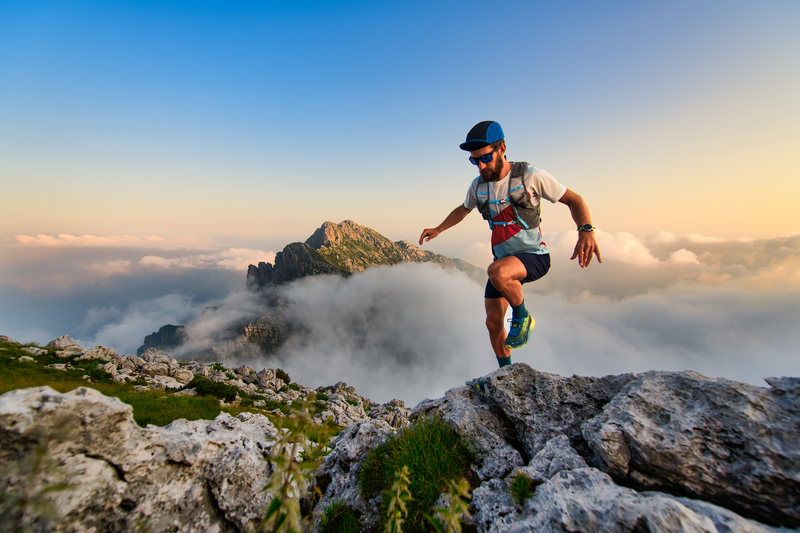
94% of researchers rate our articles as excellent or good
Learn more about the work of our research integrity team to safeguard the quality of each article we publish.
Find out more
ORIGINAL RESEARCH article
Front. Syst. Neurosci. , 14 January 2020
Volume 13 - 2019 | https://doi.org/10.3389/fnsys.2019.00087
This article is part of the Research Topic Spring Hippocampal Research Conference and Beyond View all 25 articles
White matter pathways that surround the hippocampus comprise its afferent and efferent connections, and are therefore crucial in mediating the function of the hippocampus. We recently demonstrated a role for the hippocampus in both spatial memory and olfactory identification in humans. In the current study, we focused our attention on the fimbria-fornix white matter bundle and investigated its relationship with spatial memory and olfactory identification. We administered a virtual navigation task and an olfactory identification task to 55 young healthy adults and measured the volume of the fimbria-fornix. We found that the volume of the right fimbria-fornix and its subdivisions is correlated with both navigational learning and olfactory identification in those who use hippocampus-based spatial memory strategies, and not in those who use caudate nucleus-based navigation strategies. These results are consistent with our recent finding that spatial memory and olfaction rely on similar neural networks and structures.
Many people have experienced instances where a smell spontaneously brought back a vivid memory. Therefore, it would not come as a surprise that olfaction could be closely associated with hippocampal-dependent memory, characterized by its vividness and richness in detail (Moscovitch et al., 2005). Olfaction is a vital navigation tool for many species. Many animals use olfactory cues and gradients to locate prey or pups, identify territory, or to go back to previously visited places. Although not crucial for survival, humans are also capable of finding a target location using olfactory cues (Jacobs et al., 2015).
Over the years, numerous studies have investigated spatial memory and its neural correlates. Animal and human studies have found the hippocampus to play a critical role in both spatial memory (O’Keefe and Nadel, 1978; Packard et al., 1989; McDonald and White, 1993; Pigott and Milner, 1993; Maguire et al., 1998; Bohbot et al., 2002, 2004; Hartley et al., 2003; Iaria et al., 2003; Head and Isom, 2010) and olfaction (see Suzuki et al., 2001; Lundström et al., 2011 for reviews; Kjelvik et al., 2012; Wu et al., 2012).
Up until recently, the relationship between spatial memory and olfaction had not been directly investigated. In 2018, we demonstrated the existence of an intrinsic relationship between the two processes (Dahmani et al., 2018). However, this relationship is specific to certain types of navigation but not others. There are two distinct memory systems that can be used for navigation. One of these is the hippocampal memory system, which is critical for the spatial memory strategy. This strategy involves learning precise spatial relationships between landmarks in the environment, so as to form a cognitive map, or mental representation, of one’s environment (O’Keefe and Nadel, 1978). The other memory system involves the caudate nucleus, which is critical for the stimulus-response strategy. This strategy involves learning a set of stimulus-response associations, e.g., a series of motor actions in response to a stimulus (Packard et al., 1989; McDonald and White, 1993). An example of this is route learning, where one must learn to turn left or right in response to various stimuli (e.g., turn left at the gas station). The stimulus-response strategy mainly relies on the caudate nucleus/dorsal striatum (Packard et al., 1989; McDonald and White, 1993; White and McDonald, 2002; Hartley et al., 2003; Iaria et al., 2003; Bohbot et al., 2007; Head and Isom, 2010; Konishi et al., 2013). Importantly, studies from our laboratory showed that gray matter in the hippocampus and caudate nucleus correlates with the respective use of spatial memory and stimulus-response strategies in dual-solution tasks (Iaria et al., 2003; Bohbot and Corkin, 2007; Etchamendy et al., 2012; Konishi and Bohbot, 2013; Konishi et al., 2013; Dahmani and Bohbot, 2015; Dahmani et al., 2018). Using these paradigms to identify individuals’ spontaneous navigation strategies, we showed in a recent report that olfactory identification is associated with faster learning in participants who spontaneously used a spatial memory strategy (spatial learners), but not in those who used a stimulus-response strategy (response learners; Dahmani et al., 2018). This finding was consistent with our hypothesis that olfactory identification would be related to hippocampal-dependent navigation only. In the same study, we measured hippocampal gray matter volume and found it to be positively associated with both olfactory identification and spatial learning, but not with stimulus-response learning.
