- 1School of Electrical and Electronic Engineering, The University of Adelaide, Adelaide, SA, Australia
- 2Department of Electrical and Electronic Engineering, United International University, Dhaka, Bangladesh
- 3Advanced Intelligent Multidisciplinary Systems (AIMS) Lab, Department of Computer Science and Engineering, United International University, Dhaka, Bangladesh
- 4Adelaide Institute for Sleep Health, College of Medicine and Public Health, Flinders University, Adelaide, SA, Australia
- 5Healthcare Engineering Innovation Center, Department of Biomedical Engineering, Khalifa University of Science and Technology, Abu Dhabi, United Arab Emirates
Brain computer interfaces (BCI) provide a direct communication link between the brain and a computer or other external devices. They offer an extended degree of freedom either by strengthening or by substituting human peripheral working capacity and have potential applications in various fields such as rehabilitation, affective computing, robotics, gaming, and neuroscience. Significant research efforts on a global scale have delivered common platforms for technology standardization and help tackle highly complex and non-linear brain dynamics and related feature extraction and classification challenges. Time-variant psycho-neurophysiological fluctuations and their impact on brain signals impose another challenge for BCI researchers to transform the technology from laboratory experiments to plug-and-play daily life. This review summarizes state-of-the-art progress in the BCI field over the last decades and highlights critical challenges.
1. Introduction
The brain computer interface (BCI) is a direct and sometimes bidirectional communication tie-up between the brain and a computer or an external device, which involves no muscular stimulation. It has shown promise for rehabilitating subjects with motor impairments as well as for augmenting human working capacity either physically or cognitively (Lebedev and Nicolelis, 2017; Saha and Baumert, 2020). BCI was historically envisioned as a potential technology for augmenting/replacing existing neural rehabilitations or serving assistive devices controlled directly by the brain (Vidal, 1973; Birbaumer et al., 1999; Alcaide-Aguirre et al., 2017; Shahriari et al., 2019). The first systematic attempt to implement an electroencephalogram (EEG)-based BCI was made by J. J. Vidal in 1973, who recorded the evoked electrical activity of the cerebral cortex from the intact skull using EEG (Vidal, 1973), a non-invasive technique first studied in humans invented by Berger (1929). Another early endeavor to establish direct communication between a computer and the brain of people with severe motor impairments had utilized P300, an event related brain potential (Farwell and Donchin, 1988). As an alternative to conventional therapeutic rehabilitation for motor impairments, BCI technology helps to artificially augment or re-excite synaptic plasticity in affected neural circuits. By exploiting undamaged cognitive and emotional functions, BCI aims at re-establishing the link between the brain and an impaired peripheral site (Vansteensel et al., 2016). However, the research applications of BCI technology evolved significantly over the years, including brain fingerprinting for lie detection (Farwell et al., 2014), detecting drowsiness for improving human working performances (Aricò et al., 2016; Wei et al., 2018), estimating reaction time (Wu et al., 2017b), controlling virtual reality (Vourvopoulos et al., 2019), quadcopters (LaFleur et al., 2013) and video games (Singh et al., 2020), and driving humanoid robots (Choi and Jo, 2013; Spataro et al., 2017). Figure 1 demonstrates the progression of BCI in various application fields since its conception.
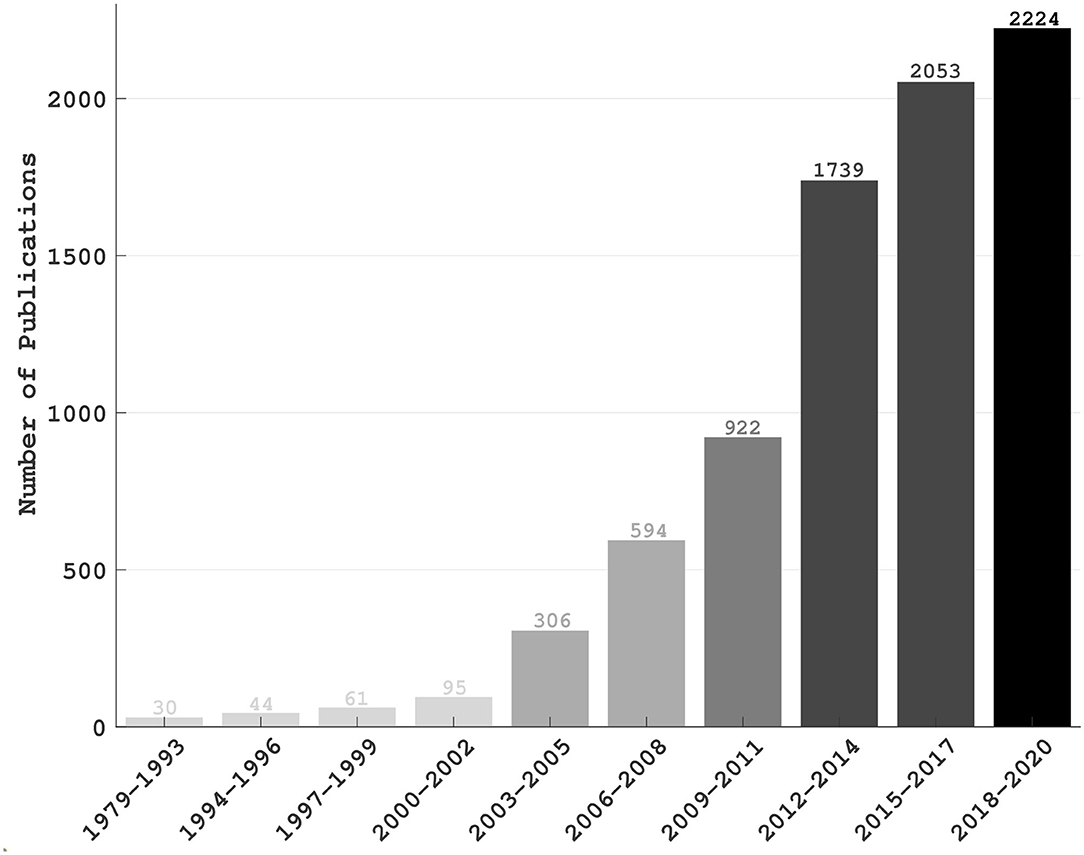
Figure 1. The number of publications over the years: The statistics was based on a search on PubMed in which “brain computer interface” was the search keyword. The publications those were listed until 4th December 2020 have been accounted only. A significant increase in the number of publications in this decade as compared to the last decade implicates the engagement of a greater community in this field and, thus the importance of BCI technology.
According to the Brain/Neural Computer Interaction Horizon 2020 project, an initiative by the European Commission for coordinating BCI research, six major application themes, i.e., restore (e.g., unlocking the completely locked-in), replace (e.g., BCI-controlled neuroprosthesis), enhance (e.g., enhanced user experience in computer games), supplement (e.g., augmented reality glasses), improve (e.g., upper limb rehabilitation after stroke), and research tool (e.g., decoding brain activity with real-time feedback) have been outlined as feasible and promising fields (Brunner et al., 2015). This overview encompasses a wide range of challenges and trends in BCI field. For specialized reviews on particular BCI topics we refer to the recent literature (McFarland et al., 2006; Schwartz et al., 2006; Bashashati et al., 2007; Lotte et al., 2007, 2018; Matthews et al., 2007; Sitaram et al., 2007; Mak and Wolpaw, 2009; Menon et al., 2009; Nicolelis and Lebedev, 2009; Summerer et al., 2009; Vaadia and Birbaumer, 2009; Milan and Carmena, 2010; Min et al., 2010; Clausen, 2011; Krusienski et al., 2011; Liao et al., 2012; Nicolas-Alonso and Gomez-Gil, 2012; Shih et al., 2012; Jebari, 2013; McCullagh et al., 2014; Ahn and Jun, 2015; Jayaram et al., 2016; Lebedev and Nicolelis, 2017; Mudgal et al., 2020; Rashid et al., 2020; Saha and Baumert, 2020).
1.1. Characterization of BCI Systems
BCI systems can be categorized by the way they use the brain: Passive BCI decode unintentional affective/cognitive states of the brain (Zander et al., 2009), while active BCI directly involve the user's voluntary intention-induced brain activity. Reactive BCI use brain waves generated as response to external stimuli. Detecting driver's drowsiness to prevent road accidents is an example of passive BCI (Lin et al., 2008; Gao et al., 2019). BCI systems driven by users' intentional motor imagery (MI) (Marchesotti et al., 2016; Saha et al., 2019a; Saha and Baumert, 2020) and visually evoked P300 produced by external stimulation (Farwell et al., 2014) can be considered active BCI and reactive BCI, respectively.
The modality of signal acquisition has been used to divide systems into invasive and non-invasive BCI (Min et al., 2010; Rosenfeld and Wong, 2017). Non-invasive BCI exploiting EEG are most common, although more recently, functional near infrared spectroscopy (fNIRS) (Matthews et al., 2007), magnetoencephalography (MEG) (Fukuma et al., 2016), functional magnetic resonance imaging (fMRI) (Kaas et al., 2019) and functional transcranial Doppler ultrasonography (Faress and Chau, 2013; Lu et al., 2015; Khalaf et al., 2019) have been exploited. In contrast, invasive intracortical electrodes (Pandarinath et al., 2017) and electrocorticography (ECoG) (Kaiju et al., 2017) have been used, providing a superior signal-to-noise ratio and better localization of brain activity. Table 1 summarizes signal acquisition modalities and their suitability for BCI applications.
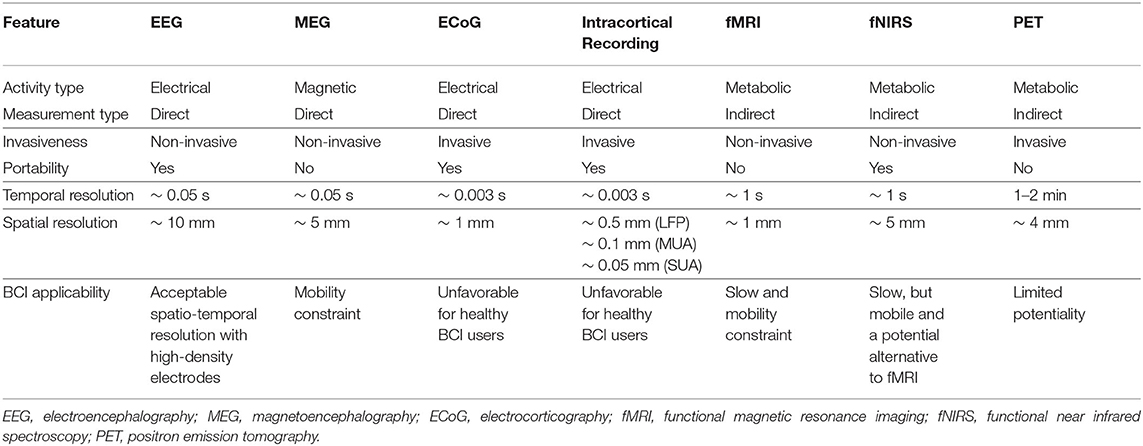
Table 1. A list of neuroimaging techniques and their suitability in brain computer interface (BCI) applications.
Recent technological advancements allow both the decoding of neural activities and the delivery of external signals into targeted brain areas to induce plasticity, i.e., remodeling of neurosynaptic organization (Lajoie et al., 2017). Plasticity is an inherent characteristic of the brain and peripheral nervous system underpinning BCI-based rehabilitation and other neuroscientific applications. While most of the BCI systems translate brain signals to computer commands, some systems utilize external stimulation modalities such as transcranial magnetic stimulation (Grau et al., 2014; Rao et al., 2014; Schaworonkow et al., 2019) and transcranial direct current stimulation (Baxter et al., 2017) to stimulate specific brain areas. The bidirectional framework of BCI comprises either one brain with feedback modality or two brains. Transcranial direct current stimulation directed by MI-related EEG signals alters the connectivity in sensorimotor networks of healthy individuals (Baxter et al., 2017). Another possible application of bidirectional BCI framework is direct brain-to-brain communication (Grau et al., 2014; Rao et al., 2014). Moreover, some BCI applications require auxiliary modalities, e.g., proprioceptive feedback and functional electrical stimulation driven by brain signals as feedback for augmenting or regaining peripheral motor actions (Darvishi et al., 2017; Bhattacharyya et al., 2019; Bockbrader et al., 2019; Murovec et al., 2020).
1.2. Factors Influencing BCI Performance
For medical applications of BCI, three criteria are essential: (1) a comfortable and convenient signal acquisition device, (2) system validation and dissemination, and (3) reliability and potentiality of BCI (Shih et al., 2012). For the restoration of mobility in patients with motor impairments, invasive intracortical recordings show better BCI performance (Hochberg, 2013) than non-invasive methods such as EEG (Milan and Carmena, 2010). The performance determines how efficiently a patient can perform an impaired motor task or communicate with an external device. Invasive modalities are also suitable for locked-in patients, because the benefits (significantly improved quality-of-life) outweigh the risks associated with implantation (Gilja et al., 2011). A pilot study found no adverse effects pertaining to surgery or tissue reaction at 1 year follow-up (Friehs et al., 2006). Invasive BCI should generally not be considered for neurologically intact people due to risks associated with surgery. However, invasive recordings may enable the utilization of localized inner cortex activities and a better interpretation of surface recordings from a non-invasive modality (Schalk, 2010; Lina et al., 2012).
Many factors influence BCI performance; taking the underlying cortical-subcortical networks into consideration is of crucial importance. For example, MI-induced signals are best recorded from premotor and motor areas, because premotor cortex, primary motor cortex and supplementary motor area along with basal ganglia and thalamus of the subcortical areas are the mostly activated areas during MI (Marchesotti et al., 2017). While EEG can capture premotor and motor area activation (Edelman et al., 2015; Saha et al., 2019a), intracortical electrodes can record signals from basal ganglia and thalamus (Sand et al., 2017).
Several issues can significantly impede BCI performance. Maintaining an acceptable signal-to-noise ratio in non-invasive long-term recordings is critical. Event-induced brain waves or oscillations are dynamic and affected by unstable resting state networks (RSNs) (Mantini et al., 2007). Time-variant psychophysiological (Gonçalves et al., 2006; Zhang et al., 2015; Acqualagna et al., 2016; Saha and Baumert, 2020), neuroanatomical (Kasahara et al., 2015) factors and users' fundamental traits (Ahn and Jun, 2015) cause unreliable estimates of RSNs, causing short and long-term signal variation within and across individuals (Saha and Baumert, 2020). Due to these intrinsic signal variations, BCI systems require subject-specific training, during which subjects attend a calibration session that is tedious and often frustrating. To eliminate subject-specific training, the concept of inter-subject associativity, demonstrated in previous works in case of natural vision (Hasson et al., 2004) and natural music listening (Abrams et al., 2013), could be exploited toward inter-subject operable BCI. Recent studies suggest that inter-subject operable sensorimotor rhythm-based BCI might become feasible for subjects who share common brain dynamics (Saha et al., 2017, 2018, 2019a; Saha and Baumert, 2020). Inter-subject BCI holds promise predominantly for healthy people and in applications such as gaming, drowsiness and lie detection, because rehabilitative BCI must consider the characteristics and severity of individual impairment (Park et al., 2016). Transfer learning can also reduce the effects of session-to-session and subject-to-subject variabilities, by using systems that were trained on data from different people exploiting commonalties and reducing training requirements (Jayaram et al., 2016; Saha et al., 2017, 2018, 2019a; He and Wu, 2019; Wu et al., 2020).
2. Challenges
2.1. Psychophysiological and Neurological Challenges
Emotional and mental processes, neurophysiology associated with cognition and neurological factors, i.e., functions, anatomy, play crucial roles in BCI performance and give rise to significant intra- and inter-individual variability (Saha and Baumert, 2020). Psychological factors such as attention, memory load, fatigue and competing cognitive processes (Gonçalves et al., 2006; Käthner et al., 2014; Calhoun and Adali, 2016) as well as users' basic characteristics such as lifestyle, gender, and age, (Kasahara et al., 2015) influence instantaneous brain dynamics. For example, individuals with lower empathy participate less emotionally in a P300-BCI paradigm and can produce higher amplitudes of P300 waves than subjects with greater empathetic involvement (Kleih and Kübler, 2013). Motivation is also related to P300-BCI performance (Nijboer et al., 2010).
