- 1Department of Biochemistry and Molecular Biology, Faculty of Science, University of Buea, Buea, Cameroon
- 2Department of Molecular Biology, Institute of Biology and Molecular Medicine (IBMM), Université Libre de Bruxelles, Gosselies, Belgium
- 3Randall Center for Cell and Molecular Biophysics, Faculty of Life Sciences and Medicine, King’s College London, New Hunts House, London, United Kingdom
- 4Frailty in Ageing Research Group, Vrije Universiteit Brussel, Brussels, Belgium
- 5Department of Gerontology, Faculty of Medicine and Pharmacy, Vrije Universiteit Brussel, Brussels, Belgium
- 6Department of Microbiology and Parasitology, Faculty of Science, University of Buea, Buea, Cameroon
- 7Division of Human Genetics, Health Sciences Campus, Department of Pathology, University of Cape Town, Cape Town, South Africa
- 8Department of Parasitology and Medical Entomology, Center for Research in Infectious Diseases, Yaoundé, Cameroon
- 9Ngonpong Therapeutics, Wilmington, DE, United States
- 10Department of Public Health and Hygiene, Faculty of Health Science, University of Buea, Buea, Cameroon
- 11Malaria Research Unit, Centre Pasteur Cameroon, Yaoundé, Cameroon
Almost a decade ago, it was recognized that the global elimination of onchocerciasis by 2030 will not be feasible without, at least, an effective prophylactic and/or therapeutic vaccine to complement chemotherapy and vector control strategies. Recent advances in computational immunology (immunoinformatics) have seen the design of novel multi-epitope onchocerciasis vaccine candidates which are however yet to be evaluated in clinical settings. Still, continued research to increase the pool of vaccine candidates, and therefore the chance of success in a clinical trial remains imperative. Here, we designed a multi-epitope vaccine candidate by assembling peptides from 14 O. volvulus (Ov) proteins using an immunoinformatics approach. An initial 126 Ov proteins, retrieved from the Wormbase database, and at least 90% similar to orthologs in related nematode species of economic importance, were screened for localization, presence of transmembrane domain, and antigenicity using different web servers. From the 14 proteins retained after the screening, 26 MHC-1 and MHC-II (T-cell) epitopes, and linear B-lymphocytes epitopes were predicted and merged using suitable linkers. The Mycobacterium tuberculosis Resuscitation-promoting factor E (RPFE_MYCTU), which is an agonist of TLR4, was then added to the N-terminal of the vaccine candidate as a built-in adjuvant. Immune simulation analyses predicted strong B-cell and IFN-γ based immune responses which are necessary for protection against O. volvulus infection. Protein-protein docking and molecular dynamic simulation predicted stable interactions between the 3D structure of the vaccine candidate and human TLR4. These results show that the designed vaccine candidate has the potential to stimulate both humoral and cellular immune responses and should therefore be subject to further laboratory investigation.
Introduction
Onchocerciasis is a devastating neglected tropical disease (NTD) caused by the parasitic nematode Onchocerca volvulus (O. volvulus) and transmitted by the Simulium blackflies (1). The flies breed around areas with cool-water rivers and streams, consequently, the disease is also called “river blindness” (2). The disease which manifests mainly as visual impairment and severe skin lesions (though in some cases nodules and lymphadenopathy) (3) exerts a significant socio-economic and public health burden (4) and is associated with susceptibility to HIV infection (5), immune-complex nephropathy (6), epilepsy (7), blindness (8), and human mortality (9). The Global Burden of Disease Study (10) estimates the number of infected persons at 21 million, with about 14.7 million people manifesting a range of onchocerciasis-related skin diseases and an additional 1.2 million suffering from various forms of visual impairment (10). Overall, an estimated 200 million people live in regions of disease endemicity with more than 99% of all cases (infected and at-risk) occurring in 31 countries in sub-Saharan Africa (11).
Though previous control programs focusing mainly on the use of chemotherapy with Ivermectin (IVM) mass drug administration (MDA) have succeeded to eliminate the disease in selected foci in Africa (12) and in 4 of the 6 previously endemic countries in the Americas (11 out of 13 foci) (13), the continuous use of IVM as the only tool (especially in Africa) to achieve global disease elimination is flawed by several factors and raises concerns about the feasibility of disease elimination by 2030 as initially planned (11): (1) Ivermectin is only microfilaricidal, requiring annual MDA for at least 14 years; which corresponds to the number of years adult female worms can reproduce (14). (2) The long-term treatment of infected persons with IVM has resulted in suboptimal microfilaricidal responses in several geographic locations (15, 16). (3) Severe neurologic adverse reactions to IVM have been reported in individuals coinfected with high titers of Loa microfilaria (17, 18). (4) Non-compliance/adherence to IVM treatment in some endemic populations impedes effective control of transmission (19, 20) and could contribute to the drive towards drug resistance (21). IVM is not recommended for pregnant women and children below the age of five, leaving this sizable population untreated and acting as a potential reservoir for the continuous spread of O. volvulus (22–24). In addition, some of the recommendations for accelerated elimination like maintaining a sufficiently high and prolonged IVM treatment, and biannual IVM administration are financially and logistically challenging (25). Moreover, some endemic areas are either highly enclaved and inaccessible or hit by conflicts and civil strife (12). These factors limit both the geographical and chemotherapeutic coverage needed to shorten the period required for interruption of parasite transmission and consequently attain the elimination goal (4).
The challenges encountered with the use of IVM MDA as the key strategy for control underscore the profound importance of continued investments in novel and alternative intervention strategies, including amongst other macrofilaricides, vector control, and vaccines (26). In fact, it has been postulated that preventative and therapeutic vaccines might expedite eradication efforts and protect the significant advancements gained in onchocerciasis control (27). This argument has been supported by the results from modeling studies which predicted that a preventative vaccine will lessen the disease burden in populations where IVM cannot be administered safely, forestalling the emergence of anthelmintic drug resistance, as well as decreasing the chance of disease recrudescence (28).
Several studies with humans and animal models have reported the feasibility of generating both natural and artificial protective immunity against onchocerciasis (29). Firstly, studies of human populations have postulated the feasibility of naturally acquired immunity against infection Onchocerca spp (30, 31). It has been reported that up to 1-5% of the populations living in onchocerciasis-endemic regions and exposed to bites of the vector do not exhibit any symptoms of the disease. These persons are therefore considered to have putative immunity (32). Secondly, concomitant immunity, characterized by the predisposition of human hosts to eliminate the newly introduced infective-stage larvae (L3), without having any effect on already established adult worms and microfilariae has also been reported (33). Lastly, immuno-epidemiological data supports the concept of zooprophylaxis, in which the concurrent and dominating transmission of O. ochengi by the Simulium damnosum to humans in sub-Saharan Africa could result in cross-protective immunity against O. volvulus (34).
For complex multi-stage parasites like O. volvulus, the selection of the appropriate antigen as a vaccine candidate remains a major challenge (35). Historically, two key approaches have been used to select target antigens. The first approach involved immunoscreening of parasite cDNA libraries (principally from the infective L3, molting L3, and L4 larval stages) using serum samples from putative immune (PI) individuals. The second approach involved the identification and isolation of proteins considered to be essential during the infection process (including proteins with vital metabolic or defense functions). This was based on the rationale that targeting such antigens for vaccine development could impede infection of the human host by the parasite (36).
Though these strategies led to the identification of a total of 44 candidates tested in the O. volvulus mouse model, presently only two candidates, Ov-RAL-2 and Ov-103 are planned for further clinical development (37). Nevertheless, suboptimal outcomes often obtained from human proof-of-concept clinical trials testify to the challenges/limitations of making extrapolations on vaccine efficacy studies during the translation from animal models to humans (38). A critical bottleneck for the generation of protective vaccines is the generation of suboptimal responses (27). In addition, the previous use of irradiated parasites presents logistical challenges which make this approach unsustainable (39). This points out the fact that there remains an urgent need for the continuous (preferably rational) search for novel vaccine candidates (40, 41).
Several reports have indicated that the use of single recombinant antigens as vaccine candidates produced inferior immune responses, consequently, reduced protective effects (42). Previous studies on onchocerciasis vaccine development, have focused mainly on the L3 and molting L3 (mL3) larval stages which are essential for the establishment of infection in humans. Meanwhile, the mf stage, responsible for most of the pathology observed in diseased persons has also been targeted (26). With the current challenges faced with selecting the right candidates for the development of novel vaccines for onchocerciasis, this study proposes the rational design of an epitope-based chimeric vaccine candidate for onchocerciasis generated from the ectodomains of parasite transmembrane proteins, based on the correlates of immune protection reported for the disease. It has been reported that surface proteins like those on the plasma membrane could play vital roles in protecting parasites against hosts’ defense responses (43). This localization, however, also makes the proteins accessible to the host immune response – making them suitable vaccine targets (44). In addition, for onchocerciasis, serum samples from PI and infected persons have been reported to contain antibodies that react with the L3 larval stage surface (36).
Studies in humans and animal models have reported the crucial role of both cellular and humoral immune responses in protection. In this regard, the role of CD8+ cells (45), a mixed Th1/Th2 cellular response (37), and cytophilic antibodies, acting through antibody-dependent cellular cytotoxicity, have been reported to be essential for the development of prophylactic immunity against Ov (46). In addition, the induction IFN-γ, IL-13, IL-4, and IL-5, has been reported to correlate with protection either against the L3 larvae or microfilariae (30, 47, 48). The role of an innate response in protective immunity has also been reported through TLR4 involvement (49).This work focuses on the design of a potentially cross-protective multi-epitope chimeric vaccine candidate against onchocerciasis using highly conserved parasite membrane proteins using an immuno-informatics approach taking into consideration the mechanisms involved in protection against the parasite.
Methods
Retrieval of parasite conserved proteins and preliminary analysis
The accession numbers of 106 proteins reported to share between 90-100% homology in related nematodes including A. simplex and B. malayi were obtained from the dataset previously generated by Cotton and colleagues (50). Sequences for the selected proteins were obtained from the WormBase database (wormbase.org) and then assessed for: 1. Localization (using the DeepLoc 1.0 server; http://www.cbs.dtu.dk/services/DeepLoc/); 2. The signal peptide presence (using the SignalP 5.0; http://www.cbs.dtu.dk/services/SignalP/ and PreDiSi servers; http://www.predisi.de/); 3. The presence of transmembrane domains (using the TMHMM; http://www.cbs.dtu.dk/services/TMHMM/ and TOPCONS; https://topcons.cbr.su.se/servers) and 4. Antigenicity (with the Vaxijen v2.0 server; http://www.ddg-pharmfac.net/vaxijen/VaxiJen/VaxiJen.html). All hypothetical proteins predicted to be localized, possess transmembrane domains, and are antigenic were selected for further analyses. For the selected proteins, only extracellular domains (ECDs) were used to predict epitopes.
Protein conservation in related nematodes
A BLAST search was performed on the UniProtKB database (https://www.uniprot.org/) to evaluate the possibility of cross-protection by the selected proteins used for the chimera design against related nematodes of human and veterinary importance. UniProtKB is the central resource for storing and interconnecting information from large and disparate sources, and the most comprehensive catalog of protein sequence and functional annotation (51). The degree of relatedness between the selected proteins and their homologs in Brugia malayi, Loa loa, Onchocerca flexuosa, Onchocerca ochengi, Toxocara canis, and Wuchereria bancrofti was investigated.
Cytotoxic T lymphocyte epitope prediction
Cytotoxic T lymphocyte (CTL) epitope prediction was done using the NetCTL 1.2 server (http://www.cbs.dtu.dk/services/NetCTL/). The server implements epitope prediction through a method that incorporates predictions of proteasomal cleavage, TAP (Transporter Associated with Antigen Processing) transport efficiency, and class I MHC affinity (52). Though the server allows for predictions of CTL epitopes restricted to 12 MHC class I supertypes, only the A2, A3, and B7 supertypes were used for this study. These were selected to achieve a 90% of predicted phenotypic frequency in the vaccinated population (53). For CTL epitope prediction on the NetCTL 1.2 server, MHC class I binding and proteasomal cleavage are executed using artificial neural networks (54) whereas TAP transport efficiency prediction is done using a weight matrix (55). CTL epitope prediction was set at the default server threshold value of 0.75.
Helper T lymphocyte epitope prediction
HTL epitopes (15-mer) for all human alleles were predicted using the NetMHCII 2.3 server (http://www.cbs.dtu.dk/services/NetMHCII/) which predicts binding affinities to molecules covering HLA-DR, HLA-DQ, and HLA-DP alleles using artificial neural networks (56). The input extracellular domain sequences were submitted for HTL epitope prediction with the default threshold % rank for strong binder (SB) and weak binder (WB) set to 2 and 10 respectively. HLA-DR, HLA-DQ, and HLA-DP alleles were predicted for all the ECDs, and only highly promiscuous epitopes capable of binding to several alleles and classified as strong binders were considered for designing the chimera.
B-cell epitope prediction
The BepiPred-2.0 webserver (http://www.cbs.dtu.dk/services/BepiPred/) was used for linear B-lymphocyte epitope prediction. The server is based on a random forest algorithm trained on epitopes annotated from antibody-antigen protein structures. This method outperformed other methods for sequence-based epitope prediction on a large number of linear epitopes obtained from the IEDB database as well as epitope data produced from solved 3D structures (57). Employing the standard server threshold of 0.5, linear B-epitopes of various lengths were selected for the next steps.
Antibody class prediction
The role of specific immunoglobulins, predominantly IgG has been reported in immune protection against Onchocerca spp. To assess the specific antibody classes elicited by the vaccine candidate, the predicted linear B-epitopes were subjected to the Antibody Class(es) Predictor for Epitopes (AbCPE) server (http://bioinfo.unipune.ac.in/AbCPE/Home.html). For the prediction of the antibody class(es) that an epitope can potentially bind to, the AbCPE server is a novel method based on a multi-label classification approach. The server predicts binding to IgG, IgE, IgA, and IgM with very promising results (58).
Epitope antigenicity prediction
To generate the final list of epitopes for the chimera design, all the predicted epitopes were then assessed for antigenicity using the VaxiJen v2.0 server (http://www.ddg-pharmfac.net/vaxijen/VaxiJen/VaxiJen.html). The server allows antigen classification entirely based on the physicochemical characteristics of proteins without resorting to sequence alignment. The method is also alignment-free and homology-independent. The Vaxijen v2.0 server functions through the autocross-covariance (ACC) transformation of protein sequences into uniform vectors of key amino acid properties to predict protective antigens for bacteria, fungi, parasites, viruses, and tumors (59). For this study, the parasite model with a default threshold of 0.5 was used for the evaluation and only those with antigenicity scores above 0.5 were selected to constitute the chimeric antigen. Figure 1 shows a summary of epitope prediction, selection steps and all other analyses conducted.
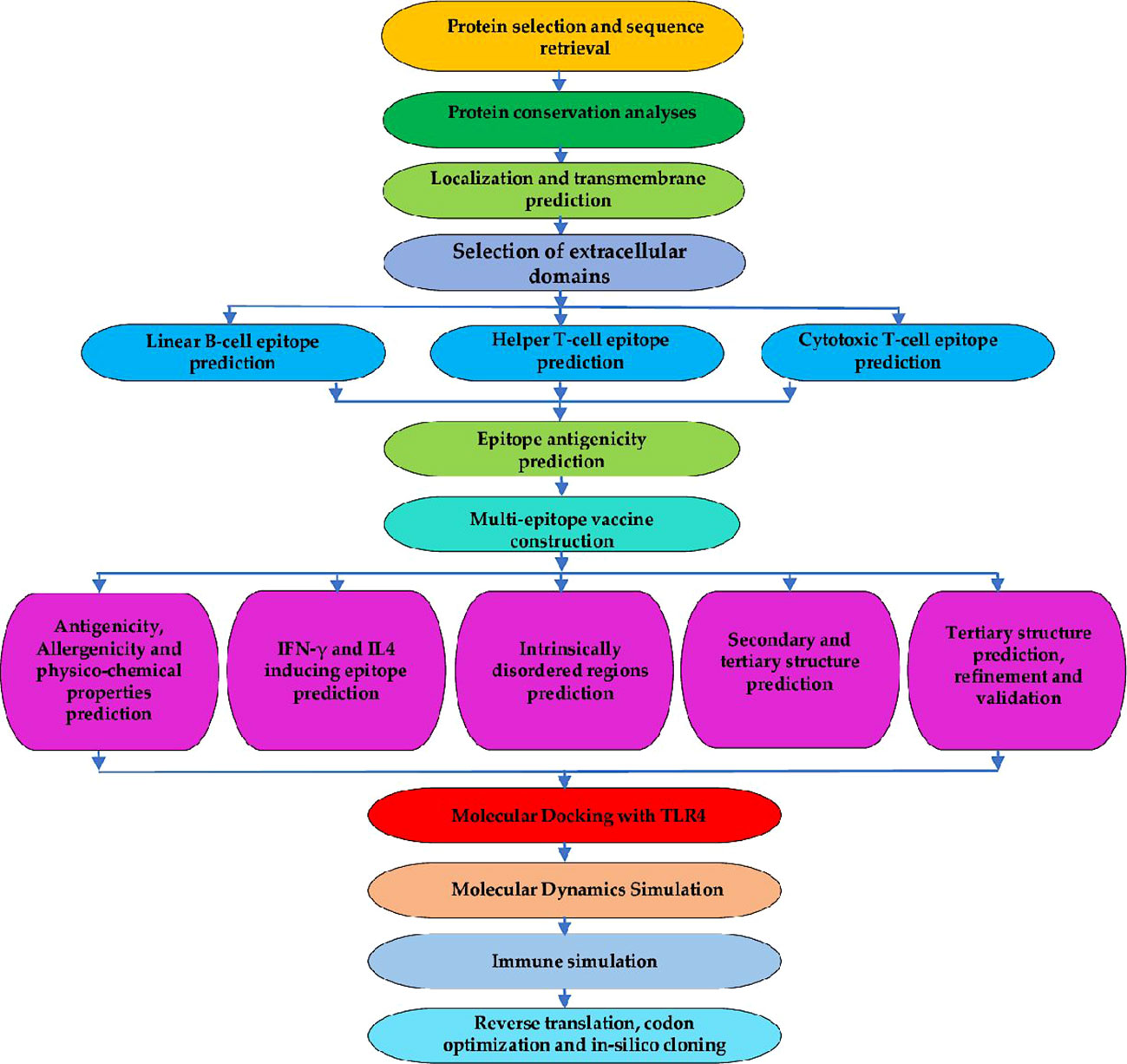
Figure 1 Flowchart for the designed study. The entire approach used in the study is comprised of several phases, which involve identifying the target protein and its conservation analysis. Epitope predictions from the chosen protein (CTL, HTL, and linear-B cell epitopes); vaccine construction, 3D modeling, refinement, and validation. Molecular Docking with immune cell receptor, molecular dynamics simulation, and in-silico immune simulation to check the potential of the vaccine to initiate an immune response. Lastly, reverse translation, codon optimization, and in-silico cloning.
