- 1Behavioural Neuroscience Lab, Institute of Psychology, SWPS University of Social Sciences and Humanities, Warsaw, Poland
- 2Faculty of Physics, University of Warsaw, Warsaw, Poland
The assessment of the level of consciousness in disorders of consciousness (DoC) is still one of the most challenging problems in contemporary medicine. Nevertheless, based on the multitude of studies conducted over the last 20 years on resting states based on electroencephalography (EEG) in DoC, it is possible to outline the brain activity profiles related to both patients without preserved consciousness and minimally conscious ones. In the case of patients without preserved consciousness, the dominance of low, mostly delta, frequency, and the marginalization of the higher frequencies were observed, both in terms of the global power of brain activity and in functional connectivity patterns. In turn, the minimally conscious patients revealed the opposite brain activity pattern—the characteristics of higher frequency bands were preserved both in global power and in functional long-distance connections. In this short review, we summarize the state of the art of EEG-based research in the resting state paradigm, in the context of providing potential support to the traditional clinical assessment of the level of consciousness.
1. Introduction
After severe brain damage, some patients suffer from disorders of consciousness (DoC), such as coma, unresponsive wakefulness syndrome (UWS), or minimally conscious state (MCS). In patients with UWS, no behavioral signs of consciousness are observed (Giacino et al., 2004). Those in MCS manifest volitional reactions, such as visual pursuit, pain localization (MCS), and/or command following movements (MCS+) but no communication is possible (Bruno et al., 2011). However, as Gosseries et al. (2014) and Thibaut et al. (2021) pointed out, some patients with UWS have brain activity patterns characteristic of MCS but no traces of consciousness are observed in their behavior. These patients are named non-behavioral MCS (MCS *). Establishing communication and/or regaining the skill of functional use of an object indicates the emergence of MCS (eMCS) and recovery of consciousness (Giacino et al., 2002, 2004). Electroencephalography (EEG) is a common diagnostic technique in routine clinical neurological management. Due to its mobility and wide availability, it offers an indication of a patient's brain functioning, allowing for diagnosis and regular monitoring of their condition. In the case of patients with DoC, brain activity shows huge inter-patient variability. Following a brain injury, EEG can be altered and many abnormal patterns can be observed, e.g., diffuse slowing of activity, polymorphic focal delta rhythm over damaged regions (Brenner, 2005), asymmetries related to brain damage, burst-suppression pattern, and epileptiform activity (Lehembre et al., 2012b). The clearest way to show the presence of consciousness is to use active experimental procedures, where a patient's direct engagement in following instructions is required. However, in the case of patients with DoC, this paradigm has several limitations, including the fluctuation of attention, aphasia, or sensory and motor deficits. These limitations are not relevant in the resting-state EEG analysis, which requires only maintaining an arousal state, without a necessity for language comprehension or patient's active cooperation. The application of resting-state EEG analysis makes it possible to determine the electrical activity of the awake brain in the absence of tasks and instructions (Bai et al., 2017).
The resting state networks were first described in 1995. Using functional magnetic resonance imaging (fMRI), it has been shown that the brains of people who were not given any task to do experienced slow and synchronous changes in sensomotoric regions of the brain that showed so-called “connectivity” relation to one's activities while resting (Biswal et al., 1995). Similar patterns of resting state coherence have been documented in other brain areas, called the default mode network (DMN), and these brain regions are involved in self-referential thinking, emotional processing, and recalling memories (Raichle et al., 2001; Raichle, 2015; Shen, 2015). Using positron emission tomography (PET) and fMRI techniques, many other brain functional networks have been demonstrated at rest to show coherent fluctuations at low frequency (0.01–0.1 Hz), related to active sensory processing (Fox et al., 2005; Damoiseaux et al., 2006). However, it is indicated that the fMRI blood-oxygen-level dependent (BOLD) response may represent the summed neuronal activity observable with EEG signal (Whitman et al., 2013). Brain connectivity can be subdivided into structural and functional connectivity. The former focuses on neuroanatomical links between particular brain structures, the latter reflects the statistical dependencies between the activity of spatially distinct brain areas (Friston, 2011). Functional connectivity is usually measured by correlation, coherence, and information theory (Cao et al., 2021). While functional connectivity can be assessed based on BOLD and EEG signals, structural connections cannot be revealed by the EEG technique. Usually, functional connections are quantified either in frequency or time domain, in particular EEG bands, such as δ, θ, α, β, and γ or for range of the EEG signal, creating so-called EEG networks.
The analyses derived from the resting-state EEG are related to the fundamental state of the brain and seem to help monitor the patient's state of consciousness (Sitt et al., 2014; Bai et al., 2017, 2021; Sebastiano et al., 2021; Wutzl et al., 2021). Owing to the properties of the EEG measurement itself, the indices obtained reflect other aspects of brain functioning, e.g., the frequencies of characteristic rhythms. The most common approaches to the analysis of resting-state activity include the assessment of spectral power, signal complexity, and functional connectivity. The use of resting state EEG in DoC should make it possible to assess the severity of brain damage, bringing us closer to a prognosis about the patient's further condition and understanding the processes acting between different states of consciousness. However, the heterogeneity of patients with DoC and the current means of application of analytical algorithms to resting EEG signals significantly impede data interpretation.
Therefore, the aim of this study is to summarize and discuss EEG resting data based on 38 studies related to the abovementioned entities. As well as outlining aspects of EEG activity that are crucial to the differentiation between unconscious and minimally conscious patients, we also show which of them have prognostic properties.
2. Method: Search Strategy
The search covered three literature databases (EBSCO, Pubmed, Google Scholar). The following keywords were used as search criteria: resting state EEG and unresponsive wakefulness syndrome and minimally conscious, rs DoC, connectivity DoC. In addition, we identified additional references from those provided in the retrieved articles. A total of 2,081 articles were identified (refer to Figure 1). Subsequently, we screened the retrieved articles for the following inclusion criteria: (a) they were peer-reviewed or conference proceedings and abstracts; b) more than one patient participated in the study; c) only quantitative data analysis was used; d) resting state during wakefulness (without sleep periods) EEG was reported. A detailed comparison of all reviewed studies is summarized in Table 1.
3. Spectral Characteristics of EEG in DoC
One of the most popular methods used to assess oscillatory brain activity is the analysis of power spectrum density. The power of brain activity was reduced overall in patients with DoC compared with healthy controls and those with severe neurological cognitive damage (Leon-Carrion et al., 2008; Babiloni et al., 2009; Naro et al., 2016, 2018). A more detailed analysis showed an increase in slower band power (δ, θ) and a prominent drop-off in higher bands (α, β) in the patient group, so the decrease of power is not uniform (Chennu et al., 2014; van den Brink et al., 2018), refer to Table 1. The power of faster oscillations is significantly reduced in patients with DoC (Coleman et al., 2005; Babiloni et al., 2009; Chennu et al., 2014; Naro et al., 2018; Stefan et al., 2018). In the case of patients with UWS, in comparison with those with MCS, brain activity is dominated by the low oscillation of the δ band (Lehembre et al., 2012a; Höller et al., 2014; Sitt et al., 2014; Naro et al., 2016, 2018; Piarulli et al., 2016; Stefan et al., 2018; Lutkenhoff et al., 2019) (refer to Figure 2). A significant increase in the δ band in patients with UWS in comparison with MCS and healthy controls was observed mainly in frontal areas (Lehembre et al., 2012a; Naro et al., 2016). As noted by Chennu et al. (2014) and Naro et al. (2018), about 80% of the overall spectral power was concentrated within the lowest EEG band, suggesting a severe impairment of cortico-thalamo connections (Gloor et al., 1977).