Besides psychological traits, resting state physiological parameters, for example, frequency domain features of resting state heart rate variability are associated with BCI performance (Kaufmann et al., 2012). In addition, the baselines of RSNs are dynamic and modify any cortical signature instantaneously (Mantini et al., 2007). Age alters RSNs and associated cognitive responses (Wang et al., 2016b). Adapting to such time-variant RSNs is more demanding when the effects of RSNs mask event-related cortical responses (Jensen et al., 2011). Moreover, the inherent complexity and diversity in the formation of human brains (Sporns, 2013) that influence the functional neural networks (Honey et al., 2010), construct highly volatile neuronal connectivity over time and across subjects (Honey et al., 2009). An efficient BCI system must be robust to such inherent physiological fluctuations over time to enable more generalized systems (Saha and Baumert, 2020).
Experiments correlating BCI performance with neuroanatomical, neurophysiological and psychological parameters have provided fascinating results: gray matter volume in sensorimotor cortical areas is associated with BCI success (Kasahara et al., 2015). Sensorimotor rhythm-based BCI has implicated that physiological predictors such as spectral entropy and power spectral density, derived from resting state EEG recordings are correlated with BCI performance (Zhang et al., 2015; Acqualagna et al., 2016). Psychological predictors such as attention and motivation, are also associated with sensorimotor rhythm-based BCI performance (Hammer et al., 2012). Corticospinal excitability could be used as another reliable marker for BCI performance (Vasilyev et al., 2017). Taking head anatomy into consideration augments BCI performance (Wronkiewicz et al., 2015; Saha et al., 2019a).
Around 15–30% of individuals are inherently not able to produce brain signals robust enough to operate a BCI (Blankertz et al., 2009; Halder et al., 2019; Cecotti, 2020). Considering neurophysiological phenomena may reduce BCI illiteracy. An adaptive machine learning approach incorporating neurophysiological and psychological traits has been proposed to reduce BCI illiteracy (Vidaurre and Blankertz, 2010). The causes of BCI illiteracy do not exclusively rely on users' ability to produce signals. Sometimes technological limitations may hinder essential features extraction for a successful BCI operation for an individual. For example, measurements of scalp EEG/MEG may not show good task-specific signals due to the folding of the cortex or scalp-to-cortex distance for that individual (Andersen et al., 2020).
Other case-specific investigations on neuro-psycho-physiological parameters contributing to BCI performance are essential. For the rehabilitation of stroke survivors, affected neural circuits, i.e., lesions are to be identified carefully, because brain responses fluctuate according to the spatial location of the stroke lesion (Park et al., 2016). Although current neuroimaging methods are effective in capturing stroke lesion sites, a case-specific BCI design that incorporates residual brain function is required for rehabilitative interventions. Highly individualized design impedes wide dissemination of BCI-driven rehabilitation of neurological conditions.
2.2. Technological Challenges
Event related potential (ERP) (McCane et al., 2015), steady-state visual evoked potential (SSVEP) (Chen et al., 2015; Abu-Alqumsan and Peer, 2016), auditory evoked potential (AEP) (Schreuder et al., 2010), steady-state somatosensory evoked potential (SSSEP) (Muller-Putz et al., 2006; Oxley et al., 2017), and motor imagery (MI) (Marchesotti et al., 2016; Saha et al., 2019a; Saha and Baumert, 2020), have been proposed to detect cognitive signatures although none of the approaches performs well for all BCI applications. For example, ERPs and SSVEPs are target-specific and elicited by external stimuli; however, if ERPs depend on visual stimuli, they cannot be used for communication by locked-in patients with impaired visual processing. In that case, auditory-based ERP (e.g., AEP) could be used if auditory processing remains intact. The SSVEP method provides the highest information transfer rate of a non-invasive EEG-based BCI (Chen et al., 2015; Abu-Alqumsan and Peer, 2016). Limitations of the SSVEP technique include visual fatigue caused by looking at a flickering display for a long time. When using this technique, the control signal could be arbitrary and counter-intuitive, although it might depend mostly on the experimental context. For example, when using a BCI speller based on SSVEP, an individual looks at the letter “A”, which flickers at 10 Hz. It is generally not given importance to any inherent relationship between “A” and 10 Hz, instead the control signal is arbitrary mapped to interface with a computer. An advantage of an MI-based BCI is the use of explicit mapping of task-related brain signals to operate (Saha and Baumert, 2020). However, MI seems too slow for action control, thus they are not suitable for controlling virtual reality environments or videogames (Lécuyer et al., 2008). Recently proposed hybrid BCIs which utilize more than one signature, i.e., SSVEP/ERP (Combaz and Van Hulle, 2015; Yin et al., 2015) and SSVEP/MI (Pfurtscheller et al., 2010; Horki et al., 2011), seem to offer more robust features. Considering asynchronous BCI where the user decides to activate a command when necessary, the performance is still unsatisfactory (Han et al., 2020).
The intrinsic neurophysiological instability of brain dynamics poses critical challenges for making BCI systems efficient. The major components of a BCI system are signal acquisition, signal processing and effector device (Schwartz et al., 2006). Various neuroimaging techniques have been used to explore cortical activities through either electrical or hemodynamic signatures (Min et al., 2010), but none of the methods shows any advantage for a lucrative BCI design meeting the four important criteria: cost efficiency, portability, easy maintenance, and little or no involvement of surgery. EEG-based BCI are relatively more compliant with the abovementioned criteria as compared to other signal acquisition modalities. Tables 2, 3 list a diverse range of BCI applications exploiting EEG. Both invasive and non-invasive signal acquisitions have recently shown that reliable long-term (i.e., for at least several months) use of BCI systems is quite feasible (Saeedi et al., 2016; Sauter-Starce et al., 2019; Shahriari et al., 2019; Oxley et al., 2020).
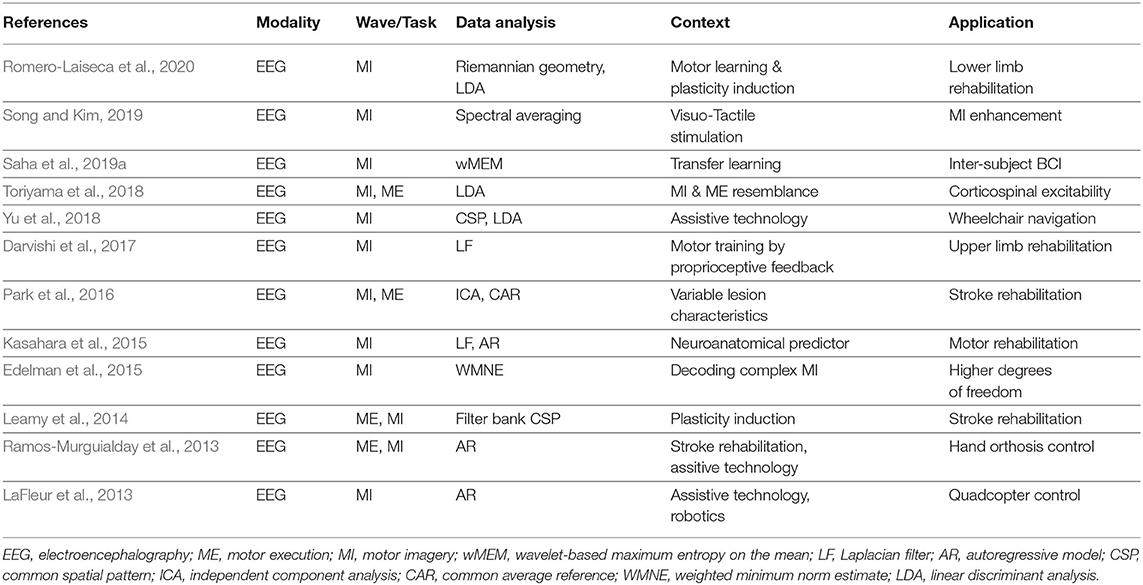
Table 2. A summary of sensorimotor rhythms and electroencephalography-based brain computer interface (BCI) studies.
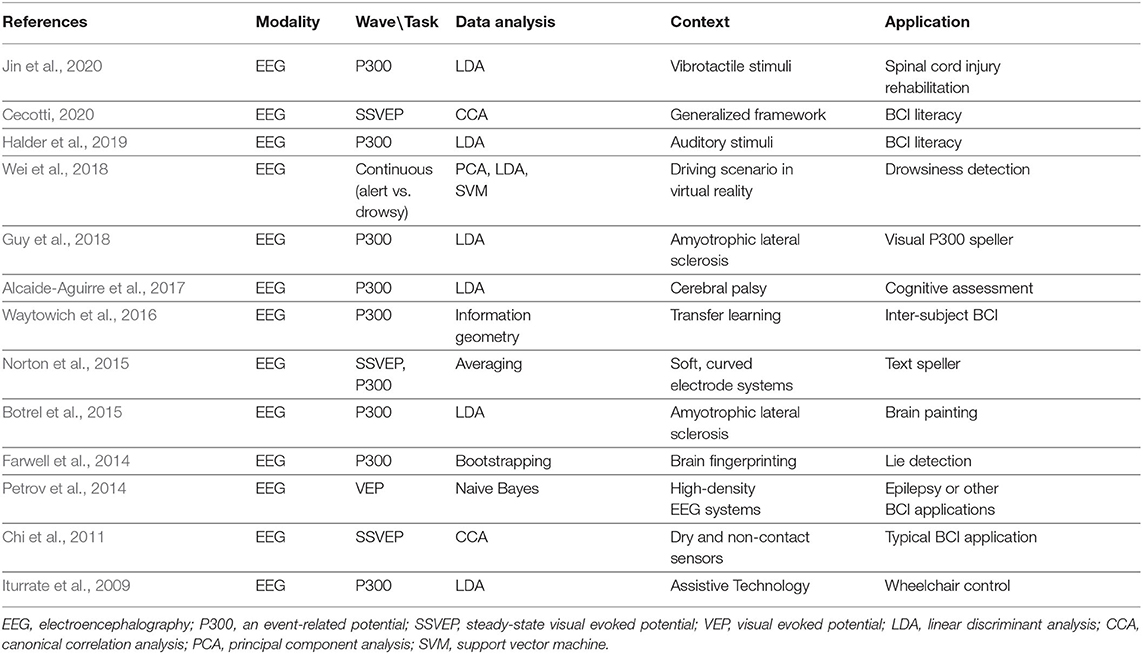
Table 3. A summary of non-sensorimotor rhythms and electroencephalography-based brain computer interface (BCI) studies.
EEG provides relatively poor spatial resolution due to non-invasive scalp recordings compared to fMRI, but finer temporal resolution (Lystad and Pollard, 2009; Min et al., 2010; He et al., 2011; Nicolas-Alonso and Gomez-Gil, 2012). Employing high density EEG mapping increases spatial resolution but results in high computational cost and efforts to maintain a reasonable signal-to-noise ratio across all channels (Chen et al., 2015). Since EEG captures only the electrical field associated cognitive processes, concomitant assessment of blood-oxygen level-dependent (BOLD) activity may improve BCI performance. BOLD activity is typically captured with fMRI (Sitaram et al., 2007), which is not feasible for most BCI applications, due to unmanageable size and cost of the device. fNIRS provides a safe, non-invasive, relatively inexpensive and portable neuroimaging alternative for recording BOLD activity (Matthews et al., 2007). Integrating fNIRS with EEG can significantly enhance classification performances regardless of low information transfer rate caused by inherent delays in hemodynamics (Fazli et al., 2012). A recent study has suggested that fNIRS is unable to adequately offer acceptable performances on its own, but can be combined with EEG to boost the performances (Ge et al., 2017). However, continuous technological advances could promote fNIRS as an exclusive tool for neuroscience research, including the development of BCI (Scholkmann et al., 2014; Naseer and Hong, 2015).
Probing sources in cortico-subcortical networks is another important limitation of scalp-based sensors such as EEG. Reconstructing task-induced networks while resolving the so-called inverse problem imposes a significant challenge. A two-equivalent-dipole model was applied on EEG data to discern the anatomical nature of the MI induced sources and to aid the classification performances (Kamousi et al., 2005). Saha et al. proposed a wavelet-based source localization approach to investigate MI-related sources and their impact on BCI performance (Saha et al., 2019a). The neuronal potentials attenuate through several tissue layers of complex geometry and diverse electrical properties; however, the magnetic permeability in the cerebrospinal fluid, skull, and skin, is consistent (da Silva, 2013). Thus, MEG can capture signal with less distortion than EEG. Although MEG provides better spatiotemporal resolution as compared to EEG, the magnetic field created by the brain is very small, requiring costly, stationary recording equipment (Mellinger et al., 2007; Corsi et al., 2019).
The BCI classifier design has to address two issues (Bashashati et al., 2007; Lotte et al., 2007, 2018). First, the dimensionality of the features set used for estimating the model parameters should be chosen for optimal performance based on the nature of the classifier. Second, the trade-off between bias and variance has to be considered and may involve regularizing the parameter estimation.
Covariate shift occurs when the features extracted from the training differ from those of test data impacting the classification performance (Krusienski et al., 2011). Covariate shift is an important issue requiring the application of adaptive methods for compensating feature space transitions (Jayaram et al., 2016; Saha and Baumert, 2020). The unsupervised subspace learning method enables session-to-session and subject-to-subject information transfers, augmenting BCI performance (Samek et al., 2013; Jayaram et al., 2016; Saha et al., 2018). The common spatial pattern, a supervised method, has been extensively used in EEG-based online and offline BCI settings (Ramoser et al., 2000; Wu et al., 2017a). A common problem with such a data-driven technique is over-fitting of the model parameters based on training sets, causing unreliable prediction on the test data (Sannelli et al., 2016). Recent studies integrated diverse methods into potential transfer learning frameworks for BCI including spatial filters (e.g., common spatial pattern), Riemannian geometry, Euclidean alignment and subspace adaptation and deep learning-based techniques (Barachant et al., 2011; Congedo et al., 2013, 2017; Marathe et al., 2015; Wu et al., 2016, 2017b; Wu et al., 2020; He and Wu, 2019, 2020; Kwon et al., 2019; Zhang and Wu, 2020).
3. Neuroplasticity, Sensors, Signal Processing, Modeling, and Applications
Exploiting neuroplasticity, designing hi-fidelity and customized neural sensors, applying advanced signal processing, and machine learning techniques are the key aspects of an effective BCI design. Tables 2–5 highlight diverse characteristics of BCI components and applications including signal acquisition modality, experimental paradigm, data analysis and pattern recognition, application field, and significance. Notably, there are no specific selection criteria for studies in Tables 2–5 due to the broad spectrum of topics covered in this review; however, they are summarized such that critical advances over the last several years can be appreciated.
3.1. Neuroplasticity and Cognitive Rehabilitation
The time-variant behavior of synapses within complex neural networks underpins the plastic characteristics of the brain and was first illustrated by Donald O. Hebb in 1949 (Brown and Milner, 2003). Neuroplasticity not only helps to assist cognitive and perceptual learning but also is the main ingredient for neurorehabilitation. How plastic a particular brain area is, may define the effectiveness of a neurofeedback strategy to induce specific activity patterns. Studies have shown visual cortices are plastic enough to produce robust neural signals for post-neurofeedback perceptual learning (Shibata et al., 2011; Amano et al., 2016). Another study has demonstrated if right/left hemispheric differences in neurofeedback-induced alpha activities are associated with visual information processing and motor behaviors, and, thus, control spatial attention (Jones and Sliva, 2020). fMRI-based neurofeedback training sessions induce the plasticity of attention-related behavior. Implications suggest that neurofeedback can offer rehabilitation of attentional deficit (Megan et al., 2015). A recent study has used neurofeedback to generate robust somatosensory oscillations associated with human perception (Brickwedde et al., 2019).
Closed-loop BCI with neurofeedback is assumed to contribute to the reorganization of cortical-subcortical neural networks and assist subjects in self-regulating specific brain rhythms; notwithstanding, the underlying mechanisms that alter neural substrates are still not fully-understood (Sitaram et al., 2017). For example, BCI-based covert visuomotor training modulates associated neural substrates, where the effects of modulated neural substrates are observed while performing that particular movement-related task (Vyas et al., 2018). Substantial changes in overt movement-related task following BCI-driven training induced learning suggest a critical role of BCI in enhanced motor learning for proficiently controlling neuroprosthetics (Orsborn et al., 2014), i.e., devices that can enhance or repair the output of the nervous system. For example, intracortical electrodes may be used to stimulate specific brain regions to regain motor control (Oxley et al., 2016; Sand et al., 2017). BCI may augment training-induced plasticity during therapeutic motor rehabilitation and, thus, re-excite corresponding neural substrates to regain control by means of neuroprosthetics or upper limb functions (Dobkin, 2007). Other examples include BCI-driven exoskeletons to enhance human working capacity (Benabid et al., 2019).
