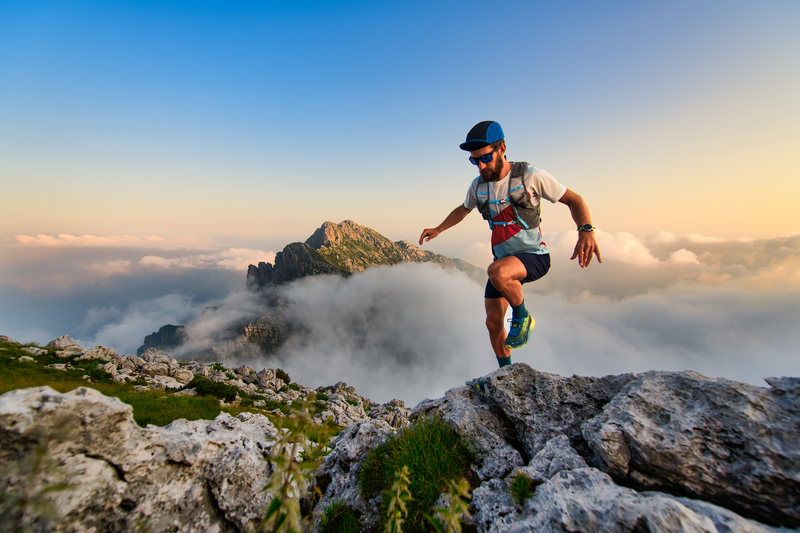
94% of researchers rate our articles as excellent or good
Learn more about the work of our research integrity team to safeguard the quality of each article we publish.
Find out more
REVIEW article
Front. Toxicol. , 17 November 2023
Sec. Regulatory Toxicology
Volume 5 - 2023 | https://doi.org/10.3389/ftox.2023.1292373
This article is part of the Research Topic Global Excellence in Toxicology: Asia, Australia and New Zealand View all 4 articles
Novel techniques and methodologies are being developed to advance food safety risk assessment into the next-generation. Considering the shortcomings of traditional animal testing, new approach methodologies (NAMs) will be the main tools for the next-generation risk assessment (NGRA), using non-animal methodologies such as in vitro and in silico approaches. The United States Environmental Protection Agency and the European Food Safety Authority have established work plans to encourage the development and application of NAMs in NGRA. Currently, NAMs are more commonly used in research than in regulatory risk assessment. China is also developing NAMs for NGRA but without a comprehensive review of the current work. This review summarizes major NAM-related research articles from China and highlights the China National Center for Food Safety Risk Assessment (CFSA) as the primary institution leading the implementation of NAMs in NGRA in China. The projects of CFSA on NAMs such as the Food Toxicology Program and the strategies for implementing NAMs in NGRA are outlined. Key issues and recommendations, such as discipline development and team building, are also presented to promote NAMs development in China and worldwide.
The food safety risk assessment is an important component in risk analysis and provides scientific support for activities of risk management. As reviewed by Wu et al. (2018), the national food safety risk assessment system of China has been developed since 2009. Established in 2011, China National Center for Food Safety Risk Assessment (CFSA) is the only technical institution for food safety risk assessment at the national level in China, under the direct leadership of the National Health Commission of the People’s Republic of China. CFSA serves as the secretariat of the National Expert Committee for Food Safety Risk Assessment, and one important responsibility of CFSA is to implement food safety risk assessment projects and provide technical support and policy-making suggestions to the related government departments.
Toxicology is the basis of food safety risk assessments. However, considering the large number and complex toxicological effects of food chemicals, traditional animal tests are costly, time-consuming, and labor-intensive to identify the hazards of food chemicals. Establishing alternative, highly sensitive, and high-throughput methods are the primary technical needs. In recent years, scientific and technological progress, especially the rapid development in the life sciences, has provided new opportunities for the development of toxicological research methods. In 2007, the United States National Research Council proposed that the toxicity in the 21st century would be transformed from in vivo experiments to alternative in vitro assays to assess health risks (Krewski et al., 2010). Using alternative technologies such as in vitro cell tests to achieve risk assessment is based on the in-depth understanding of the toxicological mechanisms of chemicals, including molecular and cellular levels of toxicity pathways, tissue and organ levels of toxicity effects, and then adverse outcome pathways at individual and population levels. This requires collecting scientific evidence at various levels (Escher et al., 2022). Based on this concept of toxicological testing development, food toxicology is also facing a transition from traditional animal experiments to new strategies based on in vitro and in silico testing.
On the other hand, people’s awareness of animal welfare protection has reached a historical height. As more countries and organizations are committed to the 3R principles (replacement, reduction, and refinement) of animal testing, the prohibition of animal experiments and their replacement by other experimental methods are the needs of the times and future trends. In this regard, Europe is at the forefront. As early as 1991, the European Center for the Validation of Alternative Methods (ECVAM) (renamed the EU Reference Laboratory for Alternatives to Animal Testing, EURL ECVAM in 2011) was established to reduce animal testing. In 2010, the EU directive 2010/63/EU on the protection of laboratory animals stated that member states should aim to develop and validate alternative methods (Directive, 2010). In 2012, the Cambridge Declaration stated that all non-human animals have a biological basis for consciousness (Low et al., 2012). In 2013, the EU took the lead in implementing a complete ban on cosmetics animal testing globally. Norway, New Zealand, Israel, India, and other countries are also following up (Sreedhar et al., 2020).
In 2020, the European Commission issued the Chemicals Strategy for Sustainability to promote interdisciplinary research and digital innovation focusing on advanced analytical tools, methods, models, and data analysis capabilities, thereby reducing animal experimentation (Commission, 2020). In 2021, European Food Safety Authority (EFSA) released Strategy 2027, advocating the promotion of new approach methodologies (NAMs) based on alternatives to animal experiments, the development of cutting-edge new methods, and the improvement of the quality and efficiency of hazard risk assessment of chemicals or food-related substances (EFSA, 2021). Compared with Europe, the strategic goals and initiatives of the United States to support animal alternative technologies are more explicit. In 2019, the United States Environmental Protection Agency (EPA) released phased goals to reduce animal testing and will stop conducting or funding research on mammals by 2035 (Grimm, 2019). In 2022, the United States Food and Drug Administration (FDA) announced that new drugs will no longer require animal testing before human clinical trials, but will rely more on computer modeling, organ chips, and other new methods developed in recent years (Wadman, 2023). The regulatory prohibition of animal experiments may soon extend to food and pharmaceuticals.
Given the above reasons, the non-animal based NAMs that include emerging technologies for the next-generation risk assessment (NGRA) are the focus worldwide. Most NAMs are toxicologically related, including in vitro alternative methods, extrapolation based on physiologically based toxicokinetic (PBTK), and computational toxicological methods. Other NAMs cover the rest aspects of NGRA such as exposome applied in the exposure assessment and integrated approaches for testing and assessment (IATA) method used to comprehensively integrate all the related information from different methods for a specific risk assessment scenario. The United States EPA and EFSA have established work plans to encourage the development and application of NAMs in NGRA (EPA, 2021; Escher et al., 2022). In 2023, EFSA also reviewed its activities taken to implement NAMs in food safety risk assessments (Cattaneo et al., 2023). In support of the European Union’s Chemicals Strategy for Sustainability and the European Green Deal’s “Zero pollution”, a Partnership for the Assessment of Risks from Chemicals (PARC) has been established involving approximately 200 institutions from 28 countries as well as the European Chemical Agency (ECHA), EFSA, and the European Environment Agency (EEA), for the development of next-generation chemical risk assessment to protect human health and the environment (Partnership for the Assessment). Most NAMs are still in the research stage rather than in regulatory risk assessment. China is also developing NAMs for NGRA, and CFSA is the primary institution leading the implementation of NAMs in NGRA in China. This review provides an overview of the work of CFSA related to NAMs and summarizes major NAM-related research articles from China. This review also describes the key issues and the corresponding countermeasures of CFSA to develop and apply NAMs in food safety risk assessment.
