- 1Department of Public Health, Julia Jones Matthews School of Population and Public Health, Texas Tech University Health Science Center, Abilene, TX, United States
- 2Fulbright Scholar Program, Institute of Public Health, Rīga Stradiņš University, Rīga, Latvia
- 3Beckman Coulter Diagnostics, Brea, CA, United States
- 4Department of Engineering Technology, University of Houston, Houston, TX, United States
mHealth interventions have the potential to increase access to healthcare for the most hard-to-reach communities. For rural communities suffering disproportionately from skin-related NTDs, and Buruli ulcer, there is a need for low-cost, non-invasive and mobile tools for the early detection and management of disease. Dermoscopy is a noninvasive in-vivo technique that has been useful in improving the diagnostic accuracy of pigmented skin lesions based on anatomical features and morphological structures of lesions.
Objectives: Using dermoscopy, this study develops the automated tools necessary for developing an effective mHealth intervention towards identifying BU lesions in the early stages. Methods: This imaging methodology relies on an external attachment, a dermoscope, which uses polarized light to cancel out skin surface reflections. In our initial studies we used a dermoscope with only crosspolarized white-light (DL100, 3Gen) but later we adopted a more advanced multispectral dermoscope (DLIIm, 3Gen). The latter employed additional monochromatic light at different wavelengths of the visible spectral range, specifically blue (470 nm), yellow (580 nm), and red (660 nm) color, to visualize pigmented structures of skin layers at different depths.
Results: Results obtained using a subset of 58 white-light images with confirmed diagnosis (16 lesions BU and 42 lesions non-BU) resulting in sensitivity of 100 and specificity of 88.10, with an overall accuracy of 94.05 at 95% CI. Performance obtained using a second dataset of 197 dermoscopic multispectral images (16 lesions BU and 181 lesions non-BU) resulted in sensitivity of 90.00% and specificity of 93.39% with a balanced accuracy of 91.69% (86.95% to 95.12% at 95% CI).
Conclusions: This system will continue to perform even as the technology evolves and newer dermoscopes are available. Subsequent studies involve the DL4 which provides more uniform and brighter illumination, higher lesion magnification, and wider field of view which, combined with the superb resolution of modern smartphones, can result in faster and more accurate lesion assessment. This is an important step for the development of mHealth tools for use by non-specialists in community settings for the early detection of Buruli ulcer, skin-NTDs, and other dermatologic conditions associated with disease, including wound healing and management of disease progression.
Introduction
The WHO Global Observatory for eHealth defines mHealth as “medical and public health practice supported by mobile devices, such as mobile phones, patient monitoring devices, personal digital assistants, and other wireless devices” (1). Dedicated to the study of eHealth, the World Health Organization Global Observatory for eHealth is playing a crucial role in providing guidance for the policy, practice, and management of eHealth initiatives, especially for low-and middle-income countries (2).
The potential of mobile technologies for health interventions serves a particular value for under-resourced, and hard-to-reach communities, in that, mHealth technologies are mobile, low-cost, and non-invasive. Central to the development of mHealth tools is the utilization of the core components of the already ubiquitous mobile phone which already carries with it communication tools for voice and text, global positioning, 3G, 4G, and 5G telecommunication connectivity, and internal processing capabilities. Opportunities to capitalize on these tools and the processing capabilities of such devices lends to the creation and development of applications for epidemiological surveillance, screening and diagnosis of disease, communication tools to support health and wellness, and the introduction of tools for the self-management of illness.
During the last decade we have been focusing on the development of patented software tools (3, 4) that utilize dermoscopic images to detect skin lesions, in particular skin cancer (5–10). More recently we have employed the same engine in the analysis of Buruli ulcer (BU) images. To help health care workers identify neglected tropical diseases of the skin including BU, in 2020 WHO developed a pictorial training guide that was released into an interactive mobile phone application (Skin NTDs app) (11). To the best of our knowledge, our own reports (10, 12–15) are the only published studies to employ automatic dermoscopic image analysis to detect BU.
This study focuses on the development of automated tools for the identification of the structural properties of BU using dermoscopy. Dermoscopy is a noninvasive in-vivo technique that has been useful in improving the diagnostic accuracy of pigmented skin lesions (16) based on anatomical features and morphological structures of the lesions. Compared to clinical assessment which requires specialized clinical expertise, and PCR lab testing which involves advanced equipment, computerized analysis of dermoscopic images is fast, economical, and less dependent on experienced personnel; thus, it can potentially be used for prescreening patients and for improving overall decision-making.