The extent of BCI-induced plasticity entails several factors, including (1) the selection of the signal acquisition modality, which plays an important role in diagnosing neural states, (2) the design of feedback modality that has explicit association with the neural signal classification performance, (3) the consideration of application-specific feedback delays, and (4) the utilization of a suitable feedback modality (Grosse-Wentrup et al., 2011). Neural ensemble recordings using signal acquisition modalities such as EEG, MEG, fNIRS, and fMRI have become dominant over single unit recordings. Behavioral activities are likely to be distributed across three-dimensional cortical-subcortical networks and that cannot be captured within single unit recordings (Nicolelis and Lebedev, 2009).
Rehabilitative BCI can be designed either by attaching neural prostheses to the impaired body parts or by re-stimulating the damaged synaptic networks; in any of the cases, the idea is to exploit and promote neuroplasticity (Wang et al., 2010; Ramos-Murguialday et al., 2013; Park et al., 2016; Darvishi et al., 2017; Toriyama et al., 2018; Song and Kim, 2019; Romero-Laiseca et al., 2020). In stroke patients with paretic muscles without residual finger movement, increased electromyographic activity post rehabilitation by BCI-driven orthoses exhibits increased neuromuscular coherence that is essential for restoring movement control (Pfurtscheller et al., 2000; Ramos-Murguialday et al., 2013). Explicit application of functional electrical stimulation regulated by EEG-based movement-related signatures further suggests a role of BCI in rehabilitation (Zhao et al., 2016). Increased electromyographic activity in paretic muscles is indicative of plasticity induced by electrical stimulation (De Marchis et al., 2016). For BCI-based rehabilitation in a real-life environment, differentiating between task-induced activities and resting state activities is a key factor for controlling the prosthesis or stimulation modality (Pahwa et al., 2015).
Externally stimulating the affected brain areas by electric or magnetic fields holds promise for stroke rehabilitation. A recent study demonstrated the induction of neuroplasticity in white matter and cortical functions in chronic stroke patients by motor imagery-based BCI and transcranial direct current stimulation applied to targeted brain areas (Hong et al., 2017). Magnetic stimulation of brain areas driven by BCI increases cortical activation in stroke patients (Johnson et al., 2018). The level of neuroplasticity achieved post-rehabilitation varies across subjects and, thus, an individual-specific training session is necessary (Leamy et al., 2014). The use of BCI-based motor rehabilitation for locked-in patients is limited because they are unable to fully interact with the system (Birbaumer and Cohen, 2007). Other examples of BCI-driven rehabilitations include optimizing the parameters for deep brain stimulation applied into the subthalamic nucleus in patients with Parkinson's disease (Sand et al., 2017) and treating major depressive disorder by BCI-driven transcranial magnetic stimulation (Ray et al., 2015).
Either by providing direct control of assistive technologies or by direct neurostimulation, BCI can help patients who may suffer from amyotrophic lateral sclerosis, cerebral palsy, brainstem stroke, spinal cord injuries, muscular dystrophies, or chronic peripheral neuropathies (Kauhanen et al., 2006; Iturrate et al., 2009; Mak and Wolpaw, 2009; Allison et al., 2010; Ramos-Murguialday et al., 2013; Leamy et al., 2014; Botrel et al., 2015; Combaz and Van Hulle, 2015; Edelman et al., 2015; Park et al., 2016; Zhao et al., 2016; Alcaide-Aguirre et al., 2017; Ge et al., 2017; Chiarelli et al., 2018; Guy et al., 2018; Yu et al., 2018; Rezazadeh Sereshkeh et al., 2019; Jin et al., 2020; Zuo et al., 2020). Providing auxiliary degrees of freedom improves the quality of life of people with disabilities significantly. Brain signals can be translated to drive wheelchairs (Galán et al., 2008; Iturrate et al., 2009; Perdikis et al., 2017; Tonin and Millán, 2020). Integration of BCI with a vision-guided autonomous system was shown to effectively perform the grasping task using a prosthetic arm in a tetraplegic patient (Downey et al., 2016). An implanted microelectrode array has been proposed to operate a three-dimensional neuroprosthetic device (Taylor et al., 2002).
3.2. Signal Acquisition, Signal Processing, and Modeling
A significant number of studies are now involved in combining multimodal signal acquisition modalities to augment current BCI systems. For example, simultaneous EEG and fMRI yield complementary features by exploiting good temporal resolution of EEG and good spatial resolution of fMRI (Debener et al., 2006). Enhanced multiclass sensorimotor tasks classification performance using hybrid EEG and fNIRS signals implicates the importance of features extracted from both hemodynamic and electrical activities (Buccino et al., 2016). MEG is another potential tool to combine with EEG, as it captures radially/tangentially dipole sources in cortical-subcortical networks and adds complementary information to EEG signals (Kauhanen et al., 2006). Skepticism might still present about the detection of brain activities originated from subcortical areas; however, an increasing number of studies argue that EEG and MEG could capture subcortical activities (Andersen et al., 2019; Min et al., 2020; Piastra et al., 2020). A recent trend is to combine different signal acquisition modalities together to improve BCI efficiency. Table 4 highlights multimodal and hybrid BCI applications.
The combination of signal processing and machine learning approaches plays critical role in translating any brain signal to a command for a computer or other external devices. Tables 2–5 highlight different signal processing and machine learning techniques. Representing signals in the time-frequency-space is necessary to obtain physiological correlates of BCI outcomes (McFarland et al., 2006; Bashashati et al., 2007). Fourier transform (FT) and autoregressive models are examples of time domain representations of brain signals while short time FT and wavelet transform are examples of time-frequency representations (McFarland et al., 2006; Bashashati et al., 2007). In case of spatial filtering, the most popular filtering approaches are common spatial pattern, independent component analysis and the Laplacian filter. A diverse range of inverse models allow to discern the actual sources projected on three-dimensional cortical-subcortical networks (Wronkiewicz et al., 2015; Saha et al., 2019a). Extracted features can be translated using various linear and non-linear classification algorithms. Examples of linear and non-linear classifier models are linear discriminant analysis and non-linear kernel-based support vector machines (Lotte et al., 2007, 2018).
Since the first publication in 2000, common spatial pattern is still one of the most popular methods to represent multichannel EEG signals by corresponding spatial contents (Ramoser et al., 2000). As a data-driven method, it requires a significant number of training samples to model the filtering parameters. In case of small training trials, regularizing the covariance estimation works better than the traditional algorithm (Lotte and Guan, 2010). Other modifications in spatial filtering include projecting EEG by using sparse representation and filter bank spectral division of raw signals (Arvaneh et al., 2014). Generally, spatial filtering is applicable in subject-specific BCI development although recent studies have proposed estimating the filter coefficients from a subject and applied that filter to another subject, which contributed no training sample (Saha et al., 2017, 2018, 2019a). Other popular data-driven methods include linear discriminant analysis, support vector machine and principal component analysis (Lotte et al., 2007, 2018). With the exceptional advancements in computational facilities in the last decade, deep learning-based BCI paradigms by allowing the evaluation of large datasets could soon become a trend in the community (Chiarelli et al., 2018; Kwon et al., 2019; Nagel and Spüler, 2019).
On the other hand, independent component analysis is a blind source separation method requiring no training. The estimation of independent components is based on statistical properties of the signals (Bell and Sejnowski, 1995). However, modeling the actual cortical sources as dipoles in the complex brain anatomy from the scalp EEG recordings seeks to solve the so-called inverse problem (Qin et al., 2004; Kamousi et al., 2005; Wronkiewicz et al., 2015; Saha et al., 2019a). More recent source localization methods such as wavelet-based maximum entropy on the mean represent EEG/MEG signals as relevant time-frequency contents and finally transform them into spatial representations (Lina et al., 2012; Saha et al., 2019a). Notably, different inverse methods and toolboxes demonstrate considerable variability in localized sources (Mahjoory et al., 2017). Even it is not very straightforward to know the exact sources, which are to be modeled using EEG/MEG. For example, the ground truth defined by implanted electrodes might not be 100% reliable because of sparse (spatial) sampling. In the case of fMRI, the measurement of neural activity is indirect. Notwithstanding, inverse methods have shown promise for designing various BCI models (Qin et al., 2004; Kamousi et al., 2005; Wronkiewicz et al., 2015; Saha et al., 2019a).
3.3. Neurosensors: The-State-of-the-Art
Deeper regions of the brain, e.g., subcortical and cerebellar regions, contribute to various neuronal activities (Müller et al., 2002; Wardman et al., 2014). Interpreting the genesis of cortical sources from cellular to scalp levels and RSNs spanned throughout the three-dimensional brain space can guide BCI development (Donoghue, 2008). Sensors with customized design are developed to advance brain signal acquisition modalities. Neurosensors can be constructed in different forms like electrical, optical, chemical and biological (Deisseroth and Schnitzer, 2013). Dry EEG electrodes are convenient, but assumed to provide lower signal-to-noise ratio compared to conventional wet electrodes. Wet electrodes may cause inconvenience to users as they use conductive gel and require proper skin preparation for minimizing the skin-electrode impedance (Liao et al., 2012). However, a study on dry electrodes-based BCI suggested that dry electrode could be used to collect good quality signals by designing the circuits carefully (Chi et al., 2011). Further studies support dry electrodes with wireless systems that could offer comparable signal quality as of wet electrodes, but with more convenience (Di Flumeri et al., 2019; Marini et al., 2019; Hinrichs et al., 2020). While utilizing the advantages of both dry and wet electrodes, quasi-dry electrodes exploiting the mechanical properties of polymer can capture signals as comparable to commercial Ag/AgCl electrodes (Mota et al., 2013). To increase the spatial resolution of EEG, Petrov et al. have proposed an ultra-dense sensor array of 700–800 electrodes (Petrov et al., 2014). The signal-to-noise ratio was twice as high as for high-density EEG that has up to 256 gold-coated electrodes. An auricle electrode with stretchable connector was proposed that not only can increase portability but also can offer a comfortable alternative for long term recordings (Norton et al., 2015). The electrode is flexible with the alterations of electrical and mechanical properties of skin.
Invasive sensors must be biocompatible. A novel organic electrochemical transistor-based sensor enables to collect neural signals directly from the brain surface (Khodagholy et al., 2013). This sensor is biocompatible and mechanically flexible, and the transistor-based design amplifies captured signals locally, thus providing much better signal-to-noise ratio than conventional ECoG. To enhance the signal quality, carbon nanotube coating can decrease the electrode impedance and, thus, increase the charge transfer (Keefer et al., 2008). Another invasive biocompatible sensor, designed for recording previously inaccessible spectra of large neuron populations, includes data transmission for use in natural environments (Yin et al., 2014). With the outstanding progress of nanotechnology, nanowire Field Effect Transistor and other p/n junction devices have potential for neuro-sensing modalities for intracellular recordings, even in the deep brain regions (Kruskal et al., 2015). Oxley et al. have proposed stent-electrode array (stentrode) that involves minimal invasiveness (Oxley et al., 2016, 2017). Using computer-guided catheter angiography, the stentrode can be placed within arteries or veins located inside the brain anatomy. Capturing high-fidelity cortical signals, this technology will significantly reduce the risk factors of craniotomy. A follow-up study has recently demonstrated successful implantation of the strentrode in humans for long-term neural signal recording (Oxley et al., 2020). The information transfer rate for strentrode-based BCI was comparable to the landmark study by Vansteensel et al. with implanted electrodes (Vansteensel et al., 2016). Another implanted ECoG recorder called as WIMAGINE (Wireless Implantable Multi-channel Acquisition system for Generic Interface with Neurons) allows wireless neural data access (Mestais et al., 2014). The WIMAGINE has recently been tested for long-term reliability of data acquisition and any risk associated with craniotomy (Kruskal et al., 2015; Sauter-Starce et al., 2019).
Besides large-scale recording modalities like EEG and MEG, very small-scale recordings of neuronal activities are crucial for understanding brain circuits' functions and intra- and inter-neuron interactions. Representation of any cognitive task as functions of both small-scale and large-scale neuronal interactions is crucial for the advancement of neuroengineering and BCI. In this regard, a high-density neurosensor array made from silicon probes combined with optogenetics enables single unit recordings (Buzsáki et al., 2015). Yang et al. proposed a novel multi-plane two-photon microscope that can be used to capture multi-layer neuronal structure and mechanism with cellular resolution (Yang et al., 2016). Other potential imaging methods for investigating cell signaling include calcium imaging (Grienberger and Konnerth, 2012) and advanced microscope with chronically implanted lenses (Resendez et al., 2016). Designer receptor exclusively activated by designer drugs, provides a chemogenetic tool to understand cell-signaling including electrical activities in molecularly clustered cell groups (Sternson and Roth, 2014; Roth, 2016). A new ultrasonic-based wireless system, called neural dust, enables the recording of electromyogram and electroneurogram on the millimeter scale (Seo et al., 2016).
3.4. Affective Computing, Gaming, Robotics, and Miscellaneous Applications
Future computers are assumed to have emotional and perceptual capabilities, which could extend the use not only to assisting humans but also to making decisions (Picard, 2000). Computers might able to recognize and interpret underlying affective states based on physiological and behavioral variables. Recent studies demonstrated BCI is a potential tool to investigate affective states, expanding the applications into psychology (Piho and Tjahjadi, 2018; Song et al., 2018; Huang et al., 2019). Huang et al. have proposed an EEG-based BCI to detect positive and negative emotions induced by video stimulus (Huang et al., 2019).
Integration of arts into BCI is referred to as artistic BCI (Andujar et al., 2015). In the late 1960s, David Rosenboom began experimenting with ways to link brain functions with musical production, perception of musical forms and musical proprioception (Rosenboom, 2014). Other examples of artistic BCI include affective states detection, playing video games and controlling virtual/augmented reality environment. Studies have demonstrated that a user can fully operate video games by SSVEP-BCI (van Vliet et al., 2012; Filiz and Arslan, 2020). Other studies have proposed how multiple users can participate in a collaborative game, in which joint decision making is required to control the gaming environment (Nijholt and Poel, 2016; Sekhavat, 2020). Another study previously suggested the aggregation of information from two intelligence analysts' brain signals may lead to better decision making than one's brain signals (Stoica, 2012). The underlying cause could be explained by inter-individual differences in human cognitive and perceptual skills (Kleinschmidt et al., 2012). Collaboration between users might assist an individual's decision making by diversity inclusion. A modified setup could investigate how people interact in different social contexts, extending BCI applications in sociology (Amaral et al., 2017).
Virtual/augmented reality (V/AR) technologies together with BCI could offer immersive experiences and have many potential applications including arts and neurofeedback (Andujar et al., 2015; Tremmel et al., 2019; Putze et al., 2020). Brain painting allows a user to draw lines in a virtual canvas by brain signals, which gives an alternative communication channel for people with paretic motor functions (Botrel et al., 2015). McClinton et al. have developed a brain painting application using VR environment (McClinton et al., 2019). Another work has evinced VR-BCI to measure cognitive workload that can contribute to neuroergonomics (Tremmel et al., 2019). Studies have also used immersive VR as a better neurofeedback option as compared to the computer screen leading to increased BCI accuracy (Luu et al., 2016; Škola et al., 2019; Vourvopoulos et al., 2019; Juliano et al., 2020). Vourvopoulos et al. have integrated the principles of VR and BCI into a platform called REINVENT for motor rehabilitation (Vourvopoulos et al., 2019). Likewise, BCI with AR can be used to remotely control a robot for rehabilitating children with attention-deficit/hyperactivity disorder (Arpaia et al., 2020).
While BCI-driven robotic controllers can offer advanced assistive technology for people with mobility constraint, it may also augment human ergonomic performance for healthy subjects (Millan et al., 2004; Gandhi et al., 2014; Tidoni et al., 2016; Perdikis et al., 2017; Spataro et al., 2017; Yuan and Li, 2018; Deng et al., 2019; Tonin et al., 2019; Tonin and Millán, 2020). EEG-based BCI-driven controller of mobile robot or wheelchair has demonstrated the possibility of this technology in robotics industry (Millan et al., 2004; Tidoni et al., 2016; Perdikis et al., 2017; Yuan and Li, 2018; Deng et al., 2019; Tonin et al., 2019). BCI can also be used for controlling humanoid robots remotely using EEG (Spataro et al., 2017), suitable in hazardous environments, for example by sending a robot in a coal mine for executing a task that is potentially unsafe for a human. In space, BCI can be used to monitor astronauts' working capacity and to drive an exoskeleton (Menon et al., 2009; de Negueruela et al., 2011). In the absence of gravity, working becomes tedious and inconvenient. Furthermore, astronauts' working time is precious. BCI-driven systems could be practical for improving astronauts' functionality, efficiency and safety (Summerer et al., 2009; Farwell et al., 2014; Botrel et al., 2015; Ortiz et al., 2016; Wang et al., 2016a; Vourvopoulos et al., 2019; Singh et al., 2020).
