compeán JG, Rojas JC, Weber M, Ulloa-García A. Oogenic development and gonotrophic cycle of Aedes aegypti and Aedes albopictus in laboratory. Salud Pública México (2020) 62(4):372–8. doi: 10.21149/10164
37. Clements AN, Paterson GD. The analysis of mortality and survival rates in wild populations of mosquitoes. J Appl Ecol (1981) 8:373–99. doi: 10.2307/2402401
38. Roca AL, Kahila Bar-Gal G, Eizirik E, Helgen KM, Maria R, Springer MS, et al. Mesozoic origin for West Indian insectivores. Nature (2004) 429:649–51. doi: 10.1038/nature02597
39. Paupy C, Le Goff G, Brengues C, Guerra M, Revollo J, Simon ZB, et al. Genetic structure and phylogeography of Aedes aegypti, the dengue and yellow-fever mosquito vector in Bolivia. Infect Genet Evol (2012) 12:1260–9. doi: 10.1016/j.meegid.2012.04.012
40. Lim JK, Matendechero SH, Alexander N, Lee JS, Lee KS, Namkung S. Et alClinical and epidemiologic characteristics associated with dengue fever in Mombasa, Kenya. Int J Infect Dis (2020) 100:207–15. doi: 10.1016/j.ijid.2020.08.074
41. International Federation of Red Cross and Red Crescent Societies. Kenya: Dengue fever outbreak - operation update report DREF no. MDRKE048. (2021). ReliefWeb.
42. Li R, Xu L, Bjørnstad ON, Liu K, Song T, Chen A, et al. Climate-driven variation in mosquito density predicts the spatiotemporal dynamics of dengue. Proc Natl Acad Sci USA (2019) 116:3624–9. doi: 10.1073/pnas.1806094116
43. Pham Thi KL, Briant L, Gavotte L, Labbe P, Perriat-Sanguinet M, Cornillot E, et al. Incidence of dengue and chikungunya viruses in mosquitoes and human patients in border provinces of Vietnam. Parasit Vectors (2017) 10:556. doi: 10.1186/s13071-017-2422-z
44. Ernst KC, Walker KR, Reyes-Castro P, Joy TK, Castro-Luque AL, Diaz-Caravantes RE, et al. Aedes aegypti (Diptera: Culicidae) longevity and differential emergence of dengue fever in two cities in Sonora, Mexico. J Med Entomol (2017) 54:204–11. doi: 10.1093/jme/tjw141
45. dos Santos Andrade P, Urbinatti PR, da Rocha Coelho R, de Sá Almeida RM, Ribeiro SS, de Lima-Camara TN. Parity and gonotrophic discordance of females of Aedes aegypti and Aedes albopictus (Diptera: Culicidae) in the city of são paulo, SP, Brazil. J Vector Ecol (2019) 44:233–40. doi: 10.1111/jvec.12354
46. Charlwood JD. May the force be with you: measuring mosquito fitness in the field. In: Takken W, Scott TW, editors. Ecological aspects for application of genetically modified mosquitoes, vol. 2 . Wageningen: Wageningen UR (2002). p. 47–62. Frontis Series.
