- 1Division of Gastroenterology, Toronto General Hospital, Toronto, ON, Canada
- 2Ajmera Transplant Center, University Health Network, Toronto, ON, Canada
- 3Department of Innovation, University Health Network, Toronto, ON, Canada
- 4Department of Laboratory Medicine and Pathobiology, The Temerty Centre for AI Research and Education in Medicine, Toronto, ON, Canada
- 5Department of Computer Science, University of Toronto, Toronto, ON, Canada
With recent advancements in deep learning (DL) techniques, the use of artificial intelligence (AI) has become increasingly prevalent in all fields. Currently valued at 9.01 billion USD, it is a rapidly growing market, projected to increase by 40% per annum. There has been great interest in how AI could transform the practice of medicine, with the potential to improve all healthcare spheres from workflow management, accessibility, and cost efficiency to enhanced diagnostics with improved prognostic accuracy, allowing the practice of precision medicine. The applicability of AI is particularly promising for transplant medicine, in which it can help navigate the complex interplay of a myriad of variables and improve patient care. However, caution must be exercised when developing DL models, ensuring they are trained with large, reliable, and diverse datasets to minimize bias and increase generalizability. There must be transparency in the methodology and extensive validation of the model, including randomized controlled trials to demonstrate performance and cultivate trust among physicians and patients. Furthermore, there is a need to regulate this rapidly evolving field, with updated policies for the governance of AI-based technologies. Taking this in consideration, we summarize the latest transplant AI developments from the Ajmera Transplant Center’s inaugural symposium.
1 Introduction
Since its first description in the 1950s by Alan Turing, there has been huge interest in the applicability of artificial intelligence (AI) in clinical practice, particularly given its potential to analyze large datasets (1). However, progress in the medical field had remained limited until the early 2000s, when the development of deep learning (DL) methods, which involved the creation of artificial neural networks, extended the capability of AI for data analysis beyond a fixed model of pattern recognition inputted by a human operator, to developing its own representations in a learning algorithm, recognizing new patterns among vast quantities of unstructured data (1–3). This sparked a wave of studies exploring the utility of DL in the identification of novel patterns, which could assist in improving diagnostic techniques and the prediction of therapeutic responses. In 2017, Arterys was the first healthcare cloud-based DL application to be approved by the US Food and Drug Administration (FDA). Arterys uses cardiac magnetic resonance imaging (MRI) exams to estimate ventricular function (4). To date, there have been 694 approved AI-based medical applications (5). It is a rapidly growing market, reaching a value of 9.01 billion USD in 2023, with a forecasted compound annual growth rate of 40% (6).
In line with these developments, there has been an array of research exploring the versatility of AI in different areas of healthcare. Within the clinical setting, DL models have demonstrated utility in aiding clinical decision-making, processing and combining multi-omics profiles with clinical, demographic, and epidemiologic data and diagnostic results, thereby enabling the practice of precision medicine, in which treatment is tailored to the specific needs of the individual, rather than being confined to standardized “one-size fits all” protocols (7–9). In addition, AI-based tools have been used to improve diagnostic testing, assisting with medical imaging and histopathology review, which has improved patient monitoring methods, engagement, and compliance (10, 11). The prospective benefits have been shown to extend to all aspects of healthcare, including medical education, research, development, and even healthcare organization, in which it has been shown to improve workflow and resource utilization, reducing costs and medical errors (7, 12, 13).
However, despite the potential for revolutionizing healthcare, the successful deployment and implementation of DL models in day-to-day practice is not without its challenges. Essentially, the models must be derived using robust datasets obtained from the target population, be well calibrated, and undergo extensive validation. This is critical as erroneous assumptions can adversely impact management and lead to harm. One notable example was observed with the external validation of the Epic Sepsis Prediction Model in the US (14). Furthermore, there are few randomized clinical trials and prospective studies in DL, and peer-reviewed publications are not a prerequisite for FDA approval (15). Assessing the reproducibility of DL research is also challenging due to limitations in the availability and accessibility of the datasets and codes from which the models are generated (15). In addition, AI-based models are generated within the confines of existing published data, which have significant age, gender, and racial biases (16–18). This inability to extrapolate beyond the inputted data comes with a significant risk of amplifying existing biases and disparities in healthcare (15–18).
Transplant medicine is a nuanced specialist area, with many variables at play. At present, there is room for significant improvements in our delivery of care, including prioritization fairness in the organ allocation process, optimizing donor-recipient matching, improving graft survival, and practicing precision medicine by tailoring immunosuppression levels to reduce the risk of rejection while addressing metabolic risk factors to improve long-term outcomes.
In this position paper, we detail the proceedings from the Ajmera Transplant Center’s inaugural Transplant AI Symposium, summarizing the latest advancements in the use of AI-based tools, current challenges, barriers to implementation, ethical considerations, and future directions aimed at modernizing and improving care for transplant recipients.