Multi-epitope vaccine sequence construction
The high-scoring CTL epitopes, promiscuous high-affinity HTL epitopes, and high-scoring linear-B cell epitopes predicted to be antigenic were merged using AAY, GPGPG, and KK linkers respectively. The Mycobacterium tuberculosis Resuscitation-promoting factor E, RpfE (RPFE_MYCTU) (UniProt ID: O53177) was added to the N-terminal of the designed chimera to serve as a built-in adjuvant using the EAAAK linker. Adjuvants are known to enhance the extent, breadth, and robustness of the immune response (60). The vaccine construct was also designed to contain the pan HLA-DR epitope (PADRE) sequence, embedded in between the adjuvant and epitopes, and linked using the GGGS linker which is used to ensure flexibility and ensure effective separation of individual epitopes (61). The EAAAK linker used to incorporate the TLR4 adjuvant sequence has been used for bifunctional proteins to improve on protein expression levels and fusion protein bioactivity (62). The GPGPG linkers have been used to avert the creation of junctional epitopes in epitope-based vaccines – facilitating antigen processing and presentation (53). Similarly, the KK linkers were used to maintain the independent immune-reactivity of each epitope (61). Finally, the 8xHis-tag was added at the C-terminal of the chimera to aid in purification and identification procedures.
Physicochemical properties and solubility analysis
Following the chimera design, various physicochemical characteristics were assessed using the ProtParam webserver (https://web.expasy.org/protparam/). These characteristics include the molecular weight, theoretical pI, aliphatic index, extinction coefficient, and predicted half-lives for three model organisms (Escherichia coli, yeast, and mammal cells) as well as the instability index and amino acid and atomic composition (63). In addition, chimera solubility upon expression in bacteria was evaluated using the SoluProt 1.0 server (https://loschmidt.chemi.muni.cz/soluprot/). SoluProt was developed by a gradient-boosting machine technique using the TargetTrack database as a training set. The server had an accuracy of 58.5% and an AUC of 0.62 when evaluated against a balanced independent test set derived from the NESG database, exceeding the scores of a suite of alternative solubility prediction tools. Additionally, there is proof that it might substantially raise the success rate of experimental protein research (64). The SOLpro server (http://scratch.proteomics.ics.uci.edu) was also used to predict solubility. SolPro is an SVM-based tool for solubility prediction from protein sequences with a global accuracy of over 74% as estimated by tenfold cross-validation (65).
Secondary structure prediction
To predict the secondary of the designed chimeric antigens, two servers, PSIPRED (http://bioinf.cs.ucl.ac.uk/psipred/) and RaptorX Property (http://raptorx.uchicago.edu/StructurePropertyPred/predict/) were used. PSIPRED incorporates two feed-forward neural networks which perform an analysis of output obtained from PSI-BLAST (Position-Specific Iterated BLAST) for the accurate prediction of protein secondary structure. The PSIPRED server is reported to be capable of achieving an average Q3 score of 76.5% using a strict cross-validation approach to assess performance (66). The RaptorX Property web server on the other hand, predicts the secondary structure properties of a protein using a template-free approach. The RaptorX Property server can achieve 84% Q3 accuracy for 3-state SS, 72% Q8 accuracy for 8-state SS, 66% Q3 accuracy for 3-state solvent accessibility, and 0.89 area under the ROC curve (AUC) for disorder prediction, according to experimental results from CASP10, CASP11 and other benchmarks (67).
Antigenicity and allergenicity prediction for multi-epitope chimeric vaccine candidate
To evaluate the antigenicity of the designed multi-epitope chimeric antigen, the VaxiJen v2.0 (described above) and ANTIGENpro servers were used. The ANTIGENpro server is a sequence-based, alignment-free prediction tool trained using a large non-redundant dataset mainly obtained from protein microarray data analysis. The server achieved an estimated accuracy of 76% using the combined dataset in cross-validation experiments (68).
In addition, the multi-epitope antigen was submitted to AllerTop v.2.0 (https://www.ddg-pharmfac.net/AllerTOP/) and AllergenPF (https://ddg-pharmfac.net/AllergenFP/) servers to evaluate its potential to generate allergenic responses. The AllerTop v2.0 server operates based on the auto- and cross-covariance (ACC) transformation of the supplied protein sequences into uniform equal-length vectors. A training dataset containing 2427 known allergens from different species and 2427 non-allergens is used for protein classification (69). The AllergenFP server is a novel alignment-free descriptor-based fingerprint approach to classify proteins as allergens and non-allergens. The approach implements a four-step process for allergenicity prediction. The AllergenFP server was tested using a dataset of 2427 known allergens and 2427 non-allergens, and 88% of them were correctly identified with Matthews correlation coefficient of 0.759 (70).
IFN-γ and IL4 inducing epitope prediction
Interferon-gamma (IFN-γ), a key cytokine in the induction of adaptive and innate immune responses (71) has been reported to be an important cytokine in immune protection against onchocerciasis (45). The IFNepitope server (https://webs.iiitd.edu.in/raghava/ifnepitope/scan.php) was used to predict 15-mer IFN-γ epitopes for the designed chimeric antigen. The server constructs overlapped sequences from which the IFN-γ epitopes are predicted. A hybrid motif and support vector machine (SVM) approach was used for IFN-γ epitope prediction. A training dataset comprising of IFN-γ inducing and non-inducing MHC class-II binder, which can activate T-helper cells was employed in developing the server (72).
Given the critical role of Th2 responses in protection against helminths and other extracellular parasites (73), the IL4pred server (https://webs.iiitd.edu.in/raghava/il4pred/scan.php) was used to predict IL4-inducing MHCII binders in the designed chimera using the hybrid (SVM + Merci motif) based approach. The dataset used to develop the server comprised 742 non-inducing and 904 experimentally validated IL4 inducing MHC class II binders. The hybrid method of amino acid pairs and motif information for IL4-inducing peptides prediction yielded the best results with maximum accuracy of 75.76% and MCC of 0.51 (74).
Prediction of Epitopes for Mouse MHC II Alleles
MHC II binding peptides (15-mer) for mice alleles in the designed chimeric antigens were predicted using the NetMHCII 2.3 server (http://www.cbs.dtu.dk/services/NetMHCII/) which predicts binding affinities to molecules covering human (HLA-DR, HLA-DQ, and HLA-DP) and 7 mouse alleles using artificial neural networks. The functioning of the server has been described above.
Immune simulation for vaccine efficacy
The C-ImmSim server (https://kraken.iac.rm.cnr.it/C-IMMSIM/index.php) which performs in-silico immune simulations was used to further predict the immune response potentiated by the chimeric vaccine candidate. The C-ImmSim server is an agent-based model that predicts immunological interactions using machine learning methods and immune epitopes using a position-specific scoring matrix (PSSM). The server “simultaneously simulates three compartments that represent three separate anatomical regions found in mammals: (i) the bone marrow, where hematopoietic stem cells are simulated and produce new lymphoid and myeloid cells; (ii) the thymus, where naive T cells are selected to avoid autoimmunity; and (iii) a tertiary lymphatic organ, such as a lymph node” (75). To predict the immune response generated by the designed chimeric antigen, in accordance with the target product profile for a prophylactic vaccine against onchocerciasis as described (76), three injections were simulated four weeks apart (76). The default settings of simulation parameters were used, and the time steps were set to 1, 84, and 168 (each time step is 8 hours, and time step 1 is injection at time = 0). A total of 1000 simulation steps was used. In addition to the 3 injections given 4 weeks apart, 12 injections of the chimeric vaccine candidate were also given at four weeks intervals to mimic repetitive exposure to the antigen (since this is what obtains in a typical endemic setting) to investigate for possible clonal selection. The Simpson index, D (a measure of diversity) was interpreted from the plot.
Tertiary structure prediction
The Iterative Threading ASSEmbly Refinement (I-TASSER) server (https://zhanglab.ccmb.med.umich.edu/I-TASSER/) was used for three-dimensional structure prediction of the designed chimera by homology modelling. The I-TASSER server using the sequence-to-structure-to-function model for the automated prediction of protein structure and function. The server initially generates three-dimensional (3D) atomic models for the query sequence from multiple threading alignments and iterative structure assembly simulations using the amino acid sequence information (77). The top five full-length models, the confidence score, the predicted TM-score and RMSD, and the standard deviation of the estimations are all included in the I-TASSER server’s output for each query (78). I-TASSER has received the best ranking among protein structure prediction servers in the last eight community-wide CASP experiments (79).
Refinement and validation of the 3D modeled structure
A two-step refinement approach was employed on the predicted vaccine 3D structure from I-TASSER to improve structure quality, both locally and globally. The first step of refining the 3D structure was done using the ModRefiner server (https://zhanggroup.org/ModRefiner/) which is used to build and improve protein structures from Cα traces based on a two-step, atomic-level energy minimization. The Initially, the main-chain structures are constructed from initial Cα traces and the side-chain rotamers are then refined jointly with the backbone atoms using a complex physics and knowledge-based force field (80). This was followed by another round of refinement on the GalaxyRefine server (http://galaxy.seoklab.org/cgi-bin/submit.cgi?type=REFINE). The GalaxyRefine server works by first rebuilding side chains, performing side-chain repacking, and then relaxing the overall structure using a molecular dynamics simulation. This method showed the best performance in improving the local structure quality according to results from the CASP10 assessment (81).
The RAMPAGE, and ProSA-web servers were used for tertiary structure validation. The RAMPAGE server generates a Ramachandran plot, which enables visualization of the proportion of residues in favored, allowed, and disallowed regions, from the dihedral angles phi (ϕ) and psi (ψ) of the amino acids in the protein (82). The ProSA-web server (https://prosa.services.came.sbg.ac.at/prosa.php) offers an intuitive interface to the ProSA program and is regularly used in validating protein tertiary structures. For a specific input structure, ProSA determines an overall quality score and displays it alongside all other known protein structures in its database. A 3D molecular viewer also displays and highlights any faults with the structures. If the estimated score is outside the range that is typical of native proteins, there is high probability that the structure contains errors (83).
Discontinuous B-Cell epitope prediction
Up to 90% of B-cell epitopes in proteins are estimated conformational (84). After validating the 3D structure of the chimeric antigen, the ElliPro server (http://tools.iedb.org/ellipro/) was used for discontinuous B-cell epitope prediction. The server implements three algorithms that perform the following functions: (i) approximating the protein shape as an ellipsoid; (ii) calculating the protrusion index for each residue; and (iii) grouping adjacent residues based on their protrusion index values. The ElliPro server has been used to test a benchmark dataset of conformational epitopes deduced from 3D structures of antibody-protein complexes. When compared with six other structure-based epitope prediction approaches, ElliPro produced the best performance, giving an AUC value of 0.732, when the most significant prediction was taken into account for each protein (85).
Molecular docking of vaccine models to the TLR4-MD2 dimeric receptor
The vaccine model with and without the adjuvant were separately docked towards the experimentally solved binding pocket of the m-shaped TLR4-MD2 dimeric receptor assembly (PDB 3FXI) using the ‘Dock’ module implemented in MOE (version 2020, Chemical Computing Group, Canada) (86). After structure preparation for each procedure, we validated the implemented docking protocol before applying it to measure affinities between the vaccine constructs and the receptor dimer. However, docking validations of large molecular partners like protein-protein interactions, in this case, is usually quite tricky because many rotatable bonds are involved allowing a broad deviation of conformations different from the original co-crystalized ones. To increase confidence in our protocol nonetheless, we first re-docked severally a large co-crystalized lipopolysaccharide antigen (with 80 rotatable bonds, 6 lipid sidechains, and a molecular weight of 2965.13g/mol) towards its binding pocket localized to the ‘dimerization interface’ on the TLR4-MD2 dimeric receptor, shown to be involved in antigen recognition (87). Indeed, the protocol was able to discriminate between good and poor conformations as it correctly ranked poses with the least RMSDs (RMSD<20Å; RMSD refine < 1Å) at the top 5 positions. When we observed that the top poses have similar interactions found in the crystalized structure after manual inspection, we implemented the protocol to dock the vaccine constructs using the generalized-born volume integral/weighted surface area (GBVI/WSA) (88) scoring function. This function estimates the enthalpic contribution to the free energy of binding of a given ligand pose using the AMBER99 (89) forcefields, trained on 99 different experimentally solved protein-ligand complexes. Structural analyses and visualization were performed with the PyMOL Molecular Graphics System, Version 1.2r3pre, Schrödinger, LLC; and statistical analyses were carried out using Microsoft ™ Excel version 16.56 with p<0.05 considered statistically significant. Generated data are summarized in the result section.
Molecular dynamics simulation of the receptor-Vaccine candidate complex
To assess the stability of the docked TLR4 receptor-vaccine candidate complex, we performed a set of triplicate all-atom molecular dynamics simulations. All simulations were run on GROMACS 2019.3, using the CHARMM36 forcefield (90). A cubic simulation box was created with three spatial dimensions of periodic boundary conditions set to a minimum distance of 1.2 nm between the atoms of the complex and the X, Y, and Z axes. When the complex was in the box, energy minimization was performed in a hypothetical vacuum and the TIP3P water model along with 19 chloride ions added to solvate and maintain a balance in the system’s electrostatic charge. A second energy minimization was performed before pre-equilibrating the system via temperature coupling and pressure coupling. Heavy atoms were restrained to fixed positions while the solvent could freely diffuse during the pre-equilibration steps. The reference temperature during coupling was set to 300K and dispersions were fixed by EnerPres to account for the cut-off van der Waals scheme. The Parrinello–Rahman method was implemented for pressure control set at 1.0 bar and all bonds were constrained by the LINCS approach. The energy and speed of the simulations were written at a frequency of 100ps. Particle Mesh Ewald (PME) was used to approximate the long-range electrostatic interactions with a cut-off value for short-range interaction to occur defined at 1.0nm, and the short-range Van der Waals cut-off was also set to 1.0nm. Each of the three simulations was run for 100 ns after the equilibrations, generating 23.4 G of data in total.
MM-PBSA binding free energy calculations
The molecular mechanics-based Poisson–Boltzmann surface area (MM-PBSA) method was used to approximate the free energy of interaction between the vaccine construct and the TLR4 receptor protein (91, 92). Molecular mechanics was used to estimate the enthalpic contributions while the polar and non-polar components of the solvent effect on free energy were assessed by solving the Poisson–Boltzmann (PB) equation and related molecular surface area (SA) estimator. With guidance from a study published by Wang et al, we were able to estimate the average binding energy with deviations using a python script incorporated in the g_mmpbsa package (93). Briefly, MD simulation trajectories were intercepted from the RMSD curves of the candidate vaccine–TLR4 complex with the MM-PBSA calculations performed as described above, except for a minor modification which was made based on the rationale that, to the best of our knowledge, the g_mmpbsa is limited to only reading files generated by specific GROMACS versions. Therefore, we had to re-generate the binary input files (.tpr) using GROMACS version 5.1.2. (As opposed to GROMACS 2019.3 which was used for the original simulations) to run the MM-PBSA approximations with g_mmpbsa. The other files including the structural (.gro), topology (.top), and parameter (.mdp) files required for the re-generation process remained unmodified as used in the original MD simulations with GROMACS 2019.3.
Codon optimization and in-silico cloning
The E. coli (strain K12) was chosen as the suitable host for the multi-epitope vaccine candidate expression. Reverse translation and codon optimization were performed on the Java Codon Adaptation Tool (JCat) server (http://www.prodoric.de/JCat). JCat provides the option to adapt the codon usage of a gene of interest to that of any host organism and presents both the codon adaptation index (CAI) and percentage GC content (which can be used to estimate protein expression levels) in the output. The CAI score offers information on codon usage biases and for the K12 strain of E. coli, for genes with CAI values >0.5, a strong association between CAI and expression levels has been reported (94). On the other hand, low GC% has been reported to contribute to the poor expression of mycobacterial genes in E. coli. A GC content of 30–70% has been suggested for optimal expression (95). To clone the in E. coli The pET-30a (+) vector was selected to clone the codon-optimized gene sequence of the designed vaccine candidate with the Nde I and Xho I restriction sites introduced at the N and C-terminals respectively using SnapGene tool (https://www.snapgene.com/free-trial/) to ensure vaccine candidate expression.
Results
Protein retrieval and preliminary characterization
The sequences of 126 proteins that show 90-100% identity with homologous proteins in the related parasites, including A. simplex and B. malayi were selected from a dataset of O. volvulus proteins previously generated by Cotton et al. (50). The sequences for all the designated proteins were downloaded from the WormBase database and used for the identification of antigen candidates to be employed for epitope prediction. SignalP 5.0 predicted the presence of signal peptides in nine of the selected proteins. This result was matched with the consensus prediction on the TOPCONS server, though an additional three proteins were predicted to have signal peptides on the PrediSi server. For TM prediction, a total of twenty-one proteins were predicted by consensus using TOPCONS to have TM regions with the number of TM domains in the proteins ranging from one to fourteen. The TMHMM server on the other hand predicted twenty-six proteins to have TM domains. DeepLoc predicted eleven to be localized to the cell membrane. These eleven together with three other proteins predicted to have TM domains but localized in the ER or Golgi apparatus were selected as the final set of antigens for the analyses. Stage-specific expression data from the WormBase database predicted that three of the proteins were L3 larval-dominant antigens, while another three were predominantly expressed in the microfilariae. Six of the antigens were expressed predominantly in the L2 while the remaining 2 were adult-stage dominant antigens. Following the prediction of TM regions, the extracellular domains of the designated proteins were then used to predict linear B-cell and T-cell epitopes. The Mycobacterium tuberculosis Resuscitation-promoting factor E, RpfE (RPFE_MYCTU), O53177 which can act as a TLR4 agonist was also downloaded from the UniProt database to be used as an adjuvant (96).