In the current artice, we turn our attention to white matter. In rodents, many lesion studies have found the fimbria-fornix to be of crucial importance in spatial memory (Olton and Samuelson, 1976; Olton and Papas, 1979; Packard et al., 1989; McDonald and White, 1993, 1995; de Bruin et al., 2001), as it connects the hippocampus to most of its output regions. In contrast, fimbria-fornix lesions do not impair stimulus-response learning (Packard et al., 1989; McDonald and White, 1993; de Bruin et al., 2001). In humans, Iaria et al. (2008) found that hippocampal fractional anisotropy, which is thought to be a measure of structural white matter integrity, was positively associated with spatial learning and memory. However, whether the fimbria-fornix has a dissociable role in human spatial learning and stimulus-response learning is still unknown. Additionally, diffusion magnetic resonance imaging (MRI) measures, including fractional anisotropy, are still under debate, as their association with underlying white matter structure is not clear (Jones et al., 2013; Scholz et al., 2014). In the current study, we investigate the volume of the fimbria-fornix and use a dual-solution virtual navigation task to examine its association with spatial memory and stimulus-response learning. Similar to our previous study (Dahmani et al., 2018), we hypothesized that greater volume of the fimbria-fornix fiber system would be positively associated with navigation and olfactory identification in spatial learners, but not response learners.
We tested 60 healthy young adults between the ages of 18 and 35 (mean age = 22.9 ± 3.5; 29 women, 31 men). This represents the same dataset as reported in Dahmani et al. (2018). Participants were excluded if they were not right-handed, if they had a history of neurological or psychiatric disorders, a history of alcohol or drug abuse, or if they suffered a head trauma followed by a loss of consciousness. Three participants did not complete the navigation task (4-on-8 Virtual Maze, described below) and two participants did not undergo MRI scanning. We, therefore, had 55 participants for the navigation and olfaction analyses. The protocol was approved by the local ethics committee of the Douglas Mental Health University Institute. All subjects gave written informed consent in accordance with the Declaration of Helsinki.
To assess olfactory identification, we administered the Monell Extended Sniffin’ Sticks Identification Test (MONEX-40; Freiherr et al., 2012). This test consists of 40 felt-tip pens, each infused with an odor. The experimenter places each pen under the participant’ nose for one to 2 s and the participant inhales the odor. For each pen, participants are asked to identify the odor from four written choices shown on a screen. The pens were developed to yield at least 35% accuracy across participants (Lundström, personal communication). Thus, in the current study, pens that yielded an overall accuracy that was lower than 35% were excluded from the analysis. Two pens were excluded (warm milk and honey) and the olfactory identification score was based on 38 items. Possible reasons for poor accuracy are degradation of the odor over time, unfamiliarity due to cultural differences, or variability in performance in specific cohorts.
The 4/8 VM (Figure 1) was developed using Unreal Tournament 2003 (Epic Games, Raleigh, NC, USA). The task was adapted from a maze task originally used in rodents (Olton and Samuelson, 1976; Packard et al., 1989) and consists in a radial maze surrounded by a rich landscape with proximal and distal landmarks. The maze is made of a central platform with eight paths branching out around it. There are two parts to each learning trial:
• Part 1: Out of the eight paths, four are blocked and four are accessible. Participants are instructed to visit the accessible paths, to retrieve objects at the end of the paths (which are not visible from the central platform), and to memorize their location. When ready, participants are taken to Part 2.
• Part 2: Here, we remove the barriers to make all the paths accessible. Participants are asked to avoid the paths they just visited in order to retrieve the remaining objects. Participants can use either a spatial memory strategy, by learning the spatial relationships between landmarks in the environment and the target paths (e.g., “there is an object to the left of the tree and one to the right of the boulder”), or they can use a stimulus-response strategy, by learning a series of motor actions in response to a stimulus (e.g., “from the starting position, I have to go straight ahead and then skip the path on the right”).
Figure 1. The 4-on-8 Virtual Maze. The 4-on-8 Virtual Maze (4/8 VM) consists in an 8-arm radial maze surrounded by landmarks. In Part 1, four of the paths are blocked and four are open. Participants have to retrieve objects at the end of the open paths. In Part 2, the barriers are removed. Participants have to avoid the paths they visited in Part 1 to retrieve the remaining objects. They can learn the object locations using a spatial memory strategy (e.g., “the path is to the left of the boulder”) or a stimulus-response strategy (“From the starting position, I have to take the path straight ahead and then skip a path on the right”). Once participants learn the task to criterion, they are taken to a probe stage, where a wall is raised around the maze that hides the landmarks. People who used a spatial memory strategy during learning make more errors than people who used stimulus-response strategies, as they can no longer use landmarks to find the target paths. At the end of the task, participants have to draw a map of the maze and are administered a verbal report, which serves to determine the strategy they used as well as the number of landmarks they used (e.g., “I used the rock and the tree to find the objects”) and noticed (e.g., “I saw a mountain but I did not use it”).
A minimum of three and a maximum of eight learning trials are administered. The location of the objects does not change throughout the task except in the second trial. Participants are required to find the objects in Part 2 without making errors in at least one of the trials in order to reach the learning criterion. Once this is achieved, we administer a probe trial. Part 1 of the probe trial is the same as above. In Part 2, a wall is erected around the radial maze, blocking the landmarks from the participants’ view. Participants who used a spatial memory strategy (“spatial learners”) to memorize the object locations make more errors than participants who used a stimulus-response strategy (“response learners”), as they can no longer rely on the landmarks to find the paths containing the objects (Iaria et al., 2003). The probe trial is followed by a normal trial. Once the task is done, we conduct a verbal report, where we ask participants to describe how they solved the task. We used the verbal report to categorize participants into spatial or response learners. If participants mentioned learning the location of the pathways relative to several landmarks, they were categorized as spatial learners. If they mentioned using a counting strategy or a sequence starting from the start position of a single position demarked by one landmark, they were categorized as response learners. We previously showed that spontaneous strategies, i.e., strategies used in the very first trial, are associated with increased functional magnetic resonance imaging (fMRI) blood oxygenation-level-dependent (BOLD) activity and gray matter in our regions of interest (Iaria et al., 2003; Bohbot et al., 2007). The verbal report is also used to determine how many landmarks participants noticed in the environment and how many landmarks they used on average throughout the learning trials.
The dependent variables are number of trials to criterion, the average number of errors on Part 2 of the learning trials (these provide two slightly different measures of navigational learning), spontaneous navigation strategy, number of landmarks noticed the average number of landmarks used during the learning phase of the task, and probe errors.
To assess potential differences between spatial and response learners in neuropsychological status, we administered the following neuropsychological tests: the Rey Auditory Verbal Learning Task (Rey, 1941) to evaluate verbal memory, the Rey-Osterrieth Complex Figure (Meyers and Meyers, 1995) to assess visuospatial memory, and the Test of Non-verbal Intelligence-3 (Brown et al., 1997) to assess non-verbal intelligence. Spatial and response learners did not differ on these tests (all Bootstrap BCa 95% CI crossed 0).