The food supply and consumption scale of China are huge, with complex structures covering all aspects from agricultural production to food processing. The potential health hazards are not only numerous in number but also often show unique characteristics of China’s food safety. Since the establishment of the national food safety risk assessment system, achievements have been made in the development of methodology and procedure, data collection, team building, and the accomplishment of a series of risk assessment projects (Wu et al., 2018).
As toxicology is the basis for hazard assessment in food safety risk assessment, CFSA officially proposed the “Food Toxicology Program” in 2013 and began to organize the development and application of new technologies based on the relevant framework. The “Food Toxicology Program” is a basic, systematic, and long-term work. This program not only strengthened the development of traditional food toxicology in China but also promoted the development of NAMs. Specifically, by implementing this program in China, 1) China’s food safety emergency response capabilities were increased, and scientific guarantees were provided for food safety risk assessment and risk management by establishing a systematic toxicological database and continuously accumulating toxicological information on chemicals; 2) hazard assessments of certain chemical substance were based on the toxicological data generated from this program, thereby enabling China’s food toxicology to have a say in the international community and play a leading role in the risk assessment and standard setting of substances unique to China; 3) research on new toxicological NAMs can be carried out to explore and establish various feasible key technologies for hazard assessment.
After 10 years of development, the “New Risk Identification and Toxicity Screening New Technology Platform” has gradually established from scratch, covering methods including toxicological threshold of concern (TTC), quantitative structure-activity relationship (QSAR), rapid detection technologies based on human cell and model organisms, alternative toxicological methods, as well as systematic toxicological methods represented by various omics (toxicogenomics, toxic transcriptomics, toxic metabolomics, etc.) and translational toxicological methods represented by adverse outcome pathways (AOPs).
The progress made including the NAMs development based on the “Food Toxicology Program” and some NAMs CFSA and research institutions in China are working on are listed below.
Based on the National Key R&D Program Project “Integrated Technology and Application Research on Hazard Assessment of Food Pollutants” (2018–2021), aiming at the key technical bottlenecks in the risk assessment of food pollutants in China, the CFSA toxicology team developed 10 hazard identification technologies, including human macrophage high-content models, human hepatocyte lipid toxicity models, human white-brown fat cell differentiation interference models, human embryonic stem cell developmental toxicity models, zebrafish liver toxicity models, and extended first-generation reproductive toxicity models, etc. Breakthrough innovations have been made in the research and application of alternative testing methods. In particular, comparable and verifiable rapid and efficient alternative testing toxicity and hazard identification techniques have been established for three toxicity endpoints with high international concern (carcinogenicity, reproductive developmental toxicity, and genotoxicity). This made up for the lack of alternative testing methods in China and promoted the shift in toxicity testing from traditional animal testing to alternative testing technologies.
To achieve rapid and accurate screening of endocrine disrupting toxicity in food related chemicals, the CFSA research team has established a high-throughput hazard identification in vitro alternative technology based on a model that employs differentiated macrophages transfected with PPRE receptor response elements to enhance the sensitivity of cell carrier signals. Through the series of upstream and downstream key molecules, the high-content analysis of multiple effect targets is realized. This model has been used for toxicity screening and classification of over 3,000 food compounds and 160 priority chemicals were identified, providing a scientific basis for subsequent hazard assessment or health effect studies. In addition, this technology found that the plasticizer diethylhexyl phthalate (DEHP) can activate macrophage peroxisome proliferator-activated receptors, interfere with lipid metabolism balance, and induce fatty liver (Xu et al., 2022a). Related research results were published in EHP and reported in the form of “Science Selection.” Peer review pointed out that “this study is an important progress in this field. We may have underestimated the role of macrophages in DEHP-induced fatty liver. This innovative discovery reflects the key role of the immune system in metabolic diseases” (Schmidt, 2022). On this basis, combined toxicity assessments of various phthalate plasticizers (DEHP, DBP, DIBP, DINP, DCHP, DMP, DEP) are currently under investigation.
For embryonic developmental toxicity, the CFSA laboratory has established a high-content hazard identification in vitro alternative model based on human embryonic stem cell embryoid bodies and differentiation of inner, middle, and outer germ layers (Fang et al., 2019). The toxicity of zearalenone and trichothecene were detected in the model and both showed toxic effects on the development of specific embryonic layers mechanisms (Fang et al., 2018; Cao et al., 2019). The developed model can be considered a promising tool to assess developmental toxicity and interpret the underlying mechanism.
Other cell-based and organoids in vitro methods were also developed in China for specific research purposes of food hazards. The in vitro Rijksinstituut voor volksgezondheiden milieu digestion model and Caco-2 cell model were used to assess the bioavailability and bioaccessibility of Cadmium in rice in China, suggesting the necessity to consider the bioavailability and bioaccessibility to accurately assess the health risk of cadmium exposure through rice (Yao et al., 2021; Deng et al., 2022). Retinal organoid models differentiated from human embryonic stem cells were used to explore the effects of bisphenols on the early development of the retina (Li et al., 2022a). Liver organoids and intestinal organoids differentiated from human pluripotent stem cells (hiPSCs) were used to analyze the hepatotoxicity of polystyrene (PS) microplastics and the accumulation of PS nanoplastics in intestinal organoids, respectively (Cheng et al., 2022; Hou et al., 2022). A murine small intestine organoid model was used to explore the effects of sunset yellow on small intestinal cells (Kong et al., 2021). Organoids from murine thyrocytes with thyroid follicle-like structures were developed to study the adverse effects of iodide excess on thyroids (Yu et al., 2023).
Given the ongoing research on NAMs, a wide range of in vitro biological assays has been developed to screen a chemical of interest for different toxicity endpoints or to elucidate the modes of action underlying the toxicity (Bernauer et al., 2005). Clearly, many in vitro experiments do not (yet) capture the toxicokinetics information, and thereby do not fully reflect the in vivo situation. To enable the use of in vitro assays in human risk assessment, a translation is required to convert the in vitro concentration-response data to in vivo human dose-response data, taking into account toxicokinetics (Blaauboer, 2010; Bell et al., 2018). As an important element of NAMs, PBTK models can link the internal concentrations upon exposure to known external doses of the chemicals (i.e., forward dosimetry), and they can also be used to extrapolate in vitro toxicity data to external dose values considering toxicokinetics, which is referred to as reverse dosimetry (Rietjens et al., 2011). CFSA is dedicated to developing a PBTK modeling-based hazard assessment strategy and actively promotes its application in food safety risk assessment. CFSA has implemented the PBTK models to define the human equivalent dose factor of Bisphenol A, as well as predicted human equivalent doses for several phenolic endocrine disrupting chemicals by using the PBTK modeling-based in vitro to in vivo extrapolation (IVIVE) approach (Xie et al., 2023). In addition, the application of PBTK modeling is further expanded to a more comprehensive compound-specific risk assessment, which provides more accurate hazard assessment information for the higher tier risk-based decision making. This is demonstrated by a case study where the PBTK modeling-based IVIVE approach was integrated with Monte Carlo simulation to quantify the inter-individual kinetic variation underlaying possible variation in cardiotoxicity of methadone, and to derive chemical-specific adjustment factors (CSAFs) for inter-individual and inter-ethnic kinetic differences for the Caucasian and Chinese populations (Shi et al., 2022). Though PBTK modeling has been used in a few regulatory risk assessment projects in China, CFSA is continuously working on the scientific and regulatory gaps, such as developing the guidance on when and how to apply PBTK modeling to replace part of the default uncertainty factors, how to better address the sensitivity of the input parameters, and developing the standardized methods for PBTK modeling, also pointed out in the roadmap for NAMs by EFSA (Escher et al., 2022).
Related studies by other research institutions in China included the case studies of using PBTK to predict liver toxicity induced by troglitazone (Yu et al., 2020) and acetaminophen (Zhang et al., 2020a), to predict cardiovascular toxicity induced by doxorubicin (Li et al., 2021a).
Computational toxicological methods such as QSAR and read across are also important in silico NAMs for the NGRA of food safety. CFSA has established the application guide of QSAR in food safety risk assessment and the application guide of read across in food safety risk assessment, including the overall principle, definition, application flow chart, and detailed explanation of the application procedure (CFSA, 2022). These guidelines serve as the basis for the application of these methods in the food safety risk assessment projects in China and the development of the next-generation computational toxicological methods.