Buruli ulcer (BU) is an ulcerative chronic infection of the skin caused by the bacterium Mycobacterium ulcerans, which commonly starts as a painless nodules, plaque, or edema, usually on the arms and legs, and progressively develops into large ulcers. Over time, it can lead to massive tissue destruction and debilitating deformities (17). BU predominantly affects rural populations in West and Central Africa (18). The World Health Organization (WHO) has classified BU as a neglected tropical disease (19, 20).
As the initial clinical manifestations are nonspecific, and the disease has a slow course, many patients do not seek medical care until there is large skin necrosis requiring extensive surgery and prolonged hospitalization, leading to permanent disfigurement and disability. However, when detected early, BU has 90% cure rate with a combination of antibiotics (21). If needed, surgery in the early stages of infection is curative and highly cost effective since it requires a simple excision. In later stages, however, wide and traumatizing excisions are needed, followed by skin grafting, and long hospital stays (11).
In most BU-endemic settings, a diagnosis is made on clinical and epidemiological grounds, and is typically followed by BU-specific antibiotics. Recent studies using an expert panel or polymerase chain reaction (PCR) testing as the reference have shown that clinical diagnosis is highly sensitivity, with high PPV and NPV (0.92, 0.92, and 0.86, respectively at 95% confidence interval (22).
Given that many of those affected by BU live in remote or resource-poor settings with no access to laboratory services, reliable diagnostic tools are not widely available to those that need them most. Additionally, some patients feel stigmatized and prefer to go to traditional healers. Very few health structures know how to deal with BU effectively, and while BU treatment is subsidized in most endemic areas, care is still expensive in specialized health centers. Therefore, accurate diagnostic procedures that are noninvasive, cost-effective, speedy, and easy to deploy in remote and resource-limited settings can reduce misclassification and improve the management of patients presenting with BU-like skin lesions.
Materials and methods
The overall analysis procedure of the BU recognition system is shown in Figure 1. Various steps of the automated analysis procedure for BU detection, and includes the following steps: multispectral image acquisition, image preprocessing to remove artifacts and spatial variations of light intensity, image segmentation to separate the lesion from healthy skin, feature extraction, features selection, and lesion classification, i.e., calculation of the probability of a lesion being Buruli ulcer.
Our imaging methodology relies on an external attachment, a dermoscope, which uses polarized light to cancel out skin surface reflections. In our initial studies we used a dermoscope with only crosspolarized white-light (DL100, 3Gen) but later on we adopted a more advanced multispectral dermoscope (DLIIm, 3Gen) shown in Figure 2A. The latter employed additional monochromatic light at different wavelengths of the visible spectral range, specifically blue (470 nm), yellow (580 nm), and red (660 nm) color, to visualize pigmented structures of skin layers at different depths. As technology evolves, newer dermoscopes are being developed, such as the DL4, shown in Figure 2B, that provide more uniform and brighter illumination, higher lesion magnification, and wider field of view which, combined with the superb resolution of modern smartphones, can result in faster and more accurate lesion assessment.
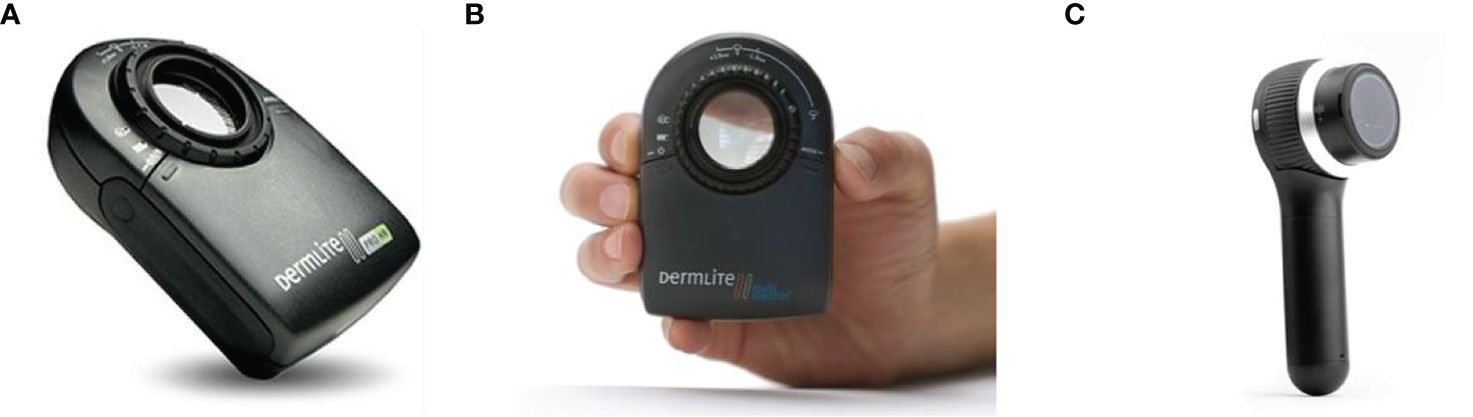
Figure 2 Various generations of dermoscopes used in our studies developed by 3Gen, LLC (23).