We acquired anatomical MRI data at the Douglas Cerebral Imaging Centre, using a 3 Tesla Siemens Magnetom Trio scanner equipped with 12-channel array coil. We immobilized participants’ heads using support cushions. A localizer scan was first acquired, followed by a T1-weighted 3D MPRAGE anatomical scan with 192 contiguous 1 mm slices in the sagittal plane (TR = 2,300 ms; TE = 2.98 ms; flip angle = 9; the field of view = 256 mm2), resulting in an acquisition time of 9:14 min.
The Multiple Automatically Generated Templates (MAGeT) Brain tool was used to automatically segment extra-hippocampal white matter fiber bundles (Amaral et al., 2018; Figure 2A). This tool was developed to use a small set of high-quality atlases that were manually segmented as input. Participants’ structural scans were processed with N4 intensity correction (Tustison et al., 2010). We then applied a head mask to ameliorate registration before running MAGeT-Brain. The Winterburn Atlas (Winterburn et al., 2013) was adapted to include the extra-hippocampal white matter bundles and is comprised of five manually-segmented brains (Amaral et al., 2018). In MAGeT-Brain, a library of 21 templates (Pipitone et al., 2014) is used to bootstrap each individual’s segmentation. The templates were chosen by first segmenting all of the samples using the five manually segmented brains and then selecting the ones presenting the best registrations in order to increase registration quality for the full sample analysis. We used non-linear atlas-to-template registration to segment and label each template, which resulted in a unique delineation of the subfields for each individual template. The bootstrapping yields 105 candidate labels for each individual (5 atlases × 21 templates), which are subsequently fused through a voxel-wise majority vote to output one final segmentation (Figure 2B). We used the Automatic Normalization Tools (ANTS) registration technique for non-linear registration1. The extra-hippocampal white matter bundles that we inspected included the fimbria, fornix, and alveus of the hippocampus. Together, these form the fimbria-fornix white matter bundle. We visually inspected each output segmentation for quality control, which all segmentations passed.
Figure 2. White matter segmentation. (A) 3D reconstruction of the white matter atlas (Amaral et al., 2018), showing the alveus, fimbria, and fornix, superimposed on the hippocampal subfield atlas (Pipitone et al., 2014). (B) Segmentations of the alveus (in orange), fimbria (in blue), and fornix (in purple) are shown for a representative participant. The other colors represent the various hippocampal subfields (not discussed in this article but see Dahmani et al., 2018). Sagittal views are shown on the left, coronal views are shown on the right. A: anterior; P: posterior; R: right; L: left; CA: cornu ammonis; DG: dentate gyrus; SR/SL/SM: stratum radiatum, lacunosum, and moleculare.
We used SPSS Statistics 20 (IBM) for data analysis. We performed partial correlations between our olfaction and 4/8 VM variables and white matter volumes. Sex was used as a covariate because men have on average a larger brain than women. We used bootstrapped bias-corrected and accelerated 95% confidence intervals (Bootstrap BCa 95% CI; Field, 2009) to determine significance. Bootstrapping is a resampling method that uses a sample dataset and simulates 1,000 datasets from this set by resampling with replacement. This method is useful in that it inherently corrects for multiple comparisons (Westfall and Young, 1993; Field et al., 2012). Confidence intervals are an estimation of the population’s true value, which makes them both more accurate and more robust than p-values (Rothman, 1978; Poole, 2001; Greenland et al., 2016), especially when bootstrapping methods are used (Westfall and Young, 1993). Resampling methods also offer the advantage of estimating Type I and Type II error rates more precisely than standard p-value adjustment methods (Field et al., 2012). It is, therefore, unnecessary to further correct for multiple comparisons. Another advantage of bootstrapping methods is that they are non-parametric, and thus do not require to transform the data when it is not normally distributed (Haukoos and Lewis, 2005; Field et al., 2012). We used one-tailed confidence intervals when analyses were hypothesis-driven. We first investigated the overall right and left fimbria-fornix white matter volumes. These were determined by calculating the sum of the fimbria, fornix, and alveus of the hippocampus, for each hemisphere. If no significant correlation was found between overall fimbria-fornix volumes and our measures of interest, then we investigated the sub-regions (fimbria, fornix, and alveus of the hippocampus) separately to see if any effect existed at a smaller scale.
Using participants’ 4/8 VM verbal report, we categorized 23 participants as spatial learners and 32 participants as response learners. We performed two sets of analyses: we looked at the associations between: (1) navigation and white matter volumes; and (2) olfaction and white matter volumes. We hypothesized that, as with our previous behavioral and structural neuroimaging results (Dahmani et al., 2018), only spatial learners would show an association between fimbria-fornix volume, olfaction, and navigation. Table 1 shows the correlation coefficients and bootstrap BCa 95% CI of the correlations between white matter volumes and navigation and olfaction variables for spatial and response learners, with sex as a covariate.
Table 1. Partial correlations between variables of interest and white matter bundle volumes for spatial and response learners.
In spatial learners, we found that faster learning correlates with fimbria-fornix white matter volume: there was a significant negative correlation between right fimbria-fornix volume and average navigational learning errors [r = −0.40, Bootstrap BCa 95% CI (−0.66, −0.15; Figure 3, left)], and between right fimbria volume and number of trials to criterion [r = −0.35, Bootstrap BCa 95% CI (−0.57, −0.15)]. Additionally, there was a marginally significant negative correlation between left fimbria volume and average navigational learning errors [r = −0.31, Bootstrap BCa 95% CI (−0.60, 0.004)]. Average navigational learning errors did not significantly correlate with the left fimbria-fornix volume, although the correlation was in the hypothesized direction [r = −0.19, Bootstrap BCa 95% CI (−0.52, 0.10)]. Thus, faster learning in spatial learners is predominantly associated with the right fimbria-fornix.