47. Briët OJT. A simple method for calculating mosquito mortality rates, correcting for seasonal variations in recruitment. Med Vet Entomol (2002) 16:22–7. doi: 10.1046/j.0269-283x.2002.00335.x
48. Ponlawat A, Harrington LC. Blood feeding patterns of Aedes aegypti and Aedes albopictus in Thailand. J Med Entomol (2005) 42(5):844–9. doi: 10.1093/jmedent/42.5.844
49. Sene NM, Diouf B, Gaye A, Ndiaye EH, Ngom EM, Gueye A, et al. Blood feeding patterns of Aedes aegypti populations in Senegal. Am J Trop Med Hyg (2022) 106:1402–5. doi: 10.4269/ajtmh.21-0508
50. Diouf B, Sene NM, Ndiaye EH, Gaye A, Ngom EH, Gueye A, et al. Resting behavior of blood-fed females and host feeding preferences of Aedes aegypti (Diptera: Culicidae) morphological forms in Senegal. J Med Entomol (2021) 58:2467–73. doi: 10.1093/jme/tjab111
51. McBride CS, Baier F, Omondi AB, Spitzer SA, Lutomiah J, Sang R, et al. Evolution of mosquito preference for humans linked to an odorant receptor. Nature (2014) 515:222–7. doi: 10.1038/nature13964
52. Futami K, Iwashita H, Higa Y, Lutiali PA, Sonye GO, Mwatele C, et al. Geographical distribution of Aedes aegypti aegypti and Aedes aegypti formosus (Diptera: Culicidae) in Kenya and environmental factors related to their relative abundance. J Med Entomol (2020) 57:772–9. doi: 10.1093/jme/tjz233
53. Weaver SC, Reisen WK. Present and future arboviral threats. Antiviral Res (2010) 85:328–45. doi: 10.1016/j.antiviral.2009.10.008
54. Scott TW, Chow E, Strickman D, Kittayapong P, Wirtz RA, Lorenz LH, et al. Blood-feeding patterns of Aedes aegypti (Diptera: Culicidae) collected in a rural Thai village. J Med Entomol (1993) 30:922–7. doi: 10.1093/jmedent/30.5.922
55. Estrada-Franco JG, Fernández-Santos NA, Adebiyi AA, López-López MD, Aguilar-Durán JA, Hernández-Triana LM, et al. Vertebrate-Aedes aegypti and Culex quinquefasciatus (Diptera)-arbovirus transmission networks: Nonhuman feeding revealed by meta-barcoding and next-generation sequencing. PLoS Negl Trop Dis (2020) 14:e0008867. doi: 10.1371/journal.pntd.0008867
56. Muturi EJ, Dunlap C, Tchouassi DP, Swanson J. Next generation sequencing approach for simultaneous identification of mosquitoes and their blood meal hosts. J Vector Ecol (2021) 46:116–21. doi: 10.52707/1081-1710-46.1.116
57. Konongoi L, Ofula V, Nyunja A, Owaka S, Koka H, Makio A, et al. Detection of dengue virus serotypes 1, 2 and 3 in selected regions of Kenya: 2011–2014. Virol J (2016) 13:182. doi: 10.1186/s12985-016-0641-0
58. Costa EA, Santos EM, Correia JC, Albuquerque CM. Impact of small variations in temperature and humidity on the reproductive activity and survival of Aedes aegypti (Diptera, culicidae). Rev Bras Entomologia (2010) 54:488–93. doi: 10.1590/S0085-56262010000300021
59. Githeko AK, Lindsay SW, Confalonieri UE, Patz JA. Climate change and vector-borne diseases: a regional analysis. Bull World Health Organ (2000) 78:1136–47.
60. Goindin D, Delannay C, Ramdini C, Gustave J, Fouque F. Parity and longevity of Aedes aegypti according to temperatures in controlled conditions and consequences on dengue transmission risks. PLoS One (2015) 10(8):e0135489. doi: 10.1371/journal.pone.0135489
Keywords: Aedes aegypti, genetic forms, vector survival, human feeding, vectorial capacity, dengue risk, urbanisation, sub-Saharan Africa
Citation: Kamau WW, Sang R, Rotich G, Agha SB, Menza N, Torto B and Tchouassi DP (2023) Patterns of Aedes aegypti abundance, survival, human-blood feeding and relationship with dengue risk, Kenya. Front. Trop. Dis 4:1113531. doi: 10.3389/fitd.2023.1113531
Received: 01 December 2022; Accepted: 23 January 2023;
Published: 06 February 2023.
Edited by:
David Weetman, Liverpool School of Tropical Medicine, United KingdomReviewed by:
Luca Facchinelli, Liverpool School of Tropical Medicine, United KingdomAthanase Badolo, University of Ouagadougou, Burkina Faso
Copyright © 2023 Kamau, Sang, Rotich, Agha, Menza, Torto and Tchouassi. This is an open-access article distributed under the terms of the Creative Commons Attribution License (CC BY). The use, distribution or reproduction in other forums is permitted, provided the original author(s) and the copyright owner(s) are credited and that the original publication in this journal is cited, in accordance with accepted academic practice. No use, distribution or reproduction is permitted which does not comply with these terms.
*Correspondence: David P. Tchouassi, dtchouassi@icipe.org