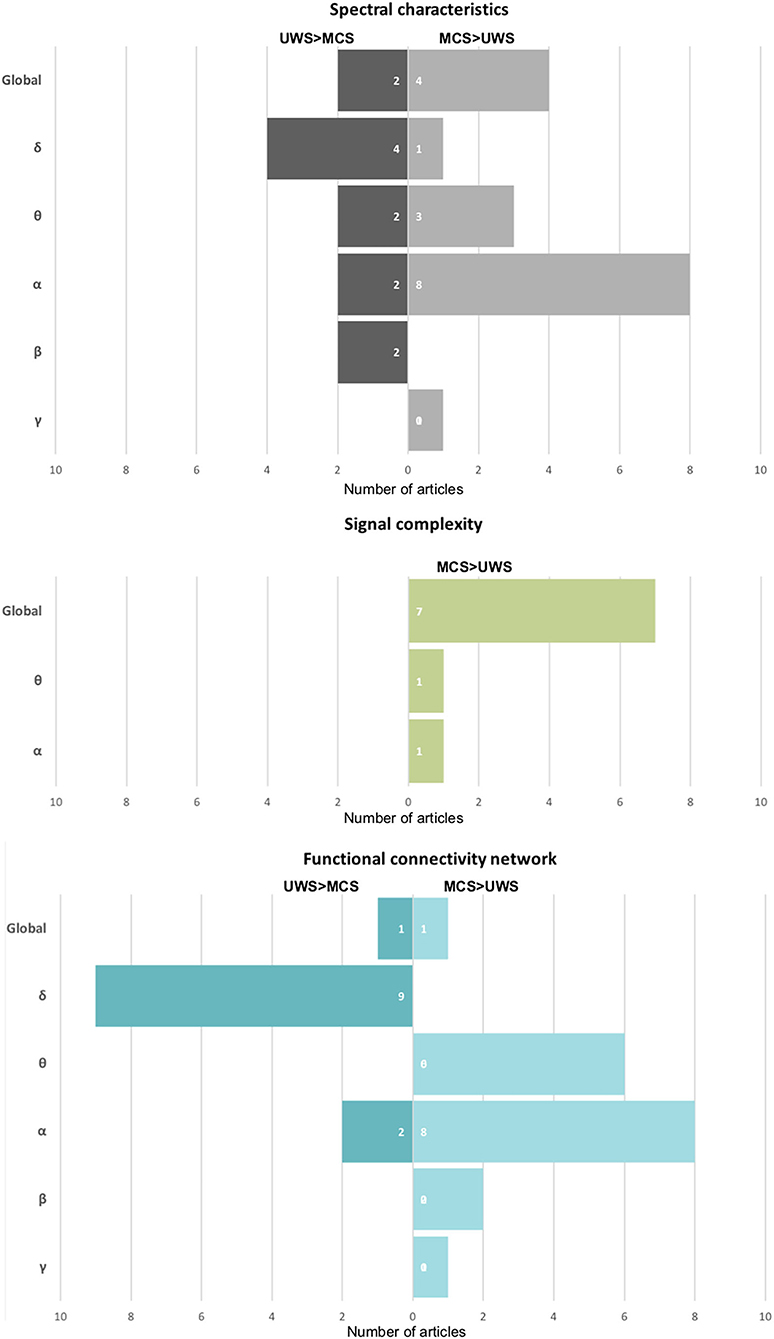
Figure 2. The summary of the number of publications shows a particular effect on spectral characteristics, signal complexity, and functional connectivity networks. On the left side of the chart: a stronger effect in UWS in comparison to MCS, on the right one—opposite—a stronger effect in MCS than in patients with UWS.
In turn, patients with MCS were usually characterized by stronger activity in higher bands (θ, α, β) and weaker in the δ band compared with UWS (Lechinger et al., 2013; Sitt et al., 2014; Naro et al., 2016, 2018; Lei et al., 2022). Thibaut et al. (2021) showed similar differences between patients with MCS* and UWS. In particular, the presence of an α band in the parietal areas seems to be crucial for consciousness (Babiloni et al., 2009; Lehembre et al., 2012a; Sitt et al., 2014; Naro et al., 2016) (refer to Figure 2). As shown by Babiloni et al. (2009), in the case of patients with UWS who had not recovered, the α band in the posterior areas was extremely weak, which seemed to be a reliable predictor of a bad outcome.
The results mentioned above are in line with the clinical observations based on visual inspection of the EEG signal of patients with DoC. Schiff (2016) proposed a theoretical framework to assess the integrity of thalamo-cortical and cortico-cortical networks. According to this theory, a higher level of anterior forebrain corticothalamic integrity is correlated with a higher level of consciousness, independent of the degree of injury to other brain areas (Schiff, 2016; Forgacs et al., 2017, 2020). The condition of thalamo-cortical integrity after severe brain injury is reflected in four different EEG regimes assessed via the signal spectrum related to predominant frequency power: A type—mostly δ activity (below 4 Hz); B type-θ activity (5–7 Hz); C type-θ, and β (15–40 Hz) activity; D type-α (8–12 Hz) and β activity. Based on a model “ABCD” spectral pattern, “A” indicates complete loss of corticothalamic integrity; “D” indicates full recovery of corticothalamic integrity; while “B” and “C” represent interim regimes with distinct physiological foundations (Schiff, 2016; Forgacs et al., 2017). The low oscillations of δ activity, uniform over the scalp, observed in patients with UWS correspond to an “A” type EEG regime and are interpreted as corticothalamic deafferentation—caused either by the impairment of synaptic transmission (van Putten and Hofmeijer, 2016) or by white matter lesions (Gloor et al., 1977). Regarding patients with MCS, the vast majority of studies showed stronger θ activity in MCS in comparison with UWS. This seems to be related to the preservation of a certain amount of afferent input to cortical neurons, which corresponds to “B” and “C” type EEG regimes. Such a hypothesis confirms stronger θ activity in patients with MCS in comparison to severe neurocognitive disorders (SND), which suggests that loss of consciousness is related to deeper brain injury, a low level of afferent input to cortical neurons, and stronger spontaneous oscillation of Layer V pyramidal cells. In comparison with patients with UWS, MCS brain activity is characterized by stronger α band oscillations that reflect normal tonic firing, and seem to be related to preserved corticothalamic integrity but at a lower level than in the controls (refer to Table 1).