Recently, brain-to-brain interface (BBI) experiments that involve decoding sender's cognitive intentions, translate them into commands for stimulating receiver's brain, have been explored (Pais-Vieira et al., 2013; Rao et al., 2014; Jiang et al., 2019). In 2013, researchers implemented a direct BBI system in which one rat was able to share sensorimotor information to another rat (Pais-Vieira et al., 2013). Intracortical microstimulation was used to stimulate the receiver's target brain areas. An early attempt to develop sensorimotor rhythm-based BBI between two human subjects used non-invasive EEG and transcranial magnetic stimulation has been proposed by Rao et al. (2014). Other total non-invasive BBI experiments have proposed sharing pseudo-random binary streams encoded words between human subjects (Grau et al., 2014) and playing collaborative games (Stocco et al., 2015). Figure 2 illustrates a timeline for current advances of BCI in diverse applications.
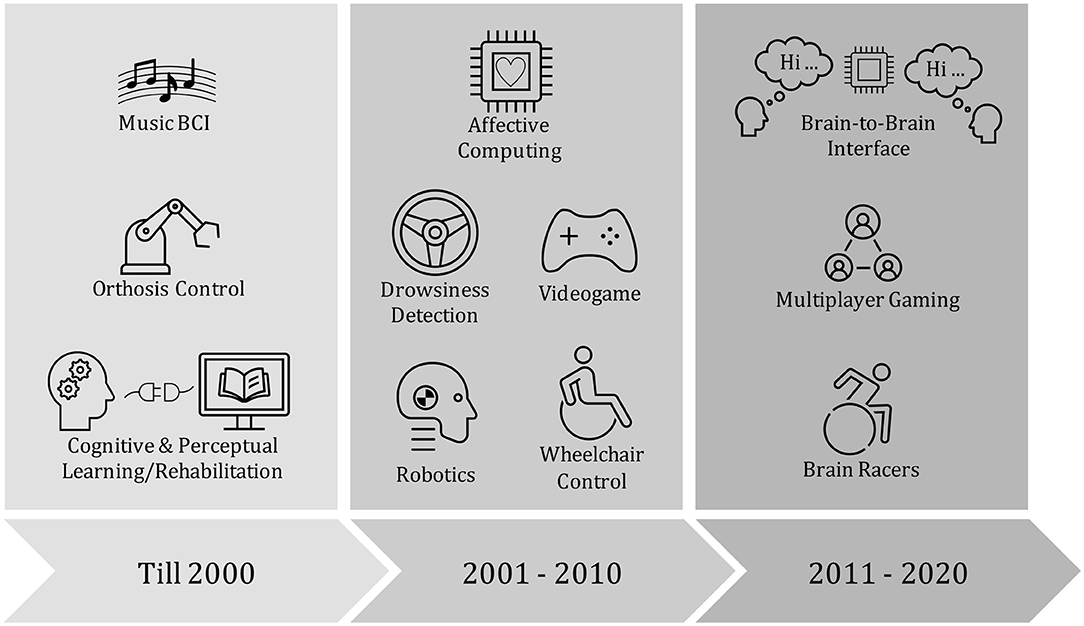
Figure 2. A schematic illustration of the evolution of the brain computer interface (BCI) applications: Cognitive & Perceptual Learning/Rehabilitation (McMillan et al., 1995); Orthosis Control (Pfurtscheller et al., 2000); Music BCI (Rosenboom, 2014); Robotics (Millan et al., 2004); Wheelchair Control (Iturrate et al., 2009); Drowsiness Detection (Lin et al., 2008); Affective Computing (Zander et al., 2009); Brain Racers (Perdikis et al., 2017); Multiplayer Gaming (Nijholt and Poel, 2016); Brain-to-Brain Interface (Rao et al., 2014).
4. Ethical Concerns and Socioeconomic Contexts
Irrespective of the scientific breakthroughs in BCI field, there are key factors pertaining to safety, ethics, privacy protection and data confidentiality, community acceptance and socioeconomic aspects that should be considered with adequate precautions to maximize users' benefits and social impacts (Illes and Bird, 2006; Bostrom and Sandberg, 2009; Jebari, 2013; McCullagh et al., 2014). Obtaining an ethically sound informed consent from a BCI worn patient may be challenging for BCI researchers due to difficulty in communicating and the lack of alternatives. However, more awareness and attention to ethics policies are recommended to improve the chance for patients to get adequate information.
Physical and mental safety of BCI users is important. Invasive procedures such as deep brain stimulation and intracortical microelectrode array may cause postoperative psychological and neurological side effects (Jotterand and Giordano, 2011; Gilbert, 2015; Maslen et al., 2015). Additionally, bleeding and infections are infrequent but do occur and may require removal or further maintenance of the implanted electrodes. Guidelines are required to safely advance neurotechnologies (Goering and Yuste, 2016), because BCI devices can alter behavior and, thus, introduce potential threats to one's emotions, personality and memories; more generally one's mind. For human brain-to-brain interface applications (Rao et al., 2014; Stocco et al., 2015), one may define an upper bound for research depths keeping in mind the necessity of ethical utilization of this technology. Because both sender and receiver play complicated roles, more specifically, sender's intentional manipulative control over neural signals might alter the anticipated outcome. Altering human cognitive and possibly moral capacity raise a serious ethical question and it is not predictable if the cognitive changes reversible and efficacious (Nakazawa et al., 2016).
A user's expectations of achieving extended or auxiliary degree of freedom may not be fulfilled, and even the unfamiliar risk factors can diminish the accomplished advantage of using BCI (Clausen, 2011; Schicktanz et al., 2015). Creating broad awareness of BCI technology and its pros and cons would educate people, who fear unnecessary technological dependency (Hobson et al., 2017). However, successful clinical trials of sophisticated devices such as strentrode or WIMAGINE are essential to demonstrate potential advantages, especially for people suffering from any form of cognitive disability (Sauter-Starce et al., 2019; Oxley et al., 2020). In the case of healthy users, it should not be too difficult to create acceptance to a broader community when dry electrodes could offer the long-term operation of a BCI application with little maintenance effort (Di Flumeri et al., 2019; Marini et al., 2019; Hinrichs et al., 2020).
It is critical to introduce a suitable act for lawful utilization of BCI and preservation of privacy and confidentiality of stored data. Recent studies have demonstrated decoding of password or recognizing faces utilizing consumer-grade BCI successfully, prompting a potential concern of any illegitimate access to users' raw data and their further exploitation (Martin et al., 2016; Alomari et al., 2019). For example, affective states define users' moral judgment and emotional traits. Thus, it is critical to limit the applications of affective BCI while preserving sensitive information (Steinert and Friedrich, 2020). Necessary precursor initiatives should propose application-specific BCI frameworks, which can restrict unauthorized access to stored data or the system (Ienca and Haselager, 2016). For example, illicit access to a wireless BCI-driven limb and manipulative reprogramming of a computer-guided neuro-stimulation have demonstrated the importance of establishing resilient safeguards to BCI use (Denning et al., 2009). Agarwal et al. have proposed cryptographic protocols as integrated parts of BCI to preserve the privacy of a user by keeping confidential information obscure to others (Agarwal et al., 2019). Without evaluating socioeconomic, ethical and policy issues, the commercialization of BCI would hinder the progress in this field (Eaton and Illes, 2007).
By creating a common networking platform for BCI researchers worldwide, the immediate proposition of a comprehensive list of universal guidelines is key to sustainable advancements of the field (Vaadia and Birbaumer, 2009). Various alliance-based projects are running as common platforms for advancing the knowledge of neuroscience, for example, by strengthening efforts to fund neuroscience research projects (Grillner et al., 2016). The European Union along with its partner universities have initiated the Human Brain Project. In addition, the Brain Initiative has been announced by the White House. In our opinion, advanced understanding of basic neuroscientific phenomena will determine the structure, efficacy and applications of futuristic BCI.
5. Conclusion
Numerous groundbreaking advances in neurosensors and computational tools herald great promise for more sophisticated and user friendly BCI systems requiring no or little maintenance. In addition to hi-fidelity signal acquisition, significant progress in signal processing and machine learning tools, their complementary roles, and high computation power and increased mobility of computers have significantly contributed in the emergence of BCI technologies. The future of BCI technology will rely greatly on addressing the following key aspects:
• Elucidating the underlying psychophysiological and neurological factors that potentially influence BCI performance.
• Designing less invasive sensors with reliable signal acquisition and resolution, while considering portability, easy maintenance, and affordability.
• Modeling session-to-session and subject-to-subject information transfer for the proposition of more generalized BCI models with insignificant or no calibration requirement.
• Establishing broad consensus on ethical issues and beneficial socioeconomic application of this technology.
Author Contributions
SS conceived the initial idea, wrote the first draft, and generated all figures and tables. KM, KA, RM, GN, and SD participated in the discussion and commented on the draft. AHK and MB provided further insight and helped SS to finalize the structure and materials. All authors read and approved the final paper.
Conflict of Interest
The authors declare that the research was conducted in the absence of any commercial or financial relationships that could be construed as a potential conflict of interest.
Acknowledgments
This manuscript has been released as a pre-print at https://arxiv.org/ (Saha et al., 2019b). Authors would like to thank Prof. Moritz Grosse-Wentrup for providing his valuable feedback. This work was partially supported by a grant (Award No. RC2-2018-022 (HEIC) and KKJRC-2019-Health 2) from Khalifa University, Abu Dhabi, UAE.
References
Abrams, D. A., Ryali, S., Chen, T., Chordia, P., Khouzam, A., Levitin, D. J., et al. (2013). Inter-subject synchronization of brain responses during natural music listening. Eur. J. Neurosci. 37, 1458–1469. doi: 10.1111/ejn.12173
Abu-Alqumsan, M., and Peer, A. (2016). Advancing the detection of steady-state visual evoked potentials in brain-computer interfaces. J. Neural Eng. 13:036005. doi: 10.1088/1741-2560/13/3/036005
Acqualagna, L., Botrel, L., Vidaurre, C., Kübler, A., and Blankertz, B. (2016). Large-scale assessment of a fully automatic co-adaptive motor imagery-based brain computer interface. PLoS ONE 11:e0148886. doi: 10.1371/journal.pone.0148886
Agarwal, A., Dowsley, R., McKinney, N. D., Wu, D., Lin, C.-T., De Cock, M., et al. (2019). Protecting privacy of users in brain-computer interface applications. IEEE Trans. Neural Syst. Rehabil. Eng. 27, 1546–1555. doi: 10.1109/TNSRE.2019.2926965
Ahn, M., and Jun, S. C. (2015). Performance variation in motor imagery brain-computer interface: a brief review. J. Neurosci. Methods 243, 103–110. doi: 10.1016/j.jneumeth.2015.01.033
Alcaide-Aguirre, R., Warschausky, S., Brown, D., Aref, A., and Huggins, J. (2017). Asynchronous brain-computer interface for cognitive assessment in people with cerebral palsy. J. Neural Eng. 14:066001. doi: 10.1088/1741-2552/aa7fc4
Allison, B. Z., Brunner, C., Kaiser, V., Müller-Putz, G. R., Neuper, C., and Pfurtscheller, G. (2010). Toward a hybrid brain-computer interface based on imagined movement and visual attention. J. Neural Eng. 7:026007. doi: 10.1088/1741-2560/7/2/026007
Alomari, R., Martin, M. V., MacDonald, S., Maraj, A., Liscano, R., and Bellman, C. (2019). Inside out-a study of users' perceptions of password memorability and recall. J. Inform. Security Appl. 47, 223–234. doi: 10.1016/j.jisa.2019.05.009
Amano, K., Shibata, K., Kawato, M., Sasaki, Y., and Watanabe, T. (2016). Learning to associate orientation with color in early visual areas by associative decoded fMRI neurofeedback. Curr. Biol. 26, 1861–1866. doi: 10.1016/j.cub.2016.05.014
Amaral, C. P., Simões, M. A., Mouga, S., Andrade, J., and Castelo-Branco, M. (2017). A novel brain computer interface for classification of social joint attention in autism and comparison of 3 experimental setups: a feasibility study. J. Neurosci. Methods 290, 105–115. doi: 10.1016/j.jneumeth.2017.07.029
Andersen, L. M., Jerbi, K., and Dalal, S. S. (2019). Can electro-and magnetoencephalography detect signals from the human cerebellum? PeerJ 7:e27901. doi: 10.7287/peerj.preprints.27901
Andersen, L. M., Jerbi, K., and Dalal, S. S. (2020). Can EEG and MEG detect signals from the human cerebellum? Neuroimage 215:116817. doi: 10.1016/j.neuroimage.2020.116817
Andujar, M., Crawford, C. S., Nijholt, A., Jackson, F., and Gilbert, J. E. (2015). Artistic brain-computer interfaces: the expression and stimulation of the user's affective state. Brain Comput. Interfaces 2, 60–69. doi: 10.1080/2326263X.2015.1104613
Aricó, P., Borghini, G., Di Flumeri, G., Colosimo, A., Bonelli, S., Golfetti, A., et al. (2016). Adaptive automation triggered by EEG-based mental workload index: a passive brain-computer interface application in realistic air traffic control environment. Front. Hum. Neurosci. 10:539. doi: 10.3389/fnhum.2016.00539
Arpaia, P., Duraccio, L., Moccaldi, N., and Rossi, S. (2020). Wearable brain-computer interface instrumentation for robot-based rehabilitation by augmented reality. IEEE Trans. Instrument. Meas. 69, 6362–6371. doi: 10.1109/TIM.2020.2970846
Arvaneh, M., Guan, C., Ang, K. K., and Quek, C. (2014). Mutual information-based optimization of sparse spatio-spectral filters in brain-computer interface. Neural Comput. Appl. 25, 625–634. doi: 10.1007/s00521-013-1523-7
Barachant, A., Bonnet, S., Congedo, M., and Jutten, C. (2011). Multiclass brain-computer interface classification by riemannian geometry. IEEE Trans. Biomed. Eng. 59, 920–928. doi: 10.1109/TBME.2011.2172210
Bashashati, A., Fatourechi, M., Ward, R. K., and Birch, G. E. (2007). A survey of signal processing algorithms in brain-computer interfaces based on electrical brain signals. J. Neural Eng. 4:R32. doi: 10.1088/1741-2560/4/2/R03
Baxter, B. S., Edelman, B. J., Sohrabpour, A., and He, B. (2017). Anodal transcranial direct current stimulation increases bilateral directed brain connectivity during motor-imagery based brain-computer interface control. Front. Neurosci. 11:691. doi: 10.3389/fnins.2017.00691
Bell, A. J., and Sejnowski, T. J. (1995). An information-maximization approach to blind separation and blind deconvolution. Neural Comput. 7, 1129–1159. doi: 10.1162/neco.1995.7.6.1129
Benabid, A. L., Costecalde, T., Eliseyev, A., Charvet, G., Verney, A., Karakas, S., et al. (2019). An exoskeleton controlled by an epidural wireless brain-machine interface in a tetraplegic patient: a proof-of-concept demonstration. Lancet Neurol. 18, 1112–1122. doi: 10.1016/S1474-4422(19)30321-7
Berger, H. (1929). Über das elektroenkephalogramm des menschen. Arch. Psychiatr. Nervenkrankheiten 87, 527–570. doi: 10.1007/BF01797193
Bhattacharyya, S., Clerc, M., and Hayashibe, M. (2019). Augmenting motor imagery learning for brain-computer interfacing using electrical stimulation as feedback. IEEE Trans. Med. Robot. Bionics 1, 247–255. doi: 10.1109/TMRB.2019.2949854
Birbaumer, N., and Cohen, L. G. (2007). Brain-computer interfaces: communication and restoration of movement in paralysis. J. Physiol. 579, 621–636. doi: 10.1113/jphysiol.2006.125633
Birbaumer, N., Ghanayim, N., Hinterberger, T., Iversen, I., Kotchoubey, B., Kübler, A., et al. (1999). A spelling device for the paralysed. Nature 398, 297–298. doi: 10.1038/18581
Blankertz, B., Sanelli, C., Halder, S., Hammer, E., Kübler, A., Müller, K.-R., et al. (2009). Predicting BCI performance to study BCI illiteracy. BMC Neurosci. 10(Suppl. 1):P84. doi: 10.1186/1471-2202-10-S1-P84
Bockbrader, M., Annetta, N., Friedenberg, D., Schwemmer, M., Skomrock, N., Colachis, I. V., et al. (2019). Clinically significant gains in skillful grasp coordination by an individual with tetraplegia using an implanted brain-computer interface with forearm transcutaneous muscle stimulation. Arch. Phys. Med. Rehabil. 100, 1201–1217. doi: 10.1016/j.apmr.2018.07.445
Bostrom, N., and Sandberg, A. (2009). Cognitive enhancement: methods, ethics, regulatory challenges. Sci. Eng. Ethics 15, 311–341. doi: 10.1007/s11948-009-9142-5
Botrel, L., Holz, E., and Kübler, A. (2015). Brain painting v2: evaluation of p300-based brain-computer interface for creative expression by an end-user following the user-centered design. Brain Comput. Interfaces 2, 135–149. doi: 10.1080/2326263X.2015.1100038
Brickwedde, M., Krüger, M. C., and Dinse, H. R. (2019). Somatosensory alpha oscillations gate perceptual learning efficiency. Nat. Commun. 10, 1–9. doi: 10.1038/s41467-018-08012-0
Brown, R. E., and Milner, P. M. (2003). The legacy of Donald O. Hebb: more than the Hebb synapse. Nat. Rev. Neurosci. 4, 1013–1019. doi: 10.1038/nrn1257
Brunner, C., Birbaumer, N., Blankertz, B., Guger, C., Kübler, A., Mattia, D., et al. (2015). BNCI horizon 2020: towards a roadmap for the BCI community. Brain Comput. Interfaces 2, 1–10. doi: 10.1080/2326263X.2015.1008956
Buccino, A. P., Keles, H. O., and Omurtag, A. (2016). Hybrid EEG-fNIRS asynchronous brain-computer interface for multiple motor tasks. PLoS ONE 11:e0146610. doi: 10.1371/journal.pone.0146610
Buzsáki, G., Stark, E., Berényi, A., Khodagholy, D., Kipke, D. R., Yoon, E., et al. (2015). Tools for probing local circuits: high-density silicon probes combined with optogenetics. Neuron 86, 92–105. doi: 10.1016/j.neuron.2015.01.028
Calhoun, V. D., and Adali, T. (2016). Time-varying brain connectivity in fMRI data: whole-brain data-driven approaches for capturing and characterizing dynamic states. IEEE Signal Process. Mag. 33, 52–66. doi: 10.1109/MSP.2015.2478915
Cecotti, H. (2020). Adaptive time segment analysis for steady-state visual evoked potential based brain-computer interfaces. IEEE Trans. Neural Syst. Rehabil. Eng. 28, 552–560. doi: 10.1109/TNSRE.2020.2968307
Chen, X., Wang, Y., Nakanishi, M., Gao, X., Jung, T.-P., and Gao, S. (2015). High-speed spelling with a noninvasive brain-computer interface. Proc. Natl. Acad. Sci. U.S.A. 112, E6058–E6067. doi: 10.1073/pnas.1508080112
Chi, Y. M., Wang, Y.-T., Wang, Y., Maier, C., Jung, T.-P., and Cauwenberghs, G. (2011). Dry and noncontact EEG sensors for mobile brain-computer interfaces. IEEE Trans. Neural Syst. Rehabil. Eng. 20, 228–235. doi: 10.1109/TNSRE.2011.2174652
Chiarelli, A. M., Croce, P., Merla, A., and Zappasodi, F. (2018). Deep learning for hybrid EEG-fNIRS brain-computer interface: application to motor imagery classification. J. Neural Eng. 15:036028. doi: 10.1088/1741-2552/aaaf82
Choi, B., and Jo, S. (2013). A low-cost EEG system-based hybrid brain-computer interface for humanoid robot navigation and rECOGnition. PLoS ONE 8:e74583. doi: 10.1371/journal.pone.0074583
Clausen, J. (2011). Conceptual and ethical issues with brain-hardware interfaces. Curr. Opin. Psychiatry 24, 495–501. doi: 10.1097/YCO.0b013e32834bb8ca
Combaz, A., and Van Hulle, M. M. (2015). Simultaneous detection of p300 and steady-state visually evoked potentials for hybrid brain-computer interface. PLoS ONE 10:e0121481. doi: 10.1371/journal.pone.0121481
Congedo, M., Barachant, A., and Andreev, A. (2013). A new generation of brain-computer interface based on riemannian geometry. arXiv preprint arXiv:1310.8115.