Protein conservation in related nematodes
A high degree of conservation for all the selected proteins in related nematodes (ranging from 30.6–100%) was observed based on the BLAST search done on the UniProt database and the BLASTp search on NCBI. For 3 of the selected proteins (OVOC1259, OVOC6950 and OVOC6796), no homologues were found in at least one of the target nematodes (B. malayi, L. loa, O. flexuosa, O. ochengi, Toxocara canisand W. bancrofti). The highest level of conservation was observed within the Onchocerca genus, (O. flexuosa, O. ochengi) with three proteins showing 100% conservation in O. ochengi (Table 1).
Cytotoxic T lymphocyte epitope prediction
High-scoring CD8+ (9-mer) epitopes were predicted from all the EC domains of the designated proteins on with NetCTL 1.2 server. The server’s default parameters for epitope identification were used. From the predicted sequences, seven epitopes were chosen to be incorporated into the chimera either because of their antigenicity or their overlap with some of the linear B-cell epitopes predicted (Table 2).
Helper T lymphocyte epitope prediction
High-affinity MHC-II epitopes (based on IC50 scores) for the HLA-DR, HLA-DQ, and HLA-DP human alleles, predicted on the NetMHCII 2.3 web server, were selected as HTL epitopes. In total, nine high-affinity HTL epitopes were chosen to design the chimeric vaccine candidate. It was observed that some of the predicted linear B-cell epitopes and the HTL epitopes had overlapping regions (Table 2).
B-cell epitopes prediction
Linear B-cell epitopes of varying residue lengths were predicted using different servers, but recurrent epitopes simultaneously predicted with BepiPred 2.0, and one other epitope prediction server (BCpreds or ABCpred) were selected for the final vaccine peptide. Some of the sequences predicted to be linear B-cell epitopes had regions of overlap with predicted T-cell epitopes and were used in to generate the chimeric antigen. A total of 10 linear B-epitopes were selected (Table 2).
Antibody class prediction
All the selected linear B-lymphocyte epitopes initially predicted to be antigenic and incorporated in the designed chimeric antigen were subjected to the antibody class prediction using the AbCPE server. All the epitopes were predicted to bind to IgG only. No epitope was predicted to bind to IgA, IgE or IgM (Table 3).
Construction of multi-epitope vaccine sequence
The chimeric vaccine candidate was designed from a total number 10 linear B-lymphocyte epitopes, 7 CTL epitopes, and 9 HTL epitopes. Epitopes with overlapping amino acids were merged to form contiguous sequences (Table 1. The chimera was designed by using KK, AAY and GPGPG linkers to merge the linear B-cell epitopes CD8+ T-epitopes and CD4+ T-epitopes respectively. Also, the TLR4 (PDB ID: 4G8A) agonist, RpfE from Mycobacterium tuberculosis, (RPFE_MYCTU) (accession number O53177) was chosen as a built-in adjuvant and incorporated at the N-terminal of the designed vaccine candidate using the EAAAK linker to boost immune responses elicited by the antigen. In addition, the universal Pan DR Epitope (PADRE), a potent stimulator of CD4+ responses was added after the M. tuberculosis RpfE TLR4 agonist sequence. The 8xHis tag was incorporated at the C-terminus of the antigen to aid in purification and identification procedures. At the end of the design, the chimeric vaccine candidate comprised of 742 amino acid residues that included 26 O. volvulus peptides (predicted epitopes), the immune potentiators (TLR4 agonist and PADRE) added, and different linkers (Figure 2).
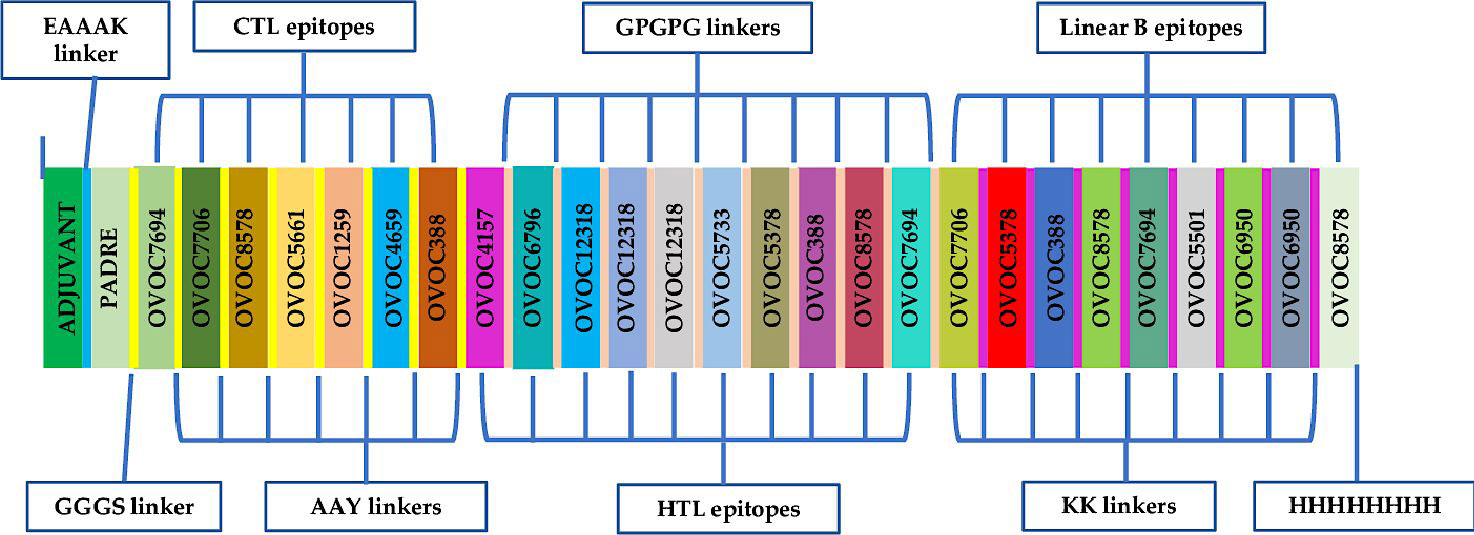
Figure 2 Schematic presentation of the final multi-epitope vaccine antigen, OvTMMEV-1. The 742-amino acid long polypeptide sequence containing a built-in adjuvant (green) at the N-terminal linked with the multi-epitope sequence through an EAAAK linker (cyan) to the PADRE sequence. Linear B epitopes are linked with KK linkers, HTL epitopes are linked using GPGPG linkers while the CTL epitopes are linked with the help of AAY linkers. An 8x-His tag is added at the C-terminal to aid in purification and identification.
Physicochemical properties and solubility analysis
The generated chimera was predicted to have a molecular weight (MW) of 77.7 kDa, while the theoretical pI was9.63, showing that the protein is basic in nature. The predicted half-life of the protein was 30 hours, >20 hours, and >10 hours in mammalian reticulocytes in vitro, yeast and E. coli in vivo respectively. The solubility score on the SoluProt 1.0 server was 0.719 (above the 0.5 threshold) and 0.855793 on the SOLpro server, predicting the protein to be soluble upon expression in bacteria. The instability index (II) was predicted to be 34.24. This II score classifies the protein as stable since an II of > 40 indicates instability). The GRand AVerage of hydropathicitY (GRAVY) was predicted to be −0.395, indicating that the protein can interact with water molecules (97). The aliphatic index, predicted to be 73.83, suggesting that the protein exhibits thermostability (98).
Secondary structure prediction
The final chimeric protein was predicted to be composed of 24% alpha helix, 16% beta-strand, and 60% coil (Figure 3A). Regarding solvent accessibility, 50% of amino acid residues were predicted as being exposed, 18% medium-exposed, and 30% were predicted as being be buried. A total of 141 amino acid residues (19%) were also predicted to be located in disordered regions by the RaptorX Property server (Figure 3B). Figure 3C shows the pictorial representation of secondary structure by color of the designed vaccine candidate.
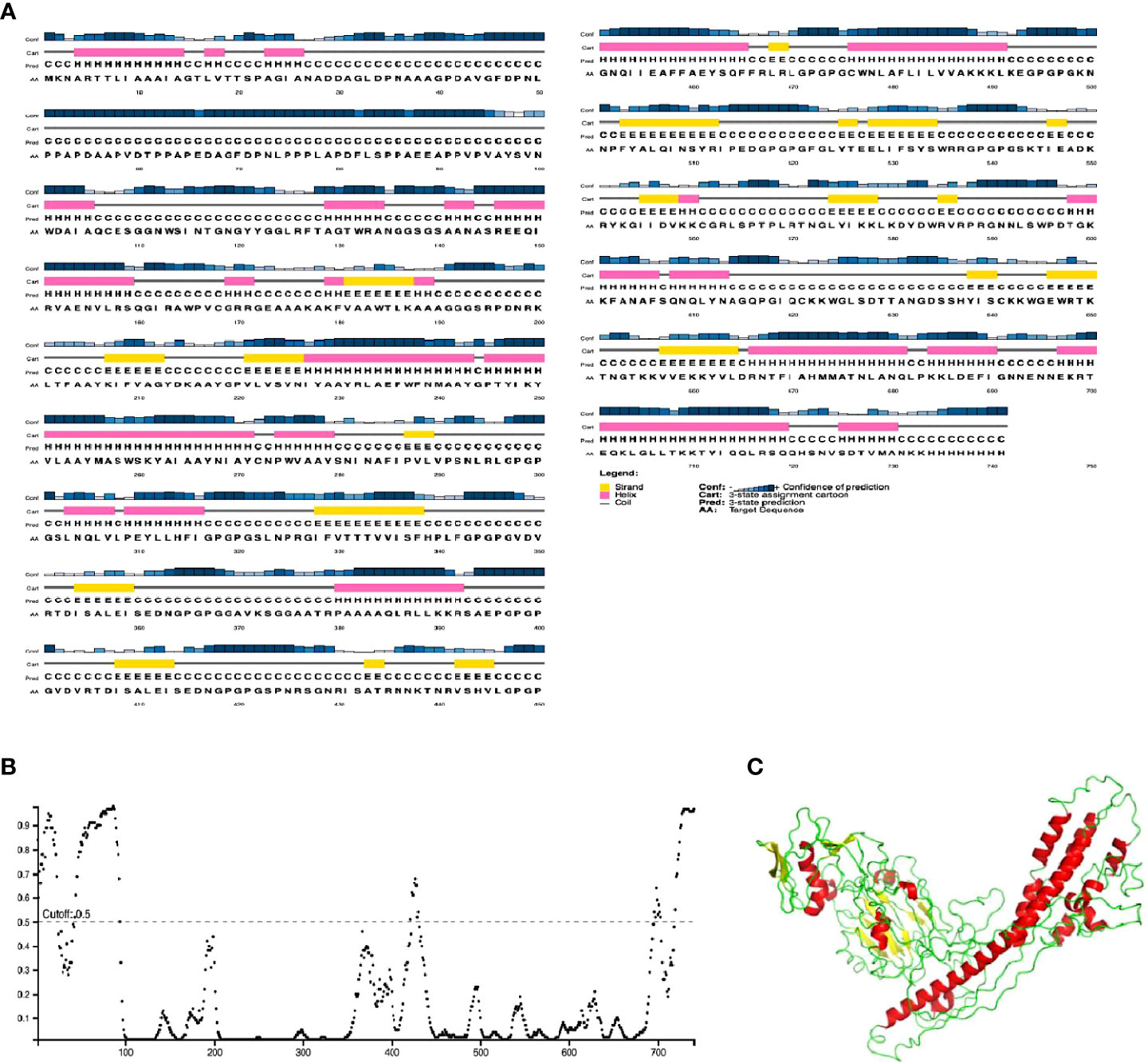
Figure 3 Graphical representation of secondary structure features of the final subunit vaccine sequence. (A) The protein is predicted to comprise alpha-helices (28.0%), beta strands (7.0%) and coils (65.0%), and (B) 20% of positions are predicted to be in intrinsically disordered regions as predicted by DISOPRED3. (C). The vaccine candidate 3D structure showing the different secondary structure elements with the helices (red), coils (green) and strands (yellow).
Antigenicity and allergenicity prediction
The predicted antigenicity score of the final sequence on the VaxiJen 2.0 server was 0.7580 (bacteria model with a threshold of 0.4) and 0.5913 (parasitic model with a default threshold of 0.5). The antigenicity score on the ANTIGENpro server was 0.877819. The results indicate that the generated protein is antigenic in nature. The AllerTOP v.2 and AllergenFP servers predicted the protein to be non-allergenic.
IFN-γ and IL4 inducing epitope prediction
Of the 164 potential IFN-γ inducing epitopes (15-mer) predicted for the adjuvant, twenty-five scored above the threshold for epitope prediction. Meanwhile, for the main vaccine sequence of the 572 potential epitopes predicted, a total of 187 IFN-γ inducing epitopes scored above the default threshold. The large number of IFN-inducing epitopes predicted was correlated with the simulated IFN-γ induction levels after injection with the chimeric vaccine following immune simulation on the C-ImmSim server (Figure 4). Similarly, for the IL4pred server, the same number of potential epitopes was predicted for the adjuvant and main vaccine sequence. From the number of potential epitopes predicted, a total of 53 and 227 IL4-inducing epitopes scored above the default threshold for adjuvant and main vaccine candidates, respectively.
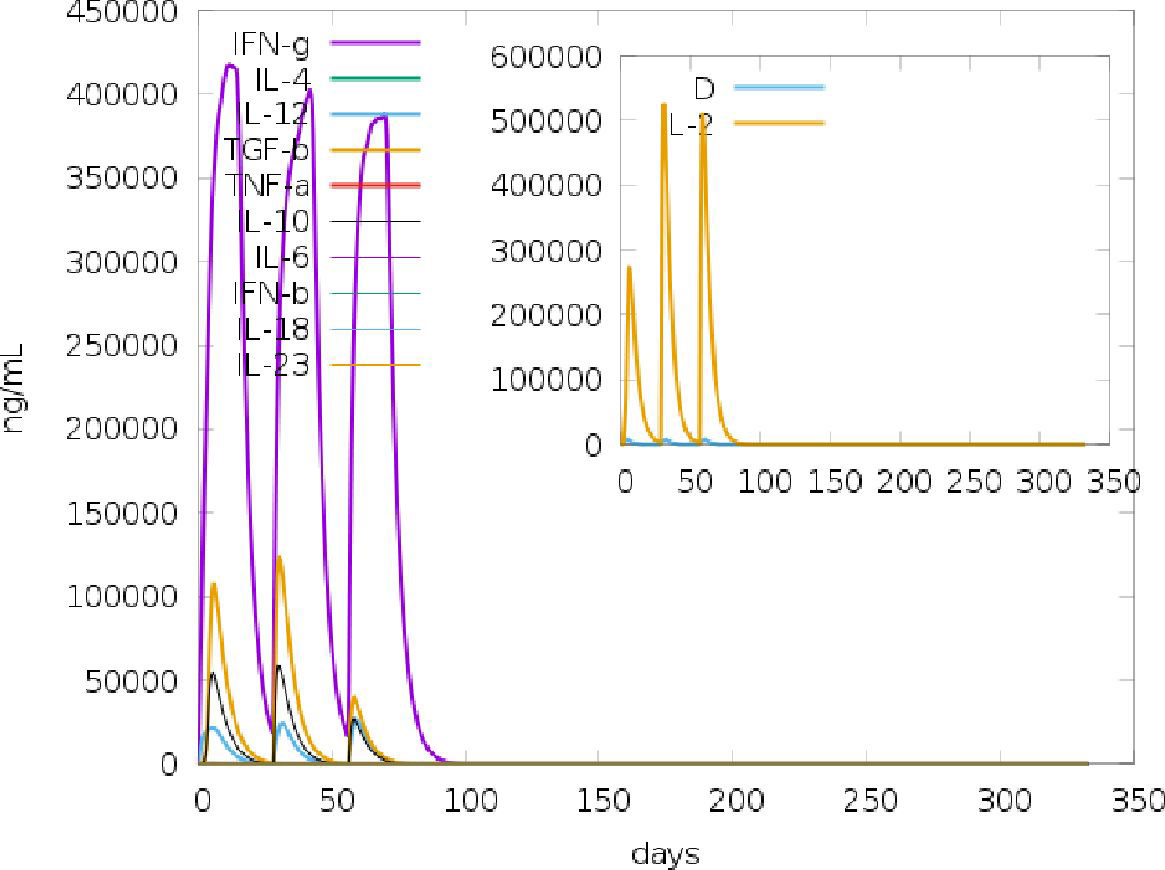
Figure 4 C-ImmSim simulation of the cytokine profile induced by three injections of the vaccine candidate injected 4 weeks apart. The main plot shows cytokine levels after the injections. The insert plot shows IL-2 level with the Simpson index; D indicated by the dotted line. D is a measure of diversity. Increase in D over time indicates emergence of different epitope-specific dominant clones of T-cells. The smaller the D value, the lower the diversity.
Prediction of epitopes for mouse MHC II alleles
A total of 138 peptides capable of binding to seven mouse MHC II alleles were predicted for the designed chimeric antigen with the NetMHC II 2.3 server with the H-2-IAb allele having the highest number of epitopes and the H-2-IEk having the least (Table 4).