4. Signal Complexity in Patients With DoC
The concept of entropy, first proposed as a thermodynamic principle, is now used widely to quantify the effects of anesthetic drugs on brain activity. EEG signal entropy methods may be based on time series (e.g., Approximate Entropy) and phase space analysis (e.g., Phase Lag Entropy), or on the frequency spectrum (e.g., Spectral Entropy) (Bandt and Pompe, 2002). Although these algorithms are sensitive to different properties of the signal, such as spectral bandwidth and amplitude distribution, and are generally difficult to interpret, they tend to have lower values if the signal slows down and becomes less complex (Ferenets et al., 2006). Different measures of entropy were used to assess differences in signal complexity among patients with DoC (Table 1). In most of the studies, these methods show a coherent picture—the global EEG signal complexity was lower in patients with UWS than in those with MCS (Sarà and Pistoia, 2010; Gosseries et al., 2011; Sarà et al., 2011; Wu et al., 2011b; Sitt et al., 2014; Stefan et al., 2018; Lee et al., 2019a) (refer to Figure 2). In particular, patients with MCS were characterized by more complex interactions between the activity of brain regions (Lee et al., 2019a), and by higher variability and periodicity of spectral entropy over time (Piarulli et al., 2016). As shown by Lei et al. (2022) in the longitudinal scheme, different entropy measures (Approximate Entropy, Sample Entropy, and Lempel-Ziv complexity) increased non-monotonically with the inflection point when the patients transferred from UWS to MCS. These results suggest that the transition from unconscious to conscious is not a linear phenomenon.
The best discrimination between UWS and conscious patient groups was obtained in θ (Sitt et al., 2014) and α bands (Stefan et al., 2018) (refer to Table 1). Moreover, lower values of complexity measures were observed in many studies on the effects of anesthetics (Liang et al., 2015) and sleep (Bruce et al., 2009) during the loss of consciousness.
5. Changes in Network Functional Connectivity in Patients With DoC
Brain function relies on the co-activation of several structures in functional networks (Damoiseaux et al., 2006). Some of them (i.e., the default mode network, DMN) seem to be related to the mechanisms of consciousness (Mason et al., 2007; Boly et al., 2008; Boveroux et al., 2010). While the complexity of brain networks is indirectly reflected by entropy measures as discussed in the previous section, the most detailed information about the functioning of the brain is provided by an examination of network functional connections. In the case of the EEG signal, there are many approaches to the assessment of this phenomenon, which have been applied to assess brain function in patients with DoC (for review refer to Bai et al., 2017; Corchs et al., 2019; Table 1). In the following subsection, we turn our attention to network functional connectivity in UWS and MCS.
5.1. Changes in Network Functional Connectivity in Patients With UWS
Considered at a global level, patients with UWS exhibit general deficits in information sharing, observed mainly over central and posterior areas and across medium-to-long distances (King et al., 2013). This has been interpreted as possibly related to disrupted thalamo-cortical and cortico-cortical interactions due to diffuse white matter lesions common in patients with persistent UWS and hypoactivation of the DMN, encompassing the precuneus and posterior cingulate cortex located over centro-parietal regions. This interpretation was supported by fMRI studies (Vanhaudenhuyse et al., 2009; Demertzi et al., 2015). Moreover, the analysis of particular bands showed more detailed, specific functional connectivity patterns related to the preserved level of consciousness. The most common pattern in patients with UWS, as compared with MCS and healthy controls, contains a decrease in the number of functional connections in higher bands (mostly α and θ) and stronger communication in the δ band-similar to the spectral power in these bands, as described in Section 3 (Höller et al., 2014; Sitt et al., 2014). Reduced functional connectivity patterns in frequencies higher than δ (mostly α and θ) in patients with UWS, compared with MCS, have been revealed in many studies (Lehembre et al., 2012a; Cavinato et al., 2014; Sitt et al., 2014; Chennu et al., 2017; Naro et al., 2018; van den Brink et al., 2018) (refer to Figure 2 and Table 1). The most significant impairment of information flow was observed over fronto-posterior areas (Lehembre et al., 2012a) in both θ (Lehembre et al., 2012a) and α (Cavinato et al., 2014; Chennu et al., 2017), which are presumably related to the preserved consciousness (Sitt et al., 2014; Chennu et al., 2017) (discussed in the next Section 5.2).
In the study of Höller et al. (2014), patients with UWS showed increased long-distance functional connectivity between frontal and parietal/occipital regions not only in θ, but, surprisingly, also in β frequencies, similar to the findings of Stefan et al. (2018), who reported stronger global coherence in the β band. It is difficult to interpret the preserved functional connectivity in higher bands in these patients, which is commonly linked with some functional networks observed in the resting state (Brookes et al., 2011). However, it should be noted that the EEG signal of patients with DoC has unusual characteristics and may be contaminated by massive artifacts, especially in the higher frequency range.
5.2. Changes in Network Functional Connectivity in Patients With MCS
Similar to healthy individuals, patients with MCS reveal the presence of functional connectivity networks, especially fronto-posterior (Bai et al., 2021; Naro et al., 2021), at higher frequencies. However, the strength of these connections was on average weaker in comparison with healthy controls (Fingelkurts et al., 2012; Chennu et al., 2014, 2017; Schorr et al., 2016) and patients with the severe neurocognitive disorder (Leon-Carrion et al., 2012). These results were also convergent with those of Chennu et al. (2014), who reported stronger functional connections in α and weaker connections in δ in healthy controls. In turn, the strength of fronto-parietal α connections was correlated with the level of preserved consciousness (from MCS-, MCS+, eMCS, locked-in syndrome, to controls) (Chennu et al., 2017). Moreover, functional networks in the α band were more pronounced on the local and global level in conscious controls than in patients with DoC (Chennu et al., 2017). However, some studies report preserved functional connectivity not only in α, but also in two other bands (θ, β) as a neural marker of consciousness in MCS (Lehembre et al., 2012a; Höller et al., 2014; Sitt et al., 2014; Naro et al., 2018). The stronger connectivity in θ and α bands is interpreted as the most important characteristic of the functional networks of conscious individuals (Lehembre et al., 2012a; Höller et al., 2014; Sitt et al., 2014). Regarding functional connections in θ, two interpretations are possible: on the one hand, increased coherence in this band is observed in patients who have suffered structural brain injuries over the entire scalp (Schiff et al., 2014). This is in line with the observation of stronger functional connectivity measures in the θ band observed in patients with DoC in general, in comparison with controls (Chennu et al., 2014; van den Brink et al., 2018). On the other hand, increased connectivity in θ and α in MCS compared to UWS occurs over mesio-parietal areas and may be related to the key role of fronto-parietal networks in a “global workspace” in generating conscious states, and it may, therefore, be expected that their activity is disrupted in patients with UWS (Sitt et al., 2014). These results are in line with those obtained by Bai et al. (2021), who showed reduced functional connectivity in patients with DoC in comparison to HC, especially fronto-parietal connections in α band. Moreover, they described four key transient brain states preserved in patients with DoC: anterior, posterior, sensorimotor, and visual. The dynamics of switching between these states were different in patients with UWS and MCS: the formers spent more time in the anterior state, while the latter—in sensorimotor, visual, and posterior ones. Moreover, the transition between the sensory and posterior states was suppressed in patients with UWS as compared to MCS. Among those with MCS, two subgroups of patients are distinguished: Patients with MCS− showing volitional reactions such as object localization and visual pursuit, and patients with MCS+ reacting to commands. A detailed examination of brain connections in these two subgroups was conducted by Chennu et al. (2017), who observed similar α connectivity in patients with MCS− and MCS+, but, in the case of the latter group, they seemed to be situated over the frontal and parietal areas. Importantly, the strength of frontal, central, and parietal functional connections was related to brain metabolism as measured by positron emission tomography. Moreover, patients with MCS− connectivity in the δ band was much stronger than in MCS+, which suggests a lower degree of cortical deafferentation in patients showing high-level behavioral responses. Similarly, the stronger connectivity in α and weaker in δ band was observed in patients with MCS* in comparison to UWS. On the other hand, patients with MCS had stronger connectivity in θ band than MCS*, which is in line with the previous hypotheses on the role of particular EEG bands in mechanisms of consciousness. To summarize, the functional connectivity patterns of patients with MCS are characterized by preserved structure at higher frequencies. In comparison with healthy controls, however, their strength is reduced. This seems to be in line with the fMRI results that show that the strength of correlation in DMN is related to the level of consciousness (Vanhaudenhuyse et al., 2009).