Congedo, M., Barachant, A., and Bhatia, R. (2017). Riemannian geometry for EEG-based brain-computer interfaces; a primer and a review. Brain Comput. Interfaces 4, 155–174. doi: 10.1080/2326263X.2017.1297192
Corsi, M.-C., Chavez, M., Schwartz, D., Hugueville, L., Khambhati, A. N., Bassett, D. S., et al. (2019). Integrating EEG and MEG signals to improve motor imagery classification in brain-computer interface. Int. J. Neural Syst. 29:1850014. doi: 10.1142/S0129065718500144
da Silva, F. L. (2013). EEG and MEG: relevance to neuroscience. Neuron 80, 1112–1128. doi: 10.1016/j.neuron.2013.10.017
Darvishi, S., Gharabaghi, A., Boulay, C. B., Ridding, M. C., Abbott, D., and Baumert, M. (2017). Proprioceptive feedback facilitates motor imagery-related operant learning of sensorimotor β-band modulation. Front. Neurosci. 11:60. doi: 10.3389/fnins.2017.00060
De Marchis, C., Monteiro, T. S., Simon-Martinez, C., Conforto, S., and Gharabaghi, A. (2016). Multi-contact functional electrical stimulation for hand opening: electrophysiologically driven identification of the optimal stimulation site. J. Neuroeng. Rehabil. 13, 1–9. doi: 10.1186/s12984-016-0129-6
de Negueruela, C., Broschart, M., Menon, C., and Millán, J. d. R. (2011). Brain-computer interfaces for space applications. Pers. Ubiquit. Comput. 15, 527–537. doi: 10.1007/s00779-010-0322-8
Debener, S., Ullsperger, M., Siegel, M., and Engel, A. K. (2006). Single-trial EEG-fMRI reveals the dynamics of cognitive function. Trends Cogn. Sci. 10, 558–563. doi: 10.1016/j.tics.2006.09.010
Deisseroth, K., and Schnitzer, M. J. (2013). Engineering approaches to illuminating brain structure and dynamics. Neuron 80, 568–577. doi: 10.1016/j.neuron.2013.10.032
Deng, X., Yu, Z. L., Lin, C., Gu, Z., and Li, Y. (2019). A bayesian shared control approach for wheelchair robot with brain machine interface. IEEE Trans. Neural Syst. Rehabil. Eng. 28, 328–338. doi: 10.1109/TNSRE.2019.2958076
Denning, T., Matsuoka, Y., and Kohno, T. (2009). Neurosecurity: security and privacy for neural devices. Neurosurg. Focus 27:E7. doi: 10.3171/2009.4.FOCUS0985
Di Flumeri, G., Aricó, P., Borghini, G., Sciaraffa, N., Di Florio, A., and Babiloni, F. (2019). The dry revolution: evaluation of three different EEG dry electrode types in terms of signal spectral features, mental states classification and usability. Sensors 19:1365. doi: 10.3390/s19061365
Dobkin, B. H. (2007). Brain-computer interface technology as a tool to augment plasticity and outcomes for neurological rehabilitation. J. Physiol. 579, 637–642. doi: 10.1113/jphysiol.2006.123067
Donoghue, J. P. (2008). Bridging the brain to the world: a perspective on neural interface systems. Neuron 60, 511–521. doi: 10.1016/j.neuron.2008.10.037
Downey, J. E., Weiss, J. M., Muelling, K., Venkatraman, A., Valois, J.-S., Hebert, M., et al. (2016). Blending of brain-machine interface and vision-guided autonomous robotics improves neuroprosthetic arm performance during grasping. J. Neuroeng. Rehabil. 13, 1–12. doi: 10.1186/s12984-016-0134-9
Eaton, M. L., and Illes, J. (2007). Commercializing cognitive neurotechnology–the ethical terrain. Nat. Biotechnol. 25, 393–397. doi: 10.1038/nbt0407-393
Edelman, B. J., Baxter, B., and He, B. (2015). EEG source imaging enhances the decoding of complex right-hand motor imagery tasks. IEEE Trans. Biomed. Eng. 63, 4–14. doi: 10.1109/TBME.2015.2467312
Faress, A., and Chau, T. (2013). Towards a multimodal brain-computer interface: combining fNIRS and ftcd measurements to enable higher classification accuracy. Neuroimage 77, 186–194. doi: 10.1016/j.neuroimage.2013.03.028
Farwell, L. A., and Donchin, E. (1988). Talking off the top of your head: toward a mental prosthesis utilizing event-related brain potentials. Electroencephalogr. Clin. Neurophysiol. 70, 510–523. doi: 10.1016/0013-4694(88)90149-6
Farwell, L. A., Richardson, D. C., Richardson, G. M., and Furedy, J. J. (2014). Brain fingerprinting classification concealed information test detects us navy military medical information with p300. Front. Neurosci. 8:410. doi: 10.3389/fnins.2014.00410
Fazli, S., Mehnert, J., Steinbrink, J., Curio, G., Villringer, A., Müller, K.-R., et al. (2012). Enhanced performance by a hybrid NIRS-EEG brain computer interface. Neuroimage 59, 519–529. doi: 10.1016/j.neuroimage.2011.07.084
Filiz, E., and Arslan, R. B. (2020). “Design and implementation of steady state visual evoked potential based brain computer interface video game,” in 2020 IEEE 20th Mediterranean Electrotechnical Conference (MELECON) (Palermo: IEEE), 335–338. doi: 10.1109/MELECON48756.2020.9140710
Friehs, G., Penn, R. D., Park, M. C., Goldman, M., Zerris, V. A., Hochberg, L. R., et al. (2006). Initial surgical experience with an intracortical microelectrode array for brain-computer interface applications: 881. Neurosurgery 59:481. doi: 10.1227/00006123-200608000-00119
Fukuma, R., Yanagisawa, T., Saitoh, Y., Hosomi, K., Kishima, H., Shimizu, T., et al. (2016). Real-time control of a neuroprosthetic hand by magnetoencephalographic signals from paralysed patients. Sci. Rep. 6, 1–14. doi: 10.1038/srep21781
Galán, F., Nuttin, M., Lew, E., Ferrez, P. W., Vanacker, G., Philips, J., et al. (2008). A brain-actuated wheelchair: asynchronous and non-invasive brain-computer interfaces for continuous control of robots. Clin. Neurophysiol. 119, 2159–2169. doi: 10.1016/j.clinph.2008.06.001
Gandhi, V., Prasad, G., Coyle, D., Behera, L., and McGinnity, T. M. (2014). EEG-based mobile robot control through an adaptive brain-robot interface. IEEE Trans. Syst. Man Cybernet. Syst. 44, 1278–1285. doi: 10.1109/TSMC.2014.2313317
Gao, Z., Wang, X., Yang, Y., Mu, C., Cai, Q., Dang, W., et al. (2019). EEG-based spatio-temporal convolutional neural network for driver fatigue evaluation. IEEE Trans. Neural Netw. Learn. Syst. 30, 2755–2763. doi: 10.1109/TNNLS.2018.2886414
Ge, S., Yang, Q., Wang, R., Lin, P., Gao, J., Leng, Y., et al. (2017). A brain-computer interface based on a few-channel EEG-fNIRS bimodal system. IEEE Access 5, 208–218. doi: 10.1109/ACCESS.2016.2637409
Gilbert, F. (2015). Self-estrangement and deep brain stimulation: ethical issues related to forced explantation. Neuroethics 8, 107–114. doi: 10.1007/s12152-014-9224-1
Gilja, V., Chestek, C. A., Diester, I., Henderson, J. M., Deisseroth, K., and Shenoy, K. V. (2011). Challenges and opportunities for next-generation intracortically based neural prostheses. IEEE Trans. Biomed. Eng. 58, 1891–1899. doi: 10.1109/TBME.2011.2107553
Goering, S., and Yuste, R. (2016). On the necessity of ethical guidelines for novel neurotechnologies. Cell 167, 882–885. doi: 10.1016/j.cell.2016.10.029
Gonçalves, S. I., De Munck, J. C., Pouwels, P. J., Schoonhoven, R., Kuijer, J. P., Maurits, N. M., et al. (2006). Correlating the alpha rhythm to bold using simultaneous EEG/fMRI: inter-subject variability. Neuroimage 30, 203–213. doi: 10.1016/j.neuroimage.2005.09.062
Grau, C., Ginhoux, R., Riera, A., Nguyen, T. L., Chauvat, H., Berg, M., et al. (2014). Conscious brain-to-brain communication in humans using non-invasive technologies. PLoS ONE 9:e105225. doi: 10.1371/journal.pone.0105225
Grienberger, C., and Konnerth, A. (2012). Imaging calcium in neurons. Neuron 73, 862–885. doi: 10.1016/j.neuron.2012.02.011
Grillner, S., Ip, N., Koch, C., Koroshetz, W., Okano, H., Polachek, M., et al. (2016). Worldwide initiatives to advance brain research. Nat. Neurosci. 19, 1118–1122. doi: 10.1038/nn.4371
Grosse-Wentrup, M., Mattia, D., and Oweiss, K. (2011). Using brain-computer interfaces to induce neural plasticity and restore function. J. Neural Eng. 8:025004. doi: 10.1088/1741-2560/8/2/025004
Guy, V., Soriani, M.-H., Bruno, M., Papadopoulo, T., Desnuelle, C., and Clerc, M. (2018). Brain computer interface with the p300 speller: usability for disabled people with amyotrophic lateral sclerosis. Ann. Phys. Rehabil. Med. 61, 5–11. doi: 10.1016/j.rehab.2017.09.004
Halder, S., Leinfelder, T., Schulz, S. M., and Kübler, A. (2019). Neural mechanisms of training an auditory event-related potential task in a brain-computer interface context. Hum. Brain Mapp. 40, 2399–2412. doi: 10.1002/hbm.24531
Hammer, E. M., Halder, S., Blankertz, B., Sannelli, C., Dickhaus, T., Kleih, S., et al. (2012). Psychological predictors of SMR-BCI performance. Biol. Psychol. 89, 80–86. doi: 10.1016/j.biopsycho.2011.09.006
Han, C.-H., Müller, K.-R., and Hwang, H.-J. (2020). Brain-switches for asynchronous brain-computer interfaces: a systematic review. Electronics 9:422. doi: 10.3390/electronics9030422
Hasson, U., Nir, Y., Levy, I., Fuhrmann, G., and Malach, R. (2004). Intersubject synchronization of cortical activity during natural vision. Science 303, 1634–1640. doi: 10.1126/science.1089506
He, B., Yang, L., Wilke, C., and Yuan, H. (2011). Electrophysiological imaging of brain activity and connectivity-challenges and opportunities. IEEE Trans. Biomed. Eng. 58, 1918–1931. doi: 10.1109/TBME.2011.2139210
He, H., and Wu, D. (2019). Transfer learning for brain-computer interfaces: a Euclidean space data alignment approach. IEEE Trans. Biomed. Eng. 67, 399–410. doi: 10.1109/TBME.2019.2913914
He, H., and Wu, D. (2020). Different set domain adaptation for brain-computer interfaces: a label alignment approach. IEEE Trans. Neural Syst. Rehabil. Eng.28, 1091–1108. doi: 10.1109/TNSRE.2020.2980299
Hinrichs, H., Scholz, M., Baum, A. K., Kam, J. W., Knight, R. T., and Heinze, H.-J. (2020). Comparison between a wireless dry electrode EEG system with a conventional wired wet electrode EEG system for clinical applications. Sci. Rep. 10, 1–14. doi: 10.1038/s41598-020-62154-0
Hobson, E. V., Fazal, S., Shaw, P. J., and McDermott, C. J. (2017). “Anything that makes life's journey better.” Exploring the use of digital technology by people living with motor neurone disease. Amyotrophic Lateral Sclerosis Frontotemporal Degeneration 18, 378–387. doi: 10.1080/21678421.2017.1288253
Hochberg, L. R. (2013). Intracortical brain-computer interfaces for the restoration of communication and mobility. Biophys. J. 104:376. doi: 10.1016/j.bpj.2012.11.2094
Honey, C. J., Sporns, O., Cammoun, L., Gigandet, X., Thiran, J.-P., Meuli, R., et al. (2009). Predicting human resting-state functional connectivity from structural connectivity. Proc. Natl. Acad. Sci. U.S.A. 106, 2035–2040. doi: 10.1073/pnas.0811168106
Honey, C. J., Thivierge, J.-P., and Sporns, O. (2010). Can structure predict function in the human brain? Neuroimage 52, 766–776. doi: 10.1016/j.neuroimage.2010.01.071
Hong, X., Lu, Z. K., Teh, I., Nasrallah, F. A., Teo, W. P., Ang, K. K., et al. (2017). Brain plasticity following MI-BCI training combined with tdcs in a randomized trial in chronic subcortical stroke subjects: a preliminary study. Sci. Rep. 7, 1–12. doi: 10.1038/s41598-017-08928-5
Horki, P., Solis-Escalante, T., Neuper, C., and Müller-Putz, G. (2011). Combined motor imagery and ssvep based BCI control of a 2 DOF artificial upper limb. Med. Biol. Eng. Comput. 49, 567–577. doi: 10.1007/s11517-011-0750-2
Huang, H., Xie, Q., Pan, J., He, Y., Wen, Z., Yu, R., et al. (2019). An EEG-based brain computer interface for emotion rECOGnition and its application in patients with disorder of consciousness. IEEE Trans. Affect. Comput. doi: 10.1109/TAFFC.2019.2901456
Ienca, M., and Haselager, P. (2016). Hacking the brain: brain-computer interfacing technology and the ethics of neurosecurity. Ethics Inform. Technol. 18, 117–129. doi: 10.1007/s10676-016-9398-9
Illes, J., and Bird, S. J. (2006). Neuroethics: a modern context for ethics in neuroscience. Trends Neurosci. 29, 511–517. doi: 10.1016/j.tins.2006.07.002
Iturrate, I., Antelis, J. M., Kubler, A., and Minguez, J. (2009). A noninvasive brain-actuated wheelchair based on a p300 neurophysiological protocol and automated navigation. IEEE Trans. Robot. 25, 614–627. doi: 10.1109/TRO.2009.2020347
Jayaram, V., Alamgir, M., Altun, Y., Scholkopf, B., and Grosse-Wentrup, M. (2016). Transfer learning in brain-computer interfaces. IEEE Comput. Intell. Mag. 11, 20–31. doi: 10.1109/MCI.2015.2501545