However, QSAR and read across are currently more used in research in China, and they have not been formally applied to the food safety risk assessment projects by CFSA. Nevertheless, relevant research articles have been published by CFSA and various research institutions in China. Ma et al. (2021) used three QSAR tools (Toxtree, VEGA, T.E.S.T) and developed a risk classification strategy for chemicals migrated from food contact materials. Sun et al. (2021) developed QSAR models to predict the acute oral toxicity of polycyclic aromatic hydrocarbons (PAHs), which had been the focus of human health risk assessment due to their existence in foods and the potential toxicological effects. To study the toxicity mechanisms of halogenated disinfection byproduct (DBPs), Zhang et al. (2020b) developed a QSAR model using the in vitro cytotoxicity data of 15 halogenated aromatic DBPs tested with mammalian Chinese Hamster Ovary cells, and they found the interaction of aromatic DBPs with catalase and the electrophilic/nucleophilic reactivity were associated with the cytotoxicity. Found in foods and as a potential human health hazard, Hao et al. (2019) constructed QSAR models to predict the mutagenicity of nitroaromatic compounds. Wang et al. (2021) developed QSAR models to predict the peroxisome proliferator-activated receptor γ binding affinity of chemicals, to evaluate the potential endocrine-disrupting effects of chemicals. Xu et al. (2023) used the QSAR model to predict the bioconcentration factors of water pollutants without toxicity data.
Food contaminants such as PAHs and DBPs mentioned above are also either parts of the current priority risk assessment projects or have been considered as the potential projects of CFSA. But these contaminants contain a series of chemicals and are more difficult to assess their health risk compared to the single chemical contaminant, and one important reason is the lack of toxicological data. Therefore, QSAR and read across methods to predict the toxicity of untested contaminants can be used as the supplement to the hazard assessment of these projects. Though these methods are currently used more in research than in regulatory fields, the CFSA risk assessment team is cooperating with experts in computational toxicology from academic institutions to apply these methods into the risk assessment projects, e.g., efforts are being made in developing the methods involving the weight of evidence approach to evaluate the input values.
TTC is the approach that can qualitatively assess the risk of a substance with little or no toxicological data. Having been developed by scientists for decades, TTC has been widely used in food safety risk assessment in China and other countries. EFSA published the guidance on the use of TTC in food safety assessment (Committee et al., 2019). Similarly, CFSA established the application guide of TTC in food safety risk assessment (CFSA, 2022). CFSA also developed the approach to apply TTC to the risk assessment of food contact materials and assessed the health risk of several PAHs, including bis (2-ethylhexyl) phthalate (DEHP), dimethyl 1,2-benzenedicarboxylate (DMP), and 1,2-benzenedicarboxylic acid diethyl ester (DEP) (Sui et al., 2012; Yong et al., 2016). The method to apply TTC for the safety assessment of non-intentionally added substances from food contact material was also developed (Zhong et al., 2017). In the last decade, CFSA has used TTC approach to assess the risk of Alternaria toxins in wheat, tomato, and citrus-based products, furfural and its derivatives in coffee products, and non-volatile compounds in polyamide food contact materials (Zhao et al., 2015a; Zhao et al., 2015b; Hu et al., 2021; Ji et al., 2022a; Liu et al., 2023a). Other academic institutions in China also adopted the TTC approach to assess the risk of flavor compounds in flavored milk (Chen et al., 2023), estrogens in milk products (Chen et al., 2014), fluorinated liquid-crystal monomers in foods (Yang et al., 2023), and mycotoxins such as Alternaria and Fusarium in different food categories (Fan et al., 2021; Zhou et al., 2022a; Ji et al., 2022b; Ji et al., 2022c; Qiao et al., 2022; Ji et al., 2023a; Ji et al., 2023b). Though TTC is not a “new” method, it remains a useful approach without testing, but with some limitations such as the method may not be applicable for some substances summarized in the guide by EFSA (Committee et al., 2019). Besides, there is currently no internationally coordinated internal TTC threshold that can be used in chemical assessments for multiple route-specific exposures (oral, dermal, inhalation) or aggregate exposures, which is also the direction of future research (Arnot et al., 2022).
With the development of artificial intelligence (AI), AI approaches such as deep learning, machine learning, and neural network have potential to be applied in food safety, which has been extensively reviewed, including the main categories of data collection, data storage and transferring, data analysis, data visualization, and data security (Zhou et al., 2022b; Liu et al., 2023b). For the data-driven NGRA, AI has the potential to be used in the whole workflow, such as being applied in hazard assessment and exposure assessment in collecting and managing data.
To explore the application of AI-related methods in evidence management to build the AOP networks, EFSA developed the “Roadmap for actions on AI for evidence management in risk assessment” and launched the project “Exploring the use of AI for extracting and integrating data obtained through NAMs” (Bersani et al., 2022; Cavalli et al., 2022; Cattaneo et al., 2023). EFSA published a scientific report earlier this year showing the result of using AI-related tools for evidence management, such as automatic text summarization, keyword identification, deduplication, screening, and semi-automated characterization or classification (Cagnoni et al., 2023).
CFSA is also exploring the application of AI-related methods in evidence management for the NGRA, starting with developing a website with the function of semi-automatic comprehensive toxicological data searching and screening for a specific chemical contaminant. Once the website passes testing and validation, more functions will be added, including data extraction, data evaluation assistance (reliability and relevance evaluations), and data integration. The website is expected to assist the evidence-based food safety risk assessment projects. CFSA is also developing the application guide of AI in alternative protein risk assessment, attempting to be the starting point of using AI in a specific area of NGRA and expecting to guide the development of AI techniques in NGRA. The ongoing research areas of AI in CFSA include but not limited to evidence management, data prediction, and usage in other in vitro and in silico NAMs.
Research on AI in food safety risk assessment has also been conducted by academic institutions in China. Most of the research articles focused on developing AI models to predict the safety levels of certain hazards of different food products. The research team of Dong used AI methods such as neural networks and deep learning to predict the safety risk levels divided by clustering machine learning technique of contaminants such as heavy metals, veterinary drugs (florfenicol, enrofloxacin, and sulfonamide) residues, and arbofuran pesticide residues in different food categories (Jiang et al., 2022a; Jiang et al., 2022b; Dong et al., 2023; Lu et al., 2023). Wang et al. (2022) used the voting-ensemble deep learning method and the sub-models to analyze the risks of heavy metals in grain products. Xu et al. (2022b) combined three machine learning models to analyze multiple hazards (heavy metal, mycotoxin, pollutants) in rice. Other AI models used include agglomerative hierarchical clustering-radial basis function neural network integrating an analytic hierarchy process approach and the entropy weight, analytic hierarchy process integrated extreme learning machine based approach, and analytic hierarchy process based on the entropy weight and the autoencoder-recurrent neural network integrated framework for early warning analyses (Geng et al., 2017; Geng et al., 2021; Zhong et al., 2023). Additionally, AI methods were also used to generate virtual sample data expanding from a small dataset, using a model of improved random forest prediction method integrated Monte Carlo algorithm (Geng et al., 2022).
Covering a series of emerging technologies (genomics, transcriptomics, proteomics, metagenomics, epigenomics, metabolomics, exposomics, etc.), omics has been applied to the food safety risk assessment, and some omics-based approaches such as whole genome sequencing in genomics have been used in regulatory risk assessment of EFSA (Authority et al., 2018; Iacono et al., 2022). CFSA is also adopting omics-based approaches such as whole genome sequencing and nontargeted metabolomics in the ongoing food safety risk assessment projects. The application of whole genome sequencing in the surveillance of foodborne disease in China and the general use of nontargeted methods such as metabolomics have been reviewed (Shao et al., 2019; Li et al., 2021b). Other research studies in genomics by CFSA included the integration of whole genome sequencing and machine learning to identify antimicrobial resistance of E. coli (Peng et al., 2022), the complete genome and plasmid sequences Salmonella enterica subsp. enterica isolates carrying mcr-1 (Hu et al., 2019), and the complete genomic analysis of a mcr-1-carrying Salmonella Typhimurium strain from ready-to-eat pork (Wang et al., 2018), etc. The development and usage of omics databases, improvement and incorporation of various omics technologies in risk assessment are the possible research areas (Iacono et al., 2022).
