- 1Laboratory of Synthetic, Perceptive, Emotive and Cognitive Systems, Institute for Bioengineering of Catalonia, The Barcelona Institute of Science and Technology, Barcelona, Spain
- 2Institucio Catalana de Recerca I Estudis Avançats, Barcelona, Spain
What are the principles underlying effective neurorehabilitation? The aim of neurorehabilitation is to exploit interventions based on human and animal studies about learning and adaptation, as well as to show that the activation of experience-dependent neuronal plasticity augments functional recovery after stroke. Instead of teaching compensatory strategies that do not reduce impairment but allow the patient to return home as soon as possible, functional recovery might be more sustainable as it ensures a long-term reduction in impairment and an improvement in quality of life. At the same time, neurorehabilitation permits the scientific community to collect valuable data, which allows inferring about the principles of brain organization. Hence neuroscience sheds light on the mechanisms of learning new functions or relearning lost ones. However, current rehabilitation methods lack the exact operationalization of evidence gained from skill learning literature, leading to an urgent need to bridge motor learning theory and present clinical work in order to identify a set of ingredients and practical applications that could guide future interventions. This work aims to unify the neuroscientific literature relevant to the recovery process and rehabilitation practice in order to provide a synthesis of the principles that constitute an effective neurorehabilitation approach. Previous attempts to achieve this goal either focused on a subset of principles or did not link clinical application to the principles of motor learning and recovery. We identified 15 principles of motor learning based on existing literature: massed practice, spaced practice, dosage, task-specific practice, goal-oriented practice, variable practice, increasing difficulty, multisensory stimulation, rhythmic cueing, explicit feedback/knowledge of results, implicit feedback/knowledge of performance, modulate effector selection, action observation/embodied practice, motor imagery, and social interaction. We comment on trials that successfully implemented these principles and report evidence from experiments with healthy individuals as well as clinical work.
Introduction
So far there is no clear understanding of the principles underlying effective neurorehabilitation approaches. Therapeutic protocols can be readily described by the following aspects: the body part trained (e.g., the legs), the tools or machines used for the training (e.g., a treadmill), the activity performed (e.g., walking), and when the therapy commences (e.g., during the acute phase after a stroke). However, an intervention typically includes more elements. For instance, the use of the less affected limb can be restricted, and the therapist can encourage the patient to spend more time exercising or give feedback about task performance. While some interventions, like CIMT, clearly define their active ingredients (Carter et al., 2010; Proffitt and Lange, 2015) that should lead to effective recovery (Kwakkel et al., 2015), most others do not. Neurorehabilitation research aims to find interventions that promote recovery and to establish whether the presence or absence of improvement can be explained by any neuronal changes that occur in the post-stroke brain (Dobkin, 2005). Neuroscience can help us to create interventions that lead to changes in the brain; however, with no clear understanding of what an intervention does, attributing causality remains difficult. One way to formalize an intervention is by breaking it into parts, studying the behavioral and neural effects of these parts, and deriving principles from them–in the case of stroke neurorehabilitation, these would be principles that optimize acquisition, retention, and generalization of skills.
While there are plenty of meta-analyses that look at training effectiveness in terms of individual body parts/functions, tools, or machines and activities (Langhorne et al., 2009; Veerbeek et al., 2014), the effect of experience remains much less clear in spite of attempts to formalize and identify the principles of neurorehabilitation. A review of principles of experience-dependent neural plasticity by Kleim and Jones (2008) explains why training is crucial for recovery. According to their work, neurorehabilitation presumes that exposure to specific training experiences leads to improvement of impairment by activating neural plasticity mechanisms. Consequently most of the work in the field focuses on the identification of scientifically grounded principles that should guide the design of these training experiences. In this vein, Kleim and Jones (2008) elaborated on five main principles of effective training experience — specificity, repetition, intensity, time, and salience — but offered little concrete applicability. Another synthesis addressed further principles (forced use, massed practice, spaced practice, task-oriented functional training, randomized training); however, the main focus of the review was on individual body functions, methods, or tools, providing a global view on rehabilitation strategies (Dobkin, 2004). Two meta-analyses investigated specific principles. One looked only at the principle of intensity and found that more therapy time did enhance functional recovery (Kwakkel, 2009). Another determined that repetition does improve upper and lower limb function (Thomas et al., 2017). However, both studies did not investigate the mechanisms that would lead to the effects observed. Similarly, a review that analyzed CIMT, which combines several principles in one method, gained interesting insights in its efficacy but did not explain the results from a neuroscientific, mechanistic point of view (Kwakkel et al., 2015). The work by Levin et al. (2015), on the other hand, tried to link the principles of motor learning to the application of these principles in novel rehabilitation methods while offering some neuroscientific reasoning for doing so. Their review addresses the difficulty of the task, the organization of movement, movements to the contralateral workspace, visual cues and objects and the interaction with them, sensory feedback, feedback about performance and results, repetitions, variability, and motivation. However, the included motor control and motor learning principles were not well defined and therefore leave room for interpretation (Levin et al., 2015).
In a previous meta-analysis (Maier et al., 2019), we compiled a list of principles for neurorehabilitation based on literature on motor learning and recovery: massed practice, dosage, structured practice, task-specific practice, variable practice, multisensory stimulation, increasing difficulty, explicit feedback/knowledge of results, implicit feedback/knowledge of performance, movement representation, and promotion of the use of the affected limb. We then performed a content analysis to determine whether these principles were present in the clinical studies included in the review, but we did not provide an analysis of the principles identified. In this work, we aim to extend the number of principles found and, for each of them, unify the neuroscientific literature from human or animal studies on motor learning and comment on the observed neuronal effects. We also include evidence from clinical studies to show its effect in recovering functionality after stroke. Some principles already serve as building blocks of effective rehabilitation programs, e.g., CIMT (Kwakkel et al., 2015), Bobath (Kollen et al., 2009), enriched rehabilitation (Livingston-Thomas et al., 2016), VR-based rehabilitation (Laver et al., 2017), and exogenous or robotic interventions (Langhorne et al., 2011). However, transferring these principles into clinical practice faces the challenge of operationalizing them. We comment on these difficulties and the gaps between theory, evidence, and operationalization that we encountered. Consequently, this work can serve clinicians and researchers as a practical guide of principles to investigate further effective neurorehabilitation approaches.
Materials and Methods
In this conceptual analysis, the rehabilitation experience is broken down into individual parts that are termed principles of neurorehabilitation. They are principles because they are evidenced by experimental data, and together, they could form the foundation of a higher-order theoretical framework. As a first attempt, a list of 11 principles was compiled based on existing literature in a recent meta-analysis on the effectiveness of VR-based rehabilitation systems for stroke recovery (Maier et al., 2019). For the current work, the list has been revised, and additional principles have been identified through a computerized search in PubMed Central using the keywords “principles of motor learning,” “principles of recovery,” “principles of experience-dependent learning” and “principles of neurorehabilitation.” We restricted the search to the last 5 years to obtain currently used principles. We focused on reviews, perspectives, and debates around rehabilitation methods and interventions for stroke recovery and excluded articles that explained study protocols or clinical trials, prevention methods, pharmaceutical or medical interventions, or stroke taxonomies. The principles mentioned in each paper were compared with the original list and added if they were not present. Afterward, we summarized for each principle the historical background based on motor learning literature and its contribution to learning based on human or animal studies. Further, where available, neurological effects and clinical outcomes were included as well.
Identification of Principles of Neurorehabilitation
Our computerized search yielded 548 records, of which 74 were deemed adequate for further screening after we examined if their titles either contained any of the search terms or appeared to discuss post-stroke rehabilitation strategies. After analysis of their abstracts and full-texts, the principles mentioned in 17 articles were extracted. We excluded papers if their title or abstract reported or compared surgical or pharmaceutical interventions as well as if they discussed stroke taxonomies, proposed study protocols or clinical trials, covered principles unrelated to stroke and/or stroke rehabilitation itself (e.g., principles for disease prevention, pre- and post-operative care, care facilities, patient management, therapist education, nursing practice, dietary recommendation, veterinary etc.), or looked into patient or caregiver perception. The articles and reviews selected spawned various research fields in neurorehabilitation: Motor learning (Winstein et al., 2014), therapies [physical therapy (Veerbeek et al., 2014), upper limb immobilization (Furlan et al., 2016), environmental enrichment (Livingston-Thomas et al., 2016), aerobic training (Billinger, 2015; Hasan et al., 2016), CIMT (Kwakkel et al., 2015; Zhang et al., 2017), cognitive rehabilitation (Middleton and Schwartz, 2012), music therapy (Zhang et al., 2016)], tools and methods [hand robotics (Yue et al., 2017), VR (Darekar et al., 2015; Fu et al., 2015), neurofeedback (Renton et al., 2017)], and principles [dose and timing (Basso and Lang, 2017)]. Together with previously collated literature, we identified 15 principles.