2 Symposium highlights
2.1 The future of AI in transplant
The inaugural symposium was opened by the keynote speaker, Dr. Alexandre Loupy, from the Necker Hospital in Paris. Introducing a multimodal approach to the use of AI in transplantation, he detailed his stepwise approach from the research and development of DL models to extensively validated decision support systems that can be successfully incorporated in day-to-day clinical practice.
Dr. Loupy highlighted the three main pillars of his AI journey. The first was centered around the development of the multimodal prognostication system for allograft loss in renal transplant recipients (iBox) in 2012, using a prospectively derived comprehensively phenotyped cohort of renal transplant recipients (19–21). The prognostication system incorporates data that are easily accessible in all transplant centers, such as donor and patient characteristics, medical history, histopathology, treatment regimens, and longitudinal clinical parameters. Designing the model exclusively for the renal transplant recipient helped increase the specificity of the algorithm, and evaluating it in a diverse patient population improved the generalizability of the model. During development, there was extensive internal validation, including a methodological validation of the statistical model required to generate the prognostic scoring system, guaranteeing a causal DL model, with parameters used for scoring being directly related to independent predictors of long-term allograft failure in renal transplant recipients. This was followed by an external independent validation in multiple transplant centers worldwide, with subsequent involvement in numerous randomized controlled trials (RCTs) demonstrating the translatability and relevance in geographically distinct populations. The model was subsequently involved in numerous RCTs (see Table 1) and has received endorsement from transplant societies and the European Medicines Agency (EMA), and is currently under review with the FDA. The iBox system currently involves data from 27,000 patients across 44 centers, in 14 different countries spanning 5 continents. The iBox score is now validated as a surrogate of long-term allograft survival and is used as a clinical endpoint in clinical trials. Work is under way to develop an equivalent pediatric model.
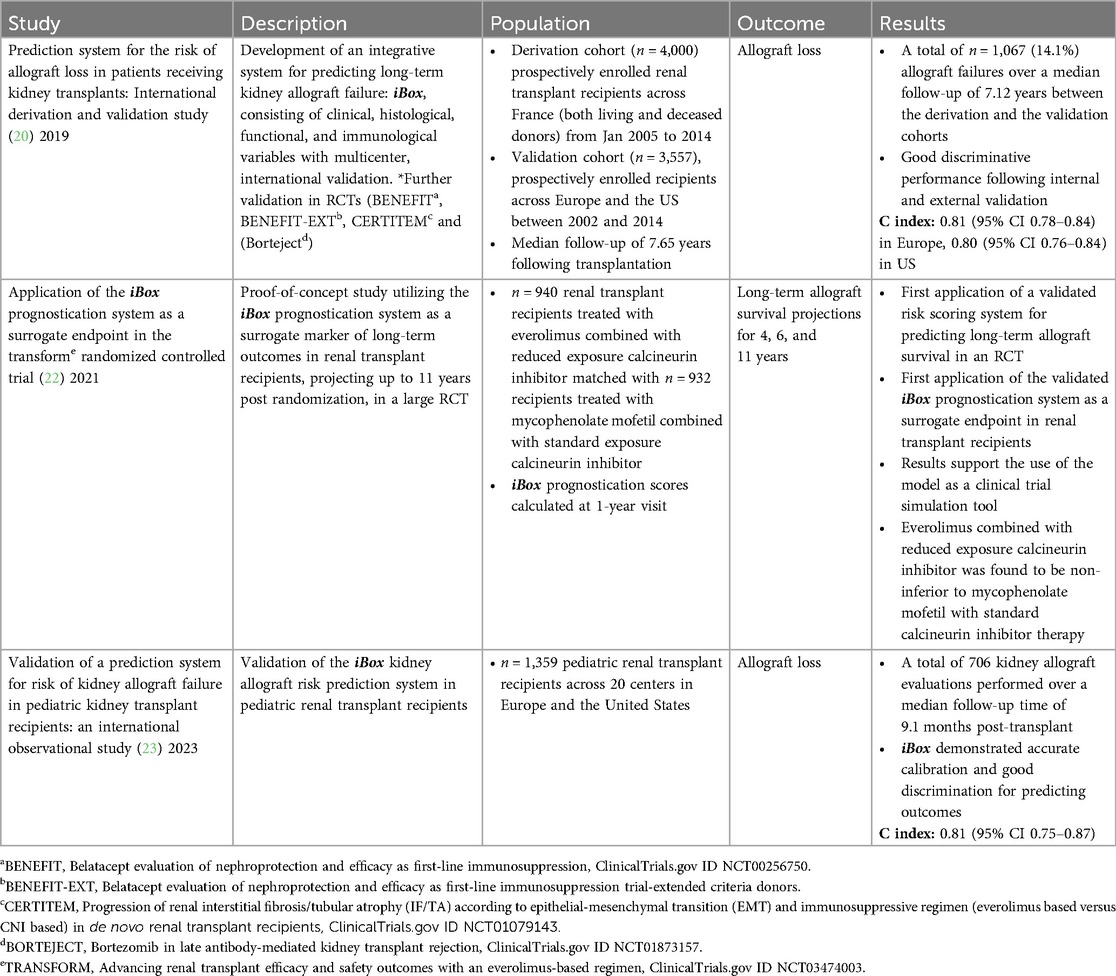
Table 1. Summary of validation studies and randomized controlled trials involving the iBox prognostication system.