Tertiary structure prediction
Five tertiary structure models for the designed chimeric vaccine candidate were predicted by the I-TASSER server based on 10 threading templates (the best of which were 7qfpA, 4ow1A, 6n23, 3eo5, and 6n7pX). All the selected templates exhibited good alignment based on the Z-score values observed (ranging from 0.92 to 4.03). The C-score values for the predicted 3D models ranged from −4.39 to −0.89. Typically, the C-score ranges from -5 to 2, with higher numbers suggesting greater confidence. The 3D model having the highest C-score was chosen for additional refinement (Figure 5A). The selected model had an estimated RMSD and TM-score of 11.7 ± 4.5 Å and 0.54 ± 0.15 respectively. of with an. The use of TM-scores has been proposed as an alternative tool to assess the structural similarity between two structures since it circumvents the challenges encountered using RMSD which is affected by local error (99). Generally, a model with correct topology will have a TM-score >0.5 while a TM-score less than 0.17 indicates random similarity. The indicated cut-off values are not dependent on the length of the protein sequence.
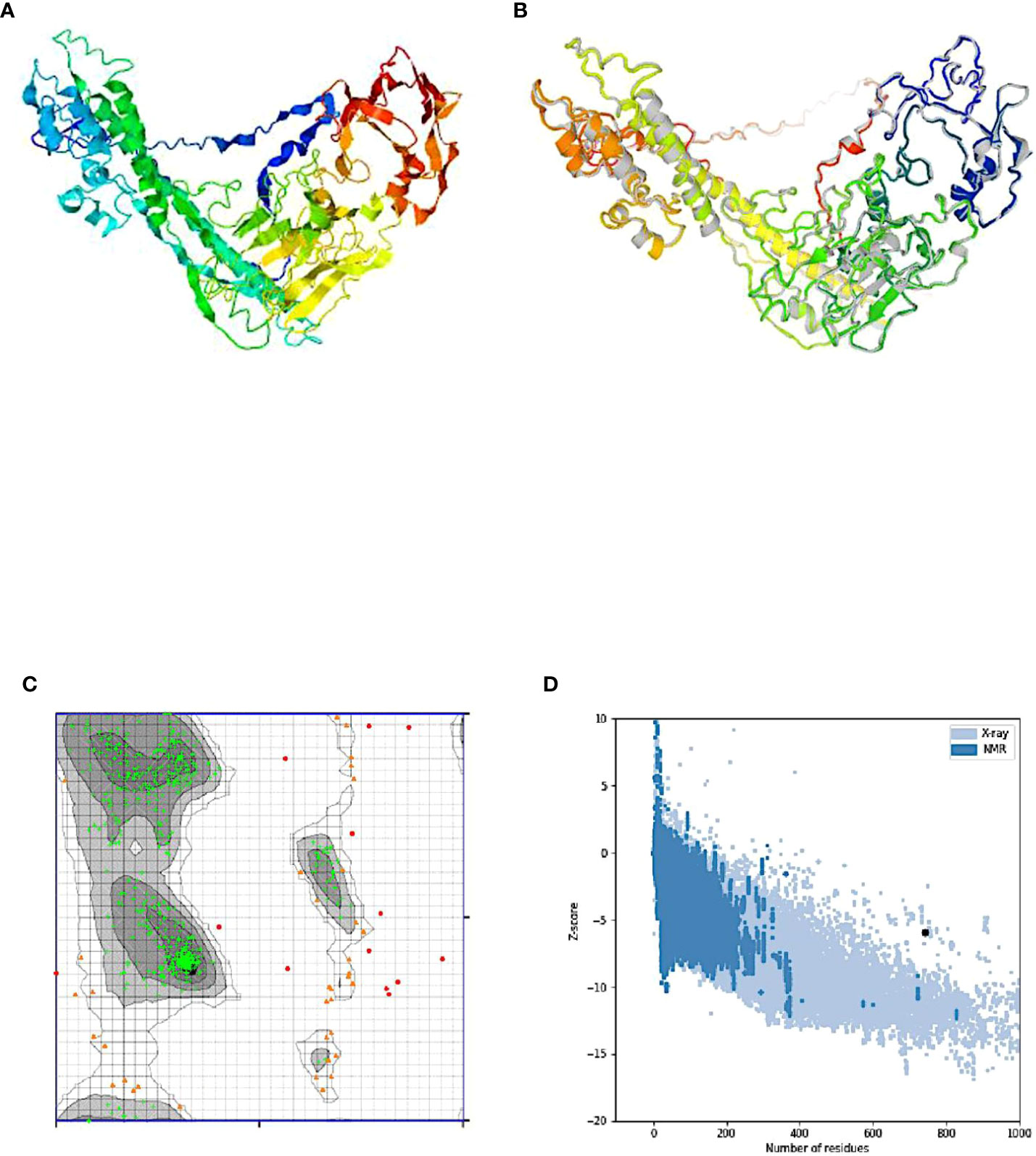
Figure 5 Protein modeling, refinement, and validation. (A) The final 3D model of the multi-epitope vaccine obtained after homology modeling on the I-TASSER server. (B) Refinement: superimposition of the refined 3D structure (colored) on the ‘crude model’ (gray) by the GalaxyRefine server. Validation of the refined model showing (C) Ramachandran plot analysis with 92.8% (green crosses), 5.3% (orange triangles), and 1.9% (red circles) of protein residues in favored, allowed, and disallowed (outlier) regions respectively, and (D) ProSA-web, giving a Z-score of -5.90.
Refinement and validation of modeled tertiary structure
Refinement of the designed vaccine candidate 3D structure generated initially on the ModRefiner server followed by the GalaxyRefine server yielded 5 models. From the quality scores model of the refined models, “model 1” (with parameters GDT-HA (0.9045), RMSD (0.528), and MolProbity (2.231) was selected as the final 3D model for further characterization (Figure 5B). In addition, the model had a clash score of 14.4, a poor rotamers score of 0.2, and a Ramachandran plot score of 89.3%. The Ramachandran plot analysis of the selected model protein predicted 87.5% of residues to be located in favored regions. This percentage is slightly lower than the score obtained from the GalaxyRefine analysis. In addition, 8.6% of e residues were predicted to be located in allowed regions while only 3.9.0% were predicted in disallowed regions (Figure 5C). Following refinement, the 3D model quality was tested on the ProSA-web and ERRAT servers. The selected model had a Z-score of −5.90 on the ProSA-webserver (Figure 5D) and an overall quality factor of 69.174 on the ERRAT server (Figure not shown). The ProSA-web score was outside the typical score range for native proteins of similar size.
Discontinuous B-cell epitope prediction
A total of 403 residues (54.3%) were predicted by the ElliPro server to be found in seven conformational B-cell epitopes, with epitope prediction scores in the range 0.501 to 0.871. The predicted discontinuous epitopes residues ranged from 3 to 150 amino acid residues (Table 5).
Protein-protein docking between designed vaccine constructs and TLR4-MD2 receptor dimer
An appropriate immune response relies on well-coordinated recognition and interaction between antigenic determinants and specific immune receptors. Several accounts associate toll-like receptor-4 (TLR-4), in particular, to protective immunity against the larval stages of Onchocerca (49, 100, 101). Therefore, a set of protein-protein docking calculations was executed to approximate the binding affinity between TLR-4 receptor in its bioactive confirmation (the m-shape dimer) and our vaccine constructs (when linked or not) to the adjuvant, resuscitation-promoting factor (Figures 6B, C) using the generalized-born volume integral/weighted surface area (GBVI/WSA ΔG) scoring function. This forcefield-based function estimates the free energy of binding of given ligand pose using the AMBER994 forcefields, trained on 99 different experimentally solved protein-ligand complexes. Keeping the receptor (TLR-4) tethered, for each construct serving as ligand, 10000 pre-placement conformations were generated and filtered to 1000 placement poses upon energy minimization to retain the top 100 low-energy poses (Supplementary Figure 1).
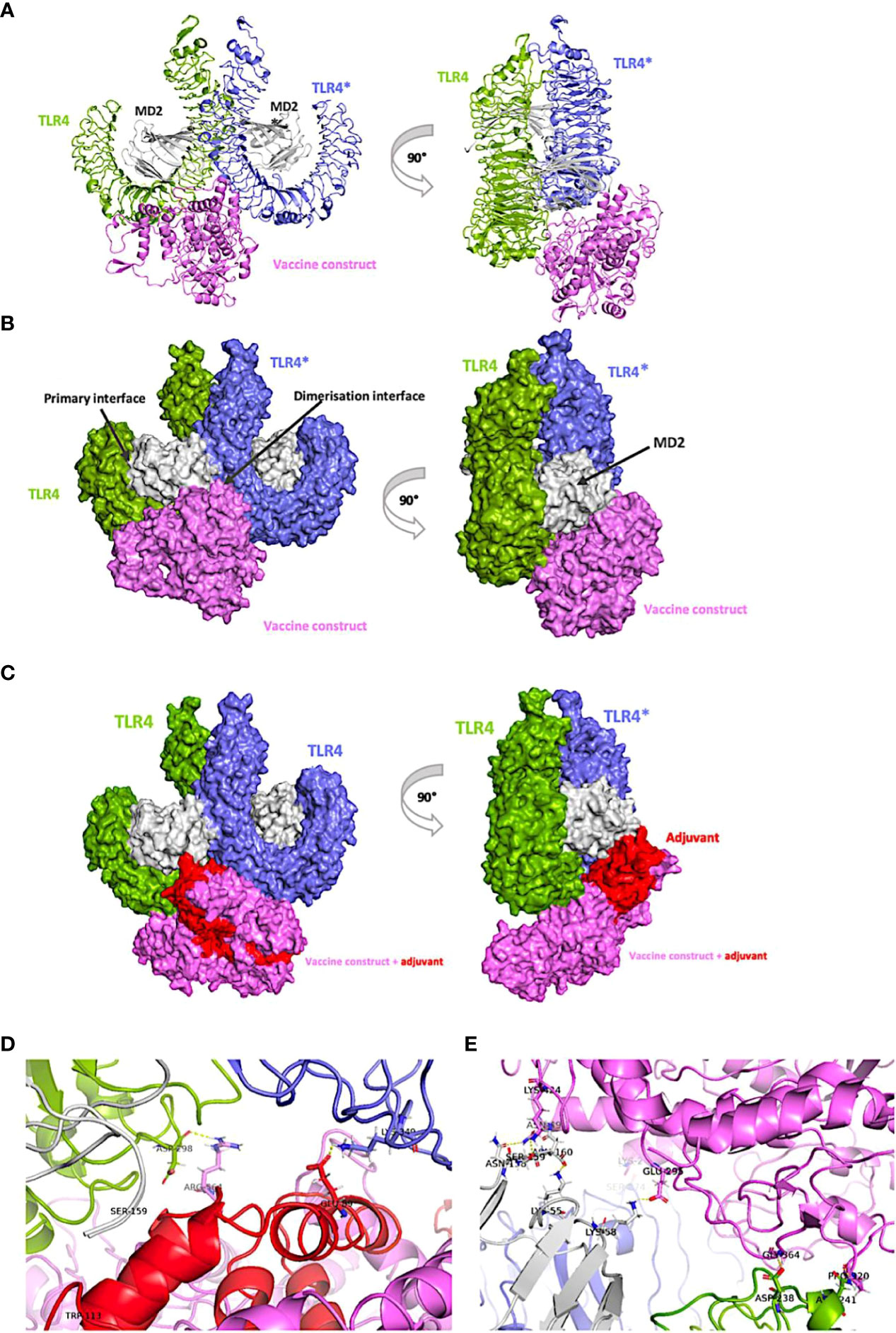
Figure 6 Vaccine model interacting with the ‘m-shaped’ MD2-TLR4 receptor dimer. In all panels, the vaccine is coloured violet and the adjuvant in red, the proximal TLR4 chain is coloured green while the distal one to the vaccine is in blue, and both MD-2 chains are coloured grey. (A) view of the carton representation of the non-adjuvanted construct bound to the receptor and rotated by 90° on the y-axis. (B) Surface representation of the non-adjuvanted construct bound to the receptor and rotated by 90° on the y-axis. (C) View of the surface representation of the adjuvanted construct (in red) bound to the receptor and rotated by 90° on the y-axis. (D) view of the representative binding mode of adjuvanted vaccine-receptor complex showing key interactions and (E) view of the representative model of the non-adjuvanted construct binding mode with key interacting residues.
Antigenic initiation of an ideal immune response only commences after TLR-4 binds myeloid differentiation factor 2 (MD-2) via extracellular domains (87, 102). When the TLR4-MD2 complex is formed, physiological recognition of the antigen occurs (87). This initiates dimerization to an ‘m-shaped’ multimer receptor complexed with the antigen in a symmetrically arranged TLR4-MD2-antigen fashion (Figures 6A-C), ultimately leading to downstream activation of the intracellular components of the response cascade. Therefore, the m-shaped receptor assembly (PDB 3FXI) was used to estimate binding affinities to our constructs (Figure 6, Supplementary Table 1) and browsed the top 30 docked poses, to identify binding modes fitting the interaction profile of the large co-crystalized lipopolysaccharide antigen used to validate the docking protocol of this study. This reference mode of binding makes key interactions with the dimerization interface of the TLR4-MD2 receptor assembly as previously reported (103). Specifically, we retrieved six poses in which either adjuvanted or non-adjuvanted structures meet these criteria, either in terms of orientation towards the pocket or precise interaction types made.
Structural analyses of representative structures (Figures 6D, E) indicate that more polar interactions are made in the non-adjuvanted compared to the adjuvant bound structure. Key contacts in the former case between either TLR4 or TLR4* chains of the receptor and the construct include Asp238, Asn241, Ser374 forming hydrogen bonds with Gly364, Pro320, and Lys2, respectively; while contacts between the proximal MD-2 and the vaccine construct include: Lys58-Glu295, Ser159-Lys424, and Asn158-Asn49 (Figure 6E). While these interactions are generally supported as the residues involved occur in the experimentally defined pocket of the receptor, Lys58 of the proximal MD-2 chain in particular which forms a hydrogen bond with Glu295 of the non-adjuvanted model, is shown to be a key interacting residue involved in antigen recognition by TLR4-MD2 complex (87). Statistical analyses of energies and deviations of the 6 poses (Supplementary Table 1) support these findings as the non-adjuvanted specie (Energy: -73.7 ± 4.9 Kcal/mol; RMSD refine: 1.1 ± 0.7 Å) has a slightly higher binding affinity matching the higher number of contacts made (Figure 6E) when compared to the TLR4–adjuvanted (Energy: -70.3 ± 2.3 Kcal/mol; RMSD refine: 0.6 ± 0.2 Å) cluster, the difference is however not significant (p=0.08). Nonetheless, both models show high affinities to the TLR4-MD2 receptor as evidenced by the very small binding energy values. These inspired further analyses of resulting binding modes by molecular dynamics simulations, to get an idea of the binding stability of the complexes.
Molecular dynamic analysis of candidate vaccine-TLR4 complex
Molecular dynamics simulation and binding free energy calculations were done to further characterize the stability and interactions between the candidate vaccine and TLR4 receptor. This was done on the non-adjuvanted vaccine in complex with the TLR4 receptor, as the docking analyses showed no advantage in terms of energy of the adjuvanted construct over the non-adjuvanted one (Supplementary Table 1). The MM-PBSA method was used to calculate the free energy of the system. To assess the structural stability of the complex, the RSMD values of the vaccine construct in the free state (unbound ligand) and when bound to the receptor (complex) (104) were compared. The backbone C-alpha atoms were used for the RMSD calculations and the data can inform whether the binding mode of the vaccine to the TLR4 receptor is stable and if the receptor has undergone conformational changes as a result of action or recognition of the vaccine (105). The overall RMSD fluctuations for the complex unbound ligand only, and TLR4 receptor only roughly remained balanced from 15 to 100ns (Figure 7A), indicating that the interaction between the vaccine ligand and the TLR4 receptor is quite stable. However, there are significant variations in fluctuation across the replicates (runs 1–3). The mean RMSD of the three runs ranged from 0.6 - 0.75 nm for the ligand candidate vaccine, 0.2 - 0.4nm for the TLR4 receptor protein, and 0.9 – 1.5 nm for the vaccine-TLR4 complex. The very low RMSD (0.2 - 0.4nm) values of the candidate vaccine are indicative of its stability (106).