The identified principles from the meta-analysis are as follows:
• Massed practice/repetitive practice (Middleton and Schwartz, 2012; Veerbeek et al., 2014; Fu et al., 2015; Kwakkel et al., 2015; Furlan et al., 2016; Zhang et al., 2016).
• Spaced practice (Middleton and Schwartz, 2012; Billinger, 2015; Hasan et al., 2016; Livingston-Thomas et al., 2016).
• Dosage/duration (Veerbeek et al., 2014; Winstein et al., 2014; Billinger, 2015; Darekar et al., 2015; Kwakkel et al., 2015; Hasan et al., 2016; Livingston-Thomas et al., 2016; Basso and Lang, 2017; Zhang et al., 2017).
• Task-specific practice (Veerbeek et al., 2014; Winstein et al., 2014; Fu et al., 2015; Kwakkel et al., 2015; Furlan et al., 2016; Livingston-Thomas et al., 2016; Yue et al., 2017).
• Variable practice (Darekar et al., 2015; Fu et al., 2015; Livingston-Thomas et al., 2016).
• Increasing difficulty (Winstein et al., 2014; Fu et al., 2015; Kwakkel et al., 2015; Furlan et al., 2016; Hasan et al., 2016; Livingston-Thomas et al., 2016; Zhang et al., 2017).
• Multisensory stimulation (Veerbeek et al., 2014; Livingston-Thomas et al., 2016; Yue et al., 2017).
• Explicit feedback/knowledge of results (Middleton and Schwartz, 2012; Veerbeek et al., 2014; Darekar et al., 2015; Fu et al., 2015; Renton et al., 2017).
• Implicit feedback/knowledge of performance (Veerbeek et al., 2014; Darekar et al., 2015; Fu et al., 2015; Zhang et al., 2016; Renton et al., 2017; Yue et al., 2017).
• Modulate effector selection (Veerbeek et al., 2014; Winstein et al., 2014; Kwakkel et al., 2015; Furlan et al., 2016; Zhang et al., 2017).
• Action observation/embodied practice (Veerbeek et al., 2014; Fu et al., 2015; Yue et al., 2017).
Additional principles encountered through the search:
• Goal-oriented practice (Winstein et al., 2014; Fu et al., 2015; Yue et al., 2017).
• Rhythmic cueing (Middleton and Schwartz, 2012; Veerbeek et al., 2014; Zhang et al., 2016).
• Motor imagery/mental practice (Veerbeek et al., 2014).
• Social interaction (Winstein et al., 2014; Fu et al., 2015; Livingston-Thomas et al., 2016; Zhang et al., 2017).
In the following sections, we summarize for each principle the theoretical background, the evidence for motor learning, and the clinical effectiveness. We also added studies that comment on the neurological changes observed after applying the principles in motor learning tasks. The detailed neurological changes reported by these studies can be found in Table 1.
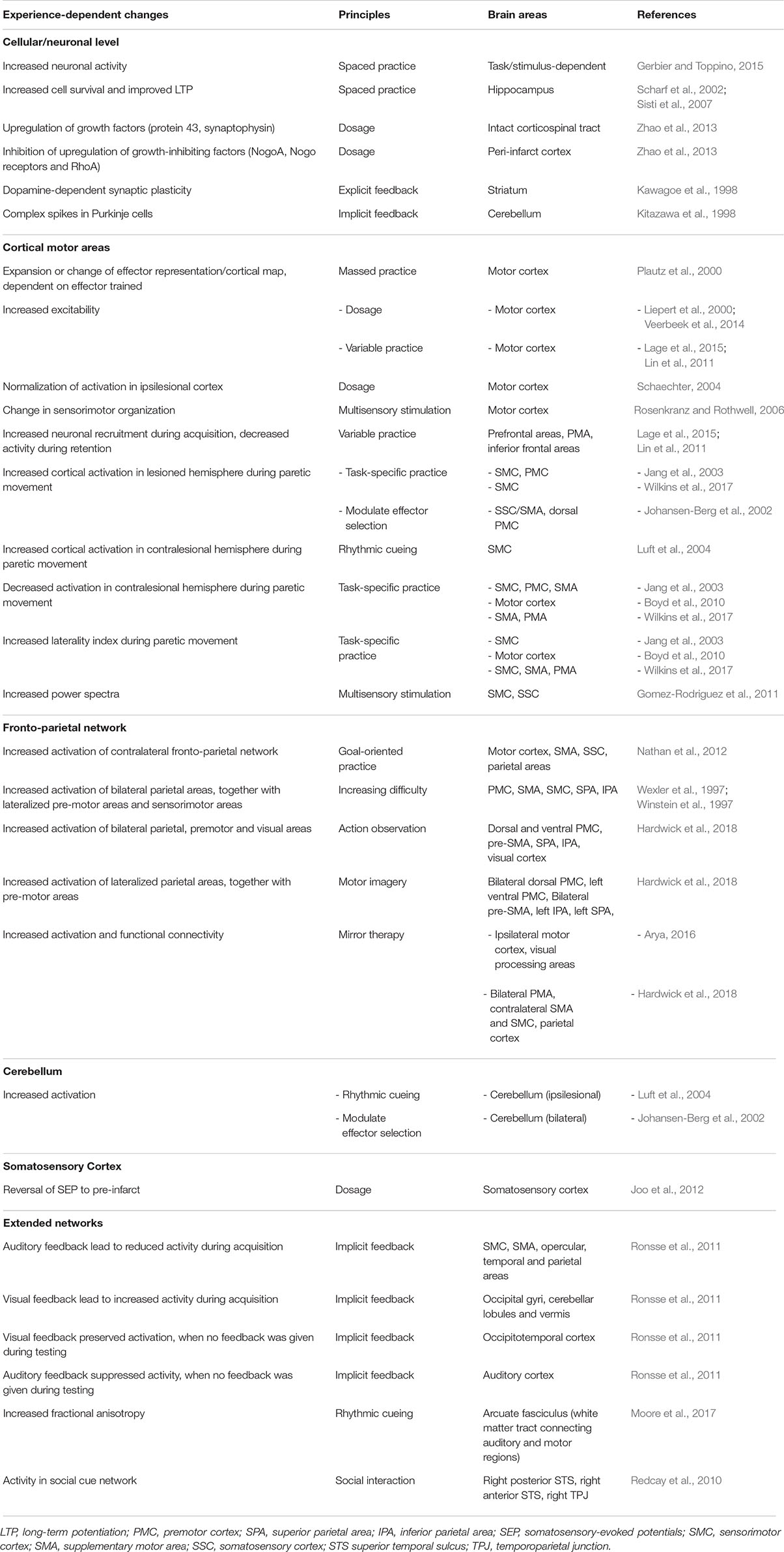
Table 1. Overview of the neuronal changes due to exposure to principles of neurorehabilitation included in this manuscript.
Massed Practice/Repetitive Practice
Massed practice was defined as work episodes with very brief to no rest periods (Schmidt and Lee, 2011). Within a work episode, a skill can be trained repeatedly in a constant or blocked fashion (Ammons, 1947; Mulligan et al., 1980). In the field of rehabilitation, the term describes the prolonged and repeated use of the more affected limb (Taub et al., 1999). Theoretically, learning through repetitions can speed-up the shaping of priors, which, together with likelihoods based on sensory input, aid in making an optimal estimate for action selection (Körding and Wolpert, 2006). Animal studies have shown that repeating skilled movements leads to localized changes in the area responsible for the movement, whereas the pure repetition of unskilled movement does not (Plautz et al., 2000). In humans, early studies have shown that blocked practice leads to faster acquisition, but poorer retention and less transfer than variable practice (Shea and Morgan, 1979) and that massed practice without breaks seems less effective for motor performance (Ammons, 1947; Ammons and Willig, 1956).