The second pillar builds on the first, incorporating data on HLA antibodies with novel biomarkers of graft pathology, including immunological markers (21, 24–28) and donor-derived cell free (DD-CF) DNA (24, 26, 27, 29–32), in addition to novel molecular technologies (27, 33–38), which enable more detailed allograft phenotyping in a multimodal algorithm-based decision support system (32, 39–43). There is currently an ongoing multicenter prospective RCT (the EU-TRACER IMPACT study) involving eight European transplant referral centers assessing whether the integration of these additional models, including DD-CF DNA, can further increase the model’s accuracy in predicting allograft failure and patient mortality.
Finally, the last pillar focuses on using AI to improve diagnostics and disease classification through precision diagnostic platforms that incorporate digital histological analysis, including digital spatial profiling and single-cell pathology with diagnostic techniques involving the “molecular microscope” system and non-invasive biomarkers, such as DD-CF DNA. This multidimensional approach to diagnostics has already helped amend the Banff diagnostic classification of antibody-mediated rejection (ABMR) in renal transplant recipients (35, 44), and pursuing a multidimensional approach to diagnostics holds further promise, including in improving precision diagnostics for xenotransplant recipients.
Dr. Andrew T. Sage, from the Latner Thoracic Research Laboratories, then discussed the development of the InsightX AI model (Transplant Hepatology and Machine Learning Departments, University of Toronto, Toronto, ON, Canada) for assessing organ injury and predicting patient outcomes during lung transplantation. Organ availability remains a significant issue in transplantation, not excepting individuals awaiting a lung transplant, with over 110,000 people in the US on the waiting list, which has a person added to it every 9 min (45). Considerable demand in the face of a supply shortage has a significant impact on waitlist mortality, with 17 people on the lung transplant waiting list dying daily (45). Lung allografts tend to be particularly susceptible to ischemia-reperfusion injury, highlighting the importance of optimizing organ preservation (46). This has led to the use of ex vivo lung perfusion (EVLP) to help restore lung physiology, reducing the rates of ischemia-reperfusion injury, and facilitate the assessment of impaired donor lungs (47). Interestingly, the machine also provides a unique platform for obtaining serial physiological, biochemical, and imaging data. Dr. Sage and his team compiled longitudinal measurements recording over 100 variables from donor lungs, including cytokine production, lactate, electrolytes, airway pressures, vascular resistance, and dynamic and static compliance. These high-resolution data profiles were then used to create the first AI model for ex vivo organs, InsightX, which aimed to improve the assessment of organ suitability, donor-recipient matching, and post-transplant outcomes (48). The long short-term memory (LSTM) model was trained using 725 clinical cases and consisted of an ensemble of decision trees in a gradient boosting framework, making it more adaptable to handling missing values while ensuring high interpretability and performance efficiency. Three outcome classifications were used: time to extubation post-transplant <72 h, ≥72 h, and unsuitable for transplant. The model performed well in the prediction of post-transplant outcomes, achieving an area under the receiver operator curve (AUROC) of 79% ± 3% in the training dataset, with AUROCs of 75% ± 4% and 85 ± 3% in the independent test sets, with an AUROC of 90% ± 4% for the correct identification of unsuitable donor lungs (48). The team further developed the model with the addition of recipient data and used data intensive in silico models otherwise known as digital twins to run accurate simulations and allow for precise treatment selection. The additional data further improved the predictive accuracy of the model, resulting in no significant differences between the predicted and observed outcomes (p = 0.88). The results have been extremely promising, enabling more accurate identification of unsuitable organs and optimal donor-recipient matching, and improving clinical outcomes, and the team are currently working on further validation following the incorporation of radiographic data.
Although the 1-year survival of liver transplant recipients has improved over the last 30 years, long-term survival has remained largely unchanged (49, 50). Mortality beyond 1 year is predominantly due to issues with graft failure, cardiovascular events, and de novo and recurrent cancers (49, 50). This clinical conundrum motivated MB and her team to design DynaComp (Transplant Hepatology and Machine Learning Departments, University of Toronto, Toronto, ON, Canada), a personalized risk calculator for the long-term management of transplant recipients. Longitudinal data incorporating 267 clinical variables and 5 outcome measures were obtained from the SRT and used in the development of the training model, including overall survival, death by graft failure, death by infection, cardiac events, and cancer (51). Of the preliminary models, the Transformer model (Transplant Hepatology and Machine Learning Departments, University of Toronto, Toronto, ON, Canada) demonstrated the greatest predictive accuracy, with overall AUROCs for 1- and 5-year survival of 0.77 and 0.711, respectively. The AUROCs for survival compromised by cardiovascular disease, cancer, infection, and graft failure were between 0.80–0.81. Shapley additive explanations (SHAP) analysis was performed to determine the relative impact of each variable on the model output, revealing the highest weighted variables to be: time since transplantation, donor and recipient age, etiology of liver disease, and body parametric data including weight and BMI, in addition to standard laboratory results. Following analysis, the results are displayed in the form of a personalized dashboard, projecting estimations of 1- and 5-year survival, including a breakdown risk of death from graft failure, cardiovascular events, infections, and cancer. The model can serve as an invaluable prompt for physicians in clinic, guiding them to react to risks, adjust immunosuppression, and initiate primary prevention measures, with the added advantage of allowing them to assess the impact of the therapeutic modifications on subsequent visits, ultimately improving the preservation of graft health and overall survival.
