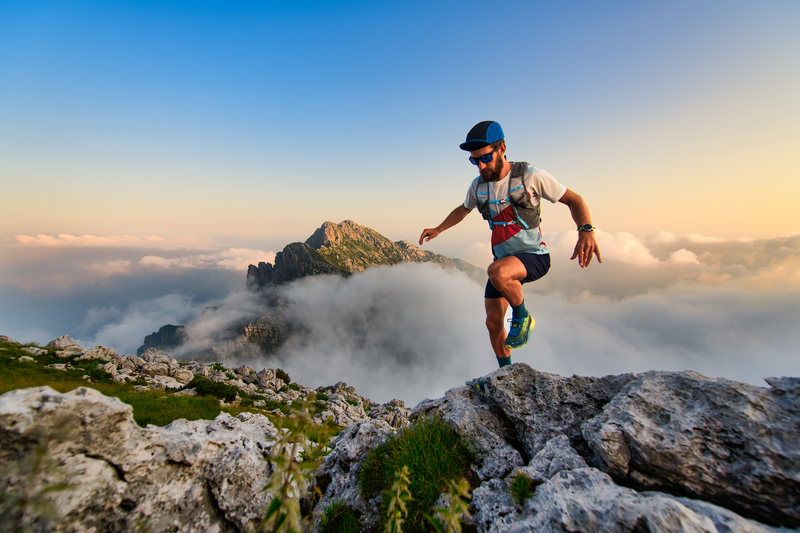
95% of researchers rate our articles as excellent or good
Learn more about the work of our research integrity team to safeguard the quality of each article we publish.
Find out more
ORIGINAL RESEARCH article
Front. Transplant. , 18 December 2024
Sec. Thoracic Transplantation
Volume 3 - 2024 | https://doi.org/10.3389/frtra.2024.1508419
The acute rejection (AR) diagnosis depends on transbronchial biopsy, which is semi-invasive and not easily performed. Our study used the Nanostring gene expression technology on PBMCs obtained from lung transplant recipients (LTRs) to search for biomarkers. We identified distinct differential gene profiles between patients with stable status (STA) and AR. Subsequently, we independently evaluated monocyte compositions in PBMCs using flow cytometry and assessed the levels of 7 chemokines in serum using Luminex. The 48 top differentially expressed genes (DEGs) were identified, utilizing a criterion of at least a 1.5-fold change between two groups, with a false discovery rate (FDR) p-Adj < 0.05. Of these 48 genes, the top 10 genes with the highest fold changes and significant p-values were selected for qPCR validation. CD68, ANXA1, ITGB, and IFI30 can be confirmed among the validated genes. A significantly lower percentage of CD14 + CD16- classical monocytes was observed in AR than in STA patients, which aligns with downregulated DEGs. Many of the DEGs were related to monocytes-macrophages and chemokines. Although these results still need to be confirmed in larger cohorts, they suggest that gene profiling of PBMC can help to identify markers related to AR in LTRs.
Lung transplantation (LTx) is a lifesaving option for patients with advanced lung disease for whom therapies are exhausted. Acute rejection (AR) is a life-threatening complication following graft transplantation, which is mainly caused by the cellular responses to human leukocyte antigens (HLAs) on the allograft and leads to 3.6% of deaths during the first month (1, 2). The incidence rate of AR varies around 50% in 5 years post-LTx, and approximately 28% of LTRs encountered at least one rejection episode within the initial year (3, 4). Additionally, frequent AR episodes may lead to chronic rejection, which is also one of the risk factors for developing chronic lung allograft dysfunction (CLAD) (5, 6). CLAD has been regarded as the leading cause of poor survival post-LTx (7). Early detection and diagnosis of AR could significantly influence the course of treatment and ultimately improve the chances of a successful outcome.
AR may present asymptomatic but also with clinical presentations such as fever, cough, dyspnea, and severe respiratory syndrome (4, 8, 9). Infection is a common complication post-LTx. Therefore, it may be challenging to distinguish AR from infection because of the similarity of the symptoms (10). Diagnosing AR is essential and depends on transbronchial biopsy in lung tissue (11–13). However, this is a semi-invasive procedure that is not easily performed and is accompanied by complications, such as oversedation, pneumothorax, and bleeding (14). Therefore, a noninvasive approach, measuring biomarkers in blood to diagnose AR, could be helpful.
Gene expression profiling has been performed in lung transplantation recipients (LTRs), mainly using bronchoalveolar lavage (BAL) samples (15). Previous research on genetic biomarkers was reported by Danger et al., who performed microarray analysis in peripheral blood mononuclear cells (PBMCs) and validated POU2AF1, TCL1A, and BLK as predictors of bronchiolitis obliterans syndrome (BOS) more than 6 months before diagnosis (16). However, similar studies for characterizing AR in LTRs have not been done so far.
In our current study, we used the NanoString® nCounter® Analysis System (NanoString Technologies, Seattle, WA, USA) with a human organ transplant Panel in PBMCs for gene profiling in LTRs with which the expression of 770 genes can be analyzed. With this technique, differential gene expression profiles have been reported in kidney (17), heart (18), lung (19), and pancreas (20) transplantation. In this study, we conducted a comparative gene expression analysis between individuals diagnosed with AR and those in stable status (STA) after LTx. The primary aim of this study was to identify potential minimally invasive biomarkers of AR to improve the clinical management of LTx patients.