In standard therapies or clinical studies, the amount of repetition is typically not quantified but was observed to be an order of magnitude lower than in studies investigating recovery in rats and monkeys (Lang et al., 2007). Instead, the evidence for massed practice relies typically on the number of sessions or duration (French et al., 2016). A study looking into the feasibility of translating repetition amounts of animals to humans found improved motor functioning after training with high-repetition doses. However, no “pure” repetition training was provided, as the protocol included a variety of tasks that increased in difficulty (Birkenmeier et al., 2010). On the contrary, a study comparing four groups with different repetition amounts did not find significant differences based on the number of repetitions (Basso and Lang, 2017). This intervention included other principles as well. Meta-analyses confirm the mixed effects of repetitive training on improvement (Langhorne et al., 2011; Veerbeek et al., 2014; French et al., 2016; Thomas et al., 2017). Hence, massed practice appears to be a commonly used ingredient, but its clinical operationalization is often confounded with other principles. In order to investigate its true effects on recovery and compare across studies, the repetitions within a training session and across therapy duration should be measured and quantified.
Spaced Practice
Spaced practice implies that training should be structured in time to include rest periods between repetitions or sessions (Lee and Genovese, 1988; Schmidt and Lee, 2011). Instead of spaced practice, the term distributed practice is often used in literature. However, some authors use the term distributed practice as a combination of spaced and massed practice (Cepeda et al., 2006). Research on human skill acquisition suggests that increasing the time spacing between learning periods improves final test performance (Cepeda et al., 2006). However, when these learning periods are too long, learning and retention rates drop (Savion-Lemieux and Penhune, 2005). The mechanisms behind the effects of distributed practice remain unclear. It has been hypothesized that the first exposure to a stimulus pre-activates its representation in memory, requiring no further activation in a subsequent repetition trial, leading to a poorer internal representation of that stimulus, which has been termed as the repetition suppression effect (Gerbier and Toppino, 2015). Animal and fMRI studies support this hypothesis, showing that neuronal activation decreases after stimulus repetition where the magnitude is modulated by the delay between the first and second presentation, with larger delays leading to greater decreases (Brown et al., 1987; Henson et al., 2000, 2004; Henson, 2003). Spaced practice might counteract the repetition suppression effect by canceling stimulus priming (Gerbier and Toppino, 2015). TMS revealed that primary and supplementary motor areas are involved in motor memory consolidation (Censor and Cohen, 2011), which might be facilitated by spaced practice. Further, learning and physical activity have been linked to hippocampal neurogenesis (Praag et al., 1999). Animal studies also suggest that spaced practice facilitates long-term memory formation (Okamoto et al., 2011; Yamazaki et al., 2015) by fostering the survival of cells in the dentate gyrus that are important for learning and memory (Sisti et al., 2007). Also, in vivo spacing of electrical stimulation facilitates the recruitment of protein-synthesis-dependent processes, which facilitates late LTP effects (Scharf et al., 2002; Gerbier and Toppino, 2015).
In the clinical field, only a few studies have investigated the effect of spacing on post-stroke recovery. A clinical study that investigated whether a CIMT protocol could be distributed over more days with less therapy time per day showed improvement in motor outcomes that were similar to previous CIMT protocols and superior outcomes in long-term quality of life (Dettmers et al., 2005).
Dosage
Unlike in pharmacology, dosage is an ill-defined term in rehabilitation (Dobkin, 2005; Kwakkel, 2009). Generally, it is operationalized as the number of hours spent in therapy (Kwakkel, 2009; Birkenmeier et al., 2010; Veerbeek et al., 2014; Basso and Lang, 2017), the frequency of training sessions and the duration of a session (Dobkin, 2005), or the training amount required to stimulate learning (Wadden et al., 2017). High dosages are often equated with high intensity of training (Kwakkel et al., 2015). However, the intensity of training could also be operationalized as the metabolic cost, work rate, or perceived intensity through exertion (Billinger, 2015; Hasan et al., 2016), which are rarely measured in standard therapies except in fitness and aerobic protocols (Kwakkel, 2009).
Typically, inpatients receive only 22 (Veerbeek et al., 2014) to 60 min of training a day, with fewer minutes at later stages (Schaechter, 2004). There is some evidence that increasing therapy hours would be beneficial to speeding up functional recovery (Lohse et al., 2014; Veerbeek et al., 2014). At least 16 h of extra training (e.g., 71 more minutes per day for 3 months) within the first 6 months seem to be required for functional gains (Kwakkel et al., 2004; Veerbeek et al., 2014). However, there is some controversy over the benefits of increased training early after stroke (Schaechter, 2004; Dromerick et al., 2009; Kwakkel, 2009), and a pooled analysis revealed no evidence of an effect of additional doses (Hayward et al., 2014). Hence, the exact dose-response for different therapies at different stages post-stroke needs to be determined (Kwakkel, 2009; Basso and Lang, 2017). Also, it seems that motor performance needs to reach an asymptotic level in the first session to facilitate delayed performance gains across sessions or days. Therefore, delayed performance gains seem not to depend on repetition or over-night consolidation, but on the amount of training that induces asymptote in the individual’s performance (Hauptmann et al., 2005). Neurologically, high-dose rehabilitation protocols with extended training hours possibly induce structural plastic changes as well as a reorganization of neural networks (summarized by Kwakkel et al., 2015), increase cortical excitability and improve motor function and use (Liepert et al., 2000; Veerbeek et al., 2014). Several studies observed a normalization in ipsilesional cortex activity, which could underlie the functional gains (Schaechter, 2004).
Task-Specific Practice
Task-specific practice postulates that changing the conditions of a task might require a change in the abilities needed to execute it; conditions during training should match the conditions during testing (Schmidt and Lee, 2011). Thus, the specific conditions of practice shape the internal sensorimotor representation of the skill learned (Nudo et al., 1996; Ridderinkhof et al., 2004), leading potentially to highly specialized skills (Keetch et al., 2005) whose performance is superior in transfer tasks that meet the training conditions (Schmidt and Lee, 2011). Grounded in this principle, conventional rehabilitation protocols focus their training on the execution of ADL, as they are deemed meaningful to the patient (Hubbard et al., 2009). Since the main target of rehabilitation is to enable the patient to perform ADL independently (Winstein et al., 2014), therapy might not prioritize the restoration of pre-stroke movement patterns but allows the patient to acquire compensatory movement skills.
One study with a large sample size found that task-specific practice appears to be similar to standard therapy in improving motor functionality (Winstein et al., 2016). On the other hand, smaller fMRI studies found that task-specific training facilitated motor learning and retention (Boyd et al., 2010) and induced a change in the laterality index, which was confirmed in other studies as well (Jang et al., 2003; Wilkins et al., 2017). However, while two studies found reduced activity in the contralesional cortex, one (Jang et al., 2003) found changes in neuronal activity patterns in both hemispheres. A study with TMS demonstrated a trend toward reduced interhemispheric inhibition following task-specific training (Singer et al., 2013).
Goal-Oriented Practice
Since a given goal (e.g., throwing a ball into the basket) could be accomplished by many different motor synergies, it is assumed that movement control is achieved through the coupling of goal-specific functional movements. Goal-oriented practice, therefore, does not emphasize primarily individual muscles or movement patterns involved in execution but requires the patient to explore the couplings that are suitable to achieve the task (Horak, 1991). In general, motor skill performance and learning are enhanced if attention is directed to the effect of movement instead to the movement itself (Wulf and Prinz, 2001). Goal-oriented movements appear to produce a better reaching performance than the same movements without a goal (Wu et al., 2000), and setting specific, difficult goals leads to higher motor learning performance than non-specific goals (Gauggel and Fischer, 2001). It appears that probing a skill in a goal-directed fashion after overnight consolidation promotes better performance than probing the skill by drawing attention to finger movements (Cohen et al., 2005). Evidence from studies looking into tool-use in animals and humans suggest that, neurologically, action goals are represented as effector-dependent in the anterior intraparietal sulcus and primary motor areas, and as effector-independent in the ventral intraparietal sulcus and premotor cortex (Gallivan and Culham, 2015). Goal-oriented movements produce higher activity in sensorimotor areas (Nathan et al., 2012).