Data and image acquisition
The aim of this study was to focus on the acquisition of early-stage lesions in order to develop the automated systems for lesion segmentation and subsequent structural properties. The goal was to capture all forms of Buruli ulcers, nodules, plaques, and ulcers, in the early stages when the size of the area could fit within the 10mm scale of the DermLite.
Image data were collected in endemic BU communities of Cote d’Ivoire and Ghana in collaboration with the National Buruli Ulcer Control Program of the Ghana Health Service and the National Buruli Ulcer Control Programme, Cote d’Ivoire. The sample for this study is an all-African sample. The study received IRB approval from the Human Subjects Protection Committee at the University of Houston, as well as from ethics committees in both Ghana and Cote d’Ivoire. Informed consent was obtained in the preferred language of the participant.
To capture images of the suspicious area of the skin, we used a DermLite II Multispectral device (www.dermlite.com) attached to a Sony Cybershot DSC-W300 high-resolution camera, which provided a resolution of 13.5 MP (Figures 2–4). The dermoscope could provide white light for crosspolarization epiluminescence imaging, blue light for surface pigmentation, yellow light for superficial vascularity, and red light for deeper coloration and vascularity, using 32 bright LEDs–eight per color. Images were 24-bit full color with typical resolution of 4320 × 3240. Additional datasets of while-light images were obtained with a DermLite DL1 attached to an iPhone 4s.
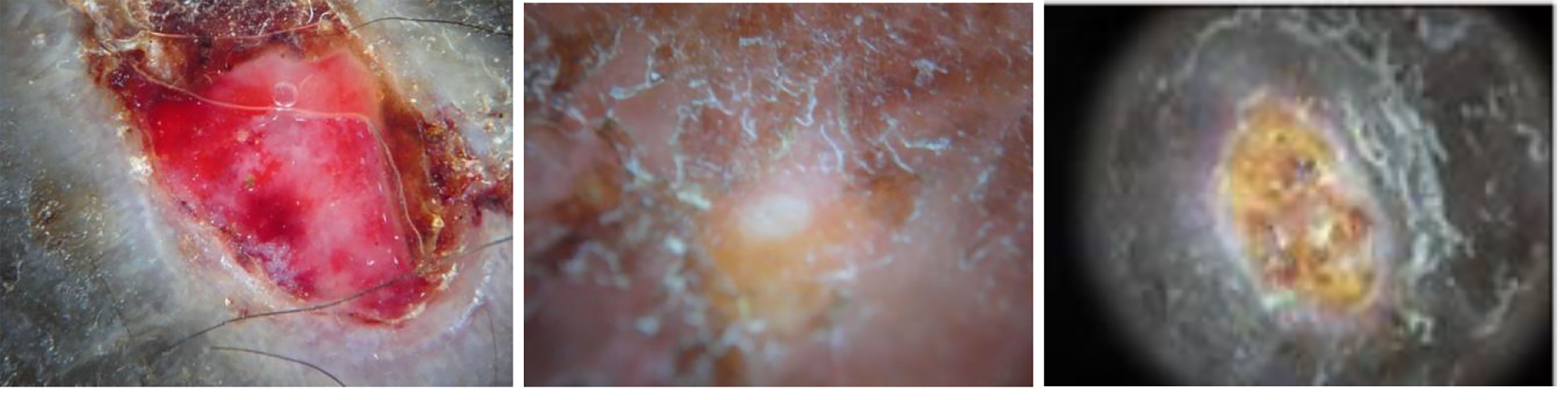
Figure 3 Examples of BU images (23).