PBMC samples of LTRs were selected from November 2016 until May 2022 at the University Medical Center Groningen (UMCG), the Netherlands. The local ethical committee approved the study (METc 2016/090), and all enrollments provided written informed consent. Twenty-four LTRs were selected and divided into 2 groups: (1) AR (n = 15), who had an occurrence of AR 1–89 months after LTx, and (2) STA (n = 9) were selected by age-matched and same follow-up time post-transplantation with AR patients, who were in a stable status after LTx until May 2022. On days 1 and 5 after LTx, LTRs were treated with Basiliximab. Maintenance therapy includes tacrolimus, mycophenolate mofetil (MMF), azathioprine (Aza), and prednisolone. The local pathologist assigned the AR patients following the International Society for Heart and Lung Transplantation (ISHLT) guidelines.
Blood was drawn for AR and STA patients, and serum was collected within 0–3, 3–6, 6–12, and more than 12 months after LTx. The PBMCs were isolated and stored in liquid nitrogen until use.
PBMCs were thawed and checked for viability above 85% by trypan blue staining. Total RNA was extracted from PBMCs using the RNeasy® Kit (QIAGEN, Hilden, Germany) with the RNase-free DNase Set (QIAGEN, Hilden, Germany) manufacturers' protocols. RNA was collected in PCR tubes (AmpliStar-II PCR Tubes, Westburg) and stored at −80 °C until use. The quantity was measured with a Nanodrop 1000 system (Thermo Scientific, USA), and the quality was evaluated with the Agilent 4200 TapeStation system (Agilent Technologies, Waldbronn, Germany). Only samples with an RNA integrity number above 5.5 were used for the Nanostring analysis.
NanoString gene expression profiling (NanoString Technologies, Seattle, WA, USA) was performed according to suppliers instructions (21). In brief, 50 ng of RNA was incubated with capture and reported probes (Human Organ Transplant Panel, XT-CSO-HOT1-12) for 16–24 h at 65 °C. An aliquot was loaded on a SPRINT cartridge for detection and analyzed on a nCounter® SPRINT Profiler platform. The platform for subsequent gene expression analyses generated reporter code count (RCC) files.
Whole content normalization of the raw data was performed using nSolver 4.0 Analysis Software, utilizing the Code Set Content Normalization factors. Subsequently, differential expression gene analysis were performed by using nSolver 4.0 software according to the instructions (NanoString Technologies, MAN-C0019-08) and ROSALIND® (San Diego, CA) (https://rosalind.onramp.bio/). Simultaneously, the normalized data was exported in comma-separated value (CSV) format for further analyses. Principal Component Analysis (PCA) and heatmap visualization were conducted using R studio software (version 4.0.2). PCA visualization was performed using the R package ggplot2, and heatmap visualization was performed with the package heatmap. Calculation of fold changes and p-values for differential genes was using the fast method described in the nCounter® Advanced Analysis 2.0 User Manual. The genes with a false discovery rate (FDR) adjusted p-value <0.05 and fold change (FC) ≥1.5 or ≤−1.5 were considered as differentially expressed genes. The Kyoto Encyclopedia of Genes and Genomes (KEGG) pathway was evaluated using DAVID Bioinformatics Resources (NIAID/NIH, USA) by uploading the differential expressed genes. The regulated pathways were selected with an adjusted p-value <0.05.
The remaining RNA from NanoString gene expression analysis was used for qPCR validation. The reverse transcriptase (Invitrogen, USA) was used for cDNA synthesis and Taqman qPCR system (Applied Biosystems, USA) was used for the amplification according to advanced universal SYBR green mastermix instruction. We selected the following Taqman qPCR primers (Taqman, USA): CD68 (Hs00154355_m1), ANXA1 (Hs00167549_m1), ITGB2 (Hs00164957_m1), IFI30 (Hs00173838_m1), LTBR (Hs01101194_m1), CCR1 (Hs00174298_m1), FKBP1A (Hs00356621_g1), MYD88 (Hs00182082_m1), TNFRSF1A (Hs01042313_m1), TNFRSF1B (Hs00961750_m1). Besides, RPLP0 (Hs99999902_m1), GAPDH (Hs99999905_m1), and B2M (Hs99999907_m1) were used as reference gene primers for normalization (22). The household genes showed no significant differences between the AR and STA samples. The data was analyzed using QuantStudioTM Real-Time PCR System (USA). The 2−ΔCt method was used to calculate the relative expression.
Levels of the chemokines CCL2, CX3CL1, CXCL10, CXCL16, CXCL6, CXCL8, and CXCL1 in serum were measured by using the Luminex Discovery Assay (Biotechne, R&D systems, USA) according to the manufacturer's instructions. Subsequently, the assay results were obtained using a Luminex Magpix instrument, and the data were analyzed using Xponent 4.2 software (Bio-Techne, R&D Systems, USA).