There is some evidence that goal-oriented practice is beneficial for recovery (Bosch et al., 2014). However, the described interventions seem to be confounded by other principles that are sometimes ascribed to goal-oriented training (Harvey, 2009).
Variable Practice
Variable practice can be achieved in two ways: (1) by providing variability within a training sequence, a method termed as variability of practice (Schmidt, 1975), or (2) by randomizing the presentation of individual training sequences, a method termed as random practice or contextual interference (Battig, 1966; Shea and Morgan, 1979). Both methods have been shown to lead to better retention (Shea and Kohl, 1991) and enhanced generalization to similar but untrained tasks (McCracken and Stelmach, 1977) or movements (Shea and Morgan, 1979; Mulder and Hochstenbach, 2001; Park et al., 2016), despite hampering initial performance (Shea and Morgan, 1979). However, a random presentation of information might be detrimental to motor learning (Mulder and Hochstenbach, 2001). Imaging studies have shed some light on the mechanisms supporting these effects. fMRI and TMS studies in humans indicate that improved performance due to variable practice correlates with increased neuronal activity and connectivity in the areas of the motor learning network during acquisition, which is associated with better performance at retention stages (Lage et al., 2015). Also, the motor cortex showed greater excitability during retention. These results point to more efficient retrieval of motor memory due to variable practice (Lin et al., 2011). More complex bimanual visuomotor tasks that were practiced randomly have shown modality-specific activation patterns that led to the recruitment of areas related to visual processing (Pauwels et al., 2018). The effect of variable practice might be related to the strong link between the neuromodulatory systems that control neuronal plasticity and novelty, for instance, the dopaminergic (Redgrave and Gurney, 2006), cholinergic (Hasselmo et al., 1996), and noradrenergic systems (Vankov et al., 1995), which are used by the brainstem activation system for controlling the global state of arousal (Gur et al., 2007).
In the clinical context, one study that investigated random versus blocked practice failed to find an effect (Hayward et al., 2014). It seems that this principle is rarely studied explicitly in clinical studies (Darekar et al., 2015; Nielsen et al., 2015), but instead applied in conjunction with other principles to overcome boredom (Birkenmeier et al., 2010).
Increasing Difficulty
According to Guadagnoli and Lee (2004) and based on the ideas from Marteniuk (1976), task difficulty can be described by the training requirements and conditions that are pertinent to the task, called the nominal task difficulty, and by how challenging the training is relative to the skill of the performer, called the functional task difficulty. Practice leads to fewer prediction errors and less need to process error information. Increasing the nominal task difficulty hence increases prediction errors and error processing demands. The optimal challenge point lies where functional task difficulty leads to a balance between information processing demands and performance, which is optimal for learning (Marteniuk, 1976; Guadagnoli and Lee, 2004). It has been shown that training with difficulty levels personalized to the learner’s capabilities leads to superior learning outcomes than when increases in difficulty are fixed (Wickens et al., 2013). Further, if subjects can control the task difficulty by themselves, their motor performance during acquisition and retention is significantly better (Andrieux et al., 2012). However, if difficulty surpasses one’s perceived ability to succeed, it might lead to detrimental effects on performance (Gendolla, 1999). Brain imaging studies showed increased activity in lateralized pre-motor and sensorimotor areas, but with an even more pronounced increase in parietal areas, pointing to a specialization of that area for task complexity (Wexler et al., 1997; Winstein et al., 1997). Potentially, noradrenergic neurons keep track of high or low task performance due to difficulty by switching their activity pattern preceding behavior (Rajkowski et al., 2004; Aston-Jones and Cohen, 2005).
In stroke rehabilitation, task difficulty has been partly investigated through shaping or graded practice. Shaping is a concept that was initially used by behaviorists studying operant conditioning in animals and that was successfully transferred from animals to humans by making it part of CIMT (Taub, 1976; Taub and Uswatte, 2003): The use of the impaired limb is augmented by progressively increasing the complexity of the required movement (Taub et al., 1994; Kwakkel et al., 2015). Although shaping appears to be one of the essential components of CIMT, its particular effect on motor recovery has not been studied on its own (Kwakkel et al., 2015). Increasing difficulty has been successfully used in standard care studies (Woldag et al., 2010), robot-assisted therapy (Lucca, 2009), and VR-based systems (Cameirão et al., 2012; Ballester Rubio et al., 2016), all of which showed beneficial effects on motor recovery. Task difficulty appears to be implicitly present in many tasks that investigate motor learning without being explicitly operationalized.
Multisensory Stimulation
The perception and integration of multiple senses are fundamental abilities of the brain. Because sensory information is noisy, the integration of various modalities requires probabilistic estimations to enhance perception (Knill and Pouget, 2004). Studies in the cat superior colliculus showed that a single neuron could be responsive to several sensory modalities (Meredith and Stein, 1986; Wallace and Stein, 1996). In primates, the classic areas associated with multisensory processing are the superior temporal sulcus, the intraparietal cortex, and the frontal cortex, with newer studies confirming multisensory processing also in areas that were previously thought to be mainly unisensory (Ghazanfar and Schroeder, 2006). One sensory input (e.g., touch) can influence how another sensory modality is perceived (e.g., vision) (Driver and Noesselt, 2008); therefore, exposure to multisensory feedback can enhance the ability to detect, discriminate and recognize sensory information (Driver and Noesselt, 2008; Shams and Seitz, 2008; Gentile et al., 2011). For instance, active physical exploration of multisensory stimuli led to greater accuracy in an associative recognition task showing enhanced connectivity between sensory and motor cortices (Butler et al., 2011). Animal studies demonstrated that sensory feedback is crucial in motor learning. Monkeys with an ablated primary sensory hand area had no problems in executing a previously known task but were unable to learn new skills (Pavlides et al., 1993). Providing multisensory stimulation during goal-oriented action execution might help to establish sensorimotor contingencies (McGann, 2010). Muscle vibrations appear to influence the sensorimotor organization, whereas paired associative stimulation with TMS increases motor-evoked-potentials (Rosenkranz and Rothwell, 2006).
Of specific interest for rehabilitation is the integration of visual and proprioceptive information to perform movements. It has been shown that vision and proprioception are weighted differently at various stages during motor planning (Sober and Sabes, 2003), suggesting a target for multisensory manipulations. Concurrent haptic feedback during motor imagery appears to enhance the classification accuracy of brain-computer interfaces when decoding movement intention, indicating that it can aid in closing the sensorimotor loop (Gomez-Rodriguez et al., 2011). Multisensory stimulation training might help patients to recover from unimodal deficits, for instance, visual deficits or auditory localization deficits (Làdavas, 2008).
Rhythmic Cueing
Neuroentrainment encompasses the study of the temporal relationship between the body’s movements and the rhythmic stimulation emerging from the environment. Any sensory modality (auditory, visual, tactile, or vestibular) can be used for entrainment (Ross and Balasubramaniam, 2014). To date, there is not much literature about visual entrainment, possibly because the auditory-motor synchronization appears to be mainly driving internal rhythmic movement control (Ross and Balasubramaniam, 2014). Hence, mainly auditory cues are used to synchronize movements to rhythmic patterns (Rossignol and Jones, 1976; Schaefer, 2014). Rhythmic patterns act like a template whose sequence can be anticipated (Nombela et al., 2013). The regularity detection and tempo tracking of rhythmic patterns increases the activity in motor network areas and cerebellum (Schaefer, 2014) and creates a mental representation of the rhythm, the so-called auditory model, which enables motor movements to anticipate the rhythmic pattern. The pooled evidence provided in the reviews by Grahn (2012) and Nombela et al. (2013) suggests that there are neuronal interactions between auditory and motor systems (Grahn, 2012; Nombela et al., 2013), and auditory-cued motor training can change their mutual structural connectivity (Moore et al., 2017). The auditory-motor action coupling relies on a subcortico-thalamic-cortical circuitry that can be activated through extrinsic cueing (Grahn, 2012; Nombela et al., 2013). Cerebellar patients cannot consciously perceive rhythm changes and show high variable motor responses. However, rhythmic synchronization, respectively, motor entrainment remains intact (Molinari et al., 2003), suggesting that the cerebellum might control the rhythmic auditory-motor synchronization by monitoring rhythmic patterns. Even without cueing, repetitive movements become periodic over time, as observed when analyzing gait patterns. The gait impairment observed in PD is ascribed to a deficiency of the internal timing ability that disturbs coordinated rhythmic locomotion, and which can be improved with rhythmical auditory stimulation (Thaut et al., 1996). Besides, rhythmic somatosensory cueing of stride frequency through vibrotactile stimulation at the wrist could improve qualitative walking performance in PD (van Wegen et al., 2006).