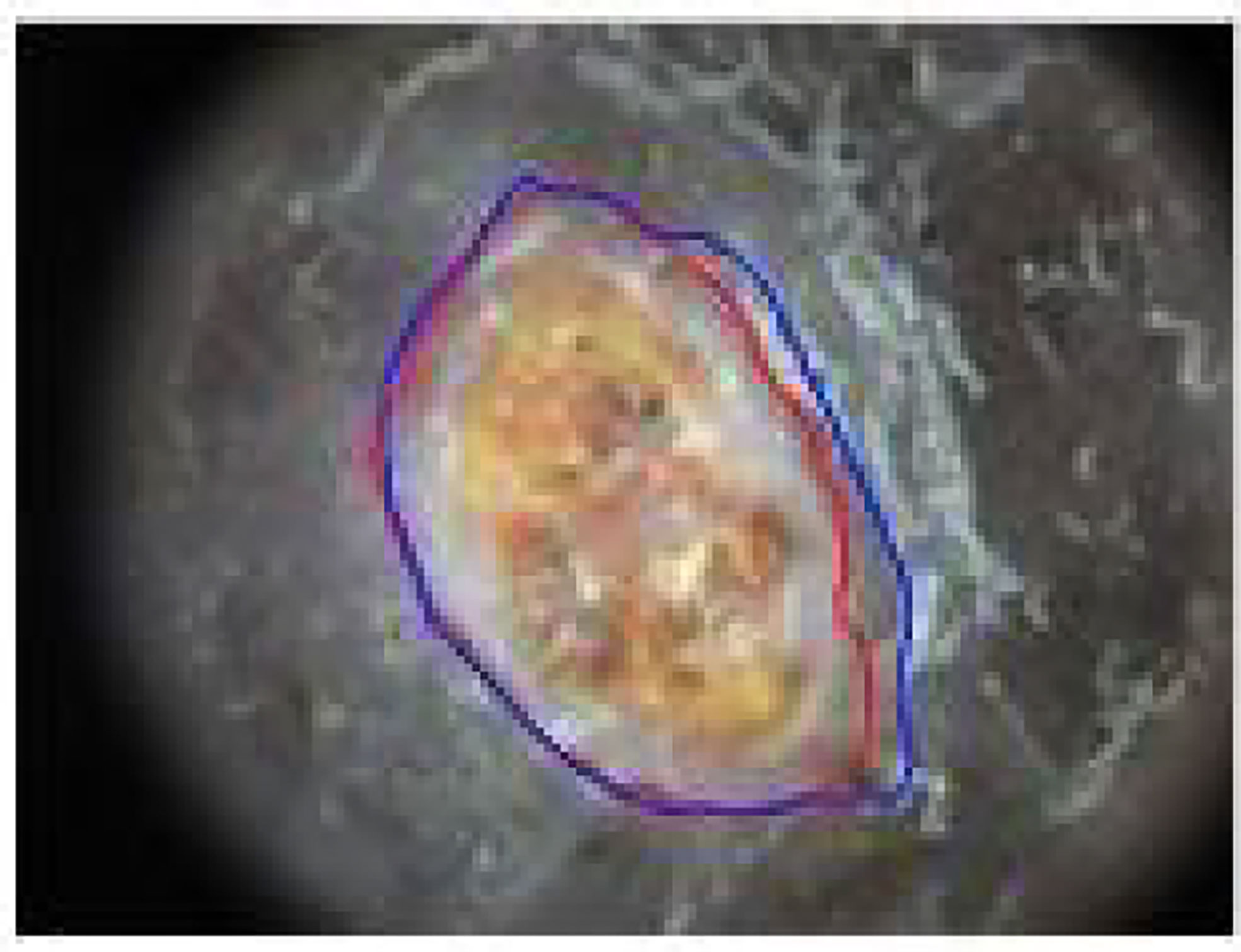
Figure 4 Example of BU lesion segmentation: The red contour is the automatic segmentation by our method and blue contour is the ground truth provided by an expert dermatologist.
The images that were ultimately included for this study were selected for inclusion by the collaborating physicians. Suboptimal images which were considered as out of focus, off center, improperly magnified, or did not include the full lesion were not included in the analysis for this study. This inclusion and exclusion criteria were established in effort to reduce the amount of noise in the data. This is the main research why the sample size for the study was relatively small.
Image preprocessing
In the preprocessing stage, images were first downsampled to 1080 × 810 pixels, and then processed with a 5 × 5 median filter and a Gaussian lowpass filter of the same size to remove extraneous artifacts and reduce the noise level.
Early analysis of the transfer function properties of the camera and dermoscope showed that even for a completely homogeneous white background, the light intensity varied radially in space with the center of the image captures being the brightest. Also, the average intensity level of the images captured under the four different colors of illumination varied across the different color images. To correct the nonuniform spatial image intensity and the overall average intensity of the images, we implemented normalization procedures to remove these elements of bias [ref].
To extract color features from the various images we defined various statistical parameters calculated from the different color channels after transforming the original RGB images in four additional color spaces, namely HSV, CIE L*a*b*, CIE L*u*v*, and YCbCr (14).