2.2 Clinical deployment of machine learning tools: practical and ethical considerations in transplant
The afternoon session was opened by Professor Doug Simonetto from the Mayo Clinic, who spoke about the development of an electrocardiogram (EKG)-enabled machine learning model designed to improve prognostication in chronic liver disease. Advanced liver disease is associated with the evolution of cardiomyopathy and cirrhotic cardiomyopathy, which lead to specific EKG changes (52). Professor Simonetto and his group worked on a binary classification model with a convoluted neural network (CNN) for the identification of cirrhosis using 12-lead EKGs. The generated output was identified as an AI cirrhosis EKG (ACE) score, a continuous value ranging from 0 and 1, reflecting the estimated strength of the “cirrhosis” signal from each EKG (53). Data from 5,212 liver transplant recipients who had cirrhosis listed as an indication for transplantation and had a digitized EKG prior to transplant, combined with 20,728 age- and sex-matched controls, were used for the training, testing, and validation cohorts. Following analysis, an AUROC of 0.98 was achieved for the prediction of cirrhosis in the testing cohort, with a sensitivity of 84.9% and a specificity of 83.2%. When adjustments were made for one-to-one matching, including comorbidities, the performance of the model did not significantly change, with an AUROC of 0.893. Following on from this, the team looked to see whether there were any longitudinal changes in the score and determined that the ACE score continued to increase from 5 years before transplant, reaching a peak immediately prior to transplantation, after which it significantly declined. On this basis, the team proceeded to explore whether the score could have utility in prognostication and predict the future risk of decompensation and death. They obtained data from 500 patients with compensated cirrhosis predominantly due to metabolic liver disease (alcohol misuse and metabolic-dysfunction associated steatohepatitis), who had longitudinal follow-up that included EKGs. The model demonstrated an AUROC of 0.932 for the prediction of decompensation, with a sensitivity of 88.4% and specificity of 83.9 % (54). Following logistic regression, the team found that for every 0.1 increase in the ACE score, there was a 4.8× increase in the odds of clinical decompensation after adjusting for the MELD-Na score. The team then split the ACE scores into quartiles and assessed 1- and 5-year survival. Patients in the top quartile with ACE scores ranging from 0.75–1 had a >50% 1-year mortality, reaching almost 100% at 5 years, whereas those in the lowest quartile with ACE scores ranging from 0–0.25 had a <10% 5-year mortality. In the fully adjusted model, each 0.1 increase in the ACE score was associated with a 42% higher risk of liver-related death. The model performed well in predicting the risk of decompensation and death in individuals with compensated chronic liver disease.
Dr. Mike Brudno, chief data scientist at the University of Toronto, then provided an entertaining illustration of the challenges when training DL models, in particular the dangers of a “black box” classification model, using the team’s latest experience developing a model for the identification of pneumothoraxes from chest radiographs as an example. From initial iteration, the model had been devised using 7,000 images obtained from University Health Network (UHN) cases, of which 1,000 cases were positive for pneumothoraxes and 6,000 were negative. The model had initially been trained using a segmentation approach; however, this did not perform well, with a low Dice similarity coefficient score (coefficient of similarity between the “predicted” DL image and the “true” image ranging from 0 to 1) of 0.49, indicating a less than 50% chance of the model correctly identifying a pneumothorax, with a high standard deviation of 0.28 (55). Reviewing the initial images, the team noted that there was huge variability in the pneumothorax “negative” radiographs, with not enough data to apply the segmentation method; as a result, they used new data to update the model and switched to a classification-based method of analysis. In addition, the team modified the aim of the model to identify high-risk radiographs for the attention of radiologists, assisting with workflow prioritization. Testing the new iteration from 2,200 UHN scans, the AUROC of the model was 87.12%, with a sensitivity of 61.9% and specificity of 91.44%. Although the performance appeared acceptable, the team used gradient-weighted class activation mapping (Grad-CAM) to identify the x-ray regions the model focused on and deemed essential for the prediction of a pneumothorax in an image-based CNN model. They noted that the model was drawing incorrect inferences when using chest drains (the treatment for pneumothoraxes) or the presence of chest leads (usually associated with more acutely unwell patients) for its predictions. In essence, the model was erroneously determining causation from association. The team then adopted a multistep model; the first step involving the identification and removal of patients with chest drains and the second step involving the identification of pneumothoraxes. This really highlighted the importance of assessing explainability with clinically applied DL models.