Figure 3. Right fimbria-fornix volume is associated with fewer errors during navigational learning in spatial learners, but not response learners. Within spatial learners, average navigational learning errors correlate negatively with right fimbria-fornix volume (shown on the left), r = −0.42 [Bootstrap BCa 95% CI (−0.66, −0.18)], but not with left fimbria-fornix volume (Bootstrap BCa 95% CI cross 0). Within the left fimbria-fornix sub-regions, average navigational learning errors show a marginally significant negative correlation with the left fimbria, r = −0.31 [Bootstrap BCa 95% CI (−0.60, 0.004)], but not with the other sub-regions (Bootstrap BCa 95% CI cross 0). Within response learners, average navigational learning errors do not correlate with left or right fimbria-fornix volume (shown on the right is the right fimbria-fornix volume correlation for comparison, r = 0.10 [Bootstrap BCa 95% CI (−0.26, 0.37)], or any of their sub-regions (Bootstrap BCa 95% CI cross 0). These results indicate that more efficient spatial learning is mainly associated with a greater volume of the right fimbria-fornix fiber system. Stimulus-response learning does not show any correlations with the left or right fimbria-fornix system. †Indicates two overlapping data points.
Response learners showed no significant associations between fimbria-fornix volumes and navigational learning [all Bootstrap BCa 95% CIs crossed 0; e.g., average navigational learning errors and right fimbria-fornix volume, r = 0.10, Bootstrap BCa 95% CI (−0.26, 0.37; Figure 3, right)]. There were also no significant associations with sub-regions of the left or right fimbria-fornix (all Bootstrap BCa 95% CIs crossed 0).
Spatial and response learners did not show any correlations between fimbria-fornix volumes and either number of landmarks noticed/used or probe errors (all Bootstrap BCa 95% CIs crossed 0).
Spatial learners showed a significant positive correlation between olfactory identification and volume of the right fimbria [r = 0.41, Bootstrap BCa 95% CI (0.08, 0.68; Figure 4, left)]. They did not show a significant correlation between olfactory identification and right [r = 0.24, Bootstrap BCa 95% CI (−0.10, 0.55)] or left [r = 0.14, Bootstrap BCa 95% CI (−0.16, 0.43)] fimbria-fornix volumes, or with any sub-regions of the left fimbria-fornix (all Bootstrap BCa 95% CIs crossed 0).
Figure 4. Olfactory identification correlates positively with right fimbria volume within spatial learners only. Within spatial learners, olfactory identification correlates positively with right fimbria volume (shown on the left), r = 0.41, Bootstrap BCa 95% CI (0.08, 0.68), but not with the left fimbria-fornix or any of its sub-regions (Bootstrap BCa 95% CI cross 0). Within response learners, olfactory identification does not correlate positively with either left or right fimbria-fornix volume or any of their sub-regions {shown on the right is the association with right fimbria volume for comparison, r = 0.18 [Bootstrap BCa 95% CI (−0.07, 0.41)]; all other Bootstrap BCa 95% CI cross 0}. These results indicate that olfactory identification correlates positively with a sub-region of the right fimbria-fornix fiber system in those who use hippocampal-dependent navigation strategies only. †Indicates two overlapping datapoints.
In response learners, there were no associations between olfactory identification and right [r = −0.12, Bootstrap BCa 95% CI (−0.37, 0.20)] or left [r = −0.03, Bootstrap BCa 95% CI (−0.35, 0.25)] fimbria-fornix volumes. There were also no correlations with any of the fimbria-fornix sub-region volumes (all Bootstrap BCa 95% CIs crossed 0; e.g., right fimbria: Figure 4, right).
In the current study, we examined whether the fimbria-fornix has a dissociable role in spatial learning and stimulus-response learning in humans. We found fimbria-fornix volume to be positively associated with spatial memory. As hypothesized, there is no association between fimbria-fornix volume and stimulus-response learning, further strengthening the idea that these two forms of navigation rely on separate neural networks (O’Keefe and Nadel, 1978; Packard et al., 1989; McDonald and White, 1993). Similarly, within spatial learners, olfactory identification shows positive correlations with the fimbria-fornix, which is not the case within response learners.
These findings are consistent with our previous study (Dahmani et al., 2018), where we found both spatial learning and olfactory identification to be associated with hippocampal gray matter volume, while there were no associations between stimulus-response learning and hippocampal volume.
In terms of lateralization, in our previous report we found an association between spatial memory, olfactory identification, and right hippocampal volume (Dahmani et al., 2018). Our white matter findings are concordant, as the effects we found predominantly involved the right fimbria-fornix fiber system. This is also consistent with a right-sided hippocampal lateralization for spatial memory and olfactory identification often reported in the literature (Habib and Sirigu, 1987; Zatorre and Jones-Gotman, 1990; Zatorre et al., 1992; Jones-Gotman and Zatorre, 1993; Barrash, 1998; Bohbot et al., 1998; Savic et al., 2000; Burgess, 2002; Frasnelli et al., 2010; Kjelvik et al., 2012; Smitka et al., 2012).
The positive association between olfactory identification and the right fimbria-fornix bundle was observed in spatial learners, but not response learners, a pattern of results that reflects that of our previous study (Dahmani et al., 2018). These findings indicate that the fimbria-fornix is involved in olfactory identification in individuals who spontaneously use the hippocampal memory system. The hippocampus itself is reported to be involved in olfactory identification in approximately half of the studies in the literature, while the other half does not report such involvement (Suzuki et al., 2001; Kjelvik et al., 2012, 2014; Seubert et al., 2013; Smitka et al., 2012; Segura et al., 2013). This inconsistency may be a product of inter-individual variability in hippocampal involvement. The fact that we found a correlation between olfactory identification and fimbria-fornix white matter among spatial learners but not response learners indicates that it may be useful to identify individuals’ spontaneous navigation strategies in order to capture this inter-individual variability. We speculated in Dahmani et al. (2018) that response learners may more heavily rely on other nodes of the olfactory network, or that they may use a different neural network for olfactory processing. There is a lot of evidence that olfaction is influenced by top-down processing (Zatorre et al., 2000; Finkel et al., 2001; Gottfried and Dolan, 2003; de Araujo et al., 2005; Grabenhorst and Rolls, 2010; Ferdenzi et al., 2017) and cognitive strategies may influence the neural networks underlying it (Karunanayaka et al., 2014). Future studies should seek to elucidate the neural networks involved in olfactory identification using functional connectivity methods and to determine whether spatial and response learners differ in the way they process olfactory information.