As one important component in food safety risk assessment, exposure assessment is essential for risk characterization. Though current exposure methods are sufficient for the risk assessments in specific scenarios, exposome data is needed to address the inter-individual variability for NGRA, e.g., epidemiological information is needed to include to analyze the diseased and healthy populations separately, and different exposure routes (oral, dermal, inhalation) and additional exposure pathways (e.g., occupational, environmental) should be considered (Wu, 2012; Wambaugh et al., 2019; Cattaneo et al., 2023). NAMs such as AI approaches can also be integrated with exposome to improve the exposure assessment (Wambaugh et al., 2019). The research gaps of exposome include the need of better causal inference of exposome data and adverse health effects, the consideration of physiologic variation, and the need for improved measurement and analysis methods (Escher et al., 2022).
CFSA has completed a project entitled “Characterizing the Exposome of Food Contamination and the China Total Diet Study.” Both the external and internal exposures of the contaminants recognized were analyzed and their correlation was studied through the development of the bioavailability, bioaccessibility, and PBTK modeling. The exposure biomarkers and the points of departure of the contaminants were also determined. The results of this project provided the data support for the food safety risk assessments in China (Lyu et al., 2022).
IATA is the approach to integrate, evaluate, and weigh the evidence from various sources, and it is preferred to be developed and used for NGRA, especially when risk assessments are carried out based on information generated from traditional methods and NAMs. OECD summarized the available guides of IATA and published related case studies (OECD, 2020; OECD). EFSA developed the guidance of weight of evidence approach in scientific assessments and developed case studies to improve the implementation of IATA in risk assessments (Hardy et al., 2017; EPoPP et al., 2021). CFSA risk assessment team is also developing the guide of IATA for NGRA, which will be the document guiding the application approaches of NAMs in the food safety risk assessment in China.
AOP includes the sequenced causally linked events at various biological organization levels between the exposure of a chemical and an adverse health effect, and it is the basis of IATA (Cattaneo et al., 2023; Viviani et al., 2023; OECD. Adverse). OECD published a guide on the use of AOP to develop IATA (OECD. Guidance, 2022). Through the establishment of AOP networks, it is expected to improve the understanding of molecular mechanisms and enhance regulatory implementation (Knapen et al., 2018; Villeneuve et al., 2018; Wiklund et al., 2023). EFSA developed AOPs to identify substances with endocrine disruptor properties (EPoPP et al., 2023; Viviani et al., 2023). The established AOPs are summarized on the website of OECD (OECD). AOPwiki is another platform with current available AOPs (validated or not) summarized, where AOP can be searched by molecular initiating events, adverse outcomes, key events, key event relationships, and prototypical stressors (AOP-Wiki 2023).
CFSA toxicology team has been working on the development of AOP based hazard assessment models at different biological organization levels. However, the other AOP related research in China focused more on the pharmacy and environment rather than food safety. To give a few examples, Chai et al. (2021) generated the AOPs of male reproductivity toxicity of inorganic arsenic; the research group of Wei studied different impairment mechanisms induced by black carbon, a typical environment pollutant, which contributed to the AOP of black carbon (Li et al., 2022b; Jiang et al., 2022c; Cui et al., 2023); Peng et al. (2021) overviewed the AOP of drug-induced liver injury. The collaboration between CFSA and the research institutions on the transformation of the related research into the field of food safety is the next step task of CFSA.
Facing the trend that the NGRA is based on NAMs, it is urgent to determine the roadmap for the short-term and long-term development of NAMs in China. As the only national level institution for food safety risk assessment in China, CFSA has the responsibility and is working on the establishment of the strategies to develop NAMs applicable for the risk assessments in China. The CFSA risk assessment team has been working in groups to develop the roadmaps for different NAMs. Principles of the strategies such as targeted NAMs with priority, development by stages and by layers are being considered. Once the strategies are determined, the document will be the basis to guide the development of the NAMs related technologies in China. Collaborating with research institutions in China, CFSA will guide and promote China’s development of NAMs to serve the development of NGRA to better ensure food safety in China, and to contribute to the NAMs for the international community as well.
In the 21st century, food toxicology is experiencing unprecedented development opportunities due to the progress in the life sciences. However, food toxicology still faces some special challenges, such as: how to accurately identify unknown hazards in food, food additives, or food-related products; how to fully simulate the long-term, mixed, oral, low-dose exposure characteristics of the human body to exogenous chemicals; and how to scientifically balance the health benefits of food ingredients with the risks. Solving these key problems depend on the continuous innovation and development of food toxicology itself as a discipline in addition to the progress in life sciences. Additionally, toxicity testing is currently shifting from traditional toxicology to more comprehensive systems toxicology research. However, systems toxicology also faces challenges in theory, technology, and application implementation. Its development requires interdisciplinary talents, high-throughput and high-content measurement technologies, powerful computer simulation capabilities and data analysis levels. Interdisciplinary and cross-field cooperation is needed to promote its theoretical and technological innovation and promote its application in practice.
Therefore, modern food toxicology needs to continuously adopt new technologies and methods from related fields such as molecular and cellular biology, analytical chemistry, statistics, and computer information science on the basis of traditional toxicological research, and integrate across disciplines to provide more accurate and efficient scientific support for toxicity assessment and risk assessment. CFSA will continue to work based on the “Food Toxicology Program” for the transition from traditional food toxicology to modern toxicology. The research on the NAMs in toxicology will be part of the work for the next stage.
Currently, NAMs are more commonly used in research than in regulatory risk assessment. Though research progress of some NAMs such as alternative in vitro methods and computational toxicology has been made, most NAMs are not confident enough for the replacement of the current risk assessment methods. Besides CFSA, the scientific research institutions in China are also working on the NAMs. But different from CFSA whose direct purpose is to apply NAMs in the risk assessment, these institutions do not share the common goals or may not have research interests in risk assessments. CFSA keeps improving the collaboration with research institutions and has established the “1 + 6” cooperative mechanism with 6 leading universities in China. Taking advantage of the universities, CFSA will better lead the development of NAMs for NGRA with more communication and multiple collaborating mechanisms. On one hand, CFSA risk assessment team is organizing and implementing NAMs developing programs directly applicable for food safety risk assessment in urgent need. On the other hand, the related research of other collaborating institutions will be adopted and guided in risk assessment. Furthermore, application of the NAMs under research in food safety risk assessment projects is being conducted, which will facilitate the transformation of the emerging technology to the risk assessment in phases. The problems met during the application attempts will guide the changes of the research focus of the NAMs in return.
To meet the needs of toxicity testing technology development, the CFSA research team of the Toxicology Program has also established targeted NAMs research directions, including alternative methods, toxic pathways, and extrapolation models. To continue the research and promote the transformation of these methods into NGRA, the next steps are: 1) continue to accelerate the research and development of hazard identification technologies based primarily on human-derived cells and zebrafish in vitro, while exploring biological carriers that are closer to human target organs based on previous alternative model research; 2) make full use of modern toxicological technologies such as genomics, transcriptomics, proteomics, and metabolomics as well as data integration and analysis for the discovery of toxic pathways and the explanation of toxic mechanisms, and develop high-content and high-throughput hazard identification technologies based on key molecular characteristics of toxicity; 3) combine structure-activity analysis, PBTK-QIVIVE, and other methods to further improve the hazard assessment system. At the same time, gradually promote the construction of efficient platforms such as laboratory intelligence and digitization to effectively support the implementation of national strategies for food safety.
Starting from the 1950s, the modern food toxicology of China formed a relatively systematic toxicology discipline, with a large number of experts and technical backbones in the field of food toxicology. However, in recent years, the research, update, and application of novel technologies and methods required more trained toxicologists, and the technical strength and talent team building are worrying. It is urgent to strengthen the construction of professional teams in food toxicology in China to provide better human resources for food safety risk assessment. The development of NAMs relies on an adequate number of professional toxicologists. The increase in capacity and the improved technical training are the keys in the talent team construction.