Lesion segmentation
The development of an accurate lesion border detection method is a critical step the development of the automated tools since it influences the precision by which all subsequent steps of image analysis are developed. The automatic segmentation method we developed is based on active contours and simply starts by considering the common foreground and background obtained by the luminance and color components as lesion and healthy skin, respectively, and then applies a supervised classifier to the remaining pixels. The procedure involves three main steps: contour initialization, contour evolution, and pixel classification. Contour initialization is based on fusion of the segmentations obtained from eight color channels by a voting system (24). Contour evolution relied on level set approaches implemented in both the color and luminance channels that provided two masks respectively. In general, the color-based mask focuses only on the central areas of the lesion, and misses areas close to the actual boundary, while the luminance-based mask includes part of normal skin due to the smooth transition. To overcome this problem, a SVM classifier is applied to the image pixel values from the RGB and L*u*v* color spaces to form a six-dimensional feature vector. A postprocessing morphological filtering was applied, and a distance transform (24) to make the lesion borders smoother.
Feature extraction
In general, features representing skin lesions include two categories, global features which describe an image as a whole, and local features which represent image patches (25). The former includes information on the shape, border, color, and texture properties of a lesion (26), whereas the for the latter we chose color moment and wavelet coefficients as patch descriptors to represent color and texture information respectively (27).
Lesion classification
Correct lesion classification relies on a bag-of-features representation of images which are loosely considered as collections of independent patches (25). The entire methodology relies on a few simple steps: sample a representative set of patches from an image, evaluate a descriptor vector for each patch independently, and use the resulting distribution of samples in the feature space to completely characterize the image. The bag-of-features framework consists of two stages, namely codebook creation and feature representation.
In the final step of the system, classification is performed using a Support Vector Machine (SVM) approach (28) which searches for the hyperplane that creates the biggest margin between the training points of two classes. We used a Gaussian Radial Basis Function nonlinear kernel which maps the feature vectors to a higher dimension feature space and thus can achieve better performance.
Results
System performance
The bag-of-feature approach used to build the classifier and the combination of color and texture provided a significant improvement in automatic detection of BU compared to any single patch descriptor. In total, we collected 562 sets of dermoscopic images. However, while 365 out of them were not usable because of bad image quality, including lesions out of focus, lesion off center or outside the view of field, or lesion was magnified improperly and occupied the whole field of view and were excluded from further analysis, we have access to two additional datasets with a total size of 58 + 197 images, including 32 BU confirmed samples. The result obtained using a subset of 58 white-light images with confirmed diagnosis (16 lesions BU and 42 lesions non-BU) resulted in sensitivity 100, specificity 88.10, and overall accuracy 94.05 at 95% CI. The performance obtained using a second dataset of 197 dermoscopic multispectral images (16 lesions BU and 181 lesions non-BU) resulted in sensitivity 90.00, specificity 93.39, and overall accuracy 91.69 at 95% CI.