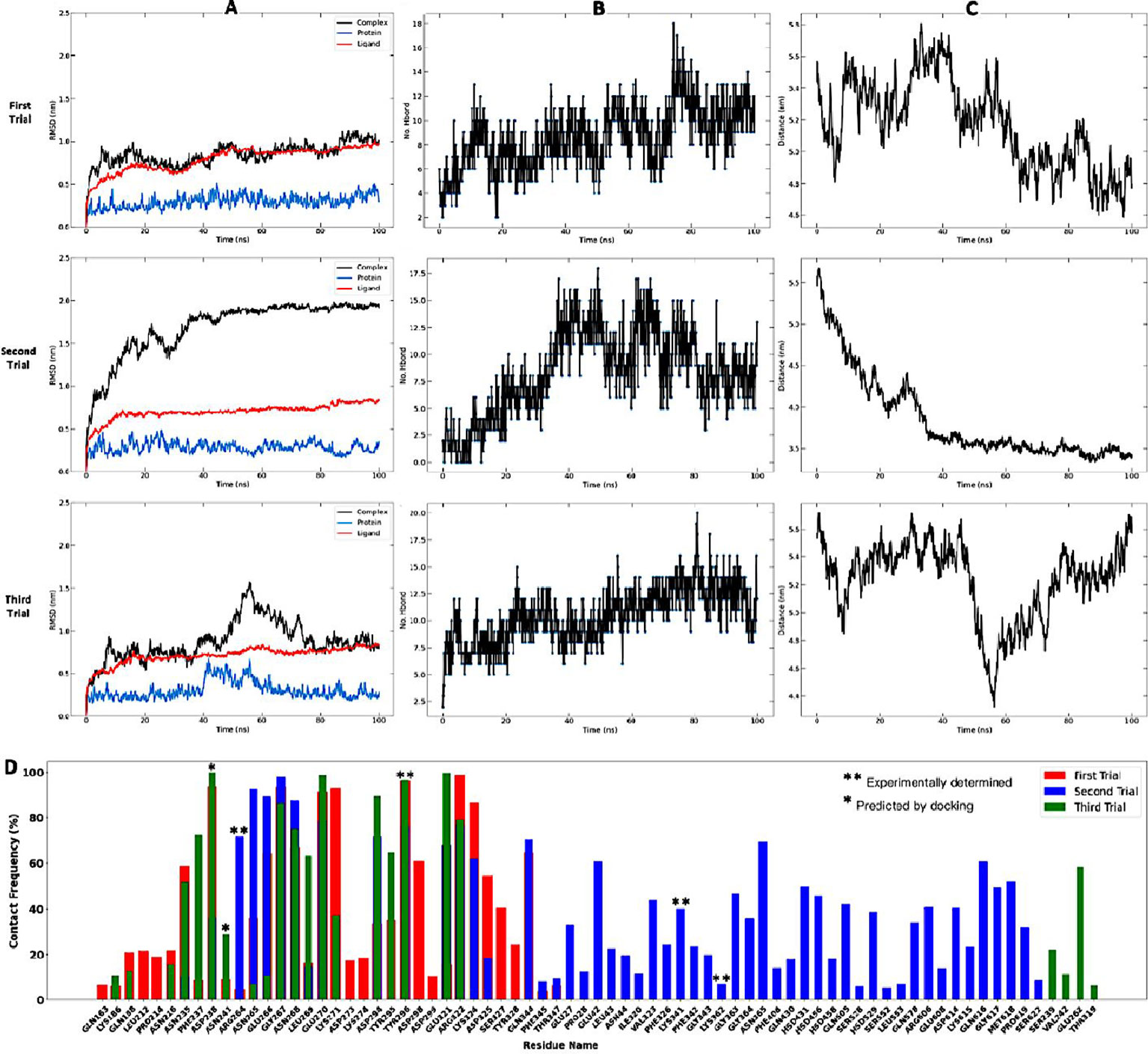
Figure 7 Molecular dynamics approximations of stability and strength of interaction between vaccine construct and TLR4 receptor complex. Simulations were performed in triplicates. (A) illustrates the RMSD fluctuations of the system with the vaccine construct represented as the ligand, TLR4 receptor as protein, and vaccine–TLR4 as complex. (B) shows the number of hydrogen bonds between the complex partners over time. (C) shows the mean distance between the complex partners over time, and (D) illustrates the number of contacts made by key residues involved signalling and recognition as pre-determined experimentally by X-ray crystallography.
The key parameter for evaluating binding affinity between molecules is the hydrogen bonding profile (93) as the amount and rate of change of hydrogen bonding patterns over time reflects the strength and stability of binding. The mean number of hydrogen bonds ranged from 8 – 10 across the three simulations and gradually increased over time from an average value of 6 at 20nm to about 8 at 100ns (Figure 7B). Except for run 3, the distance (Figure 7C) between the ligand construct and the receptor protein gradually decreased with time – an observation supported by the increasing number of hydrogen bonds over time, which reflects increasing affinity between the molecules in the complex. The bonding patterns were also very consistent with time and indicative of stable interactions between the construct and the receptor protein complex (93).
Binding free energy calculation using the MM-GBSA approach
The most popular approach to computationally estimate the binding free energy of a system is molecular mechanics-based tools like the MM-GBSA and MM-PBSA (92). In this study, we performed a binding free energy analysis using the MM-GBSA method to further appreciate the binding affinity of the candidate vaccine to the TLR4 receptor. To this effect, we extracted from the 100ns MD simulation trajectories a total of 11 frames which represent 11 conformations of the candidate vaccine-TLR4 receptor complex over time, to calculate the free binding energy. The results show that the vaccine candidate has a strong predicted mean binding affinity (-18,369.1 +/- 1,326.5 KJ/mol) for the TLR4 receptor (Table 6). This value is extremely high when compared to binding energies of similar studies involving the use of MM-GBSA to predict protein-protein interactions (107, 108). Nonetheless, this is expected as our system comprising of two relatively bigger proteins (the vaccine candidate protein and TLR4 receptor protein) is roughly x10 times larger than the proteins used in the cited studies for similar MM-GBSA calculations.
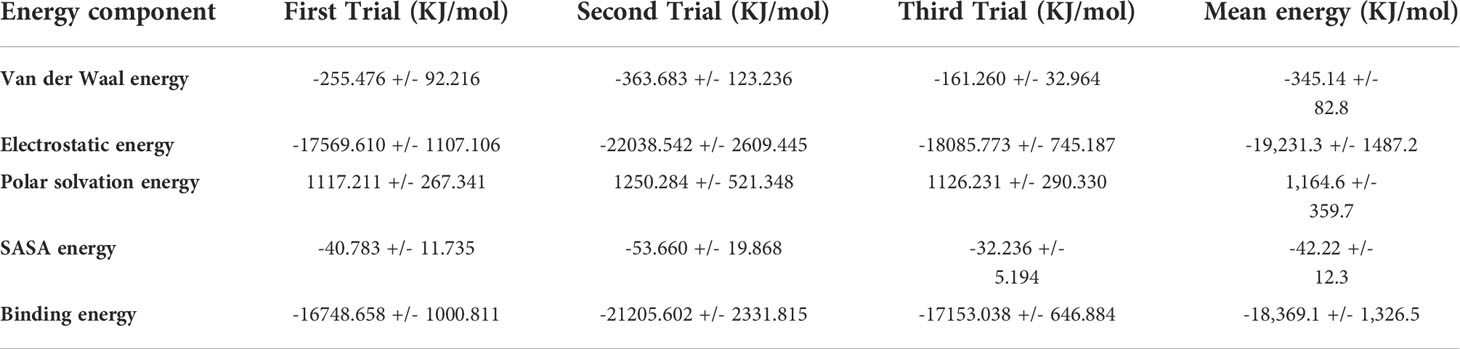
Table 6 MM-GBSA summary of the energetic components underpinning interaction between the candidate vaccine and the TLR4 receptor complex.
Immune simulation for vaccine immunogenicity
Immune simulation analyses projected an increase in the secondary responses that were elicited. In theory, the observed pattern depicts the active development of an immunological response (Figure 7A). The simulated response to the first injection was characterized by elevated IgM levels Meanwhile, significant rises in the population of B-cells, IgG1 and IgG2 subclass antibody levels, IgM, total IgG + IgM antibodies, and a corresponding decline in the antigen concentration were observed for the simulated secondary and tertiary responses (Figures 8A, B). The observation supposes the development of immunological memory, which is observed in the enhanced memory B-cell population (Figure 8C), as well as isotype switching, leading to rapid antigen clearance with successive exposures to the multiepitope chimera. The TH (helper) and TC (cytotoxic) cell populations were predicted to respond more strongly and exhibited comparable memory development after several encounters with the antigen (Figures 8D–F). Furthermore, during the immunization period, high levels of dendritic cell, macrophage, and NK cell populations were stimulated and sustained (Figures 8G–I). Recurrent exposure with 12 injections (given at 4-week intervals) showed dropping IgM levels and rising IgG levels, whereas TH cell populations and IFN- levels remained high throughout the exposure. As a result, it appeared that the designed antigen provoked both cellular and humoral immunological responses. Both types of immune response have been reported to be essential for preventing onchocerciasis. Additionally, the low Simpson D index (Figure 4), which showed a high level of T-cell response diversity, supports the existence and efficiency of different epitopes present in the chimera.
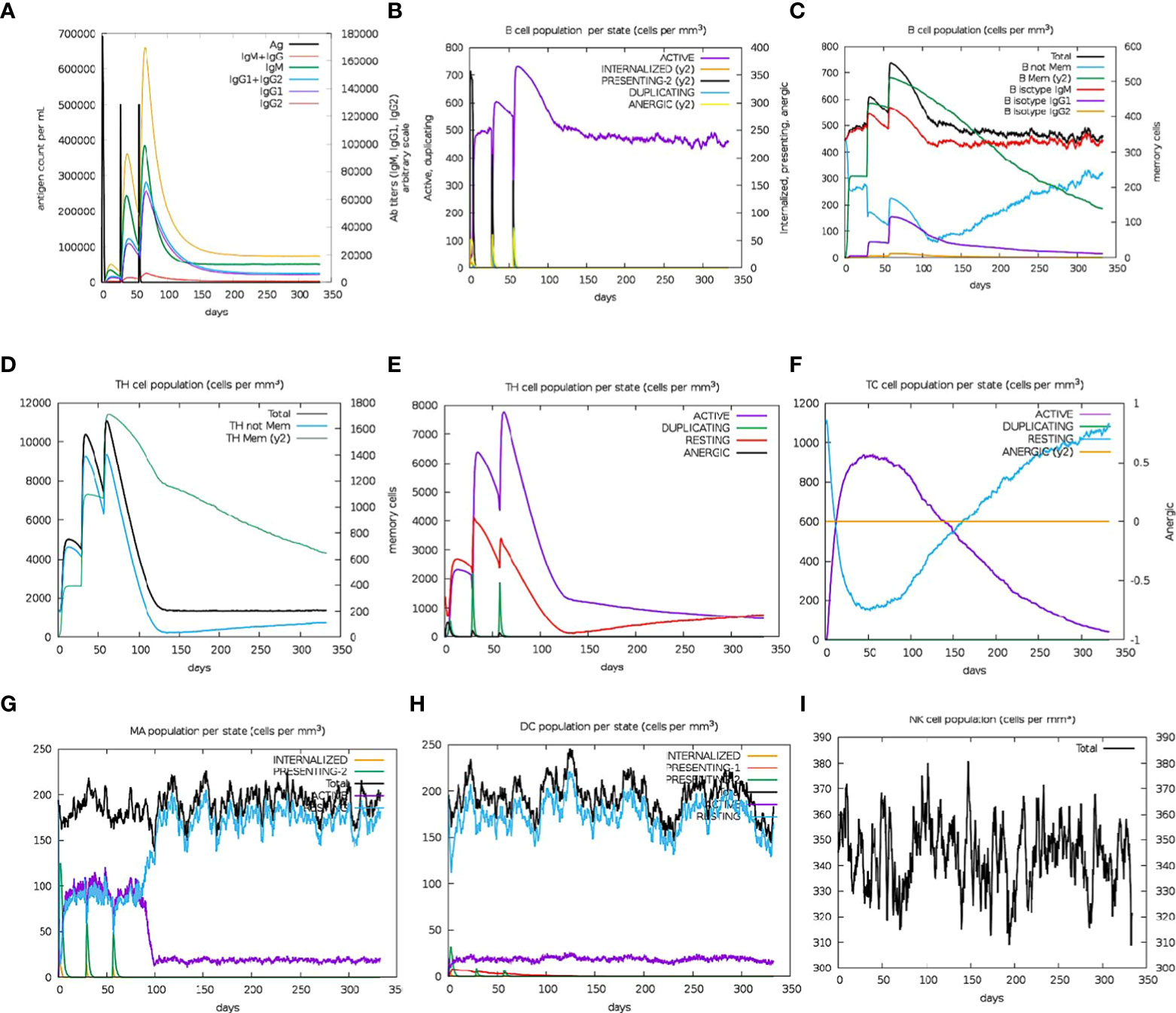
Figure 8 In silico simulation of immune response using the vaccine candidate as antigen: (A) Antigen and immunoglobulins, (B) B cell population per state, (C) B cell population, (D) TH cell population, (E) TH cell population per state, (F) TC cell population per state, (G) MA population per state, (H) DC population per state, and (I) NK cell population.
Codon optimization and in-silico cloning
The gene coding for the designed vaccine candidate was codon-optimized using the Java Codon Adaptation Tool (JCat) to guarantee high protein expression levels in E. coli. The gene sequence contained 2,226 nucleotides in total. In addition, the sequence had Codon Adaptation Index (CAI) of 1.0, and the mean GC content was 53.9%. This suggests the prospect of suitable expression of the designed vaccine candidate in the E. coli host. A GC content range between 30% and 70% is desired for optimal protein expression. Lastly, the adapted codon sequence was inserted in the appropriate sites of the pET30a (+) vector using SnapGene software to generate a recombinant plasmid in-silico (Figure 9).
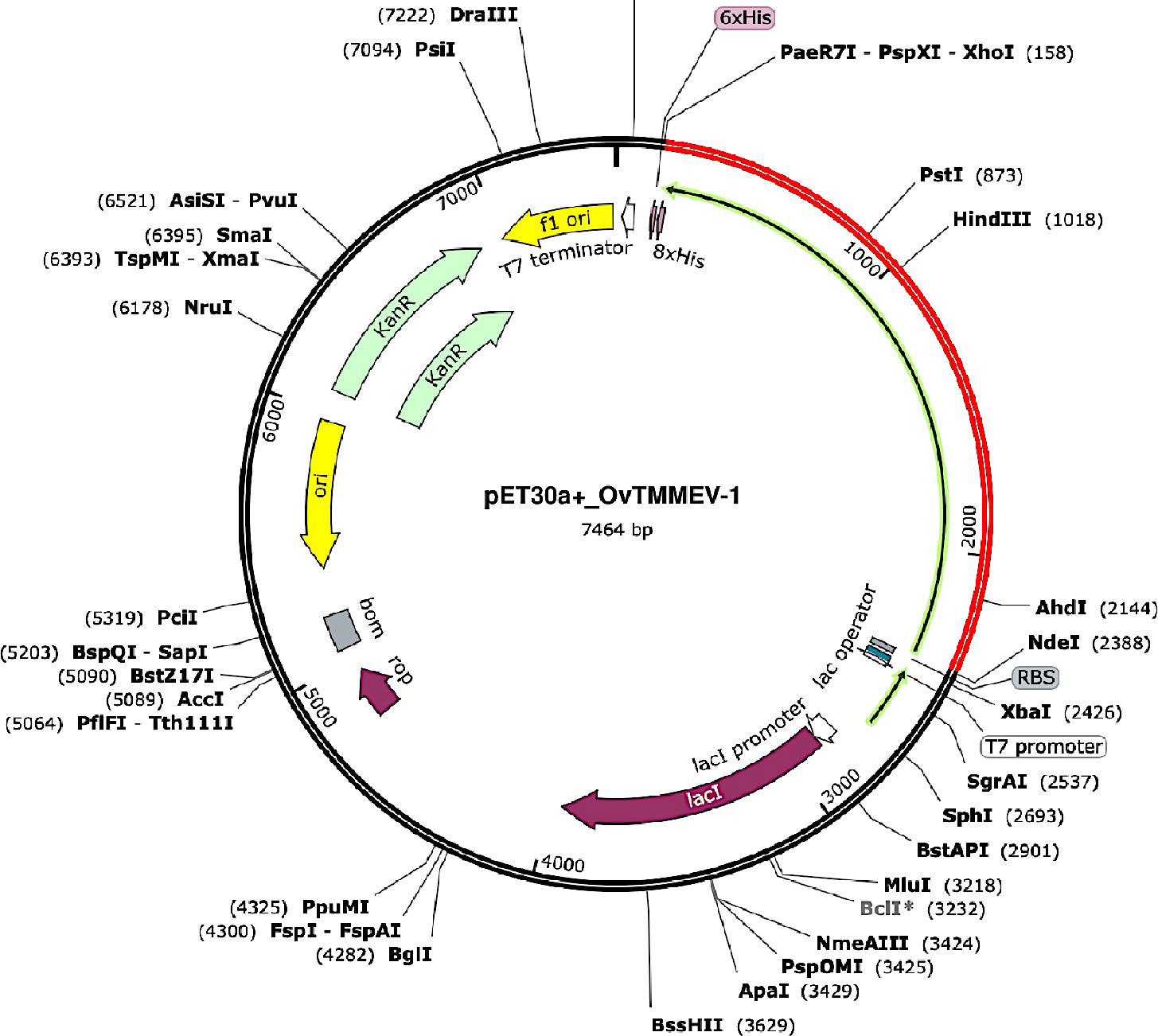
Figure 9 In silico restriction cloning of the designed vaccine gene sequence into the pET30a (+) expression vector where the red part represents the gene coding for the vaccine and the black circle represents the vector backbone. The His-tag is located at the carboxy-terminal end. The key features of the generated recombinant plasmid are also displayed.
Discussion
Neglected tropical diseases, NTDs constitute the most frequent infections affecting the world’s poorest one billion people. In contrast to more known acute or emerging infections, NTDs are largely lingering and incapacitating (and often mutilating) conditions (109). The burden exerted by the onchocerciasis which is an NTD stimulated the deployment of global control measures that have thus far focused principally on chemotherapy with IVM. Though control programs using MDA with IVM have achieved considerable success, it has recently been reiterated that as the global health goal for onchocerciasis shifts from control to elimination, only the development of new tools could make this goal a reality. Amongst the tools needed for the current toolbox for onchocerciasis elimination are vaccines, novel diagnostic tools, and chemotherapeutics. Several studies in humans and animal models have hinted at the possibility of generating protective immunity against onchocerciasis – suggesting the feasibility of artificial immunity through vaccines. Vaccine development for onchocerciasis has focused on subunit vaccines that are more logistically feasible. The challenge however has been to find an effective antigen (given that O. volvulus is a multistage parasite) and an appropriate formulation for such a vaccine. These efforts have led to the identification of two promising antigens; Ov103 and Ov-RAL-2 have demonstrated protective immunity in animal models and are currently planned for clinical development by 2022.