There is evidence that auditorily paced treadmill walking can improve gait coordination in stroke patients as well (Thaut and Abiru, 2010). Further, bilateral arm training with rhythmic auditory cueing enhances functional motor performance, which is maintained long-term (Whitall et al., 2000) and induces cortical and cerebellar changes (Luft et al., 2004). Meta-analyses found large effects that rhythmic auditory cueing improves walking velocity, cadence, and stride length (Yoo and Kim, 2016) and beneficial effects on improving upper limb impairment and function (Ghai, 2018) after stroke.
Explicit Feedback/Knowledge of Results
KR has been defined as verbal, terminal and augmented feedback about goal achievement (Salmoni et al., 1984). Although the finding that extrinsic feedback can effectively create simple stimulus-response associations was brought forward by animal research in reinforcement learning, KR signifies more than just extrinsic rewards (Winstein, 1991; Schmidt and Lee, 2011). KR contributes to learning through cognitive processing, not through conditioning (Salmoni et al., 1984). KR is provided through explicit feedback. Explicit feedback is given on quantitative or qualitative task outcomes, e.g., correctness, exactness, success, or failure (Mazzoni and Krakauer, 2006; Subramanian et al., 2010; Schmidt and Lee, 2011). This feedback does not have to be verbal. For instance, when failing to reach for a target, the subject can hear unpleasant tones or see that the failed targets change color (Taylor et al., 2014). Also, explicit feedback about kinematic outcomes can be KR, e.g., playing back a recorded movement after execution. However, this feedback supports learning only if the movement features that led to the outcome are pointed out to the subject (Salmoni et al., 1984). Explicit feedback seems to activate explicit learning mechanisms and shows only subtle effects on implicit learning mechanisms (Taylor et al., 2014). While implicit learning appears to increase the cortical motor output maps of the involved movement initially, they return to baseline topography once the learned content can be explicitly declared. Possibly through explicit feedback a global motor plan is learned that is represented by higher-order neuronal networks, which influence the cortical sensorimotor representations differently (Pascual-Leone et al., 1994). Rewarding or punishing feedbacks appear to have dissociative effects on skilled motor learning. Punishment can speed up motor learning, whereas rewards ensure long-term retention (Abe et al., 2011; Galea et al., 2015). The reinforcement of positive outcomes appears to foster a success-driven learning system, which limits decay after learning, possibly by mobilizing the dopaminergic system (Wickens et al., 2003). Reward expectations modulate the activity of caudate neurons (striatal projection neurons), which receive reward-related information through the dopaminergic input from substantia nigra and spatial information through the cortico-striatal connection. Consequently, they modulate the inhibitory output of the basal ganglia, biasing attention to rewarded items. Either reward-driven activity of caudate neurons is a result of cerebral plasticity, or activity in the cerebral cortex is influenced by caudate neurons through the output nuclei of basal ganglia (Kawagoe et al., 1998). Dopamine has a gradual build-up and can persist for longer time courses; it might support long-term memory formation of motor actions (Abe et al., 2011).
KR has been used to reinforce adherence to CIMT (Taub et al., 1994). Meta-analyses often analyze KR together with KP under the umbrella term augmented feedback (van Dijk et al., 2005; Hayward et al., 2014). A meta-analysis analyzing different feedback types reported positive effects on motor function for KR (Molier et al., 2010). However, this evidence is based on one study (Eckhouse et al., 1990), whose intervention included other principles as well. It can, therefore, not be established whether KR is effective for motor recovery.
Implicit Feedback/Knowledge of Performance
KP was defined as feedback given about movement execution in the form of verbal descriptions, demonstrations, or replays of recordings (Gentile, 1972). Advances in technology made it possible that KP can be delivered online, in an implicit manner and concurrent during movement execution, providing verbal or non-verbal feedback about ongoing intrinsic somatic processes and movement kinematics (Salmoni et al., 1984; Winstein, 1991). For instance, feedback in the form of sounds and colors can be given while trunk displacements surpass a threshold (Subramanian et al., 2007). Biofeedback uses physiological sources like electromyograms to provide patients with real-time visual or auditory signals about their motor activity (Huang et al., 2006). Ultimately arm movements can be visualized and augmented using VR representations (Ballester Rubio et al., 2015b; Ferreira dos Santos et al., 2016).
Implicit sensory feedback enhances learning from sensorimotor prediction errors, which for instance can aid the adaptation to unexpected perturbations (Shadmehr et al., 2010), possibly by contributing to implicit learning mechanisms (Taylor et al., 2014). Concurrent implicit feedback leads to lasting adaptations to visuomotor rotations, which are not (Hinder et al., 2008) or less observed (Taylor et al., 2014) when feedback about movement outcome, e.g., KR is given. Although KP appears to be beneficial during training, there is evidence that subjects can become dependent on it, showing inferior performance when feedback is removed (Ronsse et al., 2011). Ronsse et al. (2011) compared the effects of providing concurrent visual to concurrent auditory feedback during the acquisition of a bimanual movement pattern. The authors found that subjects that had obtained visual KP showed poorer performance during retention testing than subjects that were given auditory KP. During acquisition, the visual feedback increased the activity in vision/sensorimotor-specific areas, which was maintained during retention testing even in the absence of feedback. On the contrary, the concurrent auditory feedback reduced the activity in temporo-parieto-frontal areas and deactivated task-specific sensory areas during retention testing without feedback. These results suggest that subjects can become dependent on concurrent visual feedback, but not on concurrent auditory feedback because they rely on sensory processing areas that have become tuned to visual information during practice. The auditory feedback, on the other hand, might foster the formation of an internal controller, evidenced by the stronger activation of prefrontal areas. Alternatively, auditory feedback might promote reliance on proprioception and is consequently ignored during training (Ronsse et al., 2011). Results from cerebellar patients that were exposed to force-field learning tasks propose that the cerebellum may play an important role in using implicit information to correct and adapt motor commands to changed limb dynamics, and in forming internal controllers (Nezafat et al., 2001; Smith and Shadmehr, 2005; Tseng et al., 2007). In contrast to explicit error signals mediated through midbrain dopamine neurons in basal ganglia, implicit sensorimotor errors are possibly encoded by cerebellar climbing fibers and manifest in complex spikes in Purkinje cells during reaching tasks (Kitazawa et al., 1998). Computational modeling of adaptation to visuomotor rotations following concurrent visual feedback points to narrowly tuned neurons in the cortex that are driven by a prediction error that is computed by the cerebellum (Tanaka et al., 2009).
Stroke patients experienced a significant recovery in motor function and showed increased activation in the ipsilesional primary sensorimotor cortex after 4 weeks of training with a VR system that provided them with implicit feedback about their upper-limb movement (Jang et al., 2005). However, the system also included several other principles. In addition, the provision of KP has been shown to recover impaired movement patterns (Cirstea and Levin, 2007), to reduce learned non-use (Ballester Rubio et al., 2015b), and to lead to longer-lasting recovery effects (Subramanian et al., 2010). A meta-analysis found a beneficial effect for KP on motor function (Molier et al., 2010); however, the effect was based on two studies only.
Modulate Effector Selection
In the acute stage after stroke, patients typically suppress the use of the affected limb due to pain, weakness, or malfunctioning (Taub and Uswatte, 2003). As a consequence, they are prone to overuse the non-paretic limb, and the resulting under-usage of the impaired limb can cause a loss of behavioral and neuronal function (Andrews and Stewart, 1979; Taub et al., 2006). Some authors argue that this compensation strategy, called learned non-use, emerges because the spontaneous use of the paretic limb does not cross a threshold level (Han et al., 2008). Although standard therapy focuses on improving the functionality of the impaired limb, the improvement does not transfer to increased use of the arm for ADLs (Smania et al., 2012; Kwakkel et al., 2015).