Our findings may have implications for aging research. Both olfaction and spatial memory are impaired early on in cognitive aging and Alzheimer’s disease (Henderson et al., 1989; Pai and Jacobs, 2004; Tu and Pai, 2006; Wilson et al., 2007, 2009; Devanand et al., 2008, 2015; Head and Isom, 2010; Stanciu et al., 2014; Allison et al., 2016; Lafaille-Magnan et al., 2017). Atrophy of the olfactory bulb, olfactory tract, entorhinal cortex, and hippocampus is also observed early in the disease (Pearson et al., 1985; Ferreyra-Moyano and Barragan, 1989; Talamo et al., 1989; Fox et al., 1996; Kaye et al., 1997; den Heijer et al., 2006). Our results suggest that dissociating individuals based on navigation strategies (spatial vs. response) combined with measuring errors during navigational learning and olfactory identification may be more sensitive to variations in hippocampal volume and hippocampal network white matter integrity, compared to looking at navigational learning errors or olfactory identification alone. It would be of interest to pursue this question in a future line of research.
In summary, our finding that fimbria-fornix volume is associated with spatial learning and olfactory identification is in line with our previous results (Dahmani et al., 2018). Not only are these two processes behaviorally linked, but they also share many neuroanatomical substrates (Dahmani et al., 2018). In a comprehensive review, Jacobs et al. (2015) describes that the size of the olfactory bulb, a primary olfactory area, covaries with hippocampal size in many mammals (Reep et al., 2007) and with navigational demand, according to factors such as home range size (Gittleman, 1991) and predatory strategy (Reep et al., 2007). Taken together, these pieces of evidence are consistent with our findings that spatial memory and olfaction are closely linked.
The raw data supporting the conclusions of this article will be made available by the authors, without undue reservation, to any qualified researcher.
The studies involving human participants were reviewed and approved by the Institutional Review Board of the Douglas Mental Health University Institute. The patients/participants provided their written informed consent to participate in this study.
LD and VB designed the study. JN, LD, and VB devised the MRI scanning protocol. LD performed the study, analyzed the data, and wrote the manuscript. BC, JN, and RP assisted with neuroimaging data preprocessing. RP, RA, and MC assisted with the MAGeT-Brain pipeline. VB supervised the study. All authors edited the manuscript.
This research was supported by Natural Sciences and Engineering Research Council of Canada (NSERC) grant no. 239896-2013 and a Canadian Institutes of Health Research (CIHR) Frederick Banting and Charles Best Doctoral Training Award.
The authors declare that the research was conducted in the absence of any commercial or financial relationships that could be construed as a potential conflict of interest.
We would like to thank Erica Chan, Meagan Bristowe, and Melissa Berman for their help with data collection.
Allison, S. L., Fagan, A. M., Morris, J. C., and Head, D. (2016). Spatial navigation in preclinical Alzheimer’s disease. J. Alzheimers Dis. 52, 77–90. doi: 10.3233/JAD-150855
Amaral, R. S., Park, M. T. M., Devenyi, G. A., Lynn, V., Pipitone, J., Winterburn, J., et al. (2018). Manual segmentation of the fornix, fimbria, and alveus on high-resolution 3T MRI: application via fully-automated mapping of the human memory circuit white and grey matter in healthy and pathological aging. Neuroimage 170, 132–150. doi: 10.1016/j.neuroimage.2016.10.027
Barrash, J. (1998). A historical review of topographical disorientation and its neuroanatomical correlates. J. Clin. Exp. Neuropsychol. 20, 807–827. doi: 10.1076/jcen.20.6.807.1114
Bohbot, V. D., and Corkin, S. (2007). Posterior parahippocampal place learning in HM. Hippocampus 17, 863–872. doi: 10.1002/hipo.20313
Bohbot, V. D., Iaria, G., and Petrides, M. (2004). Hippocampal function and spatial memory: evidence from functional neuroimaging in healthy participants and performance of patients with medial temporal lobe resections. Neuropsychology 18, 418–425. doi: 10.1037/0894-4105.18.3.418
Bohbot, V. D., Jech, R., Ruzicka, E., Nadel, L., Kalina, M., Stepánková, K., et al. (2002). Rat spatial memory tasks adapted for humans: characterization in subjects with intact brain and subjects with selective medial temporal lobe thermal lesions. Physiol. Res. 51, S49–S65.
Bohbot, V. D., Kalina, M., Stepankova, K., Spackova, N., Petrides, M., and Nadel, L. (1998). Spatial memory deficits in patients with lesions to the right hippocampus and to the right parahippocampal cortex. Neuropsychologia 36, 1217–1238. doi: 10.1016/s0028-3932(97)00161-9
Bohbot, V. D., Lerch, J., Thorndycraft, B., Iaria, G., and Zijdenbos, A. P. (2007). Gray matter differences correlate with spontaneous strategies in a human virtual navigation task. J. Neurosci. 27, 10078–10083. doi: 10.1523/JNEUROSCI.1763-07.2007
Brown, L., Sherbenou, R. J., and Johnsen, S. K. (1997). Test of Nonverbal Intelligence. 3rd Edn. Austin, TX: Pro-Ed.