However, the failures experienced during translation from preclinical to clinical studies continue to inundate the vaccine development pipeline and highlight the need for a change in strategy for antigen selection (110). Chimeric epitope-based vaccines, represent an attractive strategy to generate a more robust protective immune response; since it offers the possibility to combine several immunogenic epitopes into a vaccine candidate (111). As a result, it is possible to elicit a broad-based immune response against a variety of antigenic proteins without necessarily producing and injecting the complete protein, most of which is frequently immunologically unimportant (112). This might reduce formulation difficulties, expenses, and safety concerns. Additionally, the usage of epitopes reduces safety concerns associated with the use of entire recombinant proteins that can have an unwanted biological activity on the host (113). The availability of parasite omics data and a plethora of bioinformatics tools have facilitated the process of assembling chimeric antigens. The selection of high affinity, promiscuous epitopes that can interact with several HLA molecules, structural characterizations of the designed candidates, antigenicity and allergenicity predictions, and the prediction of protein-protein interactions between vaccine candidates and immune receptors constitute part of the analyses that can be done during computational vaccine design. This method is advantageous because of its cost-effectiveness as well as diminished risks and shortened duration and amongst others. The approach can consequently reduce some of the uncertainties embedded in the classical vaccine development pipeline (112). Therefore, in recent years, this epitope-based approach to vaccine development has been applied against different organisms including, bacteria, viruses, and parasites like Trichuris trichuria (114) and Brugia malayi (115), with several promising candidates reported (113).
Previously, Shey et al. (41) reported the design and preliminary characterization of a potential vaccine candidate against onchocerciasis based on the correlates of immune protection reported for the disease. The results from the study showed that epitopes for chimera designed could react with serum samples for putatively immune persons. The present work is focused on the computational design of a novel chimeric vaccine candidate, from the extracellular domains of parasite transmembrane proteins which have been reported to be conserved in other species. The same procedure has been employed to design an epitope-based vaccine using Schistosoma mansoni proteins (116). The rationale is to design a vaccine that can generate a potent immune response against onchocerciasis but could also be deployed in cross-protection against other disease-causing nematode parasites.
It has been reported that surface proteins in parasites, due to their exposure, can elicit an immune response since they interact with the host immune system (117). Beginning with proteins that showed a high degree of conservation in related nematodes, several tools were deployed to select transmembrane proteins. Subsequently, the use of different servers allowed for the selection of 14 trans-membrane proteins whose extracellular domains were predicted since these are the portions exposed to the immune system.
The extracellular domains were then assessed for the presence of strong-binding HTL epitopes as well as high-scoring CTL and linear B-cell epitopes. All the predicted epitopes were then subjected to another round of selection by screening for antigenicity. The applied selection criteria ensured the generation of a chimera containing CTL and HTL epitopes with strong affinity for MHC molecules and also having the potential to be recognized by B-cells. All these features are reported to be important correlates of protection against onchocerciasis.
To combine the different epitopes together into a single molecule, various linkers were employed. The following linkers: EAAAK, GGGS, KK, GPGPG, and AAY were selected to maximize protein expression, and epitope presentation based on results that have been obtained from previous studies. While the Mycobacterium tuberculosis RpfE was selected as a built-in TLR 4 agonist. This adjuvant has been reported to stimulate a concurrent Th1- and Th17-type T-cell immunity throughTLR4-dependent maturation of dendritic cells (DCs). DCs treated with RpfE efficiently triggered naïve CD4+T lymphocytes to produce IFN-γ, IL2, and IL17-A (96). The secretion of IFN-γ has been reported to correlate with protection (30). Possible interference between the adjuvant and epitope regions of the chimera was reduced by using the EAAAK to provide rigidity. This linker has been reported to be important in improving expression and in retaining the functional domains of bifunctional proteins (62). On the other hand, the GGGS linker was selected to improve flexibility and placed before the CTL epitopes. The GPGPG and AAY linkers were used to ensure optimal epitope processing and presentation – minimizing the formation of junctional epitopes (53). Lastly, the KK epitopes were added between linear B-epitopes to ensure proper recognition of the separate epitopes and generation of the desired immune response. The designed chimera had a total of 742 amino acids which is higher than the average size of bacterial proteins (118). This larger size could lead to challenges with expressing the protein in the soluble form. However, bioinformatics analyses using the SOLpro and SoluProt servers predicted both proteins to be soluble upon expression in bacteria. In addition, though previous studies with proteins of similar lengths have resulted in the production of proteins as inclusion bodies, the use of refolding techniques following purification (119) resulted in proteins that were reactive with serum from exposed persons (41). On the other hand, in case of any challenges with the solubility of the designed protein during experimental validation analyses, other protein expression hosts more amenable to the expression of high-molecular-weight proteins, like mammalian cells can also be another alternative (120).
Both human and animal model studies addressing the immune mechanisms elicited by vaccines against onchocerciasis have reported the importance of an antibody response, CD4+ T cell responses, and IFN-γ production in protection against infection with O. volvulus parasite (121). In addition, a significantly higher CD8+ response has been reported in putative immunes (45). Mice model studies also postulate that infection with microfilariae initially provokes a CD8+ response, which exerts a damaging outcome on the initial establishment of parasites, and afterward provokes a CD4+ T-lymphocyte response which overcomes and destroys any parasites residing in the host (122). The generation of a massive population of CD8+ effector memory and effector T-cell subsets is reported to be amongst the key mechanisms for the maintenance of Rpf-specific T-cell responses in long-term M. tuberculosis nonprogressors (123). The pan DR-biding epitope (PADRE) which is a universal T helper epitope, has been reported to greatly improve the antibody immune responses elicited by a recombinant antigen vaccine against malaria (124). PADRE is a universal synthetic 13 amino acid peptide that activates CD4+ T cells (125). These observations support the choice of PADRE and M. tuberculosis RpfE adjuvants, as supplementary components of the designed chimeric vaccine candidate.
In this study, the IFNepitope and IL4pred servers predicted large numbers of IFN-γ and IL4-inducing epitopes in the designed multi-epitope vaccine candidate. Both cytokines have been reported to correlate with protection against O. volvulus (30, 47). Moreover, in-silico immune simulation analysis using the C-ImmSim server predicted an increase in antibody production after immunization in addition to high levels of IFN-γ secretion and an enduring cellular response. In addition, predicted. The analyses showed increases in antibody levels for the booster doses, indicating the development of immune memory. This correlates with the emergence of memory B- and T- lymphocytes that was apparent. The critical role of antibodies in immune protection against onchocerciasis has been reported (46). Helper T cells were particularly stimulated. Depending on the parasite/infection stage, T cell-mediated responses against filarial parasites have been reported to be characterized by both T helper 1 and T helper 2 cytokine activity (126). The ability of the designed vaccine candidate to stimulate both the cellular and humoral arms of the immune system supports its potential to provoke a robust protective immune response against onchocerciasis.
Helminth parasites deploy a range of sophisticated mechanisms to alter host immune responses. This helps them to persist in the host for long durations (127). For Onchocerca volvulus, the adult worms have been reported to persist for up to 15 years in the human host (128). A key mechanism involved in immunoregulation by parasites is through the interaction of parasite antigens with diverse Pattern Recognition Receptors (PRRs), including toll-like receptors (TLRs) (129). Conversely, TLR4 has also been reported to be implicated in protective responses against onchocerciasis (49), trypanosomiasis (130), and others (131). The capacity of the designed vaccine to elicit a protective innate immune response was potentiated through the incorporation of the TLR4 agonist, RpfE (RPFE_MYCTU) from Mycobacterium tuberculosis (96). Protein-protein docking interaction of the vaccine construct with the TLR4 receptor identified low energy binding modes matching that of interaction profile of X-ray-determined structures. This emphasizes the feasibility of interaction between the multi-epitope vaccine antigen and human TLR4 as an important step to initiate immune responses (87). Consistently, the data obtained from molecular dynamic simulation analyses predicted a stable interaction between the vaccine candidate and the TLR4. Again, this interaction is necessary to stimulate the innate immune system which will direct the adaptive immune response towards protective immunity against the parasite. Compared to an acquired response to infection, innate immune responses to a vaccine also result from the appropriate signaling and activation of TLR4 receptor present on immune cells like amongst others macrophages and dendritic cells. Park et al. experimentally examined the signaling mechanisms responsible for the activation of TLR4 and observed that Arg264, Tyr296, Lys341, Lys362, Lys388, and Glu436 are key residues involved in signaling (87). To assess if the MD calculations are supported by the experimental data, a contact frequency analysis of the TLR4 receptor was performed to monitor which residues localized to the binding region of the receptor do make what number of contacts with the candidate vaccine. Interestingly, four of experimentally solved residues known to underpin pathogenic recognition were captured by our simulations to be involved in stable interactions between the complex partners, and include Arg264, Tyr296, Lys341, and Lys362 (Figure 6D). Of these, Tyr 296 was shown to be the most relevant residue driving signaling as it accounts for about 98% of the interactions potentially responsible for the binding affinity in all three simulation replicates. Moreso, two residues (Asp238 and Asn241) predicted by our docking approximations are equally determined by the contact frequency data to be important for signaling, with Asp238 responsible for >80% of the stabilizing interactions in all three runs. These data are consistent with the experimental findings of Park et al. (87), pointing to the hotspot residues of interest as key drivers of the TLR4 innate immune responses to both infection and vaccination.
The relative sub-energy components making up the overall binding free energy include Polar Solvation energy, Van der Waals energy, Electrostatic energy, and SASA energy. Our findings suggest the Electrostatic energy (-19,231.3 +/- 1487.2 KJ/mol) and Polar Solvation energy (1,164.6 +/- 359.7 KJ/mol) are the main contributors to the binding affinity (-18,369.1 +/- 1,326.5 KJ/mol) of the complex. However, the solvation energy which reflects macromolecular solubility was positive. In a natural life system, molecules are required to dissolve for interaction or receptor signaling to occur. As such, the predicted insolubility of the vaccine construct seems to be a limiting factor for its development as a potential vaccine. We, therefore, investigated if the positive solvation energy resulted due to biases with the MM-GBSA predictor or are true predictions. Indeed, we identified two studies with positive solvation energies calculated by the same MM-GBSA method on different complex systems (93, 132). These data suggest that the construct may be soluble after all. However, these findings remain mere predictions, and so further experimental characterizations are required to assess in vitro and in vivo the validity of the construct to serve as a potential vaccine model against onchocerciasis and related filarial diseases in both animals and humans.
However, atomic resolution data from molecular dynamics simulation and binding free energy assessments in this study serve to predict the possibility and stability of interactions between the designed candidate vaccine and TLR4, and thereof recognition by the innate immunity. Although these virtual methods have rapidly evolved and conventionally adopted as reliable in silico validation strategies with applicable capacity to frequently make predictions close enough to the ground truth, they are however not without limitations – reproducibility and reliability. Different simulation replica may for several technical reasons follow alternate trajectories across the free energy landscape of a system. For best practices, simulations of >10 ns replicated three or more times are acceptable and used by many theoretical studies. In this study, we perform three different runs of up to 100 ns each and summarise the findings by considering the similarities and differences between the runs. In addition, we perform binding free energy calculations of a few sampled conformations from across the entire runs. It is, therefore, intended that these data provide the basis for further experimental studies by vaccine developers. In addition, the C-ImmSim server which was used for the immune simulation studies has been reported to produce dynamics that are stable and consistent with basic immunological knowledge (75).
The physicochemical characteristics of the designed chimeric vaccine candidate suggest the feasibility for expression in E. coli. The predicted solubility (predicted by two different servers), the half-life in E. coli (10h), the molecule stability (aliphatic index of 74.24 and instability index of 33.93) are all indicators that the bacterial platform is suitable for heterologous expression of the chimera. Given that the epitopes were selected from eukaryotic proteins, the protein sequence was reverse translated, and the obtained DNA sequence was codon-optimized, and in-silico cloned into the pET30a+ vector. This arrangement ensures optimal yield upon expression in the E. coli K12 strain. Physicochemical analyses predicted an isoelectric point of 9.52, indicating that the protein will be negatively charged under neutral conditions.
In the search for an effective vaccine candidate capable of generating the required immune response against onchocerciasis, only two candidates have passed the preclinical development criteria and are currently planned for clinical development. However, the continuous challenges experienced with the translation of vaccine candidates from animal models to first-in-human trials highlight the need for a persistent search of novel antigens. Reverse vaccinology offers a reliable approach to vaccine antigen selection with several advantages (133, 134). The careful selection of proteins conserved in other parasite species implies that the generated vaccine candidate can elicit cross-protective immune responses. This is particularly important as co-endemicity is a common occurrence for most neglected tropical diseases (135–137).
In general, the results obtained from this study support the claim that cell surface localized proteins can be potential vaccine candidates since their highly exposed to the immune system (138). It also supports the claim that studying proteins of unknown function can culminate in the discovery of potential candidates with various biological functions. These concepts have therefore been combined in this study to design a multi-epitope protein that possesses potentially desirable antigenic properties. The predicted physicochemical, immunogenic, and structural properties suggest that the designed vaccine candidate could be an additional tool that can be deployed in the fight against onchocerciasis.
Thus far, all the results obtained are from in-silico studies. There remains a need for validation through in vitro and in vivo assays. In this regard, the first major step will be to express the protein in a suitable host. The E. coli bacteria could be used in the preliminary experiments due to its advantages including the fast growth kinetics and easy manipulation, high degree of flexibility with vectors and host strains, comparatively cheaper costs, high yield, and easy scale-up, amongst others (139). However, previous studies in mice models have reported that proteins expressed in Pichia pastoris were more effective in reducing parasite survival and protecting the host (140). Expressed proteins can then be used for serological assays with sera from infected persons and PIs since the role of antibodies in protection against Onchocerca volvulus has been reported (46). In addition, the expressed protein can be used to raise antibodies in rabbits for in-vitro evaluation of antibody-dependent cell-mediated cytotoxicity (ADCC) of neutrophils on the L3 larval stage or microfilariae as previously described (33). Satisfactory results obtained from these in-vitro investigations depending on the set criteria will serve as the basis to proceed for in-vivo challenge studies in mice models with L3 larvae. Though the BALB/c mice model has been used for several studies, a humanized NSG mice model reported to be more amenable to supporting the life cycle of the Onchocerca volvulus parasite (141) could be a better host for in-vivo experiments. For the designed chimera, the number of predicted peptides capable of binding to mouse alleles indicates that the protein will be able to elicit an immune response in a mouse model (Table 4). The results from both in-vitro and in-vivo studies will then provide the basis for the investigation of the vaccine candidate in clinical trials.
Data availability statement
The original contributions presented in the study are included in the article/Supplementary Material. Further inquiries can be directed to the corresponding author.
Author contributions
RS, DN, CS, BY, NY, SG, LA, LV, RN, and JS designed the experiments. RS, CS, BY, NY, FN, RN, FB, AL, KE, TM, NT, AD, and DN carried out the analyses. RS, DN, CS, BY, KE, and NY wrote the manuscript. RS, FN, NT, and DN prepared figures. RS submitted this paper. All authors reviewed the manuscript. All authors contributed to the article and approved the submitted version.
Funding
JS is supported by the Belgian Academy of Higher Education and Research, abbreviated by its French acronym as ARES (https://www.ares-ac.be/fr/) through grant ARES-PRD2020CAMEROON. The funders had no role in study design, data collection and analysis, decision to publish, or preparation of the manuscript.
Acknowledgments
The authors acknowledge all the open-source and globally available databases that were utilized for data mining. LV is the director of research at the Belgian National Fund for Scientific Research (FRS-FNRS).
Conflict of interest
The authors declare that the research was conducted in the absence of any commercial or financial relationships that could be construed as a potential conflict of interest.
Publisher’s note
All claims expressed in this article are solely those of the authors and do not necessarily represent those of their affiliated organizations, or those of the publisher, the editors and the reviewers. Any product that may be evaluated in this article, or claim that may be made by its manufacturer, is not guaranteed or endorsed by the publisher.
Supplementary material
The Supplementary Material for this article can be found online at: https://www.frontiersin.org/articles/10.3389/fitd.2022.1046522/full#supplementary-material
References
1. Alhassan A, Makepeace BL, LaCourse EJ, Osei-Atweneboana MY, Carlow CKS. A simple isothermal DNA amplification method to screen black flies for onchocerca volvulus infection. PloS One (2014) 9(10):e108927. doi: 10.1371/journal.pone.0108927
3. Noormahomed EV, Akrami K, Mascaró-Lazcano C. Onchocerciasis, an undiagnosed disease in Mozambique: identifying research opportunities. Parasites Vectors. (2016) 9(1):180. doi: 10.1186/s13071-016-1468-7
4. Gebrezgabiher G, Mekonnen Z, Yewhalaw D, Hailu A. Reaching the last mile: main challenges relating to and recommendations to accelerate onchocerciasis elimination in Africa. Infect Dis Poverty (2019) 8(1):60. doi: 10.1186/s40249-019-0567-z
5. Gopinath R, Ostrowski M, Justement SJ, Fauci AS, Nutman TB. Filarial infections increase susceptibility to human immunodeficiency virus infection in peripheral blood mononuclear cells in vitro. J Infect Dis (2000) 182(6):1804–8. doi: 10.1086/317623
6. Ngu JL, Soothill JF. Immune complex nephropathy in the tropics. In: Chandra RK, editor. Critical reviews in tropical medicine, vol. Volume 1 . Boston, MA: Springer US (1982). p. 275–306.