For the monocytes analysis, PBMCs were thawed and stained with antibodies specific for HLA-DR (clone: TU36, BD), CD14 (clone: rmC5-3, BD), CD16 (clone: B73.1, BD). The cells were measured, and data acquisition was performed using the Symphony flow cytometer (BD). 500.000 events per sample were recorded. The data was processed using the analysis software Kaluza (Beckman Coulter, USA) with a gating strategy as described (23) in the Supplementary Figure 1. To eliminate potentially contaminating lymphocytes from the monocyte gate, negative cells for both HLA-DR and CD14 were excluded.
Continuous data are presented as median and range. Differences between the two groups were tested using Mann–Whitney U test. Additionally, differences between the clinical parameters among STA and AR groups were analyzed using a Chi-square test. Data analysis was performed using IBM SPSS statistics version 23.0, and figures were created with GraphPad Prism version 9.0. Statistical significance was defined as p-value <0.05 (two-tailed).
Initially, RNA samples from 24 participants were selected (Figure 1). Subsequently, 21 samples meeting the quality criteria were categorized into AR (n = 13) and STA (n = 8) patients. Table 1 provides an overview of the baseline characteristics of these 21 participants, which are included for further analysis. While age and sex differences between the two groups were not statistically significant, it is noteworthy that the AR group had slightly younger patients and a higher proportion of males than the STA group.
AR manifested at a median of 14.6 months (1–89 months) post-LTx, with PBMC sampling occurring at a median of 34 days (ranging from 1 to 83 days) after AR onset. Of note, 2 of 13 AR patients had AR more than one year after LTx, after they had received a dose reduction of the medication due to toxicity. PBMCs from AR and STA patients were isolated 15.7 (1–89) months and 4.0 (1.0–8.4) months after LTx, respectively.
COPD/emphysema was the leading cause of LTx in 61.5% of STA patients and 50% of AR patients. Seven of 8 STA and all AR patients received immunosuppressive treatment consisting of basiliximab induction (anti-CD25; 2 doses of 20 mg) followed by Tacrolimus (Tac), MMF, and prednisolone (Pred) maintenance therapy. MMF was switched to Azathioprine if side effects happened. A single patient in the STA group was initially treated with Tac-MMF-Pred and subsequently transitioned to a regimen of Tac-Azathioprine (Aza)-Pred. Treatment of AR patients consisted of pulse methylprednisolone (1 g intravenously for 3 days).
NanoString analysis was performed to identify differentially expressed genes between AR and STA patients. After normalization of the data, PCA analysis of the 770 analyzed genes showed significant differences in expression between the STA and AR groups (Figure 2A, p-value = 0.009). A volcano plot (Figure 2B) demonstrated increased expression for 7 genes and decreased expression for 41 genes by at least a 1.5-fold change in the AR group as compared to the STA group at an FDR-adjusted p-value < 0.05 (Table S.1). The normalized counts of these highly differentially expressed genes were visualized in a heatmap (Figure 2C). Eleven pathways with a significant adjusted p-value <0.05 in KEGG pathway analysis were observed (Figure 2D). Of note was the NF-kappa B signaling pathway (p < 0.001), which involved 11 out of 48 differential expression genes. Of the 11 genes, 9 were downregulated (CXCL2, TNFSF14, NFIL13, BCL2A1, CD14, TNFRSF1A, TLR4, LTBR, MYD88) and 2 were upregulated (CD40LG, LTA).
Figure 2. Differential expression genes. (A) Principal Component Analysis (PCA) comparing the expression profiles of 770 genes in the AR (green dots) and STA (red dots) groups. (B) The volcano plot displays transcripts that are upregulated (green dots) and downregulated (pink dots) genes with a false discovery rate (FDR) adjusted p-value ≤0.05 and a log2 fold change >1.5 in the AR group compared to the STA group (STA used as the baseline). (C) Heatmap illustrating the 48 differential expression of genes distinguishing the AR (green) and STA (red) groups. The p-value was calculated by independent t-test between PC1 and PC2 using R software. (D) KEGG pathway descriptors. The orange column represents the numeric -log10 p-value, blue column represents the percentage of involved genes of all 48 differential expression genes. The pathways were selected by adjusted p-value <0.05 of KEGG pathways evaluated in DAVID Bioinformatics Resources system.
The top 10 differentially expressed genes (highest fold changes with significant p-values) between the STA and AR groups were chosen for qPCR validation: CD68, ANXA1, ITGB, IFI30, LTBR, CCR1, FKBP1A, MYD88, TNFRSF1A, TNFRSF1B were downregulated in AR compared STA group. Expression of CD68 (p = 0.0017), ANXA1 (p = 0.0001), ITGB (p = 0.0063), and IFI30 (p = 0.0047) was significantly decreased in the AR patients compared to the STA patients (Figure 3). Also, FKBP1A, TNFRSF1A, and TNFRSF1B were decreased in AR, but no significance was reached. In contrast, expression of CCR1 was upregulated in AR compared to STA patients (p = 0.0017).