6. Resting-state EEG Measures in Sleep and After Anesthetic Administration
The patterns of brain activity described in patients with DoC corresponds to the characteristics of brain activity in loss of consciousness in other contexts. The increase in low frequency bands, observed particularly in patients with UWS, has been reported in other cases of loss or alteration of consciousness: during deep anesthesia (Steriade et al., 1993; Purdon et al., 2013), temporal lobe seizure (Englot et al., 2010), and slow wave sleep (Timofeev et al., 2001), seen as functional deafferentation. In numerous studies, the increase of low frequency power was associated with anesthetic administration and loss of consciousness, e.g., during induction with sevoflurane and propofol in combination with remifentanil, there is a gradual increase in θ and δ bands in anterior areas, which spreads to posterior ones (Gugino et al., 2001). As isoflurane concentration increases, the power spectrum below 4 Hz strengthens (Hagihira, 2015). In turn, Purdon et al. (2013) and Banks et al. (2020) reported the propofol-induced increase of δ and α bands in anterior regions. Similarly, stronger δ-range activity was reported during a complex-partial seizure (Englot et al., 2010), accompanied by a decrease in cerebral blood flow in the bilateral frontal and parietal cortices, than during a temporal lobe seizure with preserved consciousness (Blumenfeld et al., 2004). The EEG signal of patients with UWS is characterized by lower values of complexity measures than in MCS and healthy controls. Similar levels of complexity and regularity of brain activity were observed in deep anesthesia (Liang et al., 2015; Shin et al., 2020) and during sleep (Burioka et al., 2005; Bruce et al., 2009). The comparison of 12 different entropy algorithms applied to EEG data registered in anesthesia induced by gamma-aminobutyric acid-ergic agents (isoflurane or sevoflurane anesthesia) showed in each case a similar effect of decreasing entropy measures as anesthesia deepened, which then increased during recovery (Liang et al., 2015). With higher doses of anesthetic drugs, the EEG signals becomes more regular and its frequency pattern simplifies. In turn, the analysis of regularity and spectral content of EEG signal during sleep showed lower values of the entropy measures in relation to slowing brain activity during the subsequent non-rapid eye movement (NREM) stages and gradual loosening of consciousness (Burioka et al., 2005), which was negatively correlated with the power of the δ band (Bruce et al., 2009).
The vast majority of studies indicate stronger δ functional connections in UWS than in MCS and the presence of higher frequency bands in patients with MCS, which are crucial for preserved consciousness. Such hypotheses are in line with the studies of Lee et al. (2019b), who showed emphasized phase-locking at low frequencies, particularly in posterior cortical areas, in conscious experiences during NREM sleep in comparison with the unconscious. These were interpreted as cortical bistability in thalamocortical circuits connected with loss of consciousness. Notably, the conscious experience was accompanied by higher phase-locked values in the α and β bands. The importance of δ band functional connectivity in loss of consciousness was indicated during propofol-induced sedation as well, demonstrating the lower level of responsiveness, stronger δ power, and local δ functional connectivity in parietal regions (Lee et al., 2017). On the other hand, a preserved pattern of α activity seems to be connected with consciousness (Lee et al., 2019b; Banks et al., 2020). Loss of consciousness during the N2 and N3 stages of NREM and under anesthesia was associated with α-band connectivity limited to anterior regions (Banks et al., 2020). In comparison, during wakefulness α-band functional connectivity was mainly observed within and between temporal and parietal regions. Interestingly, the authors obtained no differences in long-distance connections between conscious and unconscious conditions, such as were observed in patients with MCS in comparison with UWS.
7. Prognostic Value of Resting-State EEG Measures
Finally, some of the resting-state EEG measures have prognostic value for patients' long-term recovery. One of them is the power of α oscillations, which proved to be related to recovery during follow-up (Babiloni et al., 2009; Fingelkurts et al., 2011; O'Donnell et al., 2021). To identify other prognostic indicators of a favorable outcome for patients with DoC, an analysis of brain oscillatory microstates was conducted (Fingelkurts et al., 2011). Its results indicated that a bad outcome was related to an increased rigidity in brain activity, which was reflected by a lower diversity and variability of EEG signal, and a higher probability of occurrence of δ, as well as slow θ oscillations in non-survivors. Similarly, Stefan et al. (2018), Sarà and Pistoia (2010), and Sarà et al. (2011) showed that the complexity of the EEG signal allowed prediction of patients' outcomes; however, these results are not coherent, and the study of Gosseries et al. (2011) did not confirm this hypothesis. Regarding the functional networks, Schorr et al. (2016), Chennu et al. (2017), Bareham et al. (2018), and Bareham et al. (2020) demonstrated that the functional connectivity may also have a prognostic value. As shown by Bareham et al. (2018) in a longitudinal examination of four patients with DoC, the process of regaining consciousness was accompanied by the establishment of the functional connectivity in the α band and decreasing in the δ range. These results were partially confirmed 2 years later by Bareham et al. (2020) who indicated functional connectivity in the α band, the power of θ band, age of a patient, and arousal levels as the most important predictors of behavioral recovery. On the other hand, Chennu et al. (2017) observed a clear relationship between EEG-based δ connectivity and outcome—patients with negative outcomes had strong δ functional connections across large parts of the central and parietal areas. In turn, Schorr et al. (2016) indicated that fronto-parietal (in δ, θ, α and β) and parietal (in δ and θ) coherence could predict recovery from UWS, suggesting that both short and long-distance connections may have a prognostic value and are critical for the preservation of consciousness.
8. Summary and Conclusion
The extensive literature on the EEG-based resting state research in patients with DoC shows that the brain activity of patients with UWS is dominated by low δ oscillations, both in spectral power and the connectivity domain. The flow of information in higher bands is severely reduced and the variability of brain activity time course is much lower than in patients with MCS. Additionally, the brain functional networks of patients with UWS are characterized by a less complex structure. Patients with MCS are characterized by preserved functional connectivity patterns in higher frequencies—in comparison with patients with UWS, stronger activity in higher frequencies and weaker activity in the δ band are observed. It seems that patients with MCS have a more integral fronto-parietal network, and the overall pattern of connectivity, although weaker in higher frequencies, is more comparable to that of healthy controls. Moreover, the brain activity of patients with MCS is more complex and variable over time than that of patients with UWS.
The extensive literature on the EEG-based resting state research in patients with DoC shows quite coherent profiles of EEG functional connectivity in patients with UWS and MCS. However, some contrary observations are presented. This may be due to the different data analysis approaches used in this field, the use of quite small and highly heterogeneous experimental groups, and the low quality of recorded data. It seems that the greatest variability of the results obtained is related to functional network analysis. Generally, methods of functional connectivity can be classified into two groups—model-free approaches (e.g., coherence, phase lag index) or model-based approaches dependent on the autoregressive model (Granger family indices). They all rely on different internal parameters and are sensitive to various characteristics of measured signals, and none is optimal for all types of signals with different signal-to-noise ratios, or different types of artifacts (Wang et al., 2014). Some research groups have also implemented customized algorithms that are difficult to interpret and compare with more standardized methods. The implementation itself of each method also needs to be considered, because different mathematical or implementational nuances may yield different results.