In summary, CFSA is leading the development of NAMs for NGRA. The “Food Toxicology Program” has always focused on the core work of CFSA, practiced the concept of translating toxicological research, carried out key technical support work, and provided a solid toxicological database and scientific basis for food safety risk assessment and relevant standard formulation and revision. Not only promoting the traditional food toxicology, the “Food Toxicology Program” is also the key program for the development of NAMs of toxicology.
Based on the related research and the practical needs, CFSA is working on the strategies to develop NAMs for NGRA in China. Collaborating with other research institutions, CFSA will guide the development of NAMs in China and integrate the efforts of different institutions to develop the NAMs applicable for the regulatory food safety risk assessment.
DY: Writing–original draft, writing–review and editing. HY: Writing–original draft, Writing–review and editing. MS: Writing–original draft, Writing–review and editing. XJ: Writing–review and editing. HS: Conceptualization, Supervision, Writing–review and editing, Funding acquisition. ZL: Conceptualization, Writing–review and editing. YW: Conceptualization, Writing–review and editing.
The authors declare financial support was received for the research, authorship, and/or publication of this article. This work was supported by the National Natural Science Foundation of China under grant number 32372445, the National Natural Science Foundation of China under grant number 32061160474, and the National Key Research and Development Program of China under grant number 2022YFF1103100.
The authors declare that the research was conducted in the absence of any commercial or financial relationships that could be construed as a potential conflict of interest.
All claims expressed in this article are solely those of the authors and do not necessarily represent those of their affiliated organizations, or those of the publisher, the editors and the reviewers. Any product that may be evaluated in this article, or claim that may be made by its manufacturer, is not guaranteed or endorsed by the publisher.
AOP-Wiki, 2023 AOP-Wiki 2023. Available at: https://aopwiki.org/.
Arnot, J. A., Toose, L., Armitage, J. M., Sangion, A., Looky, A., Brown, T. N., et al. (2022). Developing an internal threshold of toxicological concern (iTTC). J. Expo. Sci. Environ. Epidemiol. 32 (6), 877–884. doi:10.1038/s41370-022-00494-x
Authority, E. F. S., Aguilera, J., Aguilera-Gomez, M., Barrucci, F., Cocconcelli, P. S., Davies, H., et al. (2018). EFSA Scientific Colloquium 24–'omics in risk assessment: state of the art and next steps. EFSA Support. Publ. 15 (11), 1512E. doi:10.2903/sp.efsa.2018.en-1512
Bell, S. M., Chang, X., Wambaugh, J. F., Allen, D. G., Bartels, M., Brouwer, K. L. R., et al. (2018). In vitro to in vivo extrapolation for high throughput prioritization and decision making. Toxicol. Vitro 47, 213–227. doi:10.1016/j.tiv.2017.11.016
Bernauer, U., Oberemm, A., Madle, S., and Gundert-Remy, U. (2005). The use of in vitro data in risk assessment. Basic and Clin. Pharmacol. Toxicol. 96 (3), 176–181. doi:10.1111/j.1742-7843.2005.pto960306.x
Bersani, C., Codagnone, J., David, L., Foiniotis, A., Galasso, G., Mancini, S., et al. (2022). Roadmap for actions on artificial intelligence for evidence management in risk assessment. EFSA Support. Publ. 19 (5). doi:10.2903/sp.efsa.2022.en-7339
Blaauboer, B. J. (2010). Biokinetic modeling and in vitro–in vivo extrapolations. J. Toxicol. Environ. Health, Part B. 13 (2-4), 242–252. doi:10.1080/10937404.2010.483940
Cagnoni, S., Emiliani, V., Lombardo, G., Alkema, W., Hooijmans, C., Alkema, S., et al. (2023). Implementing AI vertical use cases-scenario 1. EFSA Support. Publ. 20 (8), 8223E. doi:10.2903/sp.efsa.2023.en-8223
Cao, H., Zhi, Y., Xu, H., Fang, H., and Jia, X. (2019). Zearalenone causes embryotoxicity and induces oxidative stress and apoptosis in differentiated human embryonic stem cells. Toxicol. Vitro 54, 243–250. doi:10.1016/j.tiv.2018.09.020
Cattaneo, I., Astuto, M. C., Binaglia, M., Devos, Y., Dorne, JLCM, Fernandez Agudo, A., et al. (2023). Implementing new approach methodologies (NAMs) in food safety assessments: strategic objectives and actions taken by the European food safety authority. Trends Food Sci. Technol. 133, 277–290. doi:10.1016/j.tifs.2023.02.006
Cavalli, E., Verloo, D., Paraskevopoulos, K., Kleiner, J., Heppner, C., Hugas, M., et al. (2022). Theme (concept) paper-Artificial Intelligence in risk assessment. EFSA Support. Publ. 19 (5). doi:10.2903/sp.efsa.2022.e200501
CFSA (2022). Technical procedures for comprehensive evaluation of food safety risk assessment data quality. Beijing: China Quality and Standards Publishing and Media Co., Ltd Standards Press of China.
Chai, Z., Zhao, C., Jin, Y., Wang, Y., Zou, P., Ling, X., et al. (2021). Generating adverse outcome pathway (AOP) of inorganic arsenic-induced adult male reproductive impairment via integration of phenotypic analysis in comparative toxicogenomics database (CTD) and AOP wiki. Toxicol. Appl. Pharmacol. 411, 115370. doi:10.1016/j.taap.2020.115370
Chen, B., Wang, X., Zhang, Y., Zhang, W., Pang, X., Zhang, S., et al. (2023). Determination and risk assessment of flavor components in flavored milk. Foods 12 (11), 2151. doi:10.3390/foods12112151
Chen, C., Mi, X., Yuan, Y., Chen, G., Ren, L., Wang, K., et al. (2014). A preliminary risk assessment of potential exposure to naturally occurring estrogens from Beijing (China) market milk products. Food Chem. Toxicol. 71, 74–80. doi:10.1016/j.fct.2014.05.028
Cheng, W., Li, X., Zhou, Y., Yu, H., Xie, Y., Guo, H., et al. (2022). Polystyrene microplastics induce hepatotoxicity and disrupt lipid metabolism in the liver organoids. Sci. Total Environ. 806, 150328. doi:10.1016/j.scitotenv.2021.150328
Commission, E. Chemicals strategy for sustainability 2020. Available at: https://echa.europa.eu/hot-topics/chemicals-strategy-for-sustainability#:∼:text=The%20Commission’s%20strategy%20provides%20an,chemicals%20when%20assessing%20chemical%20risks.
Committee, E. S., More, S. J., Bampidis, V., Benford, D., Bragard, C., Halldorsson, T. I., et al. (2019). Guidance on the use of the Threshold of Toxicological Concern approach in food safety assessment. EFSA J. 17 (6), e05708. doi:10.2903/j.efsa.2019.5708
Cui, Y., Li, Z., Xiao, Q., Ge, J., Jiang, W., Wang, X., et al. (2023). 1,4-Naphthoquinone-coated black carbon nanoparticles up-regulation POR/FTL/IL-33 axis in THP1 cells. Ecotoxicol. Environ. Saf. 249, 114381. doi:10.1016/j.ecoenv.2022.114381
Deng, Z., Yao, L., Fang, M., Wu, Y., and Gong, Z. (2022). Health risk assessment using in vitro simulation in assessing bioavailability of cadmium in rice from main producing areas across China. J. Food Sci. 87 (6), 2766–2774. doi:10.1111/1750-3841.16158
Directive, E. C. (2010). 63/EU of the European Parliament and of the Council of 22 September 2010 on the protection of animals used for scientific purposes. Official J. Eur. Union, 50.
Dong, W., Hu, T., Zhang, Q., Deng, F., Wang, M., Kong, J., et al. (2023). Prediction of food safety risk level of wheat in China based on pyraformer neural network model for heavy metal contamination. Foods 12 (9), 1843. doi:10.3390/foods12091843
EFSA (2021). EFSA Strategy 2027 – science, safe food, sustainability. Available at: https://www.efsa.europa.eu/en/corporate-pubs/efsa-strategy-2027-science-safe-food-sustainability.