An example of using the desktop-based application for automated Buruli recognition is shown in Figure 5. Once the image is loaded, the software implements all analysis steps automatically, including pre-processing, lesion segmentation, feature extraction, feature selection, and classification, and finally provides an estimate of the probability of the lesion being Buruli using a both a color bar and a numerical value.
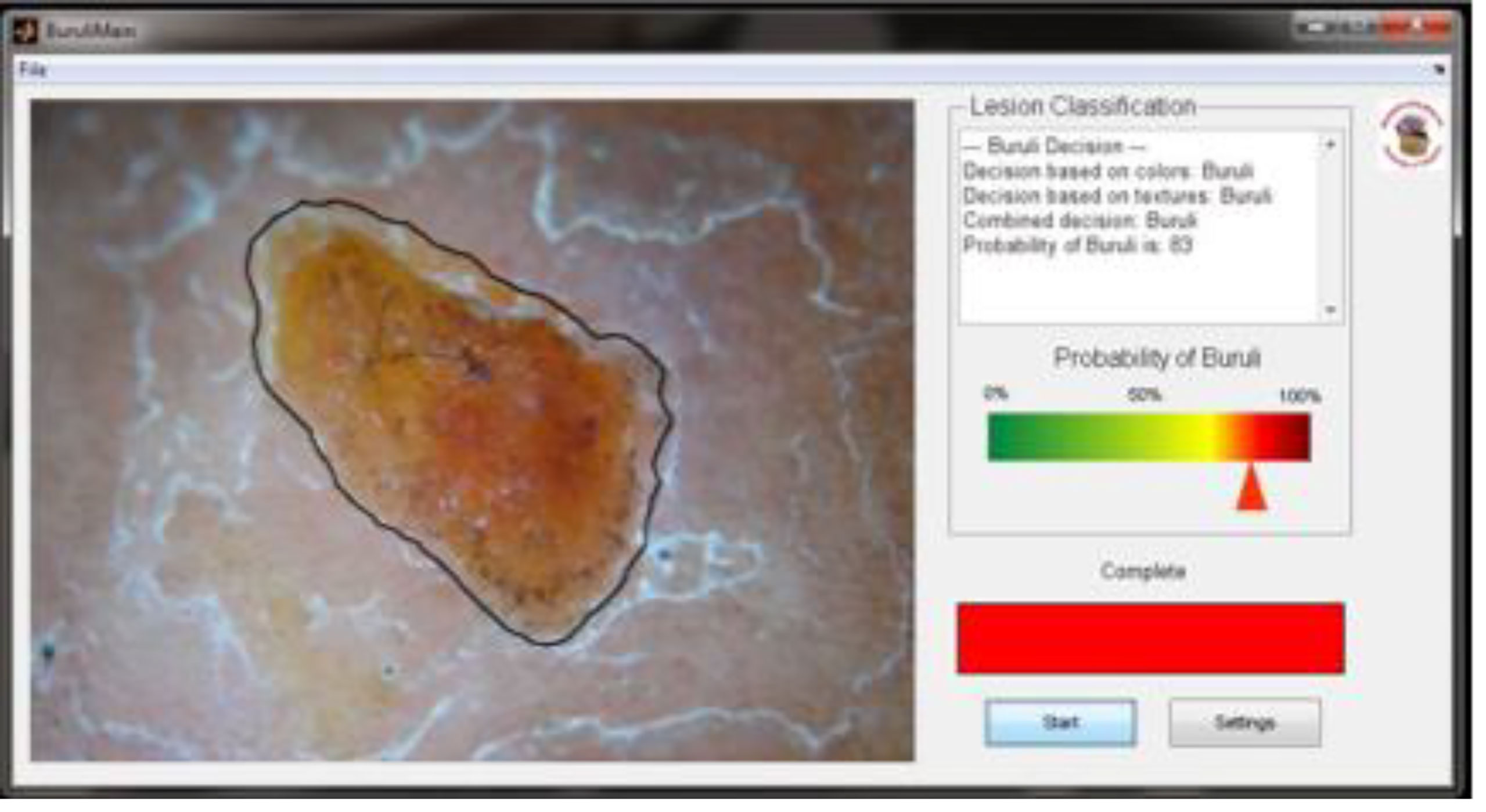
Figure 5 Example of automatic BU image analysis with estimation of the probability for a positive diagnosis.
Discussion
This is an important step for the development of mHealth tools for use by non-specialists in community settings for the early detection of BU, additional skin-NTDs, and other dermatologic conditions associated with disease, including wound healing and the self-management of disease progression. This system will continue to perform even as the technology evolves and newer dermoscopes are available. Subsequent studies involve the DL4 which provides more uniform and brighter illumination, higher lesion magnification, and wider field of view which, combined with the superb resolution of modern smartphones can result in faster and more accurate lesion assessment.
The authors of this study acknowledge the limitations of the small size of the data set which limits the statistical power of our study. However, this is the best dataset we can obtain at the moment, and we applied repeated cross validation to show the statistical significance and confidence interval. The balanced accuracy for the first dataset is 94.05% with the 95% CI from 90.44% to 96.13%; while the balanced accuracy for the second dataset is 91.69% with the 95% CI from 86.95% to 95.12%.
To improve upon this study, it is desirable to design a multicenter study that would include inviting more experts to be involved in the screening and assessment of images and ensuring improved quality of image data through improved training for those involved in the image acquisition. Each of these steps are critical for ensuring integrity of the data, but especially to build capacity for the eventually implementation and dissemination of the mHealth intervention.
This study is a valuable foundational step towards building a mHealth intervention for identifying Buruli ulcer disease in the early stages and when effective treatment is the most achievable. Future studies should include multicenter trials to include a greater number of participants, additional communities over a broader range of geographic locations, and additional Buruli ulcer-endemic countries. Additionally, there is a need to include observational studies that can contribute to the development of a surveillance system that includes geographic location, but also features of the surrounding environment, and important features of the affected patient such as location of the affected area, gender, and age.
The development of innovative methods for surveillance, screening, diagnosis, communication and management has the power to transform the delivery of health and medical care to otherwise hard-to-reach communities. While we have developed the automated tools for an mHealth intervention, the desktop version of our application can also provide a complete evaluation of skin lesion images. The advantages of having both hardware and software tools developed and available are for repository and algorithmic training purposes. Additionally, independent software development allows for deployment on future use tools such as together as a part of larger telehealth programming, eLearning, or platforms to support electronic medical records.
Data availability statement
The raw data supporting the conclusions of this article will be made available by the authors, without undue reservation.