Figure 3. Validation of differential expressed genes. Validation of differential expression genes of CD68, ANXA1, ITGB, IFI30, LTBR, CCR1, FKBP1A, MYD88, TNFRSF1A, TNFRSF1B by quantitative PCR in the same patients set for Nanostring gene expression. Differences between the two groups were tested using the Mann–Whitney U test.
As shown in Supplementary Table S1, we identified differential expression of several chemokines, specifically CXCL16 and CXCL1. Subsequently, we performed an independent evaluation study with 30 serum samples from LTRs, comprising 15 STA and 15 AR patients, matched for age and post-LTx time (as detailed in Supplementary Table S2). We extended the analysis to include other relevant chemokines: CCL2, CX3CL2, CXCL10, CXCL8, and CXCL6. However, the lower expression of CXCL16 and CXCL1 could not be confirmed at the protein level. Surprisingly, CXCL6 levels were significantly higher in AR patients compared to STA patients (Figure 4, p = 0.0292).
Figure 4. Analysis of chemokines in serum. An independent validation of extended Chemokines (CXCL1, CXCL6, CXCL8, CXCL10, CXCL16, CX3CL1, and CCL2) using Luminex for Comparing AR and STA Patients.
Because CD14 and CD68 are in the list of differentially expressed genes highly expressed by monocyte lineage cells (24, 25), we further assessed frequencies of monocytes in PBMCs of AR and STA groups by flow cytometry. Among the patients selected for chemokine analysis, 10 STA and 10 AR patients had PBMC samples available for monocyte analysis using flow cytometry. Monocytes were categorized as classical (CD14+, CD16-), intermediate (CD14+, CD16+), and non-classical (CD14−, CD16+) monocytes. As demonstrated in Figure 5, there was a significantly reduced frequency of CD14 + CD16− classical monocytes (p = 0.0232) in the AR group compared to STA.
Figure 5. An independent validation of monocytes in PBMCs. Validation of CD14 + CD16− classical monocytes, CD14 + CD16 + intermediate monocytes, and CD14 + CD16− classical monocytes by flow cytometry comparing AR and STA patients.
In our study, we profiled PBMCs to identify AR-associated genes in LTRs. We validated four genes (CD68, ANXA1, ITGB, IFI30) statistically differentiating AR and STA patients with qPCR. Additionally, our cellular analysis of monocytes highlighted significant distinctions of reduced classical monocyte CD14 + CD16- frequencies in AR patients and the upregulation of CXCL6 levels compared to STA patients.
We observed a significantly reduced expression of CD68 in AR patients in gene expression analysis. CD68, the human homolog of macrosialin, is commonly regarded as a lineage marker for human monocytes and macrophages (24). In PBMCs, CD68 is not expressed on monocytes except when they differentiate towards macrophages. Classification of monocytes in PBMCs can be further analyzed by expression of CD14 (LPS co-receptor) and CD16 (Fcγ receptor III) that define three phenotypically and functionally distinct human monocyte subsets: CD14 + CD16− (classical), CD14 + CD16+ (intermediate), and CD14-CD16+ (non-classical) monocytes. Until now, no studies have reported the relation between monocyte compositions and AR after LTx. Our results indicate that the CD14 + CD16− classical monocytes percentage was lower in AR than in STA patients. In previous studies, the experimental depletion of monocytes in murine heart and kidney transplantation models demonstrated a reduction in T cell-mediated rejection (26). Additionally, in a study of murine LTx, CD4 T cells from recipients lacking circulating classical monocytes were shielded from allorecognition, but AR in the allografts persisted (27). This evidence suggests a potential inter-connection between lower levels of monocytes and AR post-transplantation.
Furthermore, the classical monocytes can differentiate into macrophages in tissue (28), which can occur during inflammatory conditions (29). The reduced classical monocyte percentage in PBMCs of AR patients implies there may be more monocyte-to-macrophage differentiation in AR development, resulting in enhanced migration of these monocytes to the tissue and thereby reduced levels of these monocytes. This suggests that AR patients may have a heightened capacity for monocyte transformation into tissue-resident macrophages, emphasizing the importance of inflammation in rejection. Due to the lack of lung tissues from AR patients, we could not further validate macrophages in tissue. Nevertheless, it has been demonstrated that by employing immunohistochemical double staining of CD68 and CD31, the accuracy of diagnosing antibody-mediated rejection in heart transplantation can be enhanced (30). Also, a study using a rat model of LTx found that CD68 + macrophages were the predominant cell type observed during AR through immunohistochemistry (31).
What's more, a study utilizing PROMAD identifies myeloid cells as the origin of acute rejection markers, emphasizing their critical role and immunologic memory in modulating transplant rejection, supported by evidence of CD16 + monocyte/macrophage prevalence in rejecting heart transplants and resident macrophages shaping lung allograft immune responses (32).
Macrophages are crucial in acute antibody-mediated rejection (AMR) and cellular rejection (ACR). Their presence in rejected allograft tissue is related to poorer graft function and survival (33). Notably, corticosteroids in immunosuppressive treatments may alter monocyte subpopulation distribution. Still, therapies involving MMF, calcineurin inhibitors, and mammalian target of rapamycin inhibitors (mTOR) have not shown significant effects in this regard (34).