Burgess, N. (2002). The hippocampus, space, and viewpoints in episodic memory. Q. J. Exp. Psychol. A 55, 1057–1080. doi: 10.1080/02724980244000224
Dahmani, L., and Bohbot, V. D. (2015). Dissociable contributions of the prefrontal cortex to hippocampus-and caudate nucleus-dependent virtual navigation strategies. Neurobiol. Learn. Mem. 117, 42–50. doi: 10.1016/j.nlm.2014.07.002
Dahmani, L., Patel, R. M., Yang, Y., Chakravarty, M. M., Fellows, L. K., and Bohbot, V. D. (2018). An intrinsic association between olfactory identification and spatial memory in humans. Nat. Commun. 9:4162. doi: 10.1038/s41467-018-06569-4
de Araujo, I. E., Rolls, E. T., Velazco, M. I., Margot, C., and Cayeux, I. (2005). Cognitive modulation of olfactory processing. Neuron 46, 671–679. doi: 10.1016/j.neuron.2005.04.021
de Bruin, J. P. C., Moita, M. P., de Brabander, H. M., and Joosten, R. N. (2001). Place and response learning of rats in a Morris water maze: differential effects of fimbria fornix and medial prefrontal cortex lesions. Neurobiol. Learn. Mem. 75, 164–178. doi: 10.1006/nlme.2000.3962
den Heijer, T., Geerlings, M. I., Hoebeek, F. E., Hofman, A., Koudstaal, P. J., and Breteler, M. M. (2006). Use of hippocampal and amygdalar volumes on magnetic resonance imaging to predict dementia in cognitively intact elderly people. Arch. Gen. Psychiatry 63, 57–62. doi: 10.1001/archpsyc.63.1.57
Devanand, D. P., Lee, S., Manly, J., Andrews, H., Schupf, N., Doty, R. L., et al. (2015). Olfactory deficits predict cognitive decline and Alzheimer dementia in an urban community. Neurology 84, 182–189. doi: 10.1212/wnl.0000000000001132
Devanand, D. P., Liu, X., Tabert, M. H., Pradhaban, G., Cuasay, K., Bell, K., et al. (2008). Combining early markers strongly predicts conversion from mild cognitive impairment to Alzheimer’s disease. Biol. Psychiatry 64, 871–879. doi: 10.1016/j.biopsych.2008.06.020
Etchamendy, N., Konishi, K., Pike, G. B., Marighetto, A., and Bohbot, V. D. (2012). Evidence for a virtual human analog of a rodent relational memory task: a study of aging and fMRI in young adults. Hippocampus 22, 869–880. doi: 10.1002/hipo.20948
Ferdenzi, C., Joussain, P., Digard, B., Luneau, L., Djordjevic, J., and Bensafi, M. (2017). Individual differences in verbal and non-verbal affective responses to smells: influence of odor label across cultures. Chem. Senses 42, 37–46. doi: 10.1093/chemse/bjw098
Ferreyra-Moyano, H., and Barragan, E. (1989). The olfactory system and Alzheimer’s disease. Int. J. Neurosci. 49, 157–197. doi: 10.3109/00207458909084824
Field, A., Miles, J., and Field, Z. (2012). Discovering Statistics Using R. Thousand Oaks: Sage Publications.
Finkel, D., Pedersen, N. L., and Larsson, M. (2001). Olfactory functioning and cognitive abilities: a twin study. J. Gerontol. B Psychol. Sci. Soc. Sci. 56, P226–P233. doi: 10.1093/geronb/56.4.p226
Fox, N., Warrington, E., Freeborough, P., Hartikainen, P., Kennedy, A., Stevens, J., et al. (1996). Presymptomatic hippocampal atrophy in Alzheimer’s disease: a longitudinal MRI study. Brain 119, 2001–2007. doi: 10.1093/brain/119.6.2001
Frasnelli, J., Lundstrom, J. N., Boyle, J. A., Djordjevic, J., Zatorre, R. J., and Jones-Gotman, M. (2010). Neuroanatomical correlates of olfactory performance. Exp. Brain Res. 201, 1–11. doi: 10.1007/s00221-009-1999-7
Freiherr, J., Gordon, A. R., Alden, E. C., Ponting, A. L., Hernandez, M. F., Boesveldt, S., et al. (2012). The 40-item monell extended sniffin’ sticks identification test (MONEX-40). J. Neurosci. Methods 205, 10–16. doi: 10.1016/j.jneumeth.2011.12.004
Gittleman, J. L. (1991). Carnivore olfactory bulb size: allometry, phylogeny and ecology. J. Zool. 225, 253–272. doi: 10.1111/j.1469-7998.1991.tb03815.x
Gottfried, J. A., and Dolan, R. J. (2003). The nose smells what the eye sees: crossmodal visual facilitation of human olfactory perception. Neuron 39, 375–386. doi: 10.1016/s0896-6273(03)00392-1
Grabenhorst, F., and Rolls, E. T. (2010). Attentional modulation of affective versus sensory processing: functional connectivity and a top-down biased activation theory of selective attention. J. Neurophysiol. 104, 1649–1660. doi: 10.1152/jn.00352.2010
Greenland, S., Senn, S. J., Rothman, K. J., Carlin, J. B., Poole, C., Goodman, S. N., et al. (2016). Statistical tests, P values, confidence intervals and power: a guide to misinterpretations. Eur. J. Epidemiol. 31, 337–350. doi: 10.1007/s10654-016-0149-3
Habib, M., and Sirigu, A. (1987). Pure topographical disorientation: a definition and anatomical basis. Cortex 23, 73–85. doi: 10.1016/s0010-9452(87)80020-5
Hartley, T., Maguire, E. A., Spiers, H. J., and Burgess, N. (2003). The well-worn route and the path less traveled: distinct neural bases of route following and wayfinding in humans. Neuron 37, 877–888. doi: 10.1016/s0896-6273(03)00095-3
Haukoos, J. S., and Lewis, R. J. (2005). Advanced statistics: bootstrapping confidence intervals for statistics with “difficult” distributions. Acad. Emerg. Med. 12, 360–365. doi: 10.1197/j.aem.2004.11.018
Head, D., and Isom, M. (2010). Age effects on wayfinding and route learning skills. Behav. Brain Res. 209, 49–58. doi: 10.1016/j.bbr.2010.01.012
Henderson, V. W., Mack, W., and Williams, B. W. (1989). Spatial disorientation in Alzheimer’s disease. Arch. Neurol. 46, 391–394. doi: 10.1001/archneur.1989.00520400045018
Iaria, G., Lanyon, L. J., Fox, C. J., Giaschi, D., and Barton, J. J. (2008). Navigational skills correlate with hippocampal fractional anisotropy in humans. Hippocampus 18, 335–339. doi: 10.1002/hipo.20400
Iaria, G., Petrides, M., Dagher, A., Pike, B., and Bohbot, V. D. (2003). Cognitive strategies dependent on the hippocampus and caudate nucleus in human navigation: variability and change with practice. J. Neurosci. 23, 5945–5952. doi: 10.1523/JNEUROSCI.23-13-05945.2003