Of those therapeutic approaches that were successful in counteracting learned non-use CIMT is the most common and most successful one (Kwakkel et al., 2015). An fMRI study revealed changes in brain activity patterns due to paretic arm use in patients that underwent a 2 weeks CIMT program at home where the non-affected arm was constrained for 90% of the waking time. Increased grip strength in the affected limb correlated significantly with increased fMRI signal change in ipsilesional cortico-cerebellar areas (Johansen-Berg et al., 2002). However, a meta-analysis did not find a pooled effect that forcing the use of the paretic arm alone is effective (Hayward et al., 2014). Other approaches aimed at promoting paretic arm-use through positive reinforcement during bilateral arm training (Ballester Rubio et al., 2016) or through wearable devices (e.g., bracelets) that provide feedback about performance of ADLs (Ballester Rubio et al., 2015a).
Action Observation/Embodied Practice
Action observation (Martens et al., 1976) gained increased attention after the discovery of mirror neurons (Rizzolatti and Sinigaglia, 2010): in monkeys, some neurons discharged not only when the animal executed a motor command but also when it observed another individual executing it. In humans, subjects who first observed other individuals performing a novel task performed better in the same task than control subjects that did not observe other individuals or observed a slightly different task (Mattar and Gribble, 2005). It is thought that in monkeys, as in humans, action observation relies on the frontoparietal network (Rizzolatti and Sinigaglia, 2010). Indeed, a meta-analysis showed that in humans, movement observation, as well as movement execution, recruits mainly the premotor and parietal areas. Movement observation, however, exclusively activated the visual cortex, whereas execution activated the primary motor cortex (Hardwick et al., 2018). Therefore, action observation might facilitate movement execution and motor learning by facilitating the excitability of the motor system (Mulder, 2007). Indeed, TMS during action observation elicited increased muscle activation patterns (Fadiga et al., 1995). For practical reasons, action observation could be especially beneficial for stroke patients with severe hemiparesis or complete paralysis. There is some clinical evidence that action observation therapy can reduce impairment and increase brain activation in the frontoparietal network and bilateral cerebellum (Ertelt et al., 2007).
Besides internalizing someone else’s movement, humans can also ascribe ownership and agency to body parts that do not pertain to them (Botvinick and Cohen, 1998). The discovery of rubber hand illusions (Botvinick and Cohen, 1998) led to insights about the mechanisms underlying agency. Both the sense of agency (Sato and Yasuda, 2005) and ownership are susceptible to manipulations (Slater et al., 2010), that have been used for therapeutic purposes, for instance, in mirror therapy (Ramachandran and Rogers-Ramachandran, 1996). Similar to action observation, mirror therapy appears to rely on the frontoparietal circuit (Harmsen et al., 2015), which is why its motor learning effects are partly explained by the same mechanisms (Hamzei et al., 2012). However, contrary to movement observation, mirror therapy robustly activates the primary motor cortex and visual processing areas ipsilateral to the mirrored movement. Also, mirror therapy seems to increase functional connectivity between cortical motor areas and to excite the neural connection between the two hemispheres (Hamzei et al., 2012; Arya, 2016). A meta-analysis attests mirror therapy a significant long-term effect on motor function, the ADLs, the reduction of pain and the reduction of visuospatial neglect (Thieme et al., 2012).
If the impairment of the limb impedes active movement, visual illusions could be presented to the patients to simulate movements with the paretic arm. The error-prediction mechanism driven by the cerebellum could be equally activated through the alternative representation (Fiorio et al., 2014). Possibly, the stronger the visual illusion, the more agency is ascribed to it, which could explain the difference in brain activation patterns between action observation and mirror therapy. The sense of agency seems to be important when learning from sensorimotor prediction errors (Tsakiris et al., 2007), respectively agency is reduced when prediction and outcome do not match (Sato and Yasuda, 2005). However, there is no consensus on the definition of ownership and agency, which makes their operationalization in clinical practice difficult.
Mental Practice/Motor Imagery
Mental practice and motor imagery rely on the ability to simulate actions mentally without overt behavior, as summarized by the simulation theory (Jeannerod, 2001). Motor imagery can be seen as a mental rehearsal of future movements and motor plans (Naito et al., 2002; Schmidt and Lee, 2011), that can be beneficial for motor learning (Di Rienzo et al., 2016). However, actual physical practice shows superior effects on learning (Hird et al., 1991). A meta-analysis compared the brain areas that are active during mental imagery and movement execution. Both seem to recruit premotor areas, somatosensory cortex, and subcortical areas. Also, activation in the mid-cingulate cortex was found, with motor imagery activating more the anterior region that is linked to the cognitive aspects of motor control, whereas motor execution recruiting more the posterior region that is associated with basic motor functions. While motor imagery appears to activate more the parietal cortex, movement execution appears to recruit more classic sensorimotor regions like the primary motor cortex and cingulate motor areas (Hardwick et al., 2018). These findings are in line with studies showing that lesions in the frontoparietal system can diminish the ability of motor imagery (Johnson, 2000; Danckert et al., 2002). Motor imagery and physical practice also appear to induce similar learning-dependent brain changes (Di Rienzo et al., 2016). Not surprisingly, the activation pattern of motor imagery appears to be similar to the one identified in action observation and mirror therapy.
The learning effects of motor imagery and mental practice have been extensively studied in sports, whereas research regarding their clinical efficacy and efficiency is sparse and relatively recent (Mulder, 2007). However, motor imagery is thought to be advantageous for stroke recovery, especially for severely impaired patients (Mulder, 2007). Since patients retain the ability to imagine movements with the paretic limb, mental motor practice might facilitate functional reorganization (Johnson, 2000). A meta-analysis looking into the effectiveness of mental practice also found some trends for positive outcomes. However, pooled effects could not be estimated because only a few Class I studies exist, and their protocols, measurements, and interventions vary widely (Braun et al., 2006).
Social Interaction
Social interaction has been defined as a behavior in which the participants’ actions are both a response to and a stimulus for the counterpart’s behavior (Rubin et al., 2006). Many ADL implicate social interaction, and a failure to perform them might lead to an undesired dependence on others (Lilja et al., 2003). The level of self-efficacy influences motor skill performance and learning, and in turn, is influenced by the appraisal or discouragement from others (Wulf et al., 2012). fMRI recordings of a subject experiencing a live social interaction revealed activations in areas commonly identified in the perception of social cues besides other regions involved in goal-directed and visual attention as well as reward processing (Redcay et al., 2010).
Animals that are allowed social interaction when recovering from an artery occlusion show higher functional improvement (Johansson and Ohlsson, 1996), increased recovery of behavior, and lower mortality, especially if the interaction partner was healthy (Venna et al., 2014). Including and investigating the impact of social interaction as part of the rehabilitation experience seems an important but missed opportunity. We found no study that was evaluating this specific aspect in a randomized controlled trial. One study evaluating enriched environments that included social interaction found positive results in terms of activity (Janssen et al., 2014).
Discussion
This synthesis aimed at identifying a set of principles that should guide the design of effective neurorehabilitation protocols for post-stroke recovery. We identified 15 principles based on existing work on motor learning and recovery: massed practice/repetitive practice, spaced practice, dosage/duration, task-specific practice, task-oriented practice, variable practice, increasing difficulty, multisensory information, rhythmic cueing, explicit feedback/knowledge of results, implicit feedback/knowledge of performance, modulate effector selection, action observation/embodied practice, mental practice, and social interaction. Where possible, we identified the therapeutic and neurological effects of these principles from experimental work and clinical studies and commented on their limitations.