Furthermore, we cross-referenced our differentially expressed genes (DEG) data with related organ transplantation studies. CD14, IFI30, TNFRSF1B, CD27, and ITGB2 emerged as significant DEG associated with AR, aligning with our findings (35). To our knowledge, no subsequent validation studies have been conducted on these genes related to AR. Of note, ANXA1, a member of the Annexins (ANXs) family, known for its calcium and phospholipid binding properties, has been related to lung injury and inflammation (36). The lower expression of ANXA1 in AR patients compared to STA patients may be attributed to its anti-inflammatory role. IFI30 (GILT), a lysosomal thiol reductase, plays a role in catalyzing the reduction of disulfide bonds within protein antigens. This process aids antigen-presenting cells (APCs) in effectively presenting antigens to T cells while downregulating regulatory T cells (Tregs) (37, 38). Our findings of lower expression of IFI30 in AR patients might signify a potential reduction in immune tolerance. In a differential gene expression study involving mucosal biopsies of rejection and stable patients in LTx, ITGB2 was identified as a hub gene with theoretical significance in the inhibition of rejection, as revealed in drug-gene interaction analysis (39). Our findings align with this, suggesting that ITGB2 could also be a potential biomarker.
We also noticed the potential involvement of chemokines, particularly CXCL6, in AR. This corresponds with findings in kidney transplantation, where CXCL6 levels were higher in the AR group compared to the non-AR group (40). In a liver transplantation study, increased secretion of CXCL1 and CXCL2 was observed, which can boost the functional activity of the mononuclear phagocyte system and possibly contribute to processes associated with liver rejection (41).
Our KEGG analysis implied the significant involvement of the NF-kappa B signaling pathway during AR. Several organ transplantation studies have shown that NF-kappa B signaling activation can lead to rejection, and inhibiting NF-kappa B in specific cell types may enhance graft survival in solid organ allograft rejection (42–44). After transplantation, NF-kappa B activation occurs shortly after ischemia/reperfusion and is later reactivated within infiltrating cells during AR. The literature underscores the role of NF-kappa B activation in the detrimental effects of ischemia/reperfusion, the survival of activated T cells, differentiation of various effector T cell types, memory T cell formation, and dendritic cell maturation. Notably, NF-kappa B activation may counteract the peripheral development of Tregs, which can suppress the immune response against allografts and prolong graft survival (44, 45). Furthermore, the activation of NF-kappa B signaling plays a pivotal role in generating natural Tregs, underscoring its significance in the context of solid organ allograft rejection (46).
In a kidney transplantation study, the effects of sample timing and treatment on gene expression were shown in early AR (47). This emphasizes the importance of moment when assessing gene expression during AR. Future research should study biomarkers at different time points post-LTx to identify essential genes in the process of AR.
The main limitation of our study is the relatively small cohort size. Additionally, not all samples, especially from AR patients, were available at every time point due to patient no-shows and participation in other clinical studies, contributing to the unavailability of some samples at specific time points. Further studies were recommended to validate these with simplified samples.
In summary, by using minimally invasive samples, we identified significant differentially expressed genes. CD68, ANXA1, ITGB, and IFI30 were successfully validated, suggesting involvement in AR-related immune response and inflammation. Further research should reveal their precise roles as potential diagnostic or prognostic markers for AR. Additionally, our results shed light on the possible role of monocyte compositions and the NF-kappa B signaling pathway in AR development. These findings hold promise for aiding clinicians in identifying novel biomarkers to enhance the accuracy of diagnosing AR.
The original contributions presented in the study are included in the article/Supplementary Material, further inquiries can be directed to the corresponding author.
The studies involving humans were approved by the local ethical committee approved the study (METc 2016/090), and all enrollments provided written informed consent. The studies were conducted in accordance with the local legislation and institutional requirements. Written informed consent for participation in this study was provided by the participants' legal guardians/next of kin.
SL: Software, Validation, Visualization, Writing – original draft, Writing – review & editing, Conceptualization, Data curation, Formal Analysis, Funding acquisition, Investigation, Methodology. JW: Conceptualization, Data curation, Formal Analysis, Funding acquisition, Investigation, Methodology, Project administration, Resources, Software, Supervision, Validation, Visualization, Writing – original draft, Writing – review & editing. SH: Data curation, Formal Analysis, Methodology, Software, Writing – original draft, Writing – review & editing. EV: Data curation, Methodology, Resources, Supervision, Writing – original draft, Writing – review & editing. LV: Conceptualization, Data curation, Formal Analysis, Methodology, Software, Writing – original draft, Writing – review & editing. DV: Conceptualization, Data curation, Formal Analysis, Investigation, Methodology, Software, Supervision, Validation, Visualization, Writing – original draft, Writing – review & editing. NB: Conceptualization, Data curation, Formal Analysis, Funding acquisition, Investigation, Methodology, Project administration, Resources, Software, Supervision, Validation, Visualization, Writing – original draft, Writing – review & editing.