Epa, U. (2021). New approach methods work plan: reducing use of vertebrate animals in chemical testing.
EpoPP, P., Residues, t, Hernández-Jerez, A., Adriaanse, P., Aldrich, A., Berny, P., et al. (2021). Development of Integrated Approaches to Testing and Assessment (IATA) case studies on developmental neurotoxicity (DNT) risk assessment. EFSA J. 19 (6), e06599. doi:10.2903/j.efsa.2021.6599
EpoPP, P., Residues, t, Hernandez-Jerez, A. F., Adriaanse, P., Aldrich, A., Berny, P., et al. (2023). Development of adverse outcome pathways relevant for the identification of substances having endocrine disruption properties Uterine adenocarcinoma as adverse outcome. EFSA J. 21 (2), e07744. doi:10.2903/j.efsa.2023.7744
Escher, S. E., Partosch, F., Konzok, S., Jennings, P., Luijten, M., Kienhuis, A., et al. (2022). Development of a roadmap for action on new approach methodologies in risk assessment. EFSA Support. Publ. 19 (6), 7341E. doi:10.2903/sp.efsa.2022.en-7341
Fan, K., Guo, W., Huang, Q., Meng, J., Yao, Q., Nie, D., et al. (2021). Assessment of human exposure to five Alternaria mycotoxins in China by biomonitoring approach. Toxins 13 (11), 762. doi:10.3390/toxins13110762
Fang, H., Fang, W., Cao, H., Luo, S., Cong, J., Liu, S., et al. (2019). Di-(2-ethylhexyl)-phthalate induces apoptosis via the PPARγ/PTEN/AKT pathway in differentiated human embryonic stem cells. Food Chem. Toxicol. 131, 110552. doi:10.1016/j.fct.2019.05.060
Fang, H., Zhi, Y., Yu, Z., Lynch, R. A., and Jia, X. (2018). The embryonic toxicity evaluation of deoxynivalenol (DON) by murine embryonic stem cell test and human embryonic stem cell test models. Food control. 86, 234–240. doi:10.1016/j.foodcont.2017.10.018
Geng, Z., Duan, X., Li, J., Chu, C., and Han, Y. (2022). Risk prediction model for food safety based on improved random forest integrating virtual sample. Eng. Appl. Artif. Intell. 116, 105352. doi:10.1016/j.engappai.2022.105352
Geng, Z., Liu, F., Shang, D., Han, Y., Shang, Y., and Chu, C. (2021). Early warning and control of food safety risk using an improved AHC-RBF neural network integrating AHP-EW. J. Food Eng. 292, 110239. doi:10.1016/j.jfoodeng.2020.110239
Geng, Z., Zhao, S., Tao, G., and Han, Y. (2017). Early warning modeling and analysis based on analytic hierarchy process integrated extreme learning machine (AHP-ELM): application to food safety. Food control. 78, 33–42. doi:10.1016/j.foodcont.2017.02.045
Grimm, D. (2019). EPA plan to end animal testing splits scientists. American: American Association for the Advancement of Science.
Hao, Y., Sun, G., Fan, T., Sun, X., Liu, Y., Zhang, N., et al. (2019). Prediction on the mutagenicity of nitroaromatic compounds using quantum chemistry descriptors based QSAR and machine learning derived classification methods. Ecotoxicol. Environ. Saf. 186, 109822. doi:10.1016/j.ecoenv.2019.109822
Hardy, A., Benford, D., Halldorsson, T., Jeger, M. J., Knutsen, H. K., et al. (2017). Guidance on the use of the weight of evidence approach in scientific assessments. EFSA J. 15 (8), e04971. doi:10.2903/j.efsa.2017.4971
Hou, Z., Meng, R., Chen, G., Lai, T., Qing, R., Hao, S., et al. (2022). Distinct accumulation of nanoplastics in human intestinal organoids. Sci. Total Environ. 838, 155811. doi:10.1016/j.scitotenv.2022.155811
Hu, Y., Du, Z., Sun, X., Ma, X., Song, J., Sui, H., et al. (2021). Non-targeted analysis and risk assessment of non-volatile compounds in polyamide food contact materials. Food Chem. 345, 128625. doi:10.1016/j.foodchem.2020.128625
Hu, Y., Nguyen, S. V., Liu, C., Wang, W., Dong, Y., Fanning, S., et al. (2019). Complete genome and plasmid sequences of seven isolates of Salmonella enterica subsp. enterica harboring the mcr-1 gene obtained from food in China. Microbiol. Resour. Announc. 8 (31), e00114-19. doi:10.1128/mra.00114-19
Iacono, G., Guerra, B., Kass, G., Paraskevopoulos, K., Kleiner, J., Heppner, C., et al. (2022). Theme (concept) paper-application of OMICS and BIOINFORMATICS approaches: towards next generation risk assessment. EFSA Support. Publ. 19 (5). doi:10.2903/sp.efsa.2022.e200506
Ji, X., Deng, T., Xiao, Y., Jin, C., Lyu, W., Wu, Z., et al. (2023a). Emerging Alternaria and Fusarium mycotoxins in tomatoes and derived tomato products from the China market: occurrence, methods of determination, and risk evaluation. Food control. 145, 109464. doi:10.1016/j.foodcont.2022.109464
Ji, X., Jin, C., Xiao, Y., Deng, M., Wang, W., Lyu, W., et al. (2023b). Natural occurrence of regulated and emerging mycotoxins in wheat grains and assessment of the risks from dietary mycotoxins exposure in China. Toxins 15 (6), 389. doi:10.3390/toxins15060389
Ji, X., Xiao, Y., Jin, C., Wang, W., Lyu, W., Tang, B., et al. (2022b). Alternaria mycotoxins in food commodities marketed through e-commerce stores in China: occurrence and risk assessment. Food control. 140, 109125. doi:10.1016/j.foodcont.2022.109125
Ji, X., Xiao, Y., Lyu, W., Li, M., Wang, W., Tang, B., et al. (2022a). Probabilistic risk assessment of combined exposure to deoxynivalenol and emerging Alternaria toxins in cereal-based food products for infants and young children in China. Toxins 14 (8), 509. doi:10.3390/toxins14080509
Ji, X., Xiao, Y., Wang, W., Lyu, W., Wang, X., Li, Y., et al. (2022c). Mycotoxins in cereal-based infant foods marketed in China: occurrence and risk assessment. Food control. 138, 108998. doi:10.1016/j.foodcont.2022.108998
Jiang, T., Liu, T., Dong, W., Liu, Y., Hao, C., and Zhang, Q. (2022a). Prediction of safety risk levels of veterinary drug residues in freshwater products in China based on transformer. Foods 11 (12), 1690. doi:10.3390/foods11121690
Jiang, T., Liu, T., Dong, W., Liu, Y., and Zhang, Q. (2022b). Security risk level prediction of carbofuran pesticide residues in Chinese vegetables based on deep learning. Foods 11 (7), 1061. doi:10.3390/foods11071061
Jiang, W., Chu, H., Li, Z., Ge, J., Wang, X., Jiang, J., et al. (2022c). Integrated proteomic analysis to explore the molecular regulation mechanism of IL-33 mRNA increased by black carbon in the human endothelial cell line EA. hy926. Environ. Toxicol. 37 (10), 2434–2444. doi:10.1002/tox.23608
Knapen, D., Angrish, M. M., Fortin, M. C., Katsiadaki, I., Leonard, M., Margiotta-Casaluci, L., et al. (2018). Adverse outcome pathway networks I: development and applications. Environ. Toxicol. Chem. 37 (6), 1723–1733. doi:10.1002/etc.4125
Kong, X., Wang, X., Qin, Y., and Han, J. (2021). Effects of sunset yellow on proliferation and differentiation of intestinal epithelial cells in murine intestinal organoids. J. Appl. Toxicol. 41 (6), 953–963. doi:10.1002/jat.4080
Krewski, D., Acosta, D., Andersen, M., Anderson, H., Bailar, J. C., Boekelheide, K., et al. (2010). Toxicity testing in the 21st century: a vision and a strategy. J. Toxicol. Environ. Health, Part B. 13 (2-4), 51–138. doi:10.1080/10937404.2010.483176
Li, H., Yuan, H., Middleton, A., Li, J., Nicol, B., Carmichael, P., et al. (2021a). Next generation risk assessment (NGRA): bridging in vitro points-of-departure to human safety assessment using physiologically-based kinetic (PBK) modelling – a case study of doxorubicin with dose metrics considerations. Toxicol. Vitro 74, 105171. doi:10.1016/j.tiv.2021.105171
Li, M., Gong, J., Ge, L., Gao, H., Yang, J., Yang, C., et al. (2022a). Development of human retinal organoid models for bisphenol toxicity assessment. Ecotoxicol. Environ. Saf. 245, 114094. doi:10.1016/j.ecoenv.2022.114094
Li, W., Cui, Q., Bai, L., Fu, P., Han, H., Liu, J., et al. (2021b). Application of whole-genome sequencing in the national molecular tracing network for foodborne disease surveillance in China. Foodborne Pathogens Dis. 18 (8), 538–546. doi:10.1089/fpd.2020.2908
Li, Z., Jiang, W., Chu, H., Ge, J., Wang, X., Jiang, J., et al. (2022b). Exploration of potential mechanism of interleukin-33 up-regulation caused by 1,4-naphthoquinone black carbon in RAW264.7 cells. Sci. Total Environ. 835, 155357. doi:10.1016/j.scitotenv.2022.155357
Liu, Q., Zhou, P., Luo, P., and Wu, P. (2023a). Occurrence of furfural and its derivatives in coffee products in China and estimation of dietary intake. Foods 12 (1), 200. doi:10.3390/foods12010200
Liu, Z., Wang, S., Zhang, Y., Feng, Y., Liu, J., and Zhu, H. (2023b). Artificial intelligence in food safety: a decade review and bibliometric analysis. Foods 12 (6), 1242. doi:10.3390/foods12061242
Low, P., Panksepp, J., Reiss, D., Edelman, D., Van Swinderen, B., and Koch, C. (Editors) (2012). The Cambridge declaration on consciousness (Cambridge, England: Francis crick memorial conference).