Jebari, K. (2013). Brain machine interface and human enhancement-an ethical review. Neuroethics 6, 617–625. doi: 10.1007/s12152-012-9176-2
Jensen, O., Bahramisharif, A., Oostenveld, R., Klanke, S., Hadjipapas, A., Okazaki, Y. O., et al. (2011). Using brain-computer interfaces and brain-state dependent stimulation as tools in cognitive neuroscience. Front. Psychol. 2:100. doi: 10.3389/fpsyg.2011.00100
Jiang, L., Stocco, A., Losey, D. M., Abernethy, J. A., Prat, C. S., and Rao, R. P. (2019). Brainnet: a multi-person brain-to-brain interface for direct collaboration between brains. Sci. Rep. 9, 1–11. doi: 10.1038/s41598-019-41895-7
Jin, J., Chen, Z., Xu, R., Miao, Y., yu Wang, X., and Jung, T.-P. (2020). Developing a novel tactile p300 brain-computer interface with a cheeks-stim paradigm. IEEE Trans. Biomed. Eng. 67, 2585–2593. doi: 10.1109/TBME.2020.2965178
Johnson, N., Carey, J., Edelman, B., Doud, A., Grande, A., Lakshminarayan, K., et al. (2018). Combined rtms and virtual reality brain-computer interface training for motor recovery after stroke. J. Neural Eng. 15:016009. doi: 10.1088/1741-2552/aa8ce3
Jones, S. R., and Sliva, D. D. (2020). Is alpha asymmetry a byproduct or cause of spatial attention? New evidence alpha neurofeedback controls measures of spatial attention. Neuron 105, 404–406. doi: 10.1016/j.neuron.2019.12.033
Jotterand, F., and Giordano, J. (2011). Transcranial magnetic stimulation, deep brain stimulation and personal identity: ethical questions, and neuroethical approaches for medical practice. Int. Rev. Psychiatry 23, 476–485. doi: 10.3109/09540261.2011.616189
Juliano, J. M., Spicer, R. P., Vourvopoulos, A., Lefebvre, S., Jann, K., Ard, T., et al. (2020). Embodiment is related to better performance on a brain-computer interface in immersive virtual reality: a pilot study. Sensors 20:1204. doi: 10.3390/s20041204
Kaas, A. L., Goebel, R., Valente, G., and Sorger, B. (2019). Topographic somatosensory imagery for real-time fMRI brain-computer interfacing. Front. Hum. Neurosci. 13:427. doi: 10.3389/fnhum.2019.00427
Kaiju, T., Yokota, M., Watanabe, K., Inoue, M., Ando, H., Takahashi, K., et al. (2017). High spatiotemporal resolution ECOG recording of somatosensory evoked potentials with flexible micro-electrode arrays. Front. Neural Circ. 11:20. doi: 10.3389/fncir.2017.00020
Kamousi, B., Liu, Z., and He, B. (2005). Classification of motor imagery tasks for brain-computer interface applications by means of two equivalent dipoles analysis. IEEE Trans. Neural Syst. Rehabil. Eng. 13, 166–171. doi: 10.1109/TNSRE.2005.847386
Kasahara, K., DaSalla, C. S., Honda, M., and Hanakawa, T. (2015). Neuroanatomical correlates of brain-computer interface performance. Neuroimage 110, 95–100. doi: 10.1016/j.neuroimage.2015.01.055
Käthner, I., Wriessnegger, S. C., Müller-Putz, G. R., Kübler, A., and Halder, S. (2014). Effects of mental workload and fatigue on the p300, alpha and theta band power during operation of an ERP (p300) brain-computer interface. Biol. Psychol. 102, 118–129. doi: 10.1016/j.biopsycho.2014.07.014
Kaufmann, T., Vögele, C., Sütterlin, S., Lukito, S., and Kübler, A. (2012). Effects of resting heart rate variability on performance in the p300 brain-computer interface. Int. J. Psychophysiol. 83, 336–341. doi: 10.1016/j.ijpsycho.2011.11.018
Kauhanen, L., Nykopp, T., Lehtonen, J., Jylanki, P., Heikkonen, J., Rantanen, P., et al. (2006). EEG and MEG brain-computer interface for tetraplegic patients. IEEE Trans. Neural Syst. Rehabil. Eng. 14, 190–193. doi: 10.1109/TNSRE.2006.875546
Keefer, E. W., Botterman, B. R., Romero, M. I., Rossi, A. F., and Gross, G. W. (2008). Carbon nanotube coating improves neuronal recordings. Nat. Nanotechnol. 3, 434–439. doi: 10.1038/nnano.2008.174
Khalaf, A., Sejdic, E., and Akcakaya, M. (2019). A novel motor imagery hybrid brain computer interface using EEG and functional transcranial doppler ultrasound. J. Neurosci. Methods 313, 44–53. doi: 10.1016/j.jneumeth.2018.11.017
Khodagholy, D., Doublet, T., Quilichini, P., Gurfinkel, M., Leleux, P., Ghestem, A., et al. (2013). In vivo recordings of brain activity using organic transistors. Nat. Commun. 4, 1–7. doi: 10.1038/ncomms2573
Kleih, S. C., and Kübler, A. (2013). Empathy, motivation, and p300 BCI performance. Front. Hum. Neurosci. 7:642. doi: 10.3389/fnhum.2013.00642
Kleinschmidt, A., Sterzer, P., and Rees, G. (2012). Variability of perceptual multistability: from brain state to individual trait. Philos. Trans. R. Soc. B Biol. Sci. 367, 988–1000. doi: 10.1098/rstb.2011.0367
Krusienski, D. J., Grosse-Wentrup, M., Galán, F., Coyle, D., Miller, K. J., Forney, E., et al. (2011). Critical issues in state-of-the-art brain-computer interface signal processing. J. Neural Eng. 8:025002. doi: 10.1088/1741-2560/8/2/025002
Kruskal, P. B., Jiang, Z., Gao, T., and Lieber, C. M. (2015). Beyond the patch clamp: nanotechnologies for intracellular recording. Neuron 86, 21–24. doi: 10.1016/j.neuron.2015.01.004
Kwon, O.-Y., Lee, M.-H., Guan, C., and Lee, S.-W. (2019). Subject-independent brain-computer interfaces based on deep convolutional neural networks. IEEE Trans. Neural Netw. Learn. Syst. 31, 3839–3852. doi: 10.1109/TNNLS.2019.2946869
LaFleur, K., Cassady, K., Doud, A., Shades, K., Rogin, E., and He, B. (2013). Quadcopter control in three-dimensional space using a noninvasive motor imagery-based brain-computer interface. J. Neural Eng. 10:046003. doi: 10.1088/1741-2560/10/4/046003
Lajoie, G., Krouchev, N. I., Kalaska, J. F., Fairhall, A. L., and Fetz, E. E. (2017). Correlation-based model of artificially induced plasticity in motor cortex by a bidirectional brain-computer interface. PLoS Comput. Biol. 13:e1005343. doi: 10.1371/journal.pcbi.1005343
Leamy, D. J., Kocijan, J., Domijan, K., Duffin, J., Roche, R. A., Commins, S., et al. (2014). An exploration of EEG features during recovery following stroke-implications for BCI-mediated neurorehabilitation therapy. J. Neuroeng. Rehabil. 11:9. doi: 10.1186/1743-0003-11-9
Lebedev, M. A., and Nicolelis, M. A. (2017). Brain-machine interfaces: from basic science to neuroprostheses and neurorehabilitation. Physiol. Rev. 97, 767–837. doi: 10.1152/physrev.00027.2016
Lécuyer, A., Lotte, F., Reilly, R. B., Leeb, R., Hirose, M., and Slater, M. (2008). Brain-computer interfaces, virtual reality, and videogames. Computer 41, 66–72. doi: 10.1109/MC.2008.410
Liao, L.-D., Lin, C.-T., McDowell, K., Wickenden, A. E., Gramann, K., Jung, T.-P., et al. (2012). Biosensor technologies for augmented brain-computer interfaces in the next decades. Proc. IEEE 100, 1553–1566. doi: 10.1109/JPROC.2012.2184829
Lin, C.-T., Chen, Y.-C., Huang, T.-Y., Chiu, T.-T., Ko, L.-W., Liang, S.-F., et al. (2008). Development of wireless brain computer interface with embedded multitask scheduling and its application on real-time driver's drowsiness detection and warning. IEEE Trans. Biomed. Eng. 55, 1582–1591. doi: 10.1109/TBME.2008.918566
Lina, J.-M., Chowdhury, R., Lemay, E., Kobayashi, E., and Grova, C. (2012). Wavelet-based localization of oscillatory sources from magnetoencephalography data. IEEE Trans. Biomed. Eng. 61, 2350–2364. doi: 10.1109/TBME.2012.2189883
Lotte, F., Bougrain, L., Cichocki, A., Clerc, M., Congedo, M., Rakotomamonjy, A., et al. (2018). A review of classification algorithms for EEG-based brain-computer interfaces: a 10 year update. J. Neural Eng. 15:031005. doi: 10.1088/1741-2552/aab2f2
Lotte, F., Congedo, M., Lécuyer, A., Lamarche, F., and Arnaldi, B. (2007). A review of classification algorithms for EEG-based brain-computer interfaces. J. Neural Eng. 4:R1. doi: 10.1088/1741-2560/4/2/R01
Lotte, F., and Guan, C. (2010). Regularizing common spatial patterns to improve BCI designs: unified theory and new algorithms. IEEE Trans. Biomed. Eng. 58, 355–362. doi: 10.1109/TBME.2010.2082539
Lu, J., Mamun, K. A., and Chau, T. (2015). Pattern classification to optimize the performance of transcranial doppler ultrasonography-based brain machine interface. Pattern Recogn. Lett. 66, 135–143. doi: 10.1016/j.patrec.2015.07.020
Luu, T. P., He, Y., Brown, S., Nakagome, S., and Contreras-Vidal, J. L. (2016). Gait adaptation to visual kinematic perturbations using a real-time closed-loop brain-computer interface to a virtual reality avatar. J. Neural Eng. 13:036006. doi: 10.1088/1741-2560/13/3/036006
Lystad, R. P., and Pollard, H. (2009). Functional neuroimaging: a brief overview and feasibility for use in chiropractic research. J. Can. Chiropract. Assoc. 53:59.
Mahjoory, K., Nikulin, V. V., Botrel, L., Linkenkaer-Hansen, K., Fato, M. M., and Haufe, S. (2017). Consistency of EEG source localization and connectivity estimates. Neuroimage 152, 590–601. doi: 10.1016/j.neuroimage.2017.02.076
Mak, J. N., and Wolpaw, J. R. (2009). Clinical applications of brain-computer interfaces: current state and future prospects. IEEE Rev. Biomed. Eng. 2, 187–199. doi: 10.1109/RBME.2009.2035356
Mantini, D., Perrucci, M. G., Del Gratta, C., Romani, G. L., and Corbetta, M. (2007). Electrophysiological signatures of resting state networks in the human brain. Proc. Natl. Acad. Sci. U.S.A. 104, 13170–13175. doi: 10.1073/pnas.0700668104
Marathe, A. R., Lawhern, V. J., Wu, D., Slayback, D., and Lance, B. J. (2015). Improved neural signal classification in a rapid serial visual presentation task using active learning. IEEE Trans. Neural Syst. Rehabil. Eng. 24, 333–343. doi: 10.1109/TNSRE.2015.2502323
Marchesotti, S., Bassolino, M., Serino, A., Bleuler, H., and Blanke, O. (2016). Quantifying the role of motor imagery in brain-machine interfaces. Sci. Rep. 6:24076. doi: 10.1038/srep24076
Marchesotti, S., Martuzzi, R., Schurger, A., Blefari, M. L., del Millán, J. R., Bleuler, H., et al. (2017). Cortical and subcortical mechanisms of brain-machine interfaces. Hum. Brain Mapp. 38, 2971–2989. doi: 10.1002/hbm.23566
Marini, F., Lee, C., Wagner, J., Makeig, S., and Gola, M. (2019). A comparative evaluation of signal quality between a research-grade and a wireless dry-electrode mobile EEG system. J. Neural Eng. 16:054001. doi: 10.1088/1741-2552/ab21f2
Martin, M. V., Cho, V., and Aversano, G. (2016). Detection of subconscious face rECOGnition using consumer-grade brain-computer interfaces. ACM Trans. Appl. Percept. 14, 1–20. doi: 10.1145/2955097
Maslen, H., Pugh, J., and Savulescu, J. (2015). The ethics of deep brain stimulation for the treatment of anorexia nervosa. Neuroethics 8, 215–230. doi: 10.1007/s12152-015-9240-9
Matthews, F., Pearlmutter, B. A., Wards, T. E., Soraghan, C., and Markham, C. (2007). Hemodynamics for brain-computer interfaces. IEEE Signal Process. Mag. 25, 87–94. doi: 10.1109/MSP.2008.4408445
McCane, L. M., Heckman, S. M., McFarland, D. J., Townsend, G., Mak, J. N., Sellers, E. W., et al. (2015). P300-based brain-computer interface (BCI) event-related potentials (ERPs): people with amyotrophic lateral sclerosis (ALS) vs. age-matched controls. Clin. Neurophysiol. 126, 2124–2131. doi: 10.1016/j.clinph.2015.01.013
McClinton, W., Garcia, S., and Andujar, M. (2019). “An immersive brain painting: the effects of brain painting in a virtual reality environment,” in International Conference on Human-Computer Interaction (Copenhagen: Springer), 436–445. doi: 10.1007/978-3-030-22419-6_31
McCullagh, P., Lightbody, G., Zygierewicz, J., and Kernohan, W. G. (2014). Ethical challenges associated with the development and deployment of brain computer interface technology. Neuroethics 7, 109–122. doi: 10.1007/s12152-013-9188-6
McFarland, D. J., Anderson, C. W., Muller, K.-R., Schlogl, A., and Krusienski, D. J. (2006). Bci meeting 2005-workshop on BCI signal processing: feature extraction and translation. IEEE Trans. Neural Syst. Rehabil. Eng. 14, 135–138. doi: 10.1109/TNSRE.2006.875637
McMillan, G. R., Calhoun, G., Middendorf, M., Schnurer, J., Ingle, D., and Nasman, V. (1995). “Direct brain interface utilizing self-regulation of steady-state visual evoked response (SSVER),” in Proc. RESNA 95 Annual Conf . (Vancouver, BC), 693–695.
