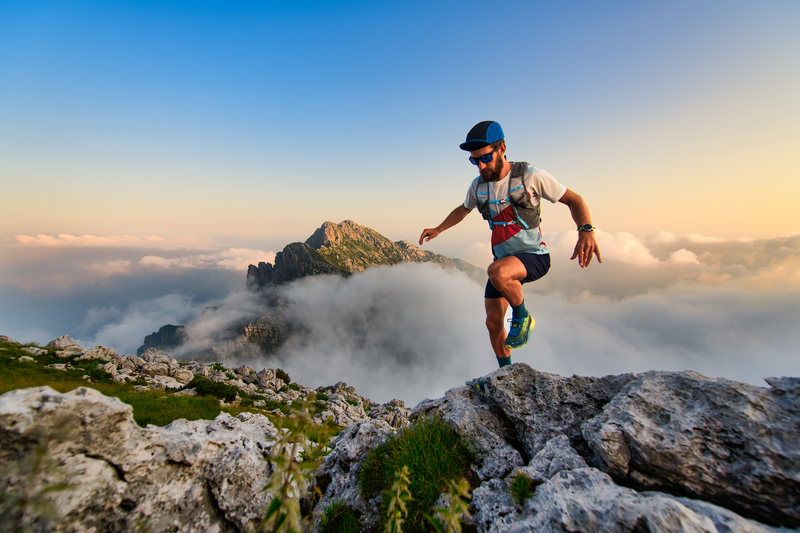
94% of researchers rate our articles as excellent or good
Learn more about the work of our research integrity team to safeguard the quality of each article we publish.
Find out more
ORIGINAL RESEARCH article
Front. Syst. Neurosci.
Volume 19 - 2025 | doi: 10.3389/fnsys.2025.1531795
The final, formatted version of the article will be published soon.
You have multiple emails registered with Frontiers:
Please enter your email address:
If you already have an account, please login
You don't have a Frontiers account ? You can register here
As users transition from drivers to passengers in automated vehicles, they often take their eyes off the road to engage in non-driving activities. In driving simulators, visual motion is presented with scaled or without physical motion, leading to a mismatch between expected and perceived motion. Both conditions elicit motion sickness, calling for enhanced vehicle and simulator motion control strategies. Given the large differences in sickness susceptibility between individuals, effective countermeasures must address this at a personal level. This paper combines a group-averaged sensory conflict model with an individualised Accumulation Model (AM) to capture individual differences in motion sickness susceptibility across various conditions. The feasibility of this framework is verified using three datasets involving sickening conditions: 1) vehicle experiments with and without outside vision, 2) corresponding vehicle and driving simulator experiments, and 3) vehicle experiments with various non-driving-related tasks. All datasets involve passive motion, mirroring experience in automated vehicles. The preferred model (AM2) can fit individual motion sickness responses across conditions using only two individualised parameters (gain K 1 and time constant T 1 ) instead of the original five, ensuring unique parameters for each participant and generalisability across conditions. An average improvement factor of 1.7 in fitting individual motion sickness responses is achieved with the AM2 model compared to the group-averaged AM0 model. This framework demonstrates robustness by accurately modelling distinct motion and vision conditions. A Gaussian mixture model of the parameter distribution across a population is developed, which predicts motion sickness in an unseen dataset with an average RMSE of 0.47. This model reduces the need for large-scale population experiments, accelerating research and development.
Keywords: Motion Sickness, Simulator sickness, modeling, driving simulators, automated vehicles
Received: 20 Nov 2024; Accepted: 13 Feb 2025.
Copyright: © 2025 Kotian, Pool and Happee. This is an open-access article distributed under the terms of the Creative Commons Attribution License (CC BY). The use, distribution or reproduction in other forums is permitted, provided the original author(s) or licensor are credited and that the original publication in this journal is cited, in accordance with accepted academic practice. No use, distribution or reproduction is permitted which does not comply with these terms.
* Correspondence:
Varun Kotian, Department of Cognitive Robotics, Faculty of Mechanical, Maritime and Materials Engineering, Delft University of Technology, Delft, Netherlands
Disclaimer: All claims expressed in this article are solely those of the authors and do not necessarily represent those of their affiliated organizations, or those of the publisher, the editors and the reviewers. Any product that may be evaluated in this article or claim that may be made by its manufacturer is not guaranteed or endorsed by the publisher.
Research integrity at Frontiers
Learn more about the work of our research integrity team to safeguard the quality of each article we publish.