Ethics statement
The studies involving human participants were reviewed and approved by IRB University of Houston, Ghana Health Service, and National Buruli Ulcer Control Program of Côte d’Ivoire. Written informed consent to participate in this study was provided by the participants’ legal guardian/next of kin.
Author contributions
GZ and CQ conceived the exploratory study. CQ collected the data. GZ and RH performed the analysis and interpretation of data. GZ and CQ contributed toward drafting the manuscript. RH developed the methods and analysis as a part of his Doctoral studies at the University of Houston. All authors contributed to the article and approved the submitted version.
Funding
The authors declare that this study received funding from the National Institutes of Health, NIH grant 5R21AR057921-02. The funder was not involved in the study design, collection, analysis, interpretation of data, the writing of this article or the decision to submit it for publication.
Acknowledgments
The authors would like to recognize our collaborators, William Opare, Mohammed Abass, and Edwin Ampadu, in Ghana and Henri Asse in Cote d’Ivoire, and the funding support of the National Institute of Arthritis and Musculoskeletal and Skin Diseases (NIAMS), National Institutes of Health, grant 5R21AR057921-02.
Conflict of interest
Patents US10593040B2 and US 8,213,695 are associated with this research and authors, CQ and GZ, have founded a company, Advanced Codex Solutions, LLC, however no royalties or income are received related to this research.
Publisher’s note
All claims expressed in this article are solely those of the authors and do not necessarily represent those of their affiliated organizations, or those of the publisher, the editors and the reviewers. Any product that may be evaluated in this article, or claim that may be made by its manufacturer, is not guaranteed or endorsed by the publisher.
References
1. Ryu S. Book review: mHealth: New horizons for health through mobile technologies: Based on the findings of the second global survey on eHealth (Global observatory for eHealth series, volume 3). Healthc Inform Res (2012) 18(3):231. doi: 10.4258/hir.2012.18.3.231
2. World Health Organization. Frequently asked questions on global task force on digital health for TB and its work . Available at: http://www.who.int/tb/areas-of-work/digital-health/faq/en/webcite.
5. Situ N, Yuan X, Chen J, Zouridakis G. Malignant melanoma detection by bag-of-features classification. In: 2008 30th annual international conference of the IEEE engineering in medicine and biology society. VANCOUVER, BC, CANADA: IEEE (2008) 3110–3.
6. Wadhawan T, Situ N, Rui H, Lancaster K, Yuan X, Zouridakis G. Implementation of the 7-point checklist for melanoma detection on smart handheld devices. In: 2011 annual international conference of the IEEE engineering in medicine and biology society. BOSTON, MASSACHUSETTS, USA: IEEE (2011) 3180–3.
7. Situ N, Yuan X, Zouridakis G, Mullani N. Automatic segmentation of skin lesion images using evolutionary strategy. In: 2007 IEEE international conference on image processing. SAN ANTONIO, TEXAS, USA: IEEE (2007) 6:VI–277.
8. Yuan X, Situ N, Zouridakis G. A narrow band graph partitioning method for skin lesion segmentation. Pattern Recognit (2009) 42(6):1017–28. doi: 10.1016/j.patcog.2008.09.006
9. Wadhawan T, Situ N, Lancaster K, Yuan X, Zouridakis G. SkinScan: a portable library for melanoma detection on handheld devices. In: 2011 IEEE international symposium on biomedical imaging: From nano to macro. CHICAGO, ILLINOIS, USA: IEEE (2011) 133–6.
10. Yuan X, Yang Z, Zouridakis G, Mullani N. SVM-based texture classification and application to early melanoma detection. In: 2006 international conference of the IEEE engineering in medicine and biology society. NEW YORK, NEW YORK, USA: IEEE (2006) 4775–8.
11. Recognizing neglected tropical diseases through changes in the skin: a training guide for front-line health workers. Geneva: World Health Organization (2018).
12. Hu R, Queen CM, Zouridakis G. Detection of buruli ulcer disease: preliminary results with dermoscopic images on smart handheld devices. In: 2013 IEEE Point-of-Care Healthcare Technologies (PHT). BANGALORE, INDIA: IEEE (2013) 168–71.
13. Hu R, Queen CM, Zouridakis G. Lesion border detection in buruli ulcer images. In: 2012 annual international conference of the IEEE engineering in medicine and biology society. SAN DIEGO, CALIFORNIA, USA: IEEE (2012) 5380–5383).
14. Zouridakis G, Wadhawan T, Situ N, Hu R, Yuan X, Lancaster K, et al. Melanoma and other skin lesion detection using smart handheld devices. In: Mobile health technologies. SAN DIEGO, CALIFORNIA, USA: Humana Press (2015) 459–96.