Megan, T. D., Cohen, J. D., Lee, R. F., Norman, K. A., and Turk-Browne, N. B. (2015). Closed-loop training of attention with real-time brain imaging. Nat. Neurosci. 18, 470–475. doi: 10.1038/nn.3940
Mellinger, J., Schalk, G., Braun, C., Preissl, H., Rosenstiel, W., Birbaumer, N., et al. (2007). An MEG-based brain-computer interface (BCI). Neuroimage 36, 581–593. doi: 10.1016/j.neuroimage.2007.03.019
Menon, C., De Negueruela, C., Millán, J. d. R., Tonet, O., Carpi, F., Broschart, M., et al. (2009). Prospects of brain-machine interfaces for space system control. Acta Astronaut. 64, 448–456. doi: 10.1016/j.actaastro.2008.09.008
Mestais, C. S., Charvet, G., Sauter-Starace, F., Foerster, M., Ratel, D., and Benabid, A. L. (2014). Wimagine: wireless 64-channel ECOG recording implant for long term clinical applications. IEEE Trans. Neural Syst. Rehabil. Eng. 23, 10–21. doi: 10.1109/TNSRE.2014.2333541
Milan, J. D. R., and Carmena, J. M. (2010). Invasive or noninvasive: understanding brain-machine interface technology [conversations in BME]. IEEE Eng. Med. Biol. Mag. 29, 16–22. doi: 10.1109/MEMB.2009.935475
Millan, J. R., Renkens, F., Mourino, J., and Gerstner, W. (2004). Noninvasive brain-actuated control of a mobile robot by human EEG. IEEE Trans. Biomed. Eng. 51, 1026–1033. doi: 10.1109/TBME.2004.827086
Min, B.-K., Hämäläinen, M. S., and Pantazis, D. (2020). New cognitive neurotechnology facilitates studies of cortical-subcortical interactions. Trends Biotechnol. 38, 952–962. doi: 10.1016/j.tibtech.2020.03.003
Min, B.-K., Marzelli, M. J., and Yoo, S.-S. (2010). Neuroimaging-based approaches in the brain-computer interface. Trends Biotechnol. 28, 552–560. doi: 10.1016/j.tibtech.2010.08.002
Mota, A. R., Duarte, L., Rodrigues, D., Martins, A., Machado, A., Vaz, F., et al. (2013). Development of a quasi-dry electrode for EEG recording. Sensors Actuat A Phys. 199, 310–317. doi: 10.1016/j.sna.2013.06.013
Mudgal, S. K., Sharma, S. S. K., Chaturvedi, I., and Sharma, A. (2020). Brain computer interface advancement in neurosciences: applications and issues. Interdiscipl. Neurosurg. 20:100694. doi: 10.1016/j.inat.2020.100694
Müller, J. L., Röder, C. H., Schuierer, G., and Klein, H. (2002). Motor-induced brain activation in cortical, subcortical and cerebellar regions in schizophrenic inpatients. A whole brain fMRI fingertapping study. Prog. Neuropsychopharmacol. Biol. Psychiatry 26, 421–426. doi: 10.1016/S0278-5846(01)00271-8
Muller-Putz, G. R., Scherer, R., Neuper, C., and Pfurtscheller, G. (2006). Steady-state somatosensory evoked potentials: suitable brain signals for brain-computer interfaces? IEEE Trans. Neural Syst. Rehabil. Eng. 14, 30–37. doi: 10.1109/TNSRE.2005.863842
Murovec, N., Heilinger, A., Xu, R., Ortner, R., Spataro, R., La Bella, V., et al. (2020). Effects of a vibro-tactile p300 based brain-computer interface on the coma recovery scale-revised in patients with disorders of consciousness. Front. Neurosci. 14:294. doi: 10.3389/fnins.2020.00294
Nagel, S., and Spüler, M. (2019). World's fastest brain-computer interface: combining EEG2code with deep learning. PLoS ONE 14:e0221909. doi: 10.1371/journal.pone.0221909
Nakazawa, E., Yamamoto, K., Tachibana, K., Toda, S., Takimoto, Y., and Akabayashi, A. (2016). Ethics of decoded neurofeedback in clinical research, treatment, and moral enhancement. AJOB Neurosci. 7, 110–117. doi: 10.1080/21507740.2016.1172134
Naseer, N., and Hong, K.-S. (2015). fNIRS-based brain-computer interfaces: a review. Front. Hum. Neurosci. 9:3. doi: 10.3389/fnhum.2015.00003
Nicolas-Alonso, L. F., and Gomez-Gil, J. (2012). Brain computer interfaces, a review. Sensors 12, 1211–1279. doi: 10.3390/s120201211
Nicolelis, M. A., and Lebedev, M. A. (2009). Principles of neural ensemble physiology underlying the operation of brain-machine interfaces. Nat. Rev. Neurosci. 10, 530–540. doi: 10.1038/nrn2653
Nijboer, F., Birbaumer, N., and Kubler, A. (2010). The influence of psychological state and motivation on brain-computer interface performance in patients with amyotrophic lateral sclerosis-A longitudinal study. Front. Neurosci. 4:55. doi: 10.3389/fnins.2010.00055
Nijholt, A., and Poel, M. (2016). “Multi-brain BCI: Characteristics and social interactions,” in International Conference on Augmented Cognition (Las Vegas, NV: Springer), 79–90. doi: 10.1007/978-3-319-39955-3_8
Norton, J. J., Lee, D. S., Lee, J. W., Lee, W., Kwon, O., Won, P., et al. (2015). Soft, curved electrode systems capable of integration on the auricle as a persistent brain-computer interface. Proc. Natl. Acad. Sci. U.S.A. 112, 3920–3925. doi: 10.1073/pnas.1424875112
Orsborn, A. L., Moorman, H. G., Overduin, S. A., Shanechi, M. M., Dimitrov, D. F., and Carmena, J. M. (2014). Closed-loop decoder adaptation shapes neural plasticity for skillful neuroprosthetic control. Neuron 82, 1380–1393. doi: 10.1016/j.neuron.2014.04.048
Ortiz, F., González, J., Montes, A., González, N., and Peña, A. (2016). Induction of emotional states in people with disabilities through film clips using brain computer interfaces. IEEE Latin Am. Trans. 14, 563–568. doi: 10.1109/TLA.2016.7437193
Oxley, T. J., Opie, N. L., John, S. E., Rind, G. S., Ronayne, S. M., Burkitt, A. N., et al. (2017). “A minimally invasive endovascular stent-electrode array for chronic recordings of cortical neural activity,” in Brain-Computer Interface Research, eds C. Guger, B. Allison, and M. Lebedev (Cham: Springer), 55–63. doi: 10.1007/978-3-319-64373-1_6
Oxley, T. J., Opie, N. L., John, S. E., Rind, G. S., Ronayne, S. M., Wheeler, T. L., et al. (2016). Minimally invasive endovascular stent-electrode array for high-fidelity, chronic recordings of cortical neural activity. Nat. Biotechnol. 34, 320–327. doi: 10.1038/nbt.3428
Oxley, T. J., Yoo, P. E., Rind, G. S., Ronayne, S. M., Lee, C. S., Bird, C., et al. (2020). Motor neuroprosthesis implanted with neurointerventional surgery improves capacity for activities of daily living tasks in severe paralysis: first in-human experience. J. NeuroIntervent. Surg. doi: 10.1136/neurintsurg-2020-016862
Pahwa, M., Kusner, M., Hacker, C. D., Bundy, D. T., Weinberger, K. Q., and Leuthardt, E. C. (2015). Optimizing the detection of wakeful and sleep-like states for future electrocorticographic brain computer interface applications. PLoS ONE 10:e0142947. doi: 10.1371/journal.pone.0142947
Pais-Vieira, M., Lebedev, M., Kunicki, C., Wang, J., and Nicolelis, M. A. (2013). A brain-to-brain interface for real-time sharing of sensorimotor information. Sci. Rep. 3:1319. doi: 10.1038/srep01319
Pandarinath, C., Nuyujukian, P., Blabe, C. H., Sorice, B. L., Saab, J., Willett, F. R., et al. (2017). High performance communication by people with paralysis using an intracortical brain-computer interface. eLife 6:e18554. doi: 10.7554/eLife.18554
Park, W., Kwon, G. H., Kim, Y.-H., Lee, J.-H., and Kim, L. (2016). EEG response varies with lesion location in patients with chronic stroke. J. Neuroeng. Rehabil. 13, 1–10. doi: 10.1186/s12984-016-0120-2
Perdikis, S., Tonin, L., and Millan, J. d. R. (2017). Brain racers. IEEE Spectrum 54, 44–51. doi: 10.1109/MSPEC.2017.8012239
Petrov, Y., Nador, J., Hughes, C., Tran, S., Yavuzcetin, O., and Sridhar, S. (2014). Ultra-dense EEG sampling results in two-fold increase of functional brain information. Neuroimage 90, 140–145. doi: 10.1016/j.neuroimage.2013.12.041
Pfurtscheller, G., Guger, C., Müller, G., Krausz, G., and Neuper, C. (2000). Brain oscillations control hand orthosis in a tetraplegic. Neurosci. Lett. 292, 211–214. doi: 10.1016/S0304-3940(00)01471-3
Pfurtscheller, G., Solis-Escalante, T., Ortner, R., Linortner, P., and Muller-Putz, G. R. (2010). Self-paced operation of an ssvep-based orthosis with and without an imagery-based “brain switch:” a feasibility study towards a hybrid BCI. IEEE Trans. Neural Syst. Rehabil. Eng. 18, 409–414. doi: 10.1109/TNSRE.2010.2040837
Piastra, M. C., Nüßing, A., Vorwerk, J., Clerc, M., Engwer, C., and Wolters, C. H. (2020). A comprehensive study on electroencephalography and magnetoencephalography sensitivity to cortical and subcortical sources. Hum. Brain Mapp. doi: 10.1002/hbm.25272
Picard, R. W. (2000). Affective Computing. Cambridge, MA; London: MIT Press. doi: 10.7551/mitpress/1140.001.0001
Piho, L., and Tjahjadi, T. (2018). A mutual information based adaptive windowing of informative EEG for emotion recognition. IEEE Trans. Affect. Comput. 11, 722–735. doi: 10.1109/TAFFC.2018.2840973
Putze, F., Vourvopoulos, A., Lécuyer, A., Krusienski, D., i Badia, S. B., Mullen, T., et al. (2020). Brain-computer interfaces and augmented/virtual reality. Front. Hum. Neurosci. 14:144. doi: 10.3389/fnhum.2020.00144
Qin, L., Ding, L., and He, B. (2004). Motor imagery classification by means of source analysis for brain-computer interface applications. J. Neural Eng. 1:135. doi: 10.1088/1741-2560/1/3/002
Ramoser, H., Muller-Gerking, J., and Pfurtscheller, G. (2000). Optimal spatial filtering of single trial EEG during imagined hand movement. IEEE Trans. Rehabil. Eng. 8, 441–446. doi: 10.1109/86.895946
Ramos-Murguialday, A., Broetz, D., Rea, M., Läer, L., Yilmaz, Ö., Brasil, F. L., et al. (2013). Brain-machine interface in chronic stroke rehabilitation: a controlled study. Ann. Neurol. 74, 100–108. doi: 10.1002/ana.23879
Rao, R. P., Stocco, A., Bryan, M., Sarma, D., Youngquist, T. M., Wu, J., et al. (2014). A direct brain-to-brain interface in humans. PLoS ONE 9:e111332. doi: 10.1371/journal.pone.0111332
Rashid, M., Sulaiman, N. P. P, Abdul Majeed, A., Musa, R. M., Ab Nasir, A. F., et al. (2020). Current status, challenges, and possible solutions of EEG-based brain-computer interface: a comprehensive review. Front. Neurorobot. 14:25. doi: 10.3389/fnbot.2020.00025
Ray, A. M., Sitaram, R., Rana, M., Pasqualotto, E., Buyukturkoglu, K., Guan, C., et al. (2015). A subject-independent pattern-based brain-computer interface. Front. Behav. Neurosci. 9:269. doi: 10.3389/fnbeh.2015.00269
Resendez, S. L., Jennings, J. H., Ung, R. L., Namboodiri, V. M. K., Zhou, Z. C., Otis, J. M., et al. (2016). Visualization of cortical, subcortical and deep brain neural circuit dynamics during naturalistic mammalian behavior with head-mounted microscopes and chronically implanted lenses. Nat. Protoc. 11:566. doi: 10.1038/nprot.2016.021
Rezazadeh Sereshkeh, A., Yousefi, R., Wong, A. T., Rudzicz, F., and Chau, T. (2019). Development of a ternary hybrid fNIRS-EEG brain-computer interface based on imagined speech. Brain Comput. Interfaces 6, 128–140. doi: 10.1080/2326263X.2019.1698928
Romero-Laiseca, M. A., Delisle-Rodriguez, D., Cardoso, V., Gurve, D., Loterio, F., Nascimento, J. H. P., et al. (2020). A low-cost lower-limb brain-machine interface triggered by pedaling motor imagery for post-stroke patients rehabilitation. IEEE Trans. Neural Syst. Rehabil. Eng. 28, 988–996. doi: 10.1109/TNSRE.2020.2974056
Rosenboom, D. (2014). Active imaginative listening–A neuromusical critique. Front. Neurosci. 8:251. doi: 10.3389/fnins.2014.00251
Rosenfeld, J. V., and Wong, Y. T. (2017). Neurobionics and the brain-computer interface: current applications and future horizons. Med. J. Australia 206, 363–368. doi: 10.5694/mja16.01011
Roth, B. L. (2016). Dreadds for neuroscientists. Neuron 89, 683–694. doi: 10.1016/j.neuron.2016.01.040
Saeedi, S., Chavarriaga, R., and Millán, J. d. R. (2016). Long-term stable control of motor-imagery BCI by a locked-in user through adaptive assistance. IEEE Trans. Neural Syst. Rehabil. Eng. 25, 380–391. doi: 10.1109/TNSRE.2016.2645681
Saha, S., Ahmed, K. I., Mostafa, R., Khandoker, A. H., and Hadjileontiadis, L. (2017). Enhanced inter-subject brain computer interface with associative sensorimotor oscillations. Healthcare Technol. Lett. 4, 39–43. doi: 10.1049/htl.2016.0073
Saha, S., Ahmed, K. I. U., Mostafa, R., Hadjileontiadis, L., and Khandoker, A. (2018). Evidence of variabilities in EEG dynamics during motor imagery-based multiclass brain-computer interface. IEEE Trans. Neural Syst. Rehabil. Eng. 26, 371–382. doi: 10.1109/TNSRE.2017.2778178
Saha, S., and Baumert, M. (2020). Intra-and inter-subject variability in EEG-based sensorimotor brain computer interface: a review. Front. Comput. Neurosci. 13:87. doi: 10.3389/fncom.2019.00087
Saha, S., Hossain, M., Ahmed, K. I. U., Mostafa, R., Hadjileontiadis, L. J., Khandoker, A. H., et al. (2019a). Wavelet entropy-based inter-subject associative cortical source localization for sensorimotor BCI. Front. Neuroinform. 13:47. doi: 10.3389/fninf.2019.00047
Saha, S., Mamun, K. A., Ahmed, K., Mostafa, R., Naik, G. R., Khandoker, A., et al. (2019b). Progress in brain computer interfaces: challenges and trends. arXiv preprint arXiv:1901.03442.