The final talk of the day was given by Dr. Joseph Cafazzo, the executive director of biomedical engineering at the UHN, on the importance of human factors in the successful deployment of AI-based tools. Although there has been a plethora of products devised specifically for the healthcare industry, many have not passed the test of usability by failing to incorporate the consideration of human factors during the design phase. Human factors relate to the application of what is known about human capabilities and limitations from cognitive, behavioral, and environmental perspectives, in the design of the world we live in, to enable safe and productive lives. In particular, there tends to be a lack of empathy in the design of many healthcare tools. The challenge when it comes to the conception of these models is being mindful that it is not just a product but an experience that is being designed. One successful example includes the design of Medly (Centre for Digital Therapeutics, Toronto, ON, Canada), an application developed with Dr. Heather Ross that facilitates self-monitoring for patients with heart failure. Since its launch in 2016, the application has empowered patients and their families to take on a more active role in their health, improved quality of life, clinical outcomes, and healthcare-associated costs with a 50% reduction in the number of hospitalizations due to heart failure, and has demonstrated continued good uptake and adherence from the older patient population (56, 57). This demonstrates the potential impact of deploying practically useful AI tools on saving lives and reducing healthcare system costs.
3 Discussion
AI is a rapidly evolving area in healthcare. With the capacity to form complex non-linear analyses from large datasets and identify novel relationships, DL offers the potential to dramatically advance our diagnostic, patient monitoring, and prognostication techniques, thereby improving patient care. However, significant care is required in the development of these models, ensuring large and diverse derivation cohorts to reduce bias and guarantee generalizability, with appropriate explainability to confirm that the conclusions drawn by the model are coherent from a logical pathophysiologic perspective. In addition, regulatory bodies, including the FDA and Health Canada, need to devise specific policies for the appropriate governance of AI-based applications. Finally, the doctor-patient relationship has the potential to be enhanced when physicians can delegate clerical tasks to AI, leaving them free to focus on patient interactions.
Data availability statement
The original contributions presented in the study are included in the article/Supplementary Material, Proceedings of the 2024 Transplant AI Symposium further inquiries can be directed to the corresponding author.
Author contributions
SN: Writing – original draft, Writing – review & editing. SK: Writing – review & editing. BW: Writing – review & editing. MBr: Writing – review & editing. AS: Writing – review & editing. MBh: Conceptualization, Supervision, Writing – original draft.
Funding
The authors declare financial support was received for the research, authorship, and/or publication of this article.
The symposium was sponsored by Paladin, Astellas, and the UHN foundation.
Acknowledgments
We would like to extend our thanks to the event organizers: Dr. Elisa Pasini, Madeline Cameron, and Jonelle Palotta.
Conflict of interest
MB is a transplant hepatologist at the Ajmera Transplant Center and was co-chair of the Transplant AI Symposium.
The remaining authors declare that the research was conducted in the absence of any commercial or financial relationships that could be construed as a potential conflict of interest.
Publisher's note
All claims expressed in this article are solely those of the authors and do not necessarily represent those of their affiliated organizations, or those of the publisher, the editors and the reviewers. Any product that may be evaluated in this article, or claim that may be made by its manufacturer, is not guaranteed or endorsed by the publisher.
References
1. Kaul V, Enslin S, Gross SA. History of artificial intelligence in medicine. Gastrointest Endosc. (2020) 92(4):807–12. doi: 10.1016/j.gie.2020.06.040
2. Haug CJ, Drazen JM. Artificial intelligence and machine learning in clinical medicine, 2023. N Engl J Med. (2023) 388(13):1201–8. doi: 10.1056/NEJMra2302038
3. Esteva A, Rabicquet A, Ramsundar B, Kuleshov V, DePristo M, Chou K, et al. A guide to deep learning in healthcare. Nat Med. (2019) 25(1):24–9. doi: 10.1038/s41591-018-0316-z
4. Benjamens S, Dhunnoo P, Mesko B. The state of artificial intelligence-based FDA-approved medical devices and algorithms: an online database. NPJ Digit Med. (2020) 3:118. doi: 10.1038/s41746-020-00324-0
5. FDA. Artificial Intelligence and Machine Learning (2024). Available online at: https://www.fda.gov/medical-devices/software-medical-device-samd/artificial-intelligence-and-machine-learning-aiml-enabled-medical-devices (Accessed January 3, 2024).
6. Nikhil S. AI in Healthcare Market (2024). Available online at: https://www.transparencymarketresearch.com/ai-in-healthcare-market.html (Accessed March 9, 2024).