The author(s) declare financial support was received for the research, authorship, and/or publication of this article. This work was financially supported by the Chinese Scholarship Council (CSC NO. 201909110111), the University Medical Center Groningen (UMCG), and the De Cock-Hadders Foundation.
We want to express our gratitude to Dr. Wayel H. Abdulahad for his invaluable assistance with the design of the flow cytometry experiment.
The authors declare that the research was conducted in the absence of any commercial or financial relationships that could be construed as a potential conflict of interest.
The author(s) declare that no Generative AI was used in the creation of this manuscript.
All claims expressed in this article are solely those of the authors and do not necessarily represent those of their affiliated organizations, or those of the publisher, the editors and the reviewers. Any product that may be evaluated in this article, or claim that may be made by its manufacturer, is not guaranteed or endorsed by the publisher.
The Supplementary Material for this article can be found online at: https://www.frontiersin.org/articles/10.3389/frtra.2024.1508419/full#supplementary-material
1. Liu SQ, Bos NA, Verschuuren EAM, van Baarle D, Westra J. Biological characteristics of HLA-G and its role in solid organ transplantation. Front Immunol. (2022) 13:902093. doi: 10.3389/fimmu.2022.902093
2. Benzimra M, Calligaro GL, Glanville AR. Acute rejection. J Thorac Dis. (2017) 9(12):5440–57. doi: 10.21037/jtd.2017.11.83
4. Parulekar AD, Kao CC. Detection, classification, and management of rejection after lung transplantation. J Thorac Dis. (2019) 11:S1732–S9. doi: 10.21037/jtd.2019.03.83
5. Kesten S, Maidenberg A, Winton T, Maurer J. Treatment of presumed and proven acute rejection following six months of lung transplant survival. Am J Respir Crit Care Med. (1995) 152(4 Pt 1):1321–4. doi: 10.1164/ajrccm.152.4.7551389
6. Werlein C, Seidel A, Warnecke G, Gottlieb J, Laenger F, Jonigk D. Lung transplant pathology: an overview on current entities and procedures. Surg Pathol Clin. (2020) 13(1):119–40. doi: 10.1016/j.path.2019.11.003
7. Yoshiyasu N, Sato M. Chronic lung allograft dysfunction post-lung transplantation: the era of bronchiolitis obliterans syndrome and restrictive allograft syndrome. World J Transplant. (2020) 10(5):104–16. doi: 10.5500/wjt.v10.i5.104
8. Martinu T, Howell DN, Palmer SM. Acute cellular rejection and humoral sensitization in lung transplant recipients. Semin Resp Crit Care. (2010) 31(2):179–88. doi: 10.1055/s-0030-1249113
9. Costa J, Benvenuto LJ, Sonett JR, Edwin C, Weiskopf AK. Long-term outcomes and management of lung transplant recipients. Best Pract Res-Clin. (2017) 31(2):285–97. doi: 10.1016/j.bpa.2017.05.006
10. De Vlaminck I, Martin L, Kertesz M, Patel K, Kowarsky M, Strehl C, et al. Noninvasive monitoring of infection and rejection after lung transplantation. Proc Natl Acad Sci USA. (2015) 112(43):13336–41. doi: 10.1073/pnas.1517494112
11. Potestio C, Jordan D, Kachulis B. Acute postoperative management after lung transplantation. Best Pract Res Clin Anaesthesiol. (2017) 31(2):273–84. doi: 10.1016/j.bpa.2017.07.004
12. Telenga ED, van der Bij W, de Vries EFJ, Verschuuren EAM, Timens W, Luurtsema G, et al. (99m)Tc-HYNIC-IL-2 scintigraphy to detect acute rejection in lung transplantation patients: a proof-of-concept study. EJNMMI Res. (2019) 9(1):41. doi: 10.1186/s13550-019-0511-z
13. Harris B, Lowy FD, Stover DE, Arcasoy SM. Diagnostic bronchoscopy in solid-organ and hematopoietic stem cell transplantation. Ann Am Thorac Soc. (2013) 10(1):39–49. doi: 10.1513/AnnalsATS.201212-114FR
14. Du Rand IA, Blaikley J, Booton R, Chaudhuri N, Gupta V, Khalid S, et al. British Thoracic society guideline for diagnostic flexible bronchoscopy in adults: accredited by NICE. Thorax. (2013) 68:1–44. doi: 10.1136/thoraxjnl-2013-203618
15. Reilly C, Cervenka T, Hertz MI, Becker T, Wendt CH. Human neutrophil peptide in lung chronic allograft dysfunction. Biomarkers. (2011) 16(8):663–9. doi: 10.3109/1354750X.2011.623789
16. Danger R, Royer PJ, Reboulleau D, Durand E, Loy J, Tissot A, et al. Blood gene expression predicts bronchiolitis obliterans syndrome. Front Immunol. (2017) 8:1841. doi: 10.3389/fimmu.2017.01841