Jacobs, L. F., Arter, J., Cook, A., and Sulloway, F. J. (2015). Olfactory orientation and navigation in humans. PLoS One 10:e0129387. doi: 10.1371/journal.pone.0129387
Jones, D. K., Knösche, T. R., and Turner, R. (2013). White matter integrity, fiber count, and other fallacies: the do’s and don’ts of diffusion MRI. Neuroimage 73, 239–254. doi: 10.1016/j.neuroimage.2012.06.081
Jones-Gotman, M., and Zatorre, R. J. (1993). Odor recognition memory in humans: role of right temporal and orbitofrontal regions. Brain Cogn. 22, 182–198. doi: 10.1006/brcg.1993.1033
Karunanayaka, P., Eslinger, P. J., Wang, J. L., Weitekamp, C. W., Molitoris, S., Gates, K. M., et al. (2014). Networks involved in olfaction and their dynamics using independent component analysis and unified structural equation modeling. Hum. Brain Mapp. 35, 2055–2072. doi: 10.1002/hbm.22312
Kaye, J. A., Swihart, T., Howieson, D., Dame, A., Moore, M., Karnos, T., et al. (1997). Volume loss of the hippocampus and temporal lobe in healthy elderly persons destined to develop dementia. Neurology 48, 1297–1304. doi: 10.1212/wnl.48.5.1297
Kjelvik, G., Evensmoen, H. R., Brezova, V., and Håberg, A. K. (2012). The human brain representation of odor identification. J. Neurophysiol. 108, 645–657. doi: 10.1152/jn.01036.2010
Kjelvik, G., Saltvedt, I., White, L. R., Stenumgård, P., Sletvold, O., Engedal, K., et al. (2014). The brain structural and cognitive basis of odor identification deficits in mild cognitive impairment and Alzheimer’s disease. BMC Neurol. 14:168. doi: 10.1186/s12883-014-0168-1
Konishi, K., and Bohbot, V. D. (2013). Spatial navigational strategies correlate with gray matter in the hippocampus of healthy older adults tested in a virtual maze. Front. Aging Neurosci. 5:1. doi: 10.3389/fnagi.2013.00001
Konishi, K., Etchamendy, N., Roy, S., Marighetto, A., Rajah, N., and Bohbot, V. D. (2013). Decreased fMRI activity in the hippocampus in favor of the caudate nucleus in older adults tested in a virtual navigation task. Hippocampus 23, 1005–1014. doi: 10.1002/hipo.22181
Lafaille-Magnan, M.-E., Poirier, J., Etienne, P., Tremblay-Mercier, J., Frenette, J., Rosa-Neto, P., et al. (2017). Odor identification as a biomarker of preclinical AD in older adults at risk. Neurology 89, 327–335. doi: 10.1212/WNL.0000000000004159
Lundström, J. N., Boesveldt, S., and Albrecht, J. (2011). Central processing of the chemical senses: an overview. ACS Chem. Neurosci. 2, 5–16. doi: 10.1021/cn1000843
Maguire, E. A., Burgess, N., Donnett, J. G., Frackowiak, R. S., Frith, C. D., and O’Keefe, J. (1998). Knowing where and getting there: a human navigation network. Science 280, 921–924. doi: 10.1126/science.280.5365.921
McDonald, R. J., and White, N. M. (1993). A triple dissociation of memory systems: hippocampus, amygdala, and dorsal striatum. Behav. Neurosci. 107, 3–22. doi: 10.1037//0735-7044.107.1.3
McDonald, R. J., and White, N. M. (1995). Hippocampal and nonhippocampal contributions to place learning in rats. Behav. Neurosci. 109, 579–593. doi: 10.1037/0735-7044.109.4.579
Meyers, J. E., and Meyers, K. R. (1995). Rey Complex Figure Test and Recognition Trial: Professional Manual. Odessa, FL: Psychological Assessment Resources.
Moscovitch, M., Rosenbaum, R. S., Gilboa, A., Addis, D. R., Westmacott, R., Grady, C., et al. (2005). Functional neuroanatomy of remote episodic, semantic and spatial memory: a unified account based on multiple trace theory. J. Anat. 207, 35–66. doi: 10.1111/j.1469-7580.2005.00421.x
Olton, D. S., and Papas, B. C. (1979). Spatial memory and hippocampal function. Neuropsychologia 17, 669–682. doi: 10.1016/0028-3932(79)90042-3
Olton, D. S., and Samuelson, R. J. (1976). Remembrance of places passed: spatial memory in rats. J. Exp. Psychol. Anim. Behav. Process. 2, 97–116. doi: 10.1037/0097-7403.2.2.97
Packard, M. G., Hirsh, R., and White, N. M. (1989). Differential effects of fornix and caudate nucleus lesions on two radial maze tasks: evidence for multiple memory systems. J. Neurosci. 9, 1465–1472. doi: 10.1523/jneurosci.09-05-01465.1989
Pai, M. C., and Jacobs, W. J. (2004). Topographical disorientation in community-residing patients with Alzheimer’s disease. Int. J. Geriatr. Psychiatry 19, 250–255. doi: 10.1002/gps.1081
Pearson, R., Esiri, M., Hiorns, R., Wilcock, G., and Powell, T. (1985). Anatomical correlates of the distribution of the pathological changes in the neocortex in Alzheimer disease. Proc. Natl. Acad. Sci. U S A 82, 4531–4534. doi: 10.1073/pnas.82.13.4531
Pigott, S., and Milner, B. (1993). Memory for different aspects of complex visual scenes after unilateral temporal-or frontal-lobe resection. Neuropsychologia 31, 1–15. doi: 10.1016/0028-3932(93)90076-c
Pipitone, J., Park, M. T. M., Winterburn, J., Lett, T. A., Lerch, J. P., Pruessner, J. C., et al. (2014). Multi-atlas segmentation of the whole hippocampus and subfields using multiple automatically generated templates. Neuroimage 101, 494–512. doi: 10.1016/j.neuroimage.2014.04.054
Poole, C. (2001). Low P-values or narrow confidence intervals: which are more durable? Epidemiology 12, 291–294. doi: 10.1097/00001648-200105000-00005
Reep, R., Finlay, B., and Darlington, R. (2007). The limbic system in mammalian brain evolution. Brain Behav. Evol. 70, 57–70. doi: 10.1159/000101491
Rey, A. (1941). L’examen psychologique dans les cas d’encéphalopathie traumatique. Arch. Psychol. 28, 215–285.
Rothman, K. J. (1978). A show of confidence. N. Engl. J. Med. 299, 1362–1363. doi: 10.1056/NEJM197812142992410
Savic, I., Gulyas, B., Larsson, M., and Roland, P. (2000). Olfactory functions are mediated by parallel and hierarchical processing. Neuron 26, 735–745. doi: 10.1016/s0896-6273(00)81209-x
Scholz, J., Tomassini, V., and Johansen-Berg, H. (2014). “Individual differences in white matter microstructure in the healthy brain,” in Diffusion MRI, eds H. Johansen-Berg and T. E. J. Behrens (Cambridge, MA: Elsevier), 301–316.