7. Omar Ali WA, Shrestha A, Elbasi E, Alryalat MAA, Dwivedi YK. A systematic literature review of artificial intelligence in the healthcare sector: benefits, challenges, methodologies, and functionalities. J Innov Knowl. (2023) 8(1):1–19. doi: 10.1016/j.jik.2023.100333
8. Quazi S. Artificial intelligence and machine learning in precision and genomic medicine. Med Oncol. (2022) 39(8):120. doi: 10.1007/s12032-022-01711-1
9. Uddin M, Wang Y, Woodbury-Smith M. Artificial intelligence for precision medicine in neurodevelopmental disorders. NPJ Digit Med. (2019) 2:112. doi: 10.1038/s41746-019-0191-0
10. Secinaro S, Calandra D, Secinaro A, Muthurangu V, Biancone P. The role of artificial intelligence in healthcare: a structured literature review. BMC Med Inform Decis Mak. (2021) 21(1):125. doi: 10.1186/s12911-021-01488-9
11. Martinez-Millana A, Saez-Saez A, Tornero-Costa R, Azzopardi-Muscat N, Traver V, Novillo-Ortiz D. Artificial intelligence and its impact on the domains of universal health coverage, health emergencies and health promotion: an overview of systematic reviews. Int J Med Inform. (2022) 166:104855. doi: 10.1016/j.ijmedinf.2022.104855
12. Khanna NN, Maindarkar MA, Viswanathan V, Fernandes JFE, Paul S, Bhagawati M, et al. Economics of artificial intelligence in healthcare: diagnosis vs. treatment. Healthcare (Basel). (2022) 10(12):2493. doi: 10.3390/healthcare10122493
13. Sallam M. ChatGPT utility in healthcare education, research, and practice: systematic review on the promising perspectives and valid concerns. Healthcare (Basel). (2023) 11(6):887. doi: 10.3390/healthcare11060887
14. Wong A, Otles E, Donnelly JP, Krumm A, McCullough J, DeTroyer-Cooley O, et al. External validation of a widely implemented proprietary sepsis prediction model in hospitalized patients. JAMA Intern Med. (2021) 181(8):1065–70. doi: 10.1001/jamainternmed.2021.2626
15. Nagendran M, Chen Y, Lovejoy CA, Gordon AC, Komorowski M, Harvey H, et al. Artificial intelligence versus clinicians: systematic review of design, reporting standards, and claims of deep learning studies. Br Med J. (2020) 368:m689. doi: 10.1136/bmj.m689
16. Celi LA, Cellini J, Charpignon ML, Dee EC, Dernoncourt F, Eber R, et al. Sources of bias in artificial intelligence that perpetuate healthcare disparities—a global review. PLoS Digit Health. (2022) 1(3):e0000022. doi: 10.1371/journal.pdig.0000022
17. Norori N, Hu Q, Aellen FM, Faraci FD, Tzovara A. Addressing bias in big data and AI for health care: a call for open science. Patterns (N Y). (2021) 2(10):100347. doi: 10.1016/j.patter.2021.100347
18. Mittermaier M, Raza MM, Kvedar JC. Bias in AI-based models for medical applications: challenges and mitigation strategies. NPJ Digit Med. (2023) 6(1):113. doi: 10.1038/s41746-023-00858-z
19. Aubert O, Kamar N, Vernerey D, Viglietti D, Martinez F, Duong-Van-Huyen JP, et al. Long term outcomes of transplantation using kidneys from expanded criteria donors: prospective, population based cohort study. Br Med J. (2015) 351:h3557. doi: 10.1136/bmj.h3557
20. Loupy A, Aubert O, Orandi BJ, Naesens M, Bouatou Y, Raynaud M, et al. Prediction system for risk of allograft loss in patients receiving kidney transplants: international derivation and validation study. Br Med J. (2019) 366:l4923. doi: 10.1136/bmj.l4923
21. Raynaud M, Al-Awadhi S, Juric I, Divard G, Lombardi Y, Basic-Jukic N, et al. Race-free estimated glomerular filtration rate equation in kidney transplant recipients: development and validation study. Br Med J. (2023) 381:e073654. doi: 10.1136/bmj-2022-073654
22. Aubert O, Divard G, Pascual J, Oppenheimer F, Sommerer C, Citterio F, et al. Application of the iBox prognostication system as a surrogate endpoint in the TRANSFORM randomised controlled trial: proof-of-concept study. BMJ Open. (2021) 11(10):e052138. doi: 10.1136/bmjopen-2021-052138
23. Hogan J, Divard G, Aubert O, Garro R, Boyer O, Cooper LAD, et al. Validation of a prediction system for risk of kidney allograft failure in pediatric kidney transplant recipients: an international observational study. Am J Transplant. (2023) 23(10):1561–9. doi: 10.1016/j.ajt.2023.07.004
24. Loupy A, Lefaucheur C, Vernerey D, Prugger C, Duong van Huyen JP, Mooney N, et al. Complement-binding anti-HLA antibodies and kidney-allograft survival. N Engl J Med. (2013) 369(13):1215–26. doi: 10.1056/NEJMoa1302506
25. Duong Van Huyen JP, Tible M, Gay A, Guillemain R, Aubert O, Varnous S, et al. MicroRNAs as non-invasive biomarkers of heart transplant rejection. Eur Heart J. (2014) 35(45):3194–202. doi: 10.1093/eurheartj/ehu346
26. Cassuto E, Pageaux GP, Cantarovich D, Rostaing L, Loupy A, Roche B, et al. Adherence to and acceptance of once-daily tacrolimus after kidney and liver transplant: results from OSIRIS, a French observational study. Transplantation. (2016) 100(10):2099–106. doi: 10.1097/TP.0000000000001307