17. Sigdel T, Nguyen M, Liberto J, Dobi D, Junger H, Vincenti F, et al. Assessment of 19 genes and validation of CRM gene panel for quantitative transcriptional analysis of molecular rejection and inflammation in archival kidney transplant biopsies. Front Med (Lausanne). (2019) 6:213. doi: 10.3389/fmed.2019.00213
18. Kim JYV, Lee B, Koitsopoulos P, Shannon CP, Chen V, Hollander Z, et al. Analytical validation of HEARTBiT: a blood-based Multiplex gene expression profiling assay for exclusionary diagnosis of acute cellular rejection in heart transplant patients. Clin Chem. (2020) 66(8):1063–71. doi: 10.1093/clinchem/hvaa123
19. Gohir W, Klement W, Singer LG, Palmer SM, Mazzulli T, Keshavjee S, et al. Identifying host microRNAs in bronchoalveolar lavage samples from lung transplant recipients infected with Aspergillus. J Heart Lung Transpl. (2020) 39(11):1228–37. doi: 10.1016/j.healun.2020.07.014
20. Roufosse C, Drachenberg C, Renaudin K, Willicombe M, Toulza F, Dominy K, et al. Molecular assessment of antibody-mediated rejection in human pancreas allograft biopsies. Clin Transplant. (2020) 34(11):e14065. doi: 10.1111/ctr.14065
21. M'Boutchou MN, van Kempen LC. Analysis of the tumor microenvironment transcriptome via NanoString mRNA and miRNA expression profiling. Methods Mol Biol. (2016) 1458:291–310. doi: 10.1007/978-1-4939-3801-8_21
22. Dheda K, Huggett JF, Bustin SA, Johnson MA, Rook G, Zumla A. Validation of housekeeping genes for normalizing RNA expression in real-time PCR. Biotechniques. (2004) 37(1):112. doi: 10.2144/04371RR03
23. Jiemy WF, van Sleen Y, van der Geest KS, Ten Berge HA, Abdulahad WH, Sandovici M, et al. Distinct macrophage phenotypes skewed by local granulocyte macrophage colony-stimulating factor (GM-CSF) and macrophage colony-stimulating factor (M-CSF) are associated with tissue destruction and intimal hyperplasia in giant cell arteritis. Clin Transl Immunol. (2020) 9(9):e1164. doi: 10.1002/cti2.1164
24. Gottfried E, Kunz-Schughart LA, Weber A, Rehli M, Peuker A, Muller A, et al. Expression of CD68 in non-myeloid cell types. Scand J Immunol. (2008) 67(5):453–63. doi: 10.1111/j.1365-3083.2008.02091.x
25. Kapellos TS, Bonaguro L, Gemund I, Reusch N, Saglam A, Hinkley ER, et al. Human monocyte subsets and phenotypes in major chronic inflammatory diseases. Front Immunol. (2019) 10:2035. doi: 10.3389/fimmu.2019.02035
26. Oberbarnscheidt MH, Zeng Q, Li Q, Dai H, Williams AL, Shlomchik WD, et al. Non-self recognition by monocytes initiates allograft rejection. J Clin Invest. (2014) 124(8):3579–89. doi: 10.1172/JCI74370
27. Gelman AE, Okazaki M, Sugimoto S, Li W, Kornfeld CG, Lai J, et al. CCR2 Regulates monocyte recruitment as well as CD4+T allorecognition after lung transplantation. Am J Transplant. (2010) 10(5):1189–99. doi: 10.1111/j.1600-6143.2010.03101.x
28. Narasimhan PB, Marcovecchio P, Hamers AAJ, Hedrick CC. Nonclassical monocytes in health and disease. Annu Rev Immunol. (2019) 37:439–56. doi: 10.1146/annurev-immunol-042617-053119
29. Lara S, Akula S, Fu Z, Olsson AK, Kleinau S, Hellman L. The human monocyte-A circulating sensor of infection and a potent and rapid inducer of inflammation. Int J Mol Sci. (2022) 23(7):3890. doi: 10.3390/ijms23073890
30. Glass C, Butt YM, Gokaslan ST, Torrealba JR. CD68/CD31 Immunohistochemistry double stain demonstrates increased accuracy in diagnosing pathologic antibody-mediated rejection in cardiac transplant patients. Am J Transplant. (2019) 19(11):3149–54. doi: 10.1111/ajt.15540
31. Jungraithmayr W, Inci I, Bain M, Hillinger S, Korom S, Weder W. Distribution of macrophages and T cells in syngrafts and allografts after experimental rat lung transplantation. Immunobiology. (2010) 215(3):206–14. doi: 10.1016/j.imbio.2009.04.003
32. Robertson H, Kim HJ, Li J, Robertson N, Robertson P, Jimenez-Vera E, et al. Decoding the hallmarks of allograft dysfunction with a comprehensive pan-organ transcriptomic atlas. Nat Med. (2024). doi: 10.1038/s41591-024-03030-6
33. van den Bosch TPP, Kannegieter NM, Hesselink DA, Baan CC, Rowshani AT. Targeting the monocyte-macrophage lineage in solid organ transplantation. Front Immunol. (2017) 8:153. doi: 10.3389/fimmu.2017.00153
34. Svachova V, Krupickova L, Novotny M, Fialova M, Mezerova K, Cecrdlova E, et al. Changes in phenotypic patterns of blood monocytes after kidney transplantation and during acute rejection. Physiol Res. (2021) 70(5):709–21. doi: 10.33549/physiolres.934700