Our motivation for this analysis is twofold. Firstly, we are confident that the quality of evidence from clinical work and its interpretation would be enhanced if interventions are described along with the included principles. Reviews or meta-analyses with ambiguous effects often state that the included protocols remained vague on the exact experience provided to the patients, which makes the comparison and interpretation difficult (Veerbeek et al., 2014; Renton et al., 2017). By focusing solely on the ingredients of therapeutic interventions and compiling their current neuroscientific evidence, we aim to raise awareness of their importance. Also, this work might serve as a guide for clinicians and researchers to construct or identify the active ingredients in their interventions and to discover evidence currently missing. Secondly, we believe that there is a need to create a link between the principles of motor learning and their current operationalization in clinical studies and practice. We have identified several difficulties and shortcomings that do not aid in obtaining a common understanding of these principles and hence complicate the clinical investigation.
It seems that many principles are poorly operationalized in clinical trials. For instance, when massed practice is investigated, the repetitions performed within a session and during the treatment duration are rarely quantified (Lang et al., 2007) such that recovery effects due exclusively to repetition cannot be singled out. Also, the clinical research of spaced practice and dosage/duration would benefit if the parameters were quantified in a standardized way. Particularly dosage should be explicitly described in treatment minutes per session in order to be able to establish a dose-response due to training (Dobkin, 2005). Furthermore, dosage/duration should not be equated with intensity since the intensiveness of training cannot be estimated through treatment minutes only (Billinger, 2015). Intensity should be an independent principle that needs to be investigated separately. Task-specific and goal-oriented practice appear to be often used interchangeably (Winstein et al., 2014; Fu et al., 2015; Yue et al., 2017) although their training target is different. While task-specific practice focuses on the acquisition of a specific skill (Keetch et al., 2005) for ADL, goal-oriented practice permits the use of any movement or skill that is deemed suitable to achieve the goal (Horak, 1991), fostering the exploration of alternative movement patterns. Variability appears to be included inherently in many protocols (Darekar et al., 2015), possibly because it renders the training less repetitive and, therefore, less boring (Birkenmeier et al., 2010), which could counteract low adherence. However, this link has not been explicitly studied. Increasing the difficulty during practice is part of many intervention protocols as well; however, personalizing the difficulty level in order to provide training at the optimal challenge point seems to be rarely addressed. Concerning multisensory integration, it would be interesting to explore whether the presence of more than two sensory stimulations could enhance learning (Sánchez et al., 2013). Similarly, rhythmic entrainment could be extended with protocols exploring if visual or haptic entrainment might aid recovery of impaired movements (Penhune et al., 1998). Explicit feedback and implicit feedback are often investigated together under the umbrella term of augmented feedback, as evidenced by the sourced meta-analysis and clinical studies (Molier et al., 2010). However, their aim and the neuronal mechanisms that they appear to stimulate are different. While explicit feedback provides terminal feedback about movement outcome, implicit feedback provides concurrent error-signals during movement execution fostering possibly different learning mechanisms. Meta-analyses also appear to interpret the sensory modality of the feedback, e.g., if it is visual, auditory or haptic as a feedback type. However, the sensory modality is a separate layer that is added to feedback. Explicit feedback, as well as implicit feedback, can be unisensory or multisensory. Action observation and mirror-therapy appear to be well studied therapeutic ingredients, whereas mental practice is only addressed in a few studies, and social interaction remains unexplored territory so far. If the principles would be better operationalized, it would not only help to identify their contribution to the recovery of motor functions, but also other learning outcomes such as cognitive or language improvements.
The neuronal changes found within each principle allow us to draw some general conclusions for the advancement of neurorehabilitation. While some principles appear to modulate more specific brain areas (massed practice, dosage, variable practice, task-specific practice, modulate effector selection, multisensory stimulation) within the motor areas of the cortex others appear to recruit or rely more on networks of brain regions (goal-oriented practice, increasing difficulty, action observation, motor imagery, mirror therapy, rhythmic cueing, implicit feedback/knowledge of results, social interaction). An effective rehabilitation approach should thus incorporate principles of both types in order to counteract neuronal degradation and promote improvement. Firstly, a training that addresses only a limited subset of the neuronal circuitry underlying a general function might limit transfer to other behaviors that depend on the same circuitry (Kleim and Jones, 2008). Secondly, not all principles are equally applicable to all patients. Some principles might be more beneficial early after stroke, whereas others benefit patients with less severe damage. Spontaneous biological recovery and activity-dependent plasticity appear to interact differently at different stages after stroke, which, aside from other factors like severity, predicts recovery (Reinkensmeyer et al., 2016; Hylin et al., 2017). It seems that in acute patients the sensorimotor cortex activity is highly abnormal, and the normalization in activity patterns is linked to better recovery (Schaechter, 2004). Principles like task-oriented practice that promote localized changes, might therefore be more beneficial at the acute stage after stroke (Schaechter, 2004), whereas therapies like CIMT, where the forced use of the impaired limb is paired with increasing difficulty and further principles, have been shown to be more suitable at later stages after stroke and for less impaired patients (Dromerick et al., 2009). More severely impaired patients, on the other hand, might benefit from action observation, mirror therapy and motor imagery (Dohle et al., 2009; Sun et al., 2013). Future studies will show the optimal combinations of principles that stimulate plasticity in a way that learning of preexisting or novel functions is enhanced.
We are aware that the view proposed here is strongly influenced by knowledge mainly derived from clinical work with hemiparetic stroke patients. However, the literature indicates that other diseases, for instance, PD (Rossiter et al., 2014) or Alzheimer’s disease (Kalaria, 2002), show similar cognitive, functional, and neuronal alterations even though they may have different pathologies. Therefore, these principles of neurorehabilitation could be potentially applied beyond the field of stroke. As our main goal was to provide a synthesis that is informative and practical, in-depth analysis of each principle and its neurological underpinnings lie outside of the scope of this work. In future work, we will unify the principles addressed here in a theoretical framework to show how each of them contributes to the restoration of sensorimotor contingencies (Verschure, 2011).
In summary, our review provides a synthesis of effective therapeutic ingredients that could be beneficial in aiding recovery after stroke. We hope that future work will extend the evidence presented here by implementing and investigating the principles of neurorehabilitation in novel rehabilitation protocols for stroke and other patient populations.
Author Contributions
MM, BB, and PV contributed to the design of the review. MM wrote the first draft of the manuscript and performed the computerized search. All authors contributed to the manuscript revision, read and approved the submitted version.
Funding
This study was supported by EIT Health ID 19277 (RGS@HOME) under H2020, SANAR (MINECO, TIN2013- 44200), cDAC (ERC 2013 ADG 341196), and socSMCs (Grant Number EC, H2020-641321).
Conflict of Interest
PV is the founder and interim CEO of Eodyne Systems S.L., which aims at bringing scientifically validated neurorehabilitation technology to society.
The remaining authors declare that the research was conducted in the absence of any commercial or financial relationships that could be construed as a potential conflict of interest.
Abbreviations
ADLs, activities of daily living; CIMT, constraint-induced movement therapy; fMRI, functional magnetic resonance imaging; KR, knowledge of results; KP, knowledge of performance; LTP, long-term potentiation; PD, Parkinson’s disease; TMS, transcranial magnetic stimulation; VR, virtual reality.