27. Loupy A, Coutance G, Bonnet G, Van Keer J, Raynaud M, Aubert O, et al. Identification and characterization of trajectories of cardiac allograft vasculopathy after heart transplantation: a population-based study. Circulation. (2020) 141(24):1954–67. doi: 10.1161/CIRCULATIONAHA.119.044924
28. Reese PP, Aubert O, Naesens M, Huang E, Potluri V, Kuypers D, et al. Assessment of the utility of kidney histology as a basis for discarding organs in the United States: a comparison of international transplant practices and outcomes. J Am Soc Nephrol. (2021) 32(2):397–409. doi: 10.1681/ASN.2020040464
29. Lefaucheur C, Loupy A, Vernerey D, Duong-Van-Huyen JP, Suberbielle C, Anglicheau D, et al. Antibody-mediated vascular rejection of kidney allografts: a population-based study. Lancet. (2013) 381(9863):313–9. doi: 10.1016/S0140-6736(12)61265-3
30. Loupy A, Vernerey D, Viglietti D, et al. Determinants and outcomes of accelerated arteriosclerosis: major impact of circulating antibodies. Circ Res. (2015) 117(5):470–82. doi: 10.1161/CIRCRESAHA.117.306340
31. Aubert O, Loupy A, Hidalgo L, Duong van Huyen JP, Higgins S, Viglietti D, et al. Antibody-mediated rejection due to preexisting versus de novo donor-specific antibodies in kidney allograft recipients. J Am Soc Nephrol. (2017) 28(6):1912–23. doi: 10.1681/ASN.2016070797
32. See SB, Aubert O, Loupy A, Veras Y, Lebreton X, Gao B, et al. Post-transplant natural antibodies associate with kidney allograft injury and reduced long-term survival. J Am Soc Nephrol. (2018) 29(6):1761–70. doi: 10.1681/ASN.2017111157
33. Loupy A, Lefaucheur C, Vernerey D, Chang J, Hidalgo LG, Beuscart T, et al. Molecular microscope strategy to improve risk stratification in early antibody-mediated kidney allograft rejection. J Am Soc Nephrol. (2014) 25(10):2267–77. doi: 10.1681/ASN.2013111149
34. Loupy A, Duong Van Huyen JP, Hidalgo L, Reeve J, Racapé M, Aubert O, et al. Gene expression profiling for the identification and classification of antibody-mediated heart rejection. Circulation. (2017) 135(10):917–35. doi: 10.1161/CIRCULATIONAHA.116.022907
35. Loupy A, Lefaucheur C. Antibody-mediated rejection of solid-organ allografts. N Engl J Med. (2018) 379(12):1150–60. doi: 10.1056/NEJMra1802677
36. Lefaucheur C, Viglietti D, Bouatou Y, Philippe A, Pievani D, Aubert O, et al. Non-HLA agonistic anti-angiotensin II type 1 receptor antibodies induce a distinctive phenotype of antibody-mediated rejection in kidney transplant recipients. Kidney Int. (2019) 96(1):189–201. doi: 10.1016/j.kint.2019.01.030
37. Raynaud M, Aubert O, Reese PP, Bouatou Y, Naesens M, Kamar N, et al. Trajectories of glomerular filtration rate and progression to end stage kidney disease after kidney transplantation. Kidney Int. (2021) 99(1):186–97. doi: 10.1016/j.kint.2020.07.025
38. Loupy A, Goutaudier V, Giarraputo A, Mezine F, Morgand E, Robin B, et al. Immune response after pig-to-human kidney xenotransplantation: a multimodal phenotyping study. Lancet. (2023) 402(10408):1158–69. doi: 10.1016/S0140-6736(23)01349-1
39. Aubert O, Reese PP, Audry B, Bouatou Y, Raynaud M, Viglietti D, et al. Disparities in acceptance of deceased donor kidneys between the United States and France and estimated effects of increased US acceptance. JAMA Intern Med. (2019) 179(10):1365–74. doi: 10.1001/jamainternmed.2019.2322
40. Loupy A, Aubert O, Reese PP, Bastien O, Bayer F, Jacquelinet C. Organ procurement and transplantation during the COVID-19 pandemic. Lancet. (2020) 395(10237):e95–6. doi: 10.1016/S0140-6736(20)31040-0
41. Aubert O, Yoo D, Zielinski D, Cozzi E, Cardillo M, Dürr M, et al. COVID-19 pandemic and worldwide organ transplantation: a population-based study. Lancet Public Health. (2021) 6(10):e709–19. doi: 10.1016/S2468-2667(21)00200-0
42. Raynaud M, Aubert O, Divard G, Reese PP, Kamar N, Yoo D, et al. Dynamic prediction of renal survival among deeply phenotyped kidney transplant recipients using artificial intelligence: an observational, international, multicohort study. Lancet Digit Health. (2021) 3(12):e795–805. doi: 10.1016/S2589-7500(21)00209-0
43. Yoo D, Goutaudier V, Divard G, Gueguen J, Astor BC, Aubert O, et al. An automated histological classification system for precision diagnostics of kidney allografts. Nat Med. (2023) 29(5):1211–20. doi: 10.1038/s41591-023-02323-6
44. Loupy A, Mengel M, Haas M. Thirty years of the international banff classification for allograft pathology: the past, present, and future of kidney transplant diagnostics. Kidney Int. (2022) 101(4):678–91. doi: 10.1016/j.kint.2021.11.028
45. Organ Donation Statistics. Published 2023. Updated October 2023. Available online at: www.organdonor.gov (Accessed January 9, 2024).