The EEG signal registered in patients with DoC is very often contaminated by different artifacts to a much higher degree than is the case in healthy subjects. Despite efforts to clean these data manually and the use of automated toolboxes, the quality of the data still seems questionable. It appears that muscle activity in particular, which is very common and strong in these patients, can influence the results obtained and their discrepancies between particular studies in higher frequency ranges.
Moreover, a large number of measures related to functional brain networks have been reported to be important for consciousness mechanisms and require further examination, e.g., cross-frequency coupling (Naro et al., 2021), time-course characteristics of functional networks (Bai et al., 2021), or neural monitoring of visceral inputs (Candia-Rivera et al., 2021). Notably, the reviewed works are based on indicators (parameters) obtained from the EEG signal. However, new machine learning approaches are applied directly to the raw EEG signal (after filtering and artifacts rejection), showing successfully the most important features related to mechanisms of consciousness (Lee et al., 2022). Finally, another interesting avenue for further research is a combination of different techniques of neuroimaging for the examination of preserved connectivity in patients with DoC in a more complex manner, i.e., taking into account the presence of brain lesions or analysis of the patterns of functional networks obtained by fMRI.
Author Contributions
AD-B, MZ, and KJ-S reviewed the articles and wrote the manuscript. All authors contributed to the article and approved the submitted version.
Funding
This research was partially supported by the Polish National Science Centre (UMO-2018/31/B/ST7/01888) and a Grant from SWPS University of Social Sciences and Humanities SUB/IPsy/04/2021/04.
Conflict of Interest
The authors declare that the research was conducted in the absence of any commercial or financial relationships that could be construed as a potential conflict of interest.
Publisher's Note
All claims expressed in this article are solely those of the authors and do not necessarily represent those of their affiliated organizations, or those of the publisher, the editors and the reviewers. Any product that may be evaluated in this article, or claim that may be made by its manufacturer, is not guaranteed or endorsed by the publisher.
References
Babiloni, C., Sarà, M., Vecchio, F., Pistoia, F., Sebastiano, F., Onorati, P., et al. (2009). Cortical sources of resting-state alpha rhythms are abnormal in persistent vegetative state patients. Clin. Neurophysiol. 120, 719–729. doi: 10.1016/j.clinph.2009.02.157
Bai, Y., Lin, Y., and Ziemann, U. (2021). Managing disorders of consciousness: the role of electroencephalography. J. Neurol. 268, 4033–4065. doi: 10.1007/s00415-020-10095-z
Bai, Y., Xia, X., and Li, X. (2017). A review of resting-state electroencephalography analysis in disorders of consciousness. Front. Neurol. 8, 471. doi: 10.3389/fneur.2017.00471
Bandt, C., and Pompe, B. (2002). Permutation entropy: a natural complexity measure for time series. Phys. Rev. Lett. 88, 174102. doi: 10.1103/PhysRevLett.88.174102
Banks, M. I., Krause, B. M., Endemann, C. M., Campbell, D. I., Kovach, C. K., Dyken, M. E., et al. (2020). Cortical functional connectivity indexes arousal state during sleep and anesthesia. Neuroimage 211, 116627. doi: 10.1016/j.neuroimage.2020.116627
Bareham, C. A., Allanson, J., Roberts, N., Hutchinson, P. J., Pickard, J. D., Menon, D. K., et al. (2018). Longitudinal bedside assessments of brain networks in disorders of consciousness: case reports from the field. Front. Neurol. 9, 676. doi: 10.3389/fneur.2018.00676
Bareham, C. A., Roberts, N., Allanson, J., Hutchinson, P. J., Pickard, J. D., Menon, D. K., et al. (2020). Bedside eeg predicts longitudinal behavioural changes in disorders of consciousness. Neuroimage Clin. 28, 102372. doi: 10.1016/j.nicl.2020.102372
Biswal, B., Zerrin Yetkin, F., Haughton, V. M., and Hyde, J. S. (1995). Functional connectivity in the motor cortex of resting human brain using echo-planar mri. Magn. Reson. Med. 34, 537–541. doi: 10.1002/mrm.1910340409
Blumenfeld, H., McNally, K. A., Vanderhill, S. D., Paige, A. L., Chung, R., Davis, K., et al. (2004). Positive and negative network correlations in temporal lobe epilepsy. Cereb. Cortex 14, 892–902. doi: 10.1093/cercor/bhh048
Boly, M., Phillips, C., Tshibanda, L., Vanhaudenhuyse, A., Schabus, M., Dang-Vu, T. T., et al. (2008). Intrinsic brain activity in altered states of consciousness: how conscious is the default mode of brain function? Ann. N. Y. Acad. Sci. 1129, 119–129. doi: 10.1196/annals.1417.015
Boveroux, P., Vanhaudenhuyse, A., Bruno, M.-A., Noirhomme, Q., Lauwick, S., Luxen, A., et al. (2010). Breakdown of within-and between-network resting state functional magnetic resonance imaging connectivity during propofol-induced loss of consciousness. Anesthesiology 13, 1038–1053. doi: 10.1097/ALN.0b013e3181f697f5
Brenner, R. P. (2005). The interpretation of the eeg in stupor and coma. Neurologist 11, 271–284. doi: 10.1097/01.nrl.0000178756.44055.f6
Brookes, M. J., Woolrich, M., Luckhoo, H., Price, D., Hale, J. R., Stephenson, M. C., et al. (2011). Investigating the electrophysiological basis of resting state networks using magnetoencephalography. Proc. Natl. Acad. Sci. U. S. A. 108, 16783–16788. doi: 10.1073/pnas.1112685108
Bruce, E. N., Bruce, M. C., and Vennelaganti, S. (2009). Sample entropy tracks changes in eeg power spectrum with sleep state and aging. J. Clin. Neurophysiol. 26, 257. doi: 10.1097/WNP.0b013e3181b2f1e3