References
Abe, M., Schambra, H., Wassermann, E. M., Luckenbaugh, D., Schweighofer, N., and Cohen, L. G. (2011). Reward improves long-term retention of a motor memory through induction of offline memory gains. Curr. Biol. 21, 557–562. doi: 10.1016/j.cub.2011.02.030
Ammons, R. B. (1947). Acquisition of motor skill: I. Quantitative analysis and theoretical formulation. Psychol. Rev. 54, 263–281. doi: 10.1037/h0062455
Ammons, R. B., and Willig, L. (1956). Acquisition of motor skill: IV. Effects of repeated periods of massed practice. J. Exp. Psychol. 51, 118–126. doi: 10.1037/h0048337
Andrews, K., and Stewart, J. (1979). Stroke recovery: he can but does he? Rheumatol. Rehabil. 18, 43–48. doi: 10.1093/rheumatology/18.1.43
Andrieux, M., Danna, J., and Thon, B. (2012). Self-Control of task difficulty during training enhances motor learning of a complex coincidence-anticipation task. Res. Q. Exerc. Sport 83, 27–35. doi: 10.1080/02701367.2012.10599822
Arya, K. N. (2016). Underlying neural mechanism of mirror therapy: implications for motor rehabilitation in stroke. Neurol. Individ. 64, 38–44. doi: 10.1056/NEJMe0902377
Aston-Jones, G., and Cohen, J. D. (2005). An integrative theory of locus Coeruleus-Norepinephrine function: adaptive gain and optimal performance. Annu. Rev. Neurosci. 28, 403–450. doi: 10.1146/annurev.neuro.28.061604.135709
Ballester Rubio, B., Lathe, A., Duarte, E., Duff, A., and Verschure, P. F. M. J. (2015a). “A wearable bracelet device for promoting arm use in stroke patients,” in Proceedings of the 3rd International Congress on Neurotechnology, Electronics and Informatics (NEUROTECHNIX 2015), Portugal, 24–31. doi: 10.5220/0005662300240031
Ballester Rubio, B., Oliva, L. S., Duff, A., and Verschure, P. F. M. J. (2015b). “Accelerating motor adaptation by virtual reality based modulation of error memories,” in Proceedings of the 2015 IEEE International Conference on Rehabilitation Robotics (ICORR), Singapore, 623–629. doi: 10.1109/ICORR.2015.7281270
Ballester Rubio, B., Maier, M., San Segundo, M. R., Castañeda, V., Duff, A., and Verschure, P. F. M. J. (2016). Counteracting learned non-use in chronic stroke patients with reinforcement-induced movement therapy. J. Neuroeng. Rehabil. 13, 1–15. doi: 10.1186/s12984-016-0178-x
Basso, D. M., and Lang, C. E. (2017). Consideration of dose and timing when applying interventions after stroke and spinal cord injury. J. Neurol. Phys. Ther. 41, 1–19. doi: 10.1097/NPT.0000000000000165.Consideration
Battig, W. F. (1966). “Facilitation and interference,” in Acquisition of Skill, ed. E. A. Bilodeau (New York, NY: Academic Press).
Billinger, S. A. (2015). Does aerobic exercise and the FITT principle fit into stroke. Curr. Neurol. Neurosci. Rep. 15, 1–14. doi: 10.1007/s11910-014-0519-8
Birkenmeier, R. L., Prager, E. M., and Lang, C. E. (2010). Translating animal doses of task-specific training to people with chronic stroke in 1-hour therapy sessions: a proof-of-concept study. Neurorehabil. Neural Repair 24, 620–635. doi: 10.1177/1545968310361957
Bosch, J., O’Donnell, M. J., Barreca, S., Thabane, L., and Wishart, L. (2014). Does task-oriented practice improve upper extremity motor recovery after stroke? A systematic review. ISRN Stroke 2014, 1–10. doi: 10.1155/2014/504910
Botvinick, M., and Cohen, J. (1998). Rubber hands ‘feel’ touch that eyes see. Nature 391:756. doi: 10.1016/0005-2736(86)90402-5
Boyd, L. A., Vidoni, E. D., and Wessel, B. D. (2010). Motor learning after stroke: is skill acquisition a prerequisite for contralesional neuroplastic change? Neurosci. Lett. 482, 21–25. doi: 10.1016/j.neulet.2010.06.082
Braun, S. M., Beurskens, A. J., Borm, P. J., Schack, T., and Wade, D. T. (2006). The effects of mental practice in stroke rehabilitation: a systematic review. Arch. Phys. Med. Rehabil. 87, 842–852. doi: 10.1016/j.apmr.2006.02.034
Brown, M. W., Wilson, F. A. W., and Riches, I. P. (1987). Neuronal evidence that inferomedial temporal cortex is more important than in certain processes underlying recognition memory. Brain Res. 409, 158–162. doi: 10.1016/0006-8993(87)90753-0
Butler, A. J., James, T. W., and James, K. H. (2011). Enhanced multisensory integration and motor reactivation after active motor learning of audiovisual associations. J. Cogn. Neurosci. 23, 3515–3528. doi: 10.1162/jocn_a_00015
Cameirão, M. S., Badia, S. B., Duarte, E., Frisoli, A., and Verschure, P. F. M. J. (2012). The combined impact of virtual reality neurorehabilitation and its interfaces on upper extremity functional recovery in patients with chronic stroke. Stroke 43, 2720–2728. doi: 10.1161/STROKEAHA.112.653196
Carter, A. R., Connor, L. T., and Dromerick, A. W. (2010). Rehabilitation after stroke: current state of the science. Curr. Neurol. Neurosci. Rep. 10, 158–166. doi: 10.1007/s11910-010-0091-9.Rehabilitation
Censor, N., and Cohen, L. G. (2011). Using repetitive transcranial magnetic stimulation to study the underlying neural mechanisms of human motor learning and memory. J. Physiol. 589, 21–28. doi: 10.1113/jphysiol.2010.198077
Cepeda, N. J., Pashler, H., Vul, E., Wixted, J., and Rohrer, D. (2006). Distributed practice in verbal recall tasks: a review and quantitative synthesis. Psychol. Bull. 132, 354–380. doi: 10.1037/0033-2909.132.3.354
Cirstea, M. C., and Levin, M. F. (2007). Improvement of arm movement patterns and endpoint control depends on type of feedback during practice in stroke survivors. Neurorehabil. Neural Repair 21, 398–411. doi: 10.1177/1545968306298414
Cohen, D. A., Pascual-Leone, A., Press, D. Z., and Robertson, E. M. (2005). Off-line learning of motor skill memory: a double dissociation of goal and movement. Proc. Natl. Acad. Sci. U.S.A. 102, 18237–18241. doi: 10.1073/pnas.0506072102
Danckert, J., Ferber, S., Doherty, T., Steinmetz, H., Nicolle, D., and Goodale, M. A. (2002). Selective, non-lateralized impairment of motor imagery following right parietal damage. Neurocase 8, 194–205. doi: 10.1093/neucas/8.3.194
Darekar, A., McFadyen, B. J., Lamontagne, A., and Fung, J. (2015). Efficacy of virtual reality-based intervention on balance and mobility disorders post-stroke: a scoping review. J. Neuroeng. Rehabil. 12, 1–14. doi: 10.1186/s12984-015-0035-3
Dettmers, C., Teske, U., Hamzei, F., Uswatte, G., Taub, E., and Weiller, C. (2005). Distributed form of constraint-induced movement therapy improves functional outcome and quality of life after stroke. Arch. Phys. Med. Rehabil. 86, 204–209. doi: 10.1016/j.apmr.2004.05.007
Di Rienzo, F., Debarnot, U., Daligault, S., Saruco, E., Delpuech, C., Doyon, J., et al. (2016). Online and offline performance gains following motor imagery practice: a comprehensive review of behavioral and neuroimaging studies. Front. Hum. Neurosci. 10:315. doi: 10.3389/fnhum.2016.00315
Dobkin, B. H. (2004). Strategies for stroke rehabilitation. Lancet Neurol. 3, 528–536. doi: 10.1016/S1474-4422(04)00851-8
Dobkin, B. H. (2005). Rehabilitation and functional neuroimaging dose-response trajectories for clinical trials. Neurorehabil. Neural Repair 19, 276–282. doi: 10.1177/1545968305281892
Dohle, C., Püllen, J., Nakaten, A., Küst, J., Rietz, C., and Karbe, H. (2009). Mirror therapy promotes recovery from severe hemiparesis: a randomized controlled trial. Neurorehabil. Neural Repair 23, 209–217. doi: 10.1177/1545968308324786
Driver, J., and Noesselt, T. (2008). Multisensory interplay reveals crossmodal influences on “Sensory-Specific” brain regions, neural responses, and judgments. Neuron 57, 11–23. doi: 10.1016/j.neuron.2007.12.013
Dromerick, A. W., Lang, C. E., Birkenmeier, R. L., Wagner, J. M., Miller, J. P., Videen, T. O., et al. (2009). Very early constraint-induced movement during stroke rehabilitation (VECTORS). Neurology 73, 195–201. doi: 10.1212/WNL.0b013e3181ab2b27
Eckhouse, R. H., Morash, R. P., and Maulucci, R. A. (1990). Sensory feedback and the impaired motor system. J. Med. Syst. 14, 93–105. doi: 10.1007/BF00996909
Ertelt, D., Small, S., Solodkin, A., Dettmers, C., McNamara, A., Binkofski, F., et al. (2007). Action observation has a positive impact on rehabilitation