Segura, B., Baggio, H. C., Solana, E., Palacios, E. M., Vendrell, P., Bargalló, N., et al. (2013). Neuroanatomical correlates of olfactory loss in normal aged subjects. Behav. Brain Res. 246, 148–153. doi: 10.1016/j.bbr.2013.02.025
Seubert, J., Freiherr, J., Frasnelli, J., Hummel, T., and Lundström, J. N. (2013). Orbitofrontal cortex and olfactory bulb volume predict distinct aspects of olfactory performance in healthy subjects. Cereb. Cortex 23, 2448–2456. doi: 10.1093/cercor/bhs230
Smitka, M., Puschmann, S., Buschhueter, D., Gerber, J. C., Witt, M., Honeycutt, N., et al. (2012). Is there a correlation between hippocampus and amygdala volume and olfactory function in healthy subjects? Neuroimage 59, 1052–1057. doi: 10.1016/j.neuroimage.2011.09.024
Stanciu, I., Larsson, M., Nordin, S., Adolfsson, R., Nilsson, L.-G., and Olofsson, J. K. (2014). Olfactory impairment and subjective olfactory complaints independently predict conversion to dementia: a longitudinal, population-based study. J. Int. Neuropsychol. Soc. 20, 209–217. doi: 10.1017/s1355617713001409
Suzuki, Y., Critchley, H. D., Suckling, J., Fukuda, R., Williams, S. C., Andrew, C., et al. (2001). Functional magnetic resonance imaging of odor identification: the effect of aging. J. Gerontol. A Biol. Sci. Med. Sci. 56, M756–M760. doi: 10.1093/gerona/56.12.m756
Talamo, B. R., Rudel, R., Kosik, K. S., Lee, V. M.-Y., Neff, S., Adelman, L., et al. (1989). Pathological changes in olfactory neurons in patients with Alzheimer’s disease. Nature 337, 736–739. doi: 10.1038/337736a0
Tu, M.-C., and Pai, M.-C. (2006). Getting lost for the first time in patients with Alzheimer’s disease. Int. Psychogeriatr. 18, 567–570. doi: 10.1017/s1041610206224025
Tustison, N. J., Avants, B. B., Cook, P. A., Zheng, Y., Egan, A., Yushkevich, P. A., et al. (2010). N4ITK: improved N3 bias correction. IEEE Trans. Med. Imaging 29, 1310–1320. doi: 10.1109/TMI.2010.2046908
Westfall, P. H., and Young, S. S. (1993). Resampling-Based Multiple Testing: Examples and Methods for p-value adjustment. New York, NY: John Wiley and Sons.
White, N. M., and McDonald, R. J. (2002). Multiple parallel memory systems in the brain of the rat. Neurobiol. Learn. Mem. 77, 125–184. doi: 10.1006/nlme.2001.4008
Wilson, R. S., Arnold, S. E., Schneider, J. A., Boyle, P. A., Buchman, A. S., and Bennett, D. A. (2009). Olfactory impairment in presymptomatic Alzheimer’s disease. Ann. N Y Acad. Sci. 1170, 730–735. doi: 10.1111/j.1749-6632.2009.04013.x
Wilson, R. S., Arnold, S. E., Schneider, J. A., Tang, Y., and Bennett, D. A. (2007). The relationship between cerebral Alzheimer’s disease pathology and odour identification in old age. J. Neurol. Neurosurg. Psychiatry 78, 30–35. doi: 10.1136/jnnp.2006.099721
Winterburn, J. L., Pruessner, J. C., Chavez, S., Schira, M. M., Lobaugh, N. J., Voineskos, A. N., et al. (2013). A novel in vivo atlas of human hippocampal subfields using high-resolution 3T magnetic resonance imaging. Neuroimage 74, 254–265. doi: 10.1016/j.neuroimage.2013.02.003
Wu, K. N., Tan, B. K., Howard, J. D., Conley, D. B., and Gottfried, J. A. (2012). Olfactory input is critical for sustaining odor quality codes in human orbitofrontal cortex. Nat. Neurosci. 15, 1313–1319. doi: 10.1038/nn.3186
Zatorre, R. J., and Jones-Gotman, M. (1990). Right-nostril advantage for discrimination of odors. Percept. Psychophys. 47, 526–531. doi: 10.3758/bf03203105
Zatorre, R. J., Jones-Gotman, M., Evans, A. C., and Meyer, E. (1992). Functional localization and lateralization of human olfactory cortex. Nature 360, 339–340. doi: 10.1038/360339a0
Keywords: spatial memory, navigation, hippocampus, fimbria-fornix, white matter, olfaction
Citation: Dahmani L, Courcot B, Near J, Patel R, Amaral RSC, Chakravarty MM and Bohbot VD (2020) Fimbria-Fornix Volume Is Associated With Spatial Memory and Olfactory Identification in Humans. Front. Syst. Neurosci. 13:87. doi: 10.3389/fnsys.2019.00087
Received: 17 October 2019; Accepted: 23 December 2019;
Published: 14 January 2020.
Edited by:
James Ainge, University of St Andrews, United KingdomReviewed by:
Benjamin J. Clark, University of New Mexico, United StatesCopyright © 2020 Dahmani, Courcot, Near, Patel, Amaral, Chakravarty and Bohbot. This is an open-access article distributed under the terms of the Creative Commons Attribution License (CC BY). The use, distribution or reproduction in other forums is permitted, provided the original author(s) and the copyright owner(s) are credited and that the original publication in this journal is cited, in accordance with accepted academic practice. No use, distribution or reproduction is permitted which does not comply with these terms.
*Correspondence: Véronique D. Bohbot, dmVyb25pcXVlLmJvaGJvdEBtY2dpbGwuY2E=
Disclaimer: All claims expressed in this article are solely those of the authors and do not necessarily represent those of their affiliated organizations, or those of the publisher, the editors and the reviewers. Any product that may be evaluated in this article or claim that may be made by its manufacturer is not guaranteed or endorsed by the publisher.
Research integrity at Frontiers
Learn more about the work of our research integrity team to safeguard the quality of each article we publish.