Bruno, M.-A., Vanhaudenhuyse, A., Thibaut, A., Moonen, G., and Laureys, S. (2011). From unresponsive wakefulness to minimally conscious plus and functional locked-in syndromes: recent advances in our understanding of disorders of consciousness. J. Neurol. 258, 1373–1384. doi: 10.1007/s00415-011-6114-x
Burioka, N., Miyata, M., Cornélissen, G., Halberg, F., Takeshima, T., Kaplan, D. T., et al. (2005). Approximate entropy in the electroencephalogram during wake and sleep. Clin. EEG Neurosci. 36, 21–24. doi: 10.1177/155005940503600106
Cai, L., Wang, J., Guo, Y., Lu, M., Dong, Y., and Wei, X. (2020). Altered inter-frequency dynamics of brain networks in disorder of consciousness. J. Neural Eng. 17, 036006. doi: 10.1088/1741-2552/ab8b2c
Candia-Rivera, D., Annen, J., Gosseries, O., Martial, C., Thibaut, A., Laureys, S., et al. (2021). Neural responses to heartbeats detect residual signs of consciousness during resting state in postcomatose patients. J. Neurosci. 41, 5251–5262. doi: 10.1523/JNEUROSCI.1740-20.2021
Cao, J., Zhao, Y., Shan, X., Wei, H.-,l., Guo, Y., Chen, L., et al. (2021). Brain functional and effective connectivity based on electroencephalography recordings: a review. Hum. Brain Mapp. 43, 860–879. doi: 10.1002/hbm.25683
Cavinato, M., Genna, C., Manganotti, P., Formaggio, E., Storti, S. F., Campostrini, S., et al. (2014). Coherence and consciousness: study of fronto-parietal gamma synchrony in patients with disorders of consciousness. Brain Topogr. 28, 570–579. doi: 10.1007/s10548-014-0383-5
Chennu, S., Annen, J., Wannez, S., Thibaut, A., Chatelle, C., Cassol, H., et al. (2017). Brain networks predict metabolism, diagnosis and prognosis at the bedside in disorders of consciousness. Brain 140, 2120–2132. doi: 10.1093/brain/awx163
Chennu, S., Finoia, P., Kamau, E., Allanson, J., Williams, G. B., Monti, M. M., et al. (2014). Spectral signatures of reorganised brain networks in disorders of consciousness. PLoS Comput. Biol. 10, e1003887. doi: 10.1371/journal.pcbi.1003887
Coleman, M., Menon, D., Fryer, T., and Pickard, J. (2005). Neurometabolic coupling in the vegetative and minimally conscious states: preliminary findings. J. Neurol. Neurosurg. Psychiatry 76, 432–434. doi: 10.1136/jnnp.2004.045930
Corchs, S., Chioma, G., Dondi, R., Gasparini, F., Manzoni, S., Markowska-Kacznar, U., et al. (2019). Computational methods for resting-state eeg of patients with disorders of consciousness. Front. Neurosci. 13, 1323. doi: 10.3389/fnins.2019.01323
Damoiseaux, J. S., Rombouts, S., Barkhof, F., Scheltens, P., Stam, C. J., Smith, S. M., et al. (2006). Consistent resting-state networks across healthy subjects. Proc. Natl. Acad. Sci. U.S.A. 103, 13848–13853. doi: 10.1073/pnas.0601417103
Demertzi, A., Antonopoulos, G., Heine, L., Voss, H. U., Crone, J. S., de Los Angeles, C., et al. (2015). Intrinsic functional connectivity differentiates minimally conscious from unresponsive patients. Brain 138, 2619–2631. doi: 10.1093/brain/awv169
Engemann, D. A., Raimondo, F., King, J.-R., Rohaut, B., Louppe, G., Faugeras, F., et al. (2018). Robust eeg-based cross-site and cross-protocol classification of states of consciousness. Brain 141, 3179–3192. doi: 10.1093/brain/awy251
Englot, D. J., Yang, L., Hamid, H., Danielson, N., Bai, X., Marfeo, A., et al. (2010). Impaired consciousness in temporal lobe seizures: role of cortical slow activity. Brain 133, 3764–3777. doi: 10.1093/brain/awq316
Ferenets, R., Lipping, T., Anier, A., Jantti, V., Melto, S., and Hovilehto, S. (2006). Comparison of entropy and complexity measures for the assessment of depth of sedation. IEEE Trans. Biomed. Eng. 53, 1067–1077. doi: 10.1109/TBME.2006.873543
Fingelkurts, A. A., Fingelkurts, A. A., Bagnato, S., Boccagni, C., and Galardi, G. (2011). Life or death: prognostic value of a resting eeg with regards to survival in patients in vegetative and minimally conscious states. PLoS ONE 6, e25967. doi: 10.1371/journal.pone.0025967
Fingelkurts, A. A., Fingelkurts, A. A., Bagnato, S., Boccagni, C., and Galardi, G. (2012). Dmn operational synchrony relates to self-consciousness: evidence from patients in vegetative and minimally conscious states. Open Neuroimag J. 6, 55. doi: 10.2174/1874440001206010055
Forgacs, P. B., Devinsky, O., and Schiff, N. D. (2020). Independent functional outcomes after prolonged coma following cardiac arrest: a mechanistic hypothesis. Ann. Neurol. 87, 618–632. doi: 10.1002/ana.25690
Forgacs, P. B., Frey, H.-P., Velazquez, A., Thompson, S., Brodie, D., Moitra, V., et al. (2017). Dynamic regimes of neocortical activity linked to corticothalamic integrity correlate with outcomes in acute anoxic brain injury after cardiac arrest. Ann. Clin. Transl. Neurol. 4, 119–129. doi: 10.1002/acn3.385
Fox, M. D., Snyder, A. Z., Vincent, J. L., Corbetta, M., Van Essen, D. C., and Raichle, M. E. (2005). The human brain is intrinsically organized into dynamic, anticorrelated functional networks. Proc. Natl. Acad. Sci. U.S.A. 102, 9673–9678. doi: 10.1073/pnas.0504136102
Friston, K. J. (2011). Functional and effective connectivity: a review. Brain Connect. 1, 13–36. doi: 10.1089/brain.2011.0008
Giacino, J. T., Ashwal, S., Childs, N., Cranford, R., Jennett, B., Katz, D. I., et al. (2002). The minimally conscious state definition and diagnostic criteria. Neurology 58, 349–353. doi: 10.1212/WNL.58.3.349
Giacino, J. T., Kalmar, K., and Whyte, J. (2004). The JFK coma recovery scale-revised: measurement characteristics and diagnostic utility. Arch. Phys. Med. Rehabil. 85, 2020–2029. doi: 10.1016/j.apmr.2004.02.033
Gloor, P., Ball, G., and Schaul, N. (1977). Brain lesions that produce delta waves in the eeg. Neurology 27, 326–326. doi: 10.1212/WNL.27.4.326
Gosseries, O., Schnakers, C., Ledoux, D., Vanhaudenhuyse, A., Bruno, M.-A., Demertzi, A., et al. (2011). Automated EEG entropy measurements in coma, vegetative state/unresponsive wakefulness syndrome and minimally conscious state. Funct. Neurol. 26, 25.