Lu, P., Dong, W., Jiang, T., Liu, T., Hu, T., and Zhang, Q. (2023). Informer-based safety risk prediction of heavy metals in rice in China. Foods 12 (3), 542. doi:10.3390/foods12030542
Lyu, B., Li, J., and Wu, Y. (2022). Characterizing the exposome of food contamination and China total Diet study: project for improving food safety risk assessment in China. China CDC Wkly. 4 (9), 157–160. doi:10.46234/ccdcw2022.039
Ma, X., Sui, H., Sun, X., Ali, M. M., Debrah, A. A., and Du, Z. (2021). A risk classification strategy for migrants of food contact material combined with three (Q)SAR tools in silico. J. Hazard. Mater. 419, 126422. doi:10.1016/j.jhazmat.2021.126422
OECD (2020). Overview of concepts and available guidance related to integrated approaches to testing and assessment (IATA), OECD series on testing and assessment, No. 329. Environment, health and safety. China: Environment Directorate, OECD.
OECD. Integrated approaches to testing and assessment (IATA). Available at: https://www.oecd.org/chemicalsafety/risk-assessment/iata/.
OECD. Adverse outcome pathways, molecular screening and toxicogenomics. Available at: https://www.oecd.org/chemicalsafety/testing/adverse-outcomepathways-molecular-screening-and-toxicogenomics.htm.
OECD. OECD series on adverse outcome pathways. Available at: https://www.oecdilibrary.org/environment/oecd-series-on-adverse-outcome-pathways_2415170x.
AOP-Wiki (2023). Available at: https://aopwiki.org/.
Partnership for the Assessment Partnership for the assessment of risks from chemicals (PARC). Available at: https://www.eu-parc.eu/.
Peng, H., Zhang, L., He, J., Liang, J., and Guo, J. (2021). Research progress in adverse outcome pathway related to drug-induced liver injury. Chin. J. Pharmacol. Toxicol. 35 (11), 868–876. doi:10.3867/j.issn.1000-3002.2021.11.009
Peng, Z., Maciel-Guerra, A., Baker, M., Zhang, X., Hu, Y., Wang, W., et al. (2022). Whole-genome sequencing and gene sharing network analysis powered by machine learning identifies antibiotic resistance sharing between animals, humans and environment in livestock farming. PLoS Comput. Biol. 18 (3), e1010018. doi:10.1371/journal.pcbi.1010018
Qiao, X., Li, G., Zhang, J., Du, J., Yang, Y., Yin, J., et al. (2022). Urinary analysis reveals high Alternaria mycotoxins exposure in the general population from Beijing, China. J. Environ. Sci. 118, 122–129. doi:10.1016/j.jes.2021.08.025
Rietjens, I. M., Louisse, J., and Punt, A. (2011). Tutorial on physiologically based kinetic modeling in molecular nutrition and food research. Mol. Nutr. food Res. 55 (6), 941–956. doi:10.1002/mnfr.201000655
Schmidt, S. (2022). Targeting the macrophage: immune cells may Be the key to phthalate-induced liver toxicity. Environ. health Perspect. 130 (3), 034003. doi:10.1289/EHP11026
Shao, B., Li, H., Shen, J., and Wu, Y. (2019). Nontargeted detection methods for food safety and integrity. Annu. Rev. food Sci. Technol. 10, 429–455. doi:10.1146/annurev-food-032818-121233
Shi, M., Dong, Y., Bouwmeester, H., Rietjens, IMCM, and Strikwold, M. (2022). In vitro–in silico-based prediction of inter-individual and inter-ethnic variations in the dose-dependent cardiotoxicity of R- and S-methadone in humans. Archives Toxicol. 96 (8), 2361–2380. doi:10.1007/s00204-022-03309-y
Sreedhar, D., Manjula, N., Pise, S., Ligade, V., and Vs, L. (2020). Ban of cosmetic testing on animals: a brief overview. Int. J. Curr. Res. Rev. 12, 113–116. doi:10.31782/ijcrr.2020.121424
Sui, H., Zhang, L., Mao, W., Li, J., Liu, A., and Liu, Z. (2012). Establishment of the threshold of toxicological concern with decision tree approach and its application in food contact materials. Chin. J. Food Hyg. 24, 109–113. doi:10.13590/j.cjfh.2012.02.007
Sun, G., Zhang, Y., Pei, L., Lou, Y., Mu, Y., Yun, J., et al. (2021). Chemometric QSAR modeling of acute oral toxicity of Polycyclic Aromatic Hydrocarbons (PAHs) to rat using simple 2D descriptors and interspecies toxicity modeling with mouse. Ecotoxicol. Environ. Saf. 222, 112525. doi:10.1016/j.ecoenv.2021.112525
Villeneuve, D. L., Angrish, M. M., Fortin, M. C., Katsiadaki, I., Leonard, M., Margiotta-Casaluci, L., et al. (2018). Adverse outcome pathway networks II: network analytics. Environ. Toxicol. Chem. 37 (6), 1734–1748. doi:10.1002/etc.4124
Viviani, B., Bernardini, E., Galbiati, V., Maddalon, A., Melzi, G., Midali, M., et al. (2023). Development of Adverse Outcome Pathways relevant for the identification of substances having endocrine disruptors properties. EFSA Support. Publ. 20 (2), 7748E. doi:10.2903/sp.efsa.2023.en-7748
Wadman, M. (2023). FDA no longer has to require animal testing for new drugs. Sci. (New York, NY) 379 (6628), 127–128. doi:10.1126/science.adg6276
Wambaugh, J. F., Bare, J. C., Carignan, C. C., Dionisio, K. L., Dodson, R. E., Jolliet, O., et al. (2019). New approach methodologies for exposure science. Curr. Opin. Toxicol. 15, 76–92. doi:10.1016/j.cotox.2019.07.001
Wang, W., Baloch, Z., Zou, M., Dong, Y., Peng, Z., Hu, Y., et al. (2018). Complete genomic analysis of a Salmonella enterica serovar Typhimurium isolate cultured from ready-to-eat pork in China carrying one large plasmid containing mcr-1. Front. Microbiol. 9, 616. doi:10.3389/fmicb.2018.00616
Wang, Z., Chen, J., and Hong, H. (2021). Developing QSAR models with defined applicability domains on PPARγ binding affinity using large data sets and machine learning algorithms. Environ. Sci. Technol. 55 (10), 6857–6866. doi:10.1021/acs.est.0c07040
Wang, Z., Wu, Z., Zou, M., Wen, X., Wang, Z., Li, Y., et al. (2022). A voting-based ensemble deep learning method focused on multi-step prediction of food safety risk levels: applications in hazard analysis of heavy metals in grain processing products. Foods 11 (6), 823. doi:10.3390/foods11060823
Wiklund, L., Caccia, S., Pípal, M., Nymark, P., and Beronius, A. (2023). Development of a data-driven approach to Adverse Outcome Pathway network generation: a case study on the EATS-modalities. Front. Toxicol. 5, 1183824. doi:10.3389/ftox.2023.1183824