7. Colebunders R, Nelson Siewe FJ, Hotterbeekx A. Onchocerciasis-associated epilepsy, an additional reason for strengthening onchocerciasis elimination programs. Trends Parasitol (2018) 34(3):208–16. doi: 10.1016/j.pt.2017.11.009
8. Little MP, Basanez MG, Breitling LP, Boatin BA, Alley ES. Incidence of blindness during the onchocerciasis control programme in western Africa, 1971-2002. J Infect Dis (2004) 189(10):1932–41. doi: 10.1086/383326
9. Walker ER, McGee RE, Druss BG. Mortality in mental disorders and global disease burden implications: a systematic review and meta-analysis. JAMA Psychiatry (2015) 72(4):334–41. doi: 10.1001/jamapsychiatry.2014.2502
10. James SL, Abate D, Abate KH, Abay SM, Abbafati C, Abbasi N, et al. Global, regional, and national incidence, prevalence, and years lived with disability for 354 diseases and injuries for 195 countries and territories, 1990–2017: a systematic analysis for the global burden of disease study 2017. Lancet (2018) 392(10159):1789–858. doi: 10.1016/S0140-6736(18)32279-7
11. Dadzie Y, Amazigo UV, Boatin BA, Sékétéli A. Is onchocerciasis elimination in Africa feasible by 2025: a perspective based on lessons learnt from the African control programmes. Infect Dis Poverty (2018) 7(1):63. doi: 10.1186/s40249-018-0446-z
12. Lakwo T, Oguttu D, Ukety T, Post R, Bakajika D. Onchocerciasis elimination: Progress and challenges. Res Rep Trop Med (2020) 11:81–95. doi: 10.2147/RRTM.S224364
13. Sauerbrey M, Rakers LJ, Richards FO. Progress toward elimination of onchocerciasis in the americas. Int Health (2018) 10(suppl_1):i71–i8. doi: 10.1093/inthealth/ihx039
14. Murdoch ME. Mapping the burden of onchocercal skin disease*. Br J Dermatol (2021) 184(2):199–207. doi: 10.1111/bjd.19143
15. Bourguinat C, Pion SDS, Kamgno J, Gardon J, Duke BOL, Boussinesq M, et al. Genetic selection of low fertile onchocerca volvulus by ivermectin treatment. PloS Negl Trop Dis (2007) 1(1):e72–e. doi: 10.1371/journal.pntd.0000072
16. Osei-Atweneboana MY, Awadzi K, Attah SK, Boakye DA, Gyapong JO, Prichard RK. Phenotypic evidence of emerging ivermectin resistance in onchocerca volvulus. PloS Negl Trop Dis (2011) 5(3):e998. doi: 10.1371/journal.pntd.0000998
17. Twum-Danso NAY. Loa loa encephalopathy temporally related to ivermectin administration reported from onchocerciasis mass treatment programs from 1989 to 2001: implications for the future. Filaria J (2003) 2 Suppl 1(Suppl 1):S7–S. doi: 10.1186/1475-2883-2-S1-S7
18. Odikamnoro OO, Uhuo C, Okoh F. Onchocerca volvulus/Loa loa co-infection in rural communities of southeast Nigeria-implication for ivermectin therapy. Int J Infect Dis (2018) 73:76. doi: 10.1016/j.ijid.2018.04.3599
19. Senyonjo L, Oye J, Bakajika D, Biholong B, Tekle A, Boakye D, et al. Factors associated with ivermectin non-compliance and its potential role in sustaining onchocerca volvulus transmission in the West region of Cameroon. PloS Negl Trop Dis (2016) 10(8):e0004905. doi: 10.1371/journal.pntd.0004905
20. Lakwo TL, Gasarasi DB. Non-adherence to community directed treatment with ivermectin for onchocerciasis control in rungwe district, southwest Tanzania. East Afr Med J (2006) 83(6):326–32. doi: 10.4314/eamj.v83i6.9440
21. Farrell SH, Anderson RM. Helminth lifespan interacts with non-compliance in reducing the effectiveness of anthelmintic treatment. Parasites Vectors. (2018) 11(1):66. doi: 10.1186/s13071-018-2670-6
22. Taylor HR, Pacqué M, Muñoz B, Greene BM. Impact of mass treatment of onchocerciasis with ivermectin on the transmission of infection. Science (1990) 250(4977):116–8. doi: 10.1126/science.2218502
23. Nikièma AS, Koala L, Sondo AK, Post RJ, Paré AB, Kafando CM, et al. The impact of ivermectin on onchocerciasis in villages co-endemic for lymphatic filariasis in an area of onchocerciasis recrudescence in Burkina Faso. PloS Negl Trop Dis (2021) 15(3):e0009117. doi: 10.1371/journal.pntd.0009117
24. Rothova A, van der Lelij A, Stilma JS, Wilson WR, Barbe RF. Side-effects of ivermectin in treatment of onchocerciasis. Lancet (1989) 1(8652):1439–41. doi: 10.1016/S0140-6736(89)90136-0
25. Verver S, Walker M, Kim YE, Fobi G, Tekle AH, Zouré HGM, et al. How can onchocerciasis elimination in Africa be accelerated? modeling the impact of increased ivermectin treatment frequency and complementary vector control. Clin Infect Dis (2018) 66(suppl_4):S267–s74. doi: 10.1093/cid/cix1137
26. Cook JA, Steel C, Ottesen EA. Towards a vaccine for onchocerciasis. Trends Parasitol (2001) 17(12):555–8. doi: 10.1016/S1471-4922(01)02115-8
27. Makepeace BL, Babayan SA, Lustigman S, Taylor DW. The case for vaccine development in the strategy to eradicate river blindness (onchocerciasis) from Africa. Expert Rev Vaccines (2015) 14(9):1163–5. doi: 10.1586/14760584.2015.1059281
28. Turner HC, Walker M, Lustigman S, Taylor DW, Basáñez M-G. Human onchocerciasis: Modelling the potential long-term consequences of a vaccination programme. PloS Negl Trop Dis (2015) 9(7):e0003938. doi: 10.1371/journal.pntd.0003938
29. Lustigman S, Makepeace BL, Klei TR, Babayan SA, Hotez P, Abraham D, et al. Onchocerca volvulus: The road from basic biology to a vaccine. Trends Parasitol (2018) 34(1):64–79. doi: 10.1016/j.pt.2017.08.011
30. Elson LH, Calvopiña M, Paredes W, Araujo E, Bradley JE, Guderian RH, et al. Immunity to onchocerciasis: putative immune persons produce a Th1-like response to onchocerca volvulus. J Infect Dis (1995) 171(3):652–8. doi: 10.1093/infdis/171.3.652
31. Tchakouté VL, Graham SP, Jensen SA, Makepeace BL, Nfon CK, Njongmeta LM, et al. In a bovine model of onchocerciasis, protective immunity exists naturally, is absent in drug-cured hosts, and is induced by vaccination. Proc Natl Acad Sci (2006) 103(15):5971. doi: 10.1073/pnas.0601385103
32. Turaga PS, Tierney TJ, Bennett KE, McCarthy MC, Simonek SC, Enyong PA, et al. Immunity to onchocerciasis: cells from putatively immune individuals produce enhanced levels of interleukin-5, gamma interferon, and granulocyte-macrophage colony-stimulating factor in response to onchocerca volvulus larval and male worm antigens. Infect Immun (2000) 68(4):1905–11. doi: 10.1128/IAI.68.4.1905-1911.2000
33. Cho-Ngwa F, Liu J, Lustigman S. The onchocerca volvulus cysteine proteinase inhibitor, ov-CPI-2, is a target of protective antibody response that increases with age. PloS Negl Trop Dis (2010) 4(8):e800–e. doi: 10.1371/journal.pntd.0000800
34. Seidenfaden R, Fischer A, Bonow I, Ekale D, Tanya V, Renz A. Combined benefits of annual mass treatment with ivermectin and cattle zooprophylaxis on the severity of human onchocerciasis in northern Cameroon. Trop Med Int Health (2001) 6(9):715–25. doi: 10.1046/j.1365-3156.2001.00771.x
35. Rollenhagen C, Sörensen M, Rizos K, Hurvitz R, Bumann D. Antigen selection based on expression levels during infection facilitates vaccine development for an intracellular pathogen. Proc Natl Acad Sci U S A (2004) 101(23):8739–44. doi: 10.1073/pnas.0401283101
36. Lustigman S, James ER, Tawe W, Abraham D. Towards a recombinant antigen vaccine against onchocerca volvulus. Trends Parasitol (2002) 18(3):135–41. doi: 10.1016/S1471-4922(01)02211-5
37. Ryan NM, Hess JA, de Villena FP, Leiby BE, Shimada A, Yu L, et al. Onchocerca volvulus bivalent subunit vaccine induces protective immunity in genetically diverse collaborative cross recombinant inbred intercross mice. NPJ Vaccines (2021) 6(1):17. doi: 10.1038/s41541-020-00276-2
38. Herati RS, Wherry EJ. What is the predictive value of animal models for vaccine efficacy in humans? consideration of strategies to improve the value of animal models. Cold Spring Harb Perspect Biol (2018) 10(4):a031583. doi: 10.1101/cshperspect.a031583
39. Knox DP, Redmond DL. Parasite vaccines – recent progress and problems associated with their development. Parasitology (2006) 133(S2):S1–8. doi: 10.1017/S0031182006001776
40. Shey RA, Ghogomu SM, Esoh KK, Nebangwa ND, Shintouo CM, Nongley NF, et al. In-silico design of a multi-epitope vaccine candidate against onchocerciasis and related filarial diseases. Sci Rep (2019) 9(1):4409. doi: 10.1038/s41598-019-40833-x
41. Shey RA, Ghogomu SM, Shintouo CM, Nkemngo FN, Nebangwa DN, Esoh K, et al. Computational design and preliminary serological analysis of a novel multi-epitope vaccine candidate against onchocerciasis and related filarial diseases. Pathogens (2021) 10(2):99. doi: 10.3390/pathogens10020099
42. Meza B, Ascencio F, Sierra-Beltrán AP, Torres J, Angulo C. A novel design of a multi-antigenic, multistage and multi-epitope vaccine against helicobacter pylori: An in silico approach. Infect Genet Evol (2017) 49:309–17. doi: 10.1016/j.meegid.2017.02.007
43. Fankhauser N, Nguyen-Ha T-M, Adler J, Mäser P. Surface antigens and potential virulence factors from parasites detected by comparative genomics of perfect amino acid repeats. Proteome Sci (2007) 5(1):20. doi: 10.1186/1477-5956-5-20
44. Loukas A, Tran M, Pearson MS. Schistosome membrane proteins as vaccines. Int J Parasitol (2007) 37(3-4):257–63. doi: 10.1016/j.ijpara.2006.12.001
45. Katawa G, Layland LE, Debrah AY, von Horn C, Batsa L, Kwarteng A, et al. Hyperreactive onchocerciasis is characterized by a combination of Th17-Th2 immune responses and reduced regulatory T cells. PloS Negl Trop Dis (2015) 9(1):e3414. doi: 10.1371/journal.pntd.0003414
46. George PJ, Hess JA, Jain S, Patton JB, Zhan T, Tricoche N, et al. Antibody responses against the vaccine antigens ov-103 and ov-RAL-2 are associated with protective immunity to onchocerca volvulus infection in both mice and humans. PloS Negl Trop Dis (2019) 13(9):e0007730. doi: 10.1371/journal.pntd.0007730
47. Lange AM, Yutanawiboonchai W, Scott P, Abraham D. IL-4- and IL-5-dependent protective immunity to onchocerca volvulus infective larvae in BALB/cBYJ mice. J Immunol (1994) 153(1):205–11.
48. Brattig NW, Lepping B, Timmann C, Büttner DW, Marfo Y, Hamelmann C, et al. Onchocerca volvulus-exposed persons fail to produce interferon-gamma in response to o. volvulus antigen but mount proliferative responses with interleukin-5 and IL-13 production that decrease with increasing microfilarial density. J Infect Dis (2002) 185(8):1148–54. doi: 10.1086/339820
49. Kerepesi LA, Leon O, Lustigman S, Abraham D. Protective immunity to the larval stages of onchocerca volvulus is dependent on toll-like receptor 4. Infect Immun (2005) 73(12):8291–7. doi: 10.1128/IAI.73.12.8291-8297.2005
50. Cotton JA, Bennuru S, Grote A, Harsha B, Tracey A, Beech R, et al. The genome of onchocerca volvulus, agent of river blindness. Nat Microbiol (2016) 2(2):16216. doi: 10.1038/nmicrobiol.2016.216
51. UniProt C. The universal protein resource (UniProt). Nucleic Acids Res (2008) 36(Database issue):D190–D5. doi: 10.1093/nar/gkm895
52. Larsen MV, Lundegaard C, Lamberth K, Buus S, Lund O, Nielsen M. Large-Scale validation of methods for cytotoxic T-lymphocyte epitope prediction. BMC Bioinf (2007) 8:424. doi: 10.1186/1471-2105-8-424
53. Sette A, Livingston B, McKinney D, Appella E, Fikes J, Sidney J, et al. The development of multi-epitope vaccines: epitope identification, vaccine design and clinical evaluation. Biologicals (2001) 29(3-4):271–6. doi: 10.1006/biol.2001.0297
54. Larsen MV, Lundegaard C, Lamberth K, Buus S, Brunak S, Lund O, et al. An integrative approach to CTL epitope prediction: A combined algorithm integrating MHC class I binding, TAP transport efficiency, and proteasomal cleavage predictions. Eur J Immunol (2005) 35(8):2295–303. doi: 10.1002/eji.200425811
55. Peters B, Bulik S, Tampe R, Van Endert PM, Holzhütter HG. Identifying MHC class I epitopes by predicting the TAP transport efficiency of epitope precursors. J Immunol (2003) 171(4):1741–9. doi: 10.4049/jimmunol.171.4.1741
56. Nielsen M, Lund O. NN-align. an artificial neural network-based alignment algorithm for MHC class II peptide binding prediction. BMC Bioinf (2009) 10(1):296. doi: 10.1186/1471-2105-10-296
57. Jespersen MC, Peters B, Nielsen M, Marcatili P. BepiPred-2.0: improving sequence-based b-cell epitope prediction using conformational epitopes. Nucleic Acids Res (2017) 45(W1):W24–W9. doi: 10.1093/nar/gkx346
58. Kadam K, Peerzada N, Karbhal R, Sawant S, Valadi J, Kulkarni-Kale U. Antibody class(es) predictor for epitopes (AbCPE): A multi-label classification algorithm. Front Bioinf (2021) 1. doi: 10.3389/fbinf.2021.709951
59. Doytchinova IA, Flower DR. VaxiJen: a server for prediction of protective antigens, tumour antigens and subunit vaccines. BMC Bioinf (2007) 8(1):4. doi: 10.1186/1471-2105-8-4
60. Pulendran B S, Arunachalam P, O’Hagan DT. Emerging concepts in the science of vaccine adjuvants. Nat Rev Drug Discov (2021) 20(6):454–75. doi: 10.1038/s41573-021-00163-y
61. Khan MT, Islam R, Jerin TJ, Mahmud A, Khatun S, Kobir A, et al. Immunoinformatics and molecular dynamics approaches: Next generation vaccine design against West Nile virus. PloS One (2021) 16(6):e0253393. doi: 10.1371/journal.pone.0253393
62. Arai R, Ueda H, Kitayama A, Kamiya N, Nagamune T. Design of the linkers which effectively separate domains of a bifunctional fusion protein. Protein Eng (2001) 14(8):529–32. doi: 10.1093/protein/14.8.529
63. Wilkins MR, Gasteiger E, Bairoch A, Sanchez JC, Williams KL, Appel RD, et al. Protein identification and analysis tools in the ExPASy server. Methods Mol Biol (1999) 112:531–52. doi: 10.1385/1-59259-584-7:531
64. Hon J, Marusiak M, Martinek T, Kunka A, Zendulka J, Bednar D, et al. SoluProt: Prediction of soluble protein expression in escherichia coli. Bioinformatics (2021) 37(1):23–8. doi: 10.1093/bioinformatics/btaa1102
65. Magnan CN, Randall A, Baldi P. SOLpro: accurate sequence-based prediction of protein solubility. Bioinformatics (2009) 25(17):2200–7. doi: 10.1093/bioinformatics/btp386
66. McGuffin LJ, Bryson K, Jones DT. The PSIPRED protein structure prediction server. Bioinformatics (2000) 16(4):404–5. doi: 10.1093/bioinformatics/16.4.404
67. Wang S, Li W, Liu S, Xu J. RaptorX-property: a web server for protein structure property prediction. Nucleic Acids Res (2016) 44(W1):W430–5. doi: 10.1093/nar/gkw306
68. Magnan CN, Zeller M, Kayala MA, Vigil A, Randall A, Felgner PL, et al. High-throughput prediction of protein antigenicity using protein microarray data. Bioinformatics (2010) 26(23):2936–43. doi: 10.1093/bioinformatics/btq551
69. Dimitrov I, Bangov I, Flower DR, Doytchinova I. AllerTOP v.2–a server for in silico prediction of allergens. J Mol Model (2014) 20(6):2278. doi: 10.1007/s00894-014-2278-5
70. Dimitrov I, Naneva L, Doytchinova I, Bangov I. AllergenFP: allergenicity prediction by descriptor fingerprints. Bioinformatics (2014) 30(6):846–51. doi: 10.1093/bioinformatics/btt619
71. Tau G, Rothman P. Biologic functions of the IFN-gamma receptors. Allergy (1999) 54(12):1233–51. doi: 10.1034/j.1398-9995.1999.00099.x
72. Dhanda SK, Vir P, Raghava GP. Designing of interferon-gamma inducing MHC class-II binders. Biol Direct (2013) 8:30. doi: 10.1186/1745-6150-8-30
73. Allen JE, Sutherland TE. Host protective roles of type 2 immunity: parasite killing and tissue repair, flip sides of the same coin. Semin Immunol(2014) 26(4):329–40.