Gosseries, O., Zasler, N. D., and Laureys, S. (2014). Recent advances in disorders of consciousness: focus on the diagnosis. Brain Injury 28, 1141–1150. doi: 10.3109/02699052.2014.920522
Gugino, L., Chabot, R., Prichep, L., John, E., Formanek, V., and Aglio, L. (2001). Quantitative eeg changes associated with loss and return of consciousness in healthy adult volunteers anaesthetized with propofol or sevoflurane. Br. J. Anaesth. 87, 421–428. doi: 10.1093/bja/87.3.421
Hagihira, S. (2015). Changes in the electroencephalogram during anaesthesia and their physiological basis. Br. J. Anaesth. 115(suppl_1), i27–i31. doi: 10.1093/bja/aev212
Höller, Y., Thomschewski, A., Bergmann, J., Kronbichler, M., Crone, J. S., Schmid, E. V., et al. (2014). Connectivity biomarkers can differentiate patients with different levels of consciousness. Clin. Neurophysiol. 125, 1545–1555. doi: 10.1016/j.clinph.2013.12.095
King, J.-R., Sitt, J. D., Faugeras, F., Rohaut, B., El Karoui, I., Cohen, L., et al. (2013). Information sharing in the brain indexes consciousness in noncommunicative patients. Curr. Biol. 23, 1914–1919. doi: 10.1016/j.cub.2013.07.075
Lechinger, J., Bothe, K., Pichler, G., Michitsch, G., Donis, J., Klimesch, W., et al. (2013). Crs-r score in disorders of consciousness is strongly related to spectral eeg at rest. J. Neurol. 260, 2348–2356. doi: 10.1007/s00415-013-6982-3
Lee, H., Golkowski, D., Jordan, D., Berger, S., Ilg, R., Lee, J., et al. (2019a). Relationship of critical dynamics, functional connectivity, and states of consciousness in large-scale human brain networks. Neuroimage 188, 228–238. doi: 10.1016/j.neuroimage.2018.12.011
Lee, M., Baird, B., Gosseries, O., Nieminen, J. O., Boly, M., Postle, B. R., et al. (2019b). Connectivity differences between consciousness and unconsciousness in non-rapid eye movement sleep: a tms-eeg study. Sci. Rep. 9, 1–9. doi: 10.1038/s41598-019-41274-2
Lee, M., Sanders, R. D., Yeom, S.-K., Won, D.-O., Seo, K.-S., Kim, H. J., et al. (2017). Network properties in transitions of consciousness during propofol-induced sedation. Sci. Rep. 7, 1–13. doi: 10.1038/s41598-017-15082-5
Lee, M., Sanz, L. R., Barra, A., Wolff, A., Nieminen, J. O., Boly, M., et al. (2022). Quantifying arousal and awareness in altered states of consciousness using interpretable deep learning. Nat. Commun. 13, 1–14. doi: 10.1038/s41467-022-28451-0
Lehembre, R., Bruno, M.-A., Vanhaudenhuyse, A., Chatelle, C., Cologan, V., Leclercq, Y., et al. (2012a). Resting-state eeg study of comatose patients: a connectivity and frequency analysis to find differences between vegetative and minimally conscious states. Funct. Neurol. 27, 41.
Lehembre, R., Gosseries, O., Lugo, Z., Jedidi, Z., Chatelle, C., Sadzot, B., et al. (2012b). Electrophysiological investigations of brain function in coma, vegetative and minimally conscious patients. Arch. Ital. Biol. 150, 122–139. doi: 10.4449/aib.v150i2.1374
Lei, L., Liu, K., Yang, Y., Doubliez, A., Hu, X., Xu, Y., et al. (2022). Spatio-temporal analysis of eeg features during consciousness recovery in patients with disorders of consciousness. Clin. Neurophysiol. 133, 135–144. doi: 10.1016/j.clinph.2021.08.027
Leon-Carrion, J., Leon-Dominguez, U., Pollonini, L., Wu, M.-H., Frye, R. E., Dominguez-Morales, M. R., et al. (2012). Synchronization between the anterior and posterior cortex determines consciousness level in patients with traumatic brain injury (tbi). Brain Res. 1476, 22–30. doi: 10.1016/j.brainres.2012.03.055
Leon-Carrion, J., Martin-Rodriguez, J., Damas-Lopez, J., y Martin, J. B., and Dominguez-Morales, M. (2008). Brain function in the minimally conscious state: a quantitative neurophysiological study. Clin. Neurophysiol. 119, 1506–1514. doi: 10.1016/j.clinph.2008.03.030
Liang, Z., Wang, Y., Sun, X., Li, D., Voss, L. J., Sleigh, J. W., et al. (2015). Eeg entropy measures in anesthesia. Front. Comput. Neurosci. 9, 16. doi: 10.3389/fncom.2015.00016
Lutkenhoff, E. S., Nigri, A., Sebastiano, D. R., Sattin, D., Visani, E., Rosazza, C., et al. (2019). Eeg power spectra and subcortical pathology in chronic disorders of consciousness. bioRxiv 695288. doi: 10.1101/695288
Marinazzo, D., Gosseries, O., Boly, M., Ledoux, D., Rosanova, M., Massimini, M., et al. (2014). Directed information transfer in scalp electroencephalographic recordings: insights on disorders of consciousness. Clin. EEG Neurosci. 45, 33–39. doi: 10.1177/1550059413510703
Mason, M. F., Norton, M. I., Van Horn, J. D., Wegner, D. M., Grafton, S. T., and Macrae, C. N. (2007). Wandering minds: the default network and stimulus-independent thought. Science 315, 393–395. doi: 10.1126/science.1131295
Naro, A., Bramanti, A., Leo, A., Cacciola, A., Manuli, A., Bramanti, P., et al. (2018). Shedding new light on disorders of consciousness diagnosis: the dynamic functional connectivity. Cortex 103, 316–328. doi: 10.1016/j.cortex.2018.03.029
Naro, A., Bramanti, P., Leo, A., Cacciola, A., Bramanti, A., Manuli, A., et al. (2016). Towards a method to differentiate chronic disorder of consciousness patients' awareness: The low-resolution brain electromagnetic tomography analysis. J. Neurol. Sci. 368, 178–183. doi: 10.1016/j.jns.2016.07.016
Naro, A., Maggio, M. G., Leo, A., and Calabro, R. S. (2021). Multiplex and multilayer network eeg analyses: a novel strategy in the differential diagnosis of patients with chronic disorders of consciousness. Int. J. Neural Syst. 31, 2050052. doi: 10.1142/S0129065720500525
O'Donnell, A., Pauli, R., Banellis, L., Sokoliuk, R., Hayton, T., Sturman, S., et al. (2021). The prognostic value of resting-state eeg in acute post-traumatic unresponsive states. Brain Commun. 3, fcab017. doi: 10.1093/braincomms/fcab017
Piarulli, A., Bergamasco, M., Thibaut, A., Cologan, V., Gosseries, O., and Laureys, S. (2016). Eeg ultradian rhythmicity differences in disorders of consciousness during wakefulness. J. Neurol. 263, 1746–1760. doi: 10.1007/s00415-016-8196-y
Pollonini, L., Pophale, S., Situ, N., Wu, M.-H., Frye, R. E., Leon-Carrion, J., et al. (2010). Information communication networks in severe traumatic brain injury. Brain Topogr. 23, 221–226. doi: 10.1007/s10548-010-0139-9
Purdon, P. L., Pierce, E. T., Mukamel, E. A., Prerau, M. J., Walsh, J. L., Wong, K. F. K., et al. (2013). Electroencephalogram signatures of loss and recovery of consciousness from propofol. Proc. Natl. Acad. Sci. U.S.A. 110, E1142–E1151. doi: 10.1073/pnas.1221180110
Raichle, M. E. (2015). The brain's default mode network. Annu. Rev. Neurosci. 38, 433–447. doi: 10.1146/annurev-neuro-071013-014030
Raichle, M. E., MacLeod, A. M., Snyder, A. Z., Powers, W. J., Gusnard, D. A., and Shulman, G. L. (2001). A default mode of brain function. Proc. Natl. Acad. Sci. U.S.A. 98, 676–682. doi: 10.1073/pnas.98.2.676
Rizkallah, J., Annen, J., Modolo, J., Gosseries, O., Benquet, P., Mortaheb, S., et al. (2019). Decreased integration of EEG source-space networks in disorders of consciousness. NeuroImage 23, 101841. doi: 10.1016/j.nicl.2019.101841
Sarà, M., and Pistoia, F. (2010). Complexity loss in physiological time series of patients in a vegetative state. Nonlinear Dyn. Psychol. Life Sci. 14, 1.