15. Hu R, Queen C, Zouridakis G. A novel tool for detecting buruli ulcer disease based on multispectral image analysis on handheld devices. In: IEEE-EMBS international conference on biomedical and health informatics (BHI) VALENCIA, SPAIN: IEEE (2014) 37–40.
16. Lorentzen H, Weismann K, Petersen CS, Grønhøj Larsen F, Secher L, Skødt V. Clinical and dermatoscopic diagnosis of malignant melanoma: assessed by expert and non-expert groups. Acta Dermato-Venereologica (1999) 79(4):301–304. doi: 10.1080/000155599750010715
17. Buntine J, Crofts K, World Health Organization, Global Buruli Ulcer Initiative. Buruli ulcer: Management of mycobacterium ulcerans disease: A manual for health care providers. World Health Organization (2001).
18. Molyneux DH, Savioli L, Engels D. Neglected tropical diseases: progress towards addressing the chronic pandemic. Lancet (2017) 389(10066):312–25. doi: 10.1016/S0140-6736(16)30171-4
19. Muhi S, Stinear TP. Systematic review of m. bovis BCG and other candidate vaccines for buruli ulcer prophylaxis. Vaccine (2021) 39(50):7238–52. doi: 10.1016/j.vaccine.2021.05.092
20. Yotsu RR, Kouadio K, Vagamon B, N’guessan K, Akpa AJ, Yao A, et al. Skin disease prevalence study in schoolchildren in rural cote d’Ivoire: Implications for integration of neglected skin diseases (skin NTDs). PloS Negl Trop Dis (2018) 12(5):e0006489. doi: 10.1371/journal.pntd.0006489
21. Velding K, Klis SA, Abass KM, Tuah W, Stienstra Y, van der Werf T. Wound care in buruli ulcer disease in Ghana and Benin. Am J Trop Med Hygiene (2014) 91(2):313. doi: 10.4269/ajtmh.13-0255
22. Eddyani M, Sopoh GE, Ayelo G, Brun LV, Roux JJ, Barogui Y, et al. Diagnostic accuracy of clinical and microbiological signs in patients with skin lesions resembling buruli ulcer in an endemic region. Clin Infect Dis (2018) 67(6):827–34. doi: 10.1093/cid/ciy197
23. Hu R, Queen C, Zouridakis G. A novel tool for detecting Buruli ulcer disease based on multispectral image analysis on handheld devices. In IEEE-EMBS International Conference on Biomedical and Health Informatics (BHI). Valencia, Spain: IEEE (2014) pp.37–40.
24. Hu R. Automatic recognition of buruli ulcer images on smart handheld devices (Doctoral dissertation). Electricial and computer engineering, cullen college of engineering, University of houston. (2013).
25. Csurka G, Dance C, Fan L, Willamowski J, Bray C. Visual categorization with bags of keypoints. In: Workshop on statistical learning in computer vision, ECCV (2004) 1:1–2.
26. Mindru F, Tuytelaars T, Van Gool L, Moons T. Moment invariants for recognition under changing viewpoint and illumination. Comput Vision Image Understanding (2004) 94(1-3):3–27. doi: 10.1016/j.cviu.2003.10.011
27. Situ N, Wadhawan T, Hu R, Lancaster K, Yuan X, Zouridakis G. Evaluating sampling strategies of dermoscopic interest points. In: 2011 IEEE international symposium on biomedical imaging: From nano to macro. BOSTON, MASSACHUSETTS, USA: IEEE (2011) 109–112.
Keywords: buruli ulcer, mHealth, early detection, skin NTDs, mycobacterium ulcerans
Citation: Queen CM, Hu R and Zouridakis G (2023) Towards the development of reliable and economical mHealth solutions: A methodology for accurate detection of Buruli ulcer for hard-to-reach communities. Front. Trop. Dis 3:1031352. doi: 10.3389/fitd.2022.1031352
Received: 29 August 2022; Accepted: 24 November 2022;
Published: 04 January 2023.
Edited by:
Tjip S van der Werf, University of Groningen, NetherlandsReviewed by:
Sammy Ohene-Aboagye, Rush University, United StatesAlejandro Llanos-Cuentas, Universidad Peruana Cayetano Heredia, Peru
Copyright © 2023 Queen, Hu and Zouridakis. This is an open-access article distributed under the terms of the Creative Commons Attribution License (CC BY). The use, distribution or reproduction in other forums is permitted, provided the original author(s) and the copyright owner(s) are credited and that the original publication in this journal is cited, in accordance with accepted academic practice. No use, distribution or reproduction is permitted which does not comply with these terms.
*Correspondence: Courtney M. Queen, Courtney.m.queen@ttuhsc.edu