74. Dhanda SK, Gupta S, Vir P, Raghava GP. Prediction of IL4 inducing peptides. Clin Dev Immunol (2013) 2013:263952. doi: 10.1155/2013/263952
75. Rapin N, Lund O, Bernaschi M, Castiglione F. Computational immunology meets bioinformatics: the use of prediction tools for molecular binding in the simulation of the immune system. PloS One (2010) 5(4):e9862. doi: 10.1371/journal.pone.0009862
76. Hotez PJ, Bottazzi ME, Zhan B, Makepeace BL, Klei TR, Abraham D, et al. The onchocerciasis vaccine for Africa–TOVA–Initiative. PloS Negl Trop Dis (2015) 9(1):e0003422–e. doi: 10.1371/journal.pntd.0003422
77. Roy A, Kucukural A, Zhang Y. I-TASSER: a unified platform for automated protein structure and function prediction. Nat Protoc (2010) 5(4):725–38. doi: 10.1038/nprot.2010.5
78. Zhang Y. I-TASSER server for protein 3D structure prediction. BMC Bioinf (2008) 9:40. doi: 10.1186/1471-2105-9-40
79. Zheng W, Zhang C, Bell EW, Zhang Y. I-TASSER gateway: A protein structure and function prediction server powered by XSEDE. Future Gener Comput Syst (2019) 99:73–85. doi: 10.1016/j.future.2019.04.011
80. Xu D, Zhang Y. Improving the physical realism and structural accuracy of protein models by a two-step atomic-level energy minimization. Biophys J (2011) 101(10):2525–34. doi: 10.1016/j.bpj.2011.10.024
81. Heo L, Park H, Seok C. GalaxyRefine: Protein structure refinement driven by side-chain repacking. Nucleic Acids Res (2013) 41(Web Server issue):W384–W8. doi: 10.1093/nar/gkt458
82. Lovell SC, Davis IW, Arendall WB 3rd, de Bakker PI, Word JM, Prisant MG, et al. Structure validation by calpha geometry: phi,psi and cbeta deviation. Proteins (2003) 50(3):437–50. doi: 10.1002/prot.10286
83. Wiederstein M, Sippl MJ. ProSA-web: interactive web service for the recognition of errors in three-dimensional structures of proteins. Nucleic Acids Res (2007) 35(Web Server issue):W407–W10. doi: 10.1093/nar/gkm290
84. Forsström B, Axnäs BB, Rockberg J, Danielsson H, Bohlin A, Uhlen M. Dissecting antibodies with regards to linear and conformational epitopes. PloS One (2015) 10(3):e0121673–e. doi: 10.1371/journal.pone.0121673
85. Ponomarenko J, Bui H-H, Li W, Fusseder N, Bourne PE, Sette A, et al. ElliPro: a new structure-based tool for the prediction of antibody epitopes. BMC Bioinf (2008) 9:514–. doi: 10.1186/1471-2105-9-514
86. Vilar S, Cozza G, Moro S. Medicinal chemistry and the molecular operating environment (MOE): application of QSAR and molecular docking to drug discovery. Curr Top Med Chem (2008) 8(18):1555–72. doi: 10.2174/156802608786786624
87. Park BS, Song DH, Kim HM, Choi B-S, Lee H, Lee J-O. The structural basis of lipopolysaccharide recognition by the TLR4–MD-2 complex. Nature (2009) 458(7242):1191–5. doi: 10.1038/nature07830
88. Naïm M, Bhat S, Rankin KN, Dennis S, Chowdhury SF, Siddiqi I, et al. Solvated interaction energy (SIE) for scoring protein–ligand binding affinities. 1. exploring the parameter space. J Chem Inf Model (2007) 47(1):122–33. doi: 10.1021/ci600406v
89. Cornell WD, Cieplak P, Bayly CI, Gould IR, Merz KM, Ferguson DM, et al. A second generation force field for the simulation of proteins, nucleic acids, and organic molecules. J Am Chem Society (1995) 117(19):5179–97. doi: 10.1021/ja00124a002
90. Van Der Spoel D, Lindahl E, Hess B, Groenhof G, Mark AE, Berendsen HJ. GROMACS: fast, flexible, and free. J Comput Chem (2005) 26(16):1701–18. doi: 10.1002/jcc.20291
91. Baker NA, Sept D, Joseph S, Holst MJ, McCammon JA. Electrostatics of nanosystems: application to microtubules and the ribosome. Proc Natl Acad Sci U S A (2001) 98(18):10037–41. doi: 10.1073/pnas.181342398
92. Kumari R, Kumar R, Lynn A. G_mmpbsa–a GROMACS tool for high-throughput MM-PBSA calculations. J Chem Inf Model (2014) 54(7):1951–62. doi: 10.1021/ci500020m
93. Wang Q, Zhao Y, Chen X, Hong A. Virtual screening of approved clinic drugs with main protease (3CL(pro)) reveals potential inhibitory effects on SARS-CoV-2. J Biomol Struct Dyn (2022) 40(2):685–95. doi: 10.1080/07391102.2020.1817786
94. dos Reis M, Wernisch L, Savva R. Unexpected correlations between gene expression and codon usage bias from microarray data for the whole escherichia coli K-12 genome. Nucleic Acids Res (2003) 31(23):6976–85. doi: 10.1093/nar/gkg897
95. Nussinov R. Eukaryotic dinucleotide preference rules and their implications for degenerate codon usage. J Mol Biol (1981) 149(1):125–31. doi: 10.1016/0022-2836(81)90264-3
96. Choi HG, Kim WS, Back YW, Kim H, Kwon KW, Kim JS, et al. Mycobacterium tuberculosis RpfE promotes simultaneous Th1- and Th17-type T-cell immunity via TLR4-dependent maturation of dendritic cells. Eur J Immunol (2015) 45(7):1957–71. doi: 10.1002/eji.201445329
97. Chang KY, Yang J-R. Analysis and prediction of highly effective antiviral peptides based on random forests. PloS One (2013) 8(8):e70166–e. doi: 10.1371/journal.pone.0070166
98. Enany S. Structural and functional analysis of hypothetical and conserved proteins of clostridium tetani. J Infect Public Health (2014) 7(4):296–307. doi: 10.1016/j.jiph.2014.02.002
99. Zhang Y, Skolnick J. Scoring function for automated assessment of protein structure template quality. Proteins (2004) 57(4):702–10. doi: 10.1002/prot.20264
100. Hise AG, Gillette-Ferguson I, Pearlman E. Immunopathogenesis of onchocerca volvulus keratitis (river blindness): a novel role for TLR4 and endosymbiotic wolbachia bacteria. J Endotoxin Res (2003) 9(6):390–4. doi: 10.1177/09680519030090060101
101. Pfarr KM, Fischer K, Hoerauf A. Involvement of toll-like receptor 4 in the embryogenesis of the rodent filaria litomosoides sigmodontis. Med Microbiol Immunol (2003) 192(1):53–6. doi: 10.1007/s00430-002-0159-5
102. Ohto U, Yamakawa N, Akashi-Takamura S, Miyake K, Shimizu T. Structural analyses of human toll-like receptor 4 polymorphisms D299G and T399I. J Biol Chem (2012) 287(48):40611–7. doi: 10.1074/jbc.M112.404608
103. Medzhitov R, Preston-Hurlburt P, Janeway CA. A human homologue of the drosophila toll protein signals activation of adaptive immunity. Nature (1997) 388(6640):394–7. doi: 10.1038/41131
104. Gopalakrishnan C, Jethi S, Kalsi N, Purohit R. Biophysical aspect of huntingtin protein during polyQ: An in silico insight. Cell Biochem Biophys (2016) 74(2):129–39. doi: 10.1007/s12013-016-0728-7
105. Teilum K, Olsen JG, Kragelund BB. Protein stability, flexibility and function. Biochim Biophys Acta (2011) 1814(8):969–76. doi: 10.1016/j.bbapap.2010.11.005
106. Rajendran V, Gopalakrishnan C, Purohit R. Impact of point mutation P29S in RAC1 on tumorigenesis. Tumour Biol (2016) 37(11):15293–304. doi: 10.1007/s13277-016-5329-y
107. Forouzesh N, Mishra N. An effective MM/GBSA protocol for absolute binding free energy calculations: A case study on SARS-CoV-2 spike protein and the human ACE2 receptor. Molecules (2021) 26(8):2383. doi: 10.3390/molecules26082383
108. Ylilauri M, Pentikäinen OT. MMGBSA as a tool to understand the binding affinities of filamin–peptide interactions. J Chem Inf Model (2013) 53(10):2626–33. doi: 10.1021/ci4002475
109. Bethony JM, Cole RN, Guo X, Kamhawi S, Lightowlers MW, Loukas A, et al. Vaccines to combat the neglected tropical diseases. Immunol Rev (2011) 239(1):237–70. doi: 10.1111/j.1600-065X.2010.00976.x
110. Buckland BC. The process development challenge for a new vaccine. Nat Med (2005) 11(4 Suppl):S16–9. doi: 10.1038/nm1218
111. De Groot AS, Moise L, McMurry JA, Martin W. Epitope-based immunome-derived vaccines: A strategy for improved design and safety. In: Falus A, editor. Clinical applications of immunomics. New York, NY: Springer US (2009). p. 39–69.
112. Hajissa K, Zakaria R, Suppian R, Mohamed Z. Epitope-based vaccine as a universal vaccination strategy against toxoplasma gondii infection: A mini-review. J Adv Vet Anim Res (2019) 6(2):174–82. doi: 10.5455/javar.2019.f329
113. Terry FE, Moise L, Martin RF, Torres M, Pilotte N, Williams SA, et al. Time for T? immunoinformatics addresses vaccine design for neglected tropical and emerging infectious diseases. Expert Rev Vaccines (2015) 14(1):21–35. doi: 10.1586/14760584.2015.955478
114. Zawawi A, Forman R, Smith H, Mair I, Jibril M, Albaqshi MH, et al. In silico design of a T-cell epitope vaccine candidate for parasitic helminth infection. PloS Pathog (2020) 16(3):e1008243. doi: 10.1371/journal.ppat.1008243
115. Anugraha G, Madhumathi J, Prince PR, Prita PJ, Khatri VK, Amdare NP, et al. Chimeric epitope vaccine from multistage antigens for lymphatic filariasis. Scand J Immunol (2015) 82(4):380–9. doi: 10.1111/sji.12340
116. Sanches RCO, Tiwari S, Ferreira LCG, Oliveira FM, Lopes MD, Passos MJF, et al. Immunoinformatics design of multi-epitope peptide-based vaccine against schistosoma mansoni using transmembrane proteins as a target. Front Immunol (2021) 12:621706–. doi: 10.3389/fimmu.2021.621706
117. Schmid-Hempel P. Immune defence, parasite evasion strategies and their relevance for ‘macroscopic phenomena’ such as virulence. Philos Trans R Soc Lond B Biol Sci (2009) 364(1513):85–98. doi: 10.1098/rstb.2008.0157
118. Tiessen A, Pérez-Rodríguez P, Delaye-Arredondo LJ. Mathematical modeling and comparison of protein size distribution in different plant, animal, fungal and microbial species reveals a negative correlation between protein size and protein number, thus providing insight into the evolution of proteomes. BMC Res Notes (2012) 5(1):85. doi: 10.1186/1756-0500-5-85
119. Shey RA, Ghogomu SM, Njume FN, Gainkam LOT, Poelvoorde P, Mutesa L, et al. Prediction and validation of the structural features of Ov58GPCR, an immunogenic determinant of onchocerca volvulus. PloS One (2018) 13(9):e0202915. doi: 10.1371/journal.pone.0202915
120. Crosnier C, Hokke CH, Protasio AV, Brandt C, Rinaldi G, Langenberg MCC, et al. Screening of a library of recombinant schistosoma mansoni proteins with sera from murine and human controlled infections identifies early serological markers. J Infect Dis (2020) 225(8):1435–46. doi: 10.1093/infdis/jiaa329
121. Doetze A, Erttmann KD, Gallin MY, Fleischer B, Hoerauf A. Production of both IFN-gamma and IL-5 by onchocerca volvulus S1 antigen-specific CD4+ T cells from putatively immune individuals. Int Immunol (1997) 9(5):721–9. doi: 10.1093/intimm/9.5.721
122. Folkard SG, Bianco AE. Roles for both CD4+ and CD8+ T cells in protective immunity against onchocerca lienalis microfilariae in the mouse. Parasite Immunol (1995) 17(10):541–53. doi: 10.1111/j.1365-3024.1995.tb00885.x
123. Commandeur S, van Meijgaarden KE, Lin MY, Franken KL, Friggen AH, Drijfhout JW, et al. Identification of human T-cell responses to mycobacterium tuberculosis resuscitation-promoting factors in long-term latently infected individuals. Clin Vaccine Immunol (2011) 18(4):676–83. doi: 10.1128/CVI.00492-10
124. Rosa DS, Tzelepis F, Cunha MG, Soares IS, Rodrigues MM. The pan HLA DR-binding epitope improves adjuvant-assisted immunization with a recombinant protein containing a malaria vaccine candidate. Immunol Lett (2004) 92(3):259–68. doi: 10.1016/j.imlet.2004.01.006
125. Ghaffari-Nazari H, Tavakkol-Afshari J, Jaafari MR, Tahaghoghi-Hajghorbani S, Masoumi E, Jalali SA. Improving multi-epitope long peptide vaccine potency by using a strategy that enhances CD4+ T help in BALB/c mice. PloS One (2015) 10(11):e0142563–e. doi: 10.1371/journal.pone.0142563
126. Kwarteng A, Asiedu E, Koranteng KK, Asiedu SO. Highlighting the relevance of CD8+ T cells in filarial infections. Front Immunol (2021) 12(3776). doi: 10.3389/fimmu.2021.714052
127. Maizels RM, Smits HH, McSorley HJ. Modulation of host immunity by helminths: The expanding repertoire of parasite effector molecules. Immunity (2018) 49(5):801–18. doi: 10.1016/j.immuni.2018.10.016
128. Voronin D, Tricoche N, Jawahar S, Shlossman M, Bulman CA, Fischer C, et al. Development of a preliminary in vitro drug screening assay based on a newly established culturing system for pre-adult fifth-stage onchocerca volvulus worms. PloS Negl Trop Dis (2019) 13(1):e0007108. doi: 10.1371/journal.pntd.0007108
129. Rajasekaran S, Anuradha R, Bethunaickan R. TLR specific immune responses against helminth infections. J Parasitol Res (2017) 2017:6865789. doi: 10.1155/2017/6865789
130. Oliveira AC, de Alencar BC, Tzelepis F, Klezewsky W, da Silva RN, Neves FS, et al. Impaired innate immunity in Tlr4(-/-) mice but preserved CD8+ T cell responses against trypanosoma cruzi in Tlr4-, Tlr2-, Tlr9- or Myd88-deficient mice. PloS Pathog (2010) 6(4):e1000870. doi: 10.1371/journal.ppat.1000870
131. Anthony RM, Rutitzky LI, Urban JF, Stadecker MJ, Gause WC. Protective immune mechanisms in helminth infection. Nat Rev Immunol (2007) 7(12):975–87. doi: 10.1038/nri2199
132. Kumari G, Nigam VK, Pandey DM. The molecular docking and molecular dynamics study of flavonol synthase and flavonoid 3’-monooxygenase enzymes involved for the enrichment of kaempferol. J Biomol Struct Dyn (2022) 2:1–14. doi: 10.1080/07391102.2022.2033324
133. Woolums AR, Swiderski C. New approaches to vaccinology made possible by advances in next generation sequencing, bioinformatics and protein modeling. Curr Issues Mol Biol (2021) 42:605–34. doi: 10.21775/cimb.042.605
134. Sette A, Rappuoli R. Reverse vaccinology: developing vaccines in the era of genomics. Immunity (2010) 33(4):530–41. doi: 10.1016/j.immuni.2010.09.017
135. Cano J, Basáñez M-G, O’Hanlon SJ, Tekle AH, Wanji S, Zouré HG, et al. Identifying co-endemic areas for major filarial infections in sub-Saharan Africa: seeking synergies and preventing severe adverse events during mass drug administration campaigns. Parasites Vectors. (2018) 11(1):70. doi: 10.1186/s13071-018-2655-5
136. Kelly-Hope LA, Blundell HJ, Macfarlane CL, Molyneux DH. Innovative surveillance strategies to support the elimination of filariasis in Africa. Trends Parasitol (2018) 34(8):694–711. doi: 10.1016/j.pt.2018.05.004
137. Clements ACA, Deville M-A, Ndayishimiye O, Brooker S, Fenwick A. Spatial co-distribution of neglected tropical diseases in the east African great lakes region: revisiting the justification for integrated control. Trop Med Int Health (2010) 15(2):198–207. doi: 10.1111/j.1365-3156.2009.02440.x
138. Monterrubio-López GP, González-Y-Merchand JA, Ribas-Aparicio RM. Identification of novel potential vaccine candidates against tuberculosis based on reverse vaccinology. BioMed Res Int (2015) 2015:483150. doi: 10.1155/2015/483150
139. Langlais C, Korn B. Recombinant protein expression in bacteria. encyclopedic reference of genomics and proteomics in molecular medicine. Berlin, Heidelberg: Springer Berlin Heidelberg (2006) p. 1609–16.
140. Hess JA, Zhan B, Bonne-Année S, Deckman JM, Bottazzi ME, Hotez PJ, et al. Vaccines to combat river blindness: expression, selection and formulation of vaccines against infection with onchocerca volvulus in a mouse model. Int J Parasitol (2014) 44(9):637–46. doi: 10.1016/j.ijpara.2014.04.006
Keywords: Onchocerciasis, immunoinformatics, multi-epitope vaccine, chimeric antigen, bioinformatics, transmembrane proteins
Citation: Shey RA, Ghogomu SM, Nebangwa DN, Shintouo CM, Yaah NE, Yengo BN, Nkemngo FN, Esoh KK, Tchatchoua NMT, Mbachick TT, Dede AF, Lemoge AA, Ngwese RA, Asa BF, Ayong L, Njemini R, Vanhamme L and Souopgui J (2022) Rational design of a novel multi-epitope peptide-based vaccine against Onchocerca volvulus using transmembrane proteins. Front. Trop. Dis 3:1046522. doi: 10.3389/fitd.2022.1046522
Received: 16 September 2022; Accepted: 13 October 2022;
Published: 03 November 2022.
Edited by:
Kathryn Jane Else, The University of Manchester, United KingdomReviewed by:
Ayat Tariq Zawawi, King Abdulaziz University, Saudi ArabiaBin Zhan, Baylor College of Medicine, United States
Copyright © 2022 Shey, Ghogomu, Nebangwa, Shintouo, Yaah, Yengo, Nkemngo, Esoh, Tchatchoua, Mbachick, Dede, Lemoge, Ngwese, Asa, Ayong, Njemini, Vanhamme and Souopgui. This is an open-access article distributed under the terms of the Creative Commons Attribution License (CC BY). The use, distribution or reproduction in other forums is permitted, provided the original author(s) and the copyright owner(s) are credited and that the original publication in this journal is cited, in accordance with accepted academic practice. No use, distribution or reproduction is permitted which does not comply with these terms.
*Correspondence: Jacob Souopgui, SmFjb2IuU291b3BndWlAdWxiLmJl
†These authors have contributed equally to this work and share last authorship