Sarà, M., Pistoia, F., Pasqualetti, P., Sebastiano, F., Onorati, P., and Rossini, P. M. (2011). Functional isolation within the cerebral cortex in the vegetative state: a nonlinear method to predict clinical outcomes. Neurorehabil. Neural Repair. 25, 35–42. doi: 10.1177/1545968310378508
Schiff, N. D. (2016). “Mesocircuit mechanisms underlying recovery of consciousness following severe brain injuries: Model and predictions,” in Brain Function and Responsiveness in Disorders of Consciousness, eds M. Monti, and W. Sannita (Cham: Springer). doi: 10.1007/978-3-319-21425-2_15
Schiff, N. D., Nauvel, T., and Victor, J. D. (2014). Large-scale brain dynamics in disorders of consciousness. Curr. Opin. Neurobiol. 25, 7–14. doi: 10.1016/j.conb.2013.10.007
Schnakers, C., Ledoux, D., Majerus, S., Damas, P., Damas, F., Lambermont, B., et al. (2008). Diagnostic and prognostic use of bispectral index in coma, vegetative state and related disorders. Brain Injury 22, 926–931. doi: 10.1080/02699050802530565
Schorr, B., Schlee, W., Arndt, M., and Bender, A. (2016). Coherence in resting-state eeg as a predictor for the recovery from unresponsive wakefulness syndrome. J. Neurol. 263, 937–953. doi: 10.1007/s00415-016-8084-5
Sebastiano, D. R., Varotto, G., Sattin, D., and Franceschetti, S. (2021). Eeg assessment in patients with disorders of consciousness: Aims, advantages, limits, and pitfalls. Front. Neurol. 12, 649849. doi: 10.3389/fneur.2021.649849
Shen, H. H. (2015). Core concept: resting-state connectivity. Proc. Natl. Acad. Sci. U.S.A. 112, 14115–14116. doi: 10.1073/pnas.1518785112
Shin, H. W., Kim, H. J., Jang, Y. K., You, H. S., Huh, H., Choi, Y. J., et al. (2020). Monitoring of anesthetic depth and eeg band power using phase lag entropy during propofol anesthesia. BMC Anesthesiol. 20, 1–10. doi: 10.1186/s12871-020-00964-5
Sitt, J. D., King, J.-R., El Karoui, I., Rohaut, B., Faugeras, F., Gramfort, A., et al. (2014). Large scale screening of neural signatures of consciousness in patients in a vegetative or minimally conscious state. Brain 137, 2258–2270. doi: 10.1093/brain/awu141
Stefan, S., Schorr, B., Lopez-Rolon, A., Kolassa, I.-T., Shock, J. P., Rosenfelder, M., et al. (2018). Consciousness indexing and outcome prediction with resting-state eeg in severe disorders of consciousness. Brain Topogr. 31, 848–862. doi: 10.1007/s10548-018-0643-x
Steriade, M., Nunez, A., and Amzica, F. (1993). A novel slow (<1 hz) oscillation of neocortical neurons in vivo: depolarizing and hyperpolarizing components. J. Neurosci. 13, 3252–3265. doi: 10.1523/JNEUROSCI.13-08-03252.1993
Thibaut, A., Panda, R., Annen, J., Sanz, L. R., Naccache, L., Martial, C., et al. (2021). Preservation of brain activity in unresponsive patients identifies mcs star. Ann. Neurol. 90, 89–100. doi: 10.1002/ana.26095
Timofeev, I., Grenier, F., and Steriade, M. (2001). Disfacilitation and active inhibition in the neocortex during the natural sleep-wake cycle: an intracellular study. Proc. Natl. Acad. Sci. U.S.A. 98, 1924–1929. doi: 10.1073/pnas.98.4.1924
van den Brink, R., Nieuwenhuis, S., van Boxtel, G., van Luijtelaar, G., Eilander, H., and Wijnen, V. (2018). Task-free spectral eeg dynamics track and predict patient recovery from severe acquired brain injury. Neuroimage Clin. 17, 43–52. doi: 10.1016/j.nicl.2017.10.003
van Putten, M. J., and Hofmeijer, J. (2016). Eeg monitoring in cerebral ischemia: basic concepts and clinical applications. J. Clin. Neurophysiol. 33, 203–210 doi: 10.1097/WNP.0000000000000272
Vanhaudenhuyse, A., Noirhomme, Q., Tshibanda, L. J.-F., Bruno, M.-A., Boveroux, P., Schnakers, C., et al. (2009). Default network connectivity reflects the level of consciousness in non-communicative brain-damaged patients. Brain 133, 161–171. doi: 10.1093/brain/awp313
Varotto, G., Fazio, P., Sebastiano, D. R., Duran, D., D'Incerti, L., Parati, E., et al. (2014). Altered resting state effective connectivity in long-standing vegetative state patients: an eeg study. Clin. Neurophysiol. 125, 63–68. doi: 10.1016/j.clinph.2013.06.016
Wang, H. E., Bénar, C. G., Quilichini, P. P., Friston, K. J., Jirsa, V. K., and Bernard, C. (2014). A systematic framework for functional connectivity measures. Front. Neurosci. 8, 405. doi: 10.3389/fnins.2014.00405
Whitman, J. C., Ward, L. M., and Woodward, T. (2013). Patterns of cortical oscillations organize neural activity into whole-brain functional networks evident in the fmri bold signal. Front. Hum. Neurosci. 7, 80. doi: 10.3389/fnhum.2013.00080
Wu, D., Cai, G., Yuan, Y., Liu, L., Li, G.-Q., Song, W.-Q., et al. (2011a). Application of nonlinear dynamics analysis in assessing unconsciousness: a preliminary study. Clin. Neurophysiol. 122, 490–498. doi: 10.1016/j.clinph.2010.05.036
Wu, D., Cai, G., Zorowitz, R. D., Yuan, Y., Wang, J., and Song, W.-Q. (2011b). Measuring interconnection of the residual cortical functional islands in persistent vegetative state and minimal conscious state with eeg nonlinear analysis. Clin. Neurophysiol. 122, 1956–1966. doi: 10.1016/j.clinph.2011.03.018
Keywords: electroencephalography, EEG connectivity, resting state EEG, disorders of consciousness, vegetative state, unresponsive wakefulness syndrome, minimally consciousness state
Citation: Duszyk-Bogorodzka A, Zieleniewska M and Jankowiak-Siuda K (2022) Brain Activity Characteristics of Patients With Disorders of Consciousness in the EEG Resting State Paradigm: A Review. Front. Syst. Neurosci. 16:654541. doi: 10.3389/fnsys.2022.654541
Received: 16 January 2021; Accepted: 25 April 2022;
Published: 27 May 2022.
Edited by:
Olivia Gosseries, University of Liège, BelgiumReviewed by:
Andrea Piarulli, Università degli Studi di Pisa, ItalyRomulo Fuentes Flores, University of Chile, Chile
Copyright © 2022 Duszyk-Bogorodzka, Zieleniewska and Jankowiak-Siuda. This is an open-access article distributed under the terms of the Creative Commons Attribution License (CC BY). The use, distribution or reproduction in other forums is permitted, provided the original author(s) and the copyright owner(s) are credited and that the original publication in this journal is cited, in accordance with accepted academic practice. No use, distribution or reproduction is permitted which does not comply with these terms.
*Correspondence: Anna Duszyk-Bogorodzka, aduszyk1@swps.edu.pl