46. Lee JC, Christie JD. Primary graft dysfunction. Proc Am Thorac Soc. (2009) 6(1):39–46. doi: 10.1513/pats.200808-082GO
47. Roman MA, Nair S, Tsui S, Dunning J, Parmar JS. Ex vivo lung perfusion: a comprehensive review of the development and exploration of future trends. Transplantation. (2013) 96(6):509–18. doi: 10.1097/TP.0b013e318295eeb7
48. Sage AT, Donahoe LL, Shamandy AA, et al. A machine-learning approach to human ex vivo lung perfusion predicts transplantation outcomes and promotes organ utilization. Nat Commun. (2023) 14(1):4810. doi: 10.1038/s41467-023-40468-7
49. Canadian Institute for Health Information. Organ Replacement in Canada: CORR Annual Statistics. (2022). Available online at: https://www.cihi.ca/en/organ-replacement-in-canada-corr-annual-statistics (Accessed March 9, 2024).
50. Haldar D, Kern B, Hodson J, Armstrong MJ, Adam R, Berlakovich G, et al. Outcomes of liver transplantation for non-alcoholic steatohepatitis: a European liver transplant registry study. J Hepatol. (2019) 71(2):313–22. doi: 10.1016/j.jhep.2019.04.011
51. Nitski O, Azhie A, Qazi-Arisar FA, Wang X, Ma S, Lilly L, et al. Long-term mortality risk stratification of liver transplant recipients: real-time application of deep learning algorithms on longitudinal data. Lancet Digit Health. (2021) 3(5):e295–305. doi: 10.1016/S2589-7500(21)00040-6
52. Wiese S, Hove JD, Bendtsen F, Moller S. Cirrhotic cardiomyopathy: pathogenesis and clinical relevance. Nat Rev Gastroenterol Hepatol. (2014) 11(3):177–86. doi: 10.1038/nrgastro.2013.210
53. Ahn JC, Attia ZI, Rattan P, Mullan AF, Buryska S, Allen AM, et al. Development of the AI-Cirrhosis-ECG score: an electrocardiogram-based deep learning model in cirrhosis. Am J Gastroenterol. (2022) 117(3):424–32. doi: 10.14309/ajg.0000000000001617
54. Ahn JC, Connell A, Simonetto DA, Hughes C, Shah VH. Application of artificial intelligence for the diagnosis and treatment of liver diseases. Hepatology. (2021) 73(6):2546–63. doi: 10.1002/hep.31603
55. Zou KH, Warfield SK, Bharatha A, Tempany CMC, Kaus MR, Haker SJ, et al. Statistical validation of image segmentation quality based on a spatial overlap index. Acad Radiol. (2004) 11(2):178–89. doi: 10.1016/S1076-6332(03)00671-8
56. Ware P, Dorai M, Ross HJ, Cafazzo JA, Laporte A, Boodoo C, et al. Patient adherence to a mobile phone-based heart failure telemonitoring program: a longitudinal mixed-methods study. JMIR Mhealth Uhealth. (2019) 7(2):e13259. doi: 10.2196/13259
Keywords: artificial intelligence, machine learning, prognostication models, transplant medicine, clinical deployment
Citation: Naimimohasses S, Keshavjee S, Wang B, Brudno M, Sidhu A and Bhat M (2024) Proceedings of the 2024 Transplant AI Symposium. Front. Transplant. 3:1399324. doi: 10.3389/frtra.2024.1399324
Received: 11 March 2024; Accepted: 23 July 2024;
Published: 29 August 2024.
Edited by:
Khodor Abou-Daya, University of Pittsburgh, United StatesReviewed by:
Steven Wisel, Cedars Sinai Medical Center, United StatesCopyright: © 2024 Naimimohasses, Keshavjee, Wang, Brudno, Sidhu and Bhat. This is an open-access article distributed under the terms of the Creative Commons Attribution License (CC BY). The use, distribution or reproduction in other forums is permitted, provided the original author(s) and the copyright owner(s) are credited and that the original publication in this journal is cited, in accordance with accepted academic practice. No use, distribution or reproduction is permitted which does not comply with these terms.
*Correspondence: Mamatha Bhat, Mamatha.Bhat@uhn.ca