Wu, Y. (2012). Translational toxicology and exposomics for food safety risk management. J. Transl. Med. 10 (2), A41. doi:10.1186/1479-5876-10-s2-a41
Wu, Y.-n., Liu, P., and Chen, J.-s. (2018). Food safety risk assessment in China: past, present and future. Food control. 90, 212–221. doi:10.1016/j.foodcont.2018.02.049
Xie, R., Wang, X., Xu, Y., Zhang, L., Ma, M., and Wang, Z. (2023). In vitro to in vivo extrapolation for predicting human equivalent dose of phenolic endocrine disrupting chemicals: PBTK model development, biological pathways, outcomes and performance. Sci. Total Environ. 897, 165271. doi:10.1016/j.scitotenv.2023.165271
Xu, J., Wang, Z., Zhang, X., Yu, J., Cui, X., Zhou, Y., et al. (2022b). A rice security risk assessment method based on the fusion of multiple machine learning models. Agriculture 12 (6), 815. doi:10.3390/agriculture12060815
Xu, J.-Y., Wang, K., Men, S.-H., Yang, Y., Zhou, Q., and Yan, Z.-G. (2023). QSAR-QSIIR-based prediction of bioconcentration factor using machine learning and preliminary application. Environ. Int. 177, 108003. doi:10.1016/j.envint.2023.108003
Xu, M., Li, Y., Wang, X., Zhang, Q., Wang, L., Zhang, X., et al. (2022a). Role of hepatocyte-and macrophage-specific PPAR γ in hepatotoxicity induced by diethylhexyl phthalate in mice. Environ. Health Perspect. 130 (1), 017005. doi:10.1289/ehp9373
Yang, R., Wang, X., Gao, Q., Sang, C., Zhao, Y., Niu, Y., et al. (2023). Dietary exposure and health risk of the emerging contaminant fluorinated liquid-crystal monomers. Environ. Sci. Technol. 57 (15), 6309–6319. doi:10.1021/acs.est.3c00322
Yao, L., Wang, Y., Deng, Z., Wu, Q., Fang, M., Wu, Y., et al. (2021). Study on the bioaccessibility and bioavailability of Cd in contaminated rice in vitro and in vivo. J. Food Sci. 86 (8), 3730–3742. doi:10.1111/1750-3841.15829
Yong, L., Yan, Y., Liu, Z., Zhang, L., Sui, H., and Song, Y. (2016). Risk assessment of dimethyl 1, 2-benzenedicarboxylate and 1, 2-benzenedicarboxylic acid diethyl ester with the threshold of toxicological concern approach. Chin. J. Food Hyg. 28 (2), 254–258. doi:10.13590/j.cjfh.2016.02.024
Yu, J., Shen, S., Yan, Y., Liu, L., Luo, R., Liu, S., et al. (2023). Iodide excess inhibits thyroid hormone synthesis pathway involving XBP1-mediated regulation. Nutrients 15 (4), 887. doi:10.3390/nu15040887
Yu, L., Li, H., Zhang, C., Zhang, Q., Guo, J., Li, J., et al. (2020). Integrating in vitro testing and physiologically-based pharmacokinetic (PBPK) modelling for chemical liver toxicity assessment—a case study of troglitazone. Environ. Toxicol. Pharmacol. 74, 103296. doi:10.1016/j.etap.2019.103296
Zhang, C., Zhang, Q., Li, J., Yu, L., Li, F., Li, W., et al. (2020a). Integration of in vitro data from three dimensionally cultured HepaRG cells and physiologically based pharmacokinetic modeling for assessment of acetaminophen hepatotoxicity. Regul. Toxicol. Pharmacol. 114, 104661. doi:10.1016/j.yrtph.2020.104661
Zhang, Z., Zhu, Q., Huang, C., Yang, M., Li, J., Chen, Y., et al. (2020b). Comparative cytotoxicity of halogenated aromatic DBPs and implications of the corresponding developed QSAR model to toxicity mechanisms of those DBPs: binding interactions between aromatic DBPs and catalase play an important role. Water Res. 170, 115283. doi:10.1016/j.watres.2019.115283
Zhao, K., Shao, B., Yang, D., and Li, F. (2015b). Natural occurrence of four Alternaria mycotoxins in tomato- and citrus-based foods in China. J. Agric. Food Chem. 63 (1), 343–348. doi:10.1021/jf5052738
Zhao, K., Shao, B., Yang, D., Li, F., and Zhu, J. (2015a). Natural occurrence of Alternaria toxins in wheat-based products and their dietary exposure in China. PLoS One 10 (6), e0132019. doi:10.1371/journal.pone.0132019
Zhong, H., Chen, J., and Feng, J. (2017). Safety assessment of non-intentionally added substance migrated from food contact material. Chin. J. Food Hyg. 29 (2), 238–243. doi:10.13590/j.cjfh.2017.02.026
Zhong, J., Sun, L., Zuo, E., Chen, C., Chen, C., Jiang, H., et al. (2023). An ensemble of AHP-EW and AE-RNN for food safety risk early warning. Plos one 18 (4), e0284144. doi:10.1371/journal.pone.0284144
Zhou, H., Xu, A., Liu, M., Yan, Z., Qin, L., Liu, H., et al. (2022a). Mycotoxins in wheat flours marketed in Shanghai, China: occurrence and dietary risk assessment. Toxins 14 (11), 748. doi:10.3390/toxins14110748
Keywords: next-generation risk assessment, new approach methodologies, China, China National Center for Food Safety Risk Assessment, food toxicology
Citation: Yang D, Yang H, Shi M, Jia X, Sui H, Liu Z and Wu Y (2023) Advancing food safety risk assessment in China: development of new approach methodologies (NAMs). Front. Toxicol. 5:1292373. doi: 10.3389/ftox.2023.1292373
Received: 11 September 2023; Accepted: 07 November 2023;
Published: 17 November 2023.
Edited by:
Anna Beronius, Karolinska Institutet (KI), SwedenReviewed by:
Dimitra Nikolopoulou, Benaki Phytopathological Institute, GreeceCopyright © 2023 Yang, Yang, Shi, Jia, Sui, Liu and Wu. This is an open-access article distributed under the terms of the Creative Commons Attribution License (CC BY). The use, distribution or reproduction in other forums is permitted, provided the original author(s) and the copyright owner(s) are credited and that the original publication in this journal is cited, in accordance with accepted academic practice. No use, distribution or reproduction is permitted which does not comply with these terms.
*Correspondence: Haixia Sui, c3VpaGFpeGlhQGNmc2EubmV0LmNu; Zhaoping Liu, bGl1emhhb3BpbmdAY2ZzYS5uZXQuY24=
Disclaimer: All claims expressed in this article are solely those of the authors and do not necessarily represent those of their affiliated organizations, or those of the publisher, the editors and the reviewers. Any product that may be evaluated in this article or claim that may be made by its manufacturer is not guaranteed or endorsed by the publisher.
Research integrity at Frontiers
Learn more about the work of our research integrity team to safeguard the quality of each article we publish.