35. Spivey TL, Uccellini L, Ascierto ML, Zoppoli G, De Giorgi V, Delogu LG, et al. Gene expression profiling in acute allograft rejection: challenging the immunologic constant of rejection hypothesis. J Transl Med. (2011) 9:174. doi: 10.1186/1479-5876-9-174
36. Ampomah PB, Kong WT, Zharkova O, Chua S, Perumal Samy R, Lim LHK. Annexins in influenza virus replication and pathogenesis. Front Pharmacol. (2018) 9:1282. doi: 10.3389/fphar.2018.01282
37. Li H, Wang Y, Ma M, Hu L, Zhang X, Xin L, et al. GILT in tumor cells improves T cell-mediated anti-tumor immune surveillance. Immunol Lett. (2021) 234:1–12. doi: 10.1016/j.imlet.2021.04.001
38. Rausch MP, Hastings KT. Diverse cellular and organismal functions of the lysosomal thiol reductase GILT. Mol Immunol. (2015) 68(2 Pt A):124–8. doi: 10.1016/j.molimm.2015.06.008
39. Xiu MX, Liu ZT, Tang J. Screening and identification of key regulatory connections and immune cell infiltration characteristics for lung transplant rejection using mucosal biopsies. Int Immunopharmacol. (2020) 87:106827. doi: 10.1016/j.intimp.2020.106827
40. Krupickova L, Fialova M, Novotny M, Svachova V, Mezerova K, Cecrdlova E, et al. Chemokine profiles are affected in serum of patients with acute rejection of kidney allograft. Mediat Inflamm. (2021) 2021:5513690. doi: 10.1155/2021/5513690
41. Li SP, Li XQ, Chen XJ, Zhang JM, Zhou GP, Zhou LX, et al. Characterization and proteomic analyses of proinflammatory cytokines in a mouse model of liver transplant rejection. Oxid Med Cell Longev. (2022) 2022:5188584. doi: 10.1155/2022/5188584
42. Csizmadia V, Gao W, Hancock SA, Rottman JB, Wu Z, Turka LA, et al. Differential NF-kappaB and IkappaB gene expression during development of cardiac allograft rejection versus CD154 monoclonal antibody-induced tolerance. Transplantation. (2001) 71(7):835–40. doi: 10.1097/00007890-200104150-00003
43. Ma LL, Xiang ZD, Sherrill TP, Wang L, Blackwell TS, Williams P, et al. Bioluminescence imaging visualizes activation of nuclear factor-kappa B in mouse cardiac transplantation. Transplantation. (2008) 85(6):903–10. doi: 10.1097/TP.0b013e318166cde1
44. Molinero LL, Alegre ML. Role of T cell-nuclear factor kappa B in transplantation. Transplant Rev-Orlan. (2012) 26(3):189–200. doi: 10.1016/j.trre.2011.07.005
45. Atif M, Conti F, Gorochov G, Oo YH, Miyara M. Regulatory T cells in solid organ transplantation. Clin Transl Immunol. (2020) 9(2):e01099. doi: 10.1002/cti2.1099
46. Schmidt-Supprian M, Courtois G, Tian J, Coyle AJ, Israel A, Rajewsky K, et al. Mature T cells depend on signaling through the IKK complex. Immunity. (2003) 19(3):377–89. doi: 10.1016/S1074-7613(03)00237-1
Keywords: acute rejection, lung transplantation, gene expression, monocytes, NanoString
Citation: Liu S, Westra J, Hu S, Verschuuren EAM, van Kempen LC, van Baarle D and Bos NA (2024) Gene expression profiling in PBMCs for acute rejection in lung transplant recipients reveals myeloid responses. Front. Transplant. 3:1508419. doi: 10.3389/frtra.2024.1508419
Received: 9 October 2024; Accepted: 9 December 2024;
Published: 18 December 2024.
Edited by:
Letizia Corinna Morlacchi, Fondazione IRCCS Ca' Granda Ospedale Maggiore Policlinico di Milano, ItalyReviewed by:
Baptiste Lamarthée, Université de Franche-Comté, EFS, INSERM, UMR RIGHT, FranceCopyright: © 2024 Liu, Westra, Hu, Verschuuren, van Kempen, van Baarle and Bos. This is an open-access article distributed under the terms of the Creative Commons Attribution License (CC BY). The use, distribution or reproduction in other forums is permitted, provided the original author(s) and the copyright owner(s) are credited and that the original publication in this journal is cited, in accordance with accepted academic practice. No use, distribution or reproduction is permitted which does not comply with these terms.
*Correspondence: Nico A. Bos, bi5hLmJvc0B1bWNnLm5s
Disclaimer: All claims expressed in this article are solely those of the authors and do not necessarily represent those of their affiliated organizations, or those of the publisher, the editors and the reviewers. Any product that may be evaluated in this article or claim that may be made by its manufacturer is not guaranteed or endorsed by the publisher.
Research integrity at Frontiers
Learn more about the work of our research integrity team to safeguard the quality of each article we publish.