Samek, W., Meinecke, F. C., and Müller, K.-R. (2013). Transferring subspaces between subjects in brain-computer interfacing. IEEE Trans. Biomed. Eng. 60, 2289–2298. doi: 10.1109/TBME.2013.2253608
Sand, D., Peremen, Z., Haor, D., Arkadir, D., Bergman, H., and Geva, A. (2017). Optimization of deep brain stimulation in stn among patients with Parkinson's disease using a novel EEG-based tool. Brain Stimulat. 10:510. doi: 10.1016/j.brs.2017.01.490
Sannelli, C., Vidaurre, C., Müller, K.-R., and Blankertz, B. (2016). Ensembles of adaptive spatial filters increase BCI performance: an online evaluation. J. Neural Eng. 13:046003. doi: 10.1088/1741-2560/13/4/046003
Sauter-Starce, F., Ratel, D., Cretallaz, C., Foerster, M., Lambert, A., Gaude, C., et al. (2019). Long-term sheep implantation of wimagine ®, a wireless 64-channels electrocorticogram recorder. Front. Neurosci. 13:847. doi: 10.3389/fnins.2019.00847
Schalk, G. (2010). Can electrocorticography (ECOG) support robust and powerful brain-computer interfaces? Front. Neuroeng. 3:9. doi: 10.3389/fneng.2010.00009
Schaworonkow, N., Triesch, J., Ziemann, U., and Zrenner, C. (2019). EEG-triggered tms reveals stronger brain state-dependent modulation of motor evoked potentials at weaker stimulation intensities. Brain Stimulat. 12, 110–118. doi: 10.1016/j.brs.2018.09.009
Schicktanz, S., Amelung, T., and Rieger, J. W. (2015). Qualitative assessment of patients' attitudes and expectations toward BCIs and implications for future technology development. Front. Syst. Neurosci. 9:64. doi: 10.3389/fnsys.2015.00064
Scholkmann, F., Kleiser, S., Metz, A. J., Zimmermann, R., Pavia, J. M., Wolf, U., et al. (2014). A review on continuous wave functional near-infrared spectroscopy and imaging instrumentation and methodology. Neuroimage 85, 6–27. doi: 10.1016/j.neuroimage.2013.05.004
Schreuder, M., Blankertz, B., and Tangermann, M. (2010). A new auditory multi-class brain-computer interface paradigm: spatial hearing as an informative cue. PLoS ONE 5:e9813. doi: 10.1371/journal.pone.0009813
Schwartz, A. B., Cui, X. T., Weber, D. J., and Moran, D. W. (2006). Brain-controlled interfaces: movement restoration with neural prosthetics. Neuron 52, 205–220. doi: 10.1016/j.neuron.2006.09.019
Sekhavat, Y. A. (2020). Battle of minds: a new interaction approach in BCI games through competitive reinforcement. Multimedia Tools Appl. 79, 3449–3464. doi: 10.1007/s11042-019-07963-w
Seo, D., Neely, R. M., Shen, K., Singhal, U., Alon, E., Rabaey, J. M., et al. (2016). Wireless recording in the peripheral nervous system with ultrasonic neural dust. Neuron 91, 529–539. doi: 10.1016/j.neuron.2016.06.034
Shahriari, Y., Vaughan, T. M., McCane, L., Allison, B. Z., Wolpaw, J. R., and Krusienski, D. J. (2019). An exploration of BCI performance variations in people with amyotrophic lateral sclerosis using longitudinal EEG data. J. Neural Eng. 16:056031. doi: 10.1088/1741-2552/ab22ea
Shibata, K., Watanabe, T., Sasaki, Y., and Kawato, M. (2011). Perceptual learning incepted by decoded fMRI neurofeedback without stimulus presentation. Science 334, 1413–1415. doi: 10.1126/science.1212003
Shih, J. J., Krusienski, D. J., and Wolpaw, J. R. (2012). “Brain-computer interfaces in medicine,” in Mayo Clinic Proceedings (Elsevier), 268–279. doi: 10.1016/j.mayocp.2011.12.008
Singh, A. K., Wang, Y.-K., King, J.-T., and Lin, C.-T. (2020). Extended interaction with a BCI video game changes resting-state brain activity. IEEE Trans. Cogn. Dev. Syst. 12, 809–823. doi: 10.1109/TCDS.2020.2985102
Sitaram, R., Ros, T., Stoeckel, L., Haller, S., Scharnowski, F., Lewis-Peacock, J., et al. (2017). Closed-loop brain training: the science of neurofeedback. Nat. Rev. Neurosci. 18, 86–100. doi: 10.1038/nrn.2016.164
Sitaram, R., Weiskopf, N., Caria, A., Veit, R., Erb, M., and Birbaumer, N. (2007). fMRI brain-computer interfaces. IEEE Signal Process. Mag. 25, 95–106. doi: 10.1109/MSP.2008.4408446
Škola, F., Tinková, S., and Liarokapis, F. (2019). Progressive training for motor imagery brain-computer interfaces using gamification and virtual reality embodiment. Front. Hum. Neurosci. 13:329. doi: 10.3389/fnhum.2019.00329
Song, M., and Kim, J. (2019). A paradigm to enhance motor imagery using rubber hand illusion induced by visuo-tactile stimulus. IEEE Trans. Neural Syst. Rehabil. Eng. 27, 477–486. doi: 10.1109/TNSRE.2019.2895029
Song, T., Zheng, W., Song, P., and Cui, Z. (2018). EEG emotion recognition using dynamical graph convolutional neural networks. IEEE Trans. Affect. Comput. 11, 532–541. doi: 10.1109/TAFFC.2018.2817622
Spataro, R., Chella, A., Allison, B., Giardina, M., Sorbello, R., Tramonte, S., et al. (2017). Reaching and grasping a glass of water by locked-in als patients through a BCI-controlled humanoid robot. Front. Hum. Neurosci. 11:68. doi: 10.3389/fnhum.2017.00068
Sporns, O. (2013). Structure and function of complex brain networks. Dialog. Clin. Neurosci. 15:247. doi: 10.31887/DCNS.2013.15.3/osporns
Steinert, S., and Friedrich, O. (2020). Wired emotions: ethical issues of affective brain-computer interfaces. Sci. Eng. Ethics 26, 351–367. doi: 10.1007/s11948-019-00087-2
Sternson, S. M., and Roth, B. L. (2014). Chemogenetic tools to interrogate brain functions. Annu. Rev. Neurosci. 37, 387–407. doi: 10.1146/annurev-neuro-071013-014048
Stocco, A., Prat, C. S., Losey, D. M., Cronin, J. A., Wu, J., Abernethy, J. A., et al. (2015). Playing 20 questions with the mind: collaborative problem solving by humans using a brain-to-brain interface. PLoS ONE 10:e0137303. doi: 10.1371/journal.pone.0137303
Stoica, A. (2012). “Multimind: multi-brain signal fusion to exceed the power of a single brain,” in 2012 Third International Conference on Emerging Security Technologies (Lisbon: IEEE), 94–98. doi: 10.1109/EST.2012.47
Summerer, L., Izzo, D., and Rossini, L. (2009). Brain-machine interfaces for space applications-research, technological development, and opportunities. Int. Rev. Neurobiol. 86, 213–223. doi: 10.1016/S0074-7742(09)86016-9
Taylor, D. M., Tillery, S. I. H., and Schwartz, A. B. (2002). Direct cortical control of 3d neuroprosthetic devices. Science 296, 1829–1832. doi: 10.1126/science.1070291
Tidoni, E., Abu-Alqumsan, M., Leonardis, D., Kapeller, C., Fusco, G., Guger, C., et al. (2016). Local and remote cooperation with virtual and robotic agents: a p300 BCI study in healthy and people living with spinal cord injury. IEEE Trans. Neural Syst. Rehabil. Eng. 25, 1622–1632. doi: 10.1109/TNSRE.2016.2626391
Tonin, L., Bauer, F. C., and Millán, J. d. R. (2019). The role of the control framework for continuous teleoperation of a brain-machine interface-driven mobile robot. IEEE Trans. Robot. 36, 78–91. doi: 10.1109/TRO.2019.2943072
Tonin, L., and Millán, J. d. R. (2020). Noninvasive brain-machine interfaces for robotic devices. Annu. Rev. Control Robot. Auton. Syst. 4. doi: 10.1146/annurev-control-012720-093904
Toriyama, H., Ushiba, J., and Ushiyama, J. (2018). Subjective vividness of kinesthetic motor imagery is associated with the similarity in magnitude of sensorimotor event-related desynchronization between motor execution and motor imagery. Front. Hum. Neurosci. 12:295. doi: 10.3389/fnhum.2018.00295
Tremmel, C., Herff, C., Sato, T., Rechowicz, K., Yamani, Y., and Krusienski, D. J. (2019). Estimating cognitive workload in an interactive virtual reality environment using EEG. Front. Hum. Neurosci. 13:401. doi: 10.3389/fnhum.2019.00401
Vaadia, E., and Birbaumer, N. (2009). Grand challenges of brain computer interfaces in the years to come. Front. Neurosci. 3:15. doi: 10.3389/neuro.01.015.2009
van Vliet, M., Robben, A., Chumerin, N., Manyakov, N. V., Combaz, A., and Van Hulle, M. M. (2012). “Designing a brain-computer interface controlled video-game using consumer grade EEG hardware,” in 2012 ISSNIP Biosignals and Biorobotics Conference: Biosignals and Robotics for Better and Safer Living (BRC) (Manaus: IEEE), 1–6. doi: 10.1109/BRC.2012.6222186
Vansteensel, M. J., Pels, E. G., Bleichner, M. G., Branco, M. P., Denison, T., Freudenburg, Z. V., et al. (2016). Fully implanted brain-computer interface in a locked-in patient with als. N. Engl. J. Med. 375, 2060–2066. doi: 10.1056/NEJMoa1608085
Vasilyev, A., Liburkina, S., Yakovlev, L., Perepelkina, O., and Kaplan, A. (2017). Assessing motor imagery in brain-computer interface training: psychological and neurophysiological correlates. Neuropsychologia 97, 56–65. doi: 10.1016/j.neuropsychologia.2017.02.005
Vidal, J. J. (1973). Toward direct brain-computer communication. Annu. Rev. Biophys. Bioeng. 2, 157–180. doi: 10.1146/annurev.bb.02.060173.001105
Vidaurre, C., and Blankertz, B. (2010). Towards a cure for BCI illiteracy. Brain Topogr. 23, 194–198. doi: 10.1007/s10548-009-0121-6
Vourvopoulos, A., Pardo, O. M., Lefebvre, S., Neureither, M., Saldana, D., Jahng, E., et al. (2019). Effects of a brain-computer interface with virtual reality (VR) neurofeedback: a pilot study in chronic stroke patients. Front. Hum. Neurosci. 13:210. doi: 10.3389/fnhum.2019.00210
Vyas, S., Even-Chen, N., Stavisky, S. D., Ryu, S. I., Nuyujukian, P., and Shenoy, K. V. (2018). Neural population dynamics underlying motor learning transfer. Neuron 97, 1177–1186. doi: 10.1016/j.neuron.2018.01.040
Wang, H., Chang, W., and Zhang, C. (2016a). Functional brain network and multichannel analysis for the p300-based brain computer interface system of lying detection. Expert Syst. Appl. 53, 117–128. doi: 10.1016/j.eswa.2016.01.024
Wang, H., McIntosh, A. R., Kovacevic, N., Karachalios, M., and Protzner, A. B. (2016b). Age-related multiscale changes in brain signal variability in pre-task versus post-task resting-state EEG. J. Cogn. Neurosci. 28, 971–984. doi: 10.1162/jocn_a_00947
Wang, W., Collinger, J. L., Perez, M. A., Tyler-Kabara, E. C., Cohen, L. G., Birbaumer, N., et al. (2010). Neural interface technology for rehabilitation: exploiting and promoting neuroplasticity. Phys. Med. Rehabil. Clin. 21, 157–178. doi: 10.1016/j.pmr.2009.07.003
Wardman, D. L., Gandevia, S. C., and Colebatch, J. G. (2014). Cerebral, subcortical, and cerebellar activation evoked by selective stimulation of muscle and cutaneous afferents: an fMRI study. Physiol. Rep. 2:e00270. doi: 10.1002/phy2.270
Waytowich, N. R., Lawhern, V. J., Bohannon, A. W., Ball, K. R., and Lance, B. J. (2016). Spectral transfer learning using information geometry for a user-independent brain-computer interface. Front. Neurosci. 10:430. doi: 10.3389/fnins.2016.00430
Wei, C.-S., Wang, Y.-T., Lin, C.-T., and Jung, T.-P. (2018). Toward drowsiness detection using non-hair-bearing EEG-based brain-computer interfaces. IEEE Trans. Neural Syst. Rehabil. Eng. 26, 400–406. doi: 10.1109/TNSRE.2018.2790359
Wronkiewicz, M., Larson, E., and Lee, A. K. (2015). Leveraging anatomical information to improve transfer learning in brain-computer interfaces. J. Neural Eng. 12:046027. doi: 10.1088/1741-2560/12/4/046027
Wu, D., King, J.-T., Chuang, C.-H., Lin, C.-T., and Jung, T.-P. (2017a). Spatial filtering for EEG-based regression problems in brain-computer interface (BCI). IEEE Trans. Fuzzy Syst. 26, 771–781. doi: 10.1109/TFUZZ.2017.2688423
Wu, D., Lance, B. J., Lawhern, V. J., Gordon, S., Jung, T.-P., and Lin, C.-T. (2017b). EEG-based user reaction time estimation using riemannian geometry features. IEEE Trans. Neural Syst. Rehabil. Eng. 25, 2157–2168. doi: 10.1109/TNSRE.2017.2699784
Wu, D., Lawhern, V. J., Hairston, W. D., and Lance, B. J. (2016). Switching EEG headsets made easy: reducing offline calibration effort using active weighted adaptation regularization. IEEE Trans. Neural Syst. Rehabil. Eng. 24, 1125–1137. doi: 10.1109/TNSRE.2016.2544108
Wu, D., Xu, Y., and Lu, B. (2020). Transfer learning for EEG-based brain-computer interfaces: a review of progress made since 2016. IEEE Trans. Cogn. Dev. Syst. doi: 10.1109/TCDS.2020.3007453
Yang, W., Miller, J. K., Carrillo-Reid, L., Pnevmatikakis, E., Paninski, L., Yuste, R., et al. (2016). Simultaneous multi-plane imaging of neural circuits. Neuron 89, 269–284. doi: 10.1016/j.neuron.2015.12.012
Yin, E., Zeyl, T., Saab, R., Chau, T., Hu, D., and Zhou, Z. (2015). A hybrid brain-computer interface based on the fusion of p300 and SSVEP scores. IEEE Trans. Neural Syst. Rehabil. Eng. 23, 693–701. doi: 10.1109/TNSRE.2015.2403270
Yin, M., Borton, D. A., Komar, J., Agha, N., Lu, Y., Li, H., et al. (2014). Wireless neurosensor for full-spectrum electrophysiology recordings during free behavior. Neuron 84, 1170–1182. doi: 10.1016/j.neuron.2014.11.010
Yu, Y., Liu, Y., Jiang, J., Yin, E., Zhou, Z., and Hu, D. (2018). An asynchronous control paradigm based on sequential motor imagery and its application in wheelchair navigation. IEEE Trans. Neural Syst. Rehabil. Eng. 26, 2367–2375. doi: 10.1109/TNSRE.2018.2881215
Yuan, W., and Li, Z. (2018). Brain teleoperation control of a nonholonomic mobile robot using quadrupole potential function. IEEE Trans. Cogn. Dev. Syst. 11, 527–538. doi: 10.1109/TCDS.2018.2869903
Zander, T. O., Kothe, C., Welke, S., and Rötting, M. (2009). “Utilizing secondary input from passive brain-computer interfaces for enhancing human-machine interaction,” in International Conference on Foundations of Augmented Cognition (Las Vegas, NV: Springer), 759–771. doi: 10.1007/978-3-642-02812-0_86
Zhang, R., Xu, P., Chen, R., Li, F., Guo, L., Li, P., et al. (2015). Predicting inter-session performance of SMR-based brain-computer interface using the spectral entropy of resting-state EEG. Brain Topogr. 28, 680–690. doi: 10.1007/s10548-015-0429-3
Zhang, W., and Wu, D. (2020). Manifold embedded knowledge transfer for brain-computer interfaces. IEEE Trans. Neural Syst. Rehabil. Eng. 28, 1117–1127. doi: 10.1109/TNSRE.2020.2985996
Zhao, X., Chu, Y., Han, J., and Zhang, Z. (2016). SSVEP-based brain-computer interface controlled functional electrical stimulation system for upper extremity rehabilitation. IEEE Trans. Syst. Man Cybernet. Syst. 46, 947–956. doi: 10.1109/TSMC.2016.2523762
Keywords: brain computer interface, hybrid/multimodal BCI, neuroimaging techniques, neurosensors, electrical/hemodynamic brain signals, cognitive rehabilitation
Citation: Saha S, Mamun KA, Ahmed K, Mostafa R, Naik GR, Darvishi S, Khandoker AH and Baumert M (2021) Progress in Brain Computer Interface: Challenges and Opportunities. Front. Syst. Neurosci. 15:578875. doi: 10.3389/fnsys.2021.578875
Received: 01 July 2020; Accepted: 06 January 2021;
Published: 25 February 2021.
Edited by:
Alessandro E. P. Villa, Neuro-Heuristic Research Group (NHRG), SwitzerlandReviewed by:
Dongrui Wu, Huazhong University of Science and Technology, ChinaDavid R. Painter, The University of Queensland, Australia
Copyright © 2021 Saha, Mamun, Ahmed, Mostafa, Naik, Darvishi, Khandoker and Baumert. This is an open-access article distributed under the terms of the Creative Commons Attribution License (CC BY). The use, distribution or reproduction in other forums is permitted, provided the original author(s) and the copyright owner(s) are credited and that the original publication in this journal is cited, in accordance with accepted academic practice. No use, distribution or reproduction is permitted which does not comply with these terms.
*Correspondence: Simanto Saha, simanto.saha@ieee.org; Mathias Baumert, mathias.baumert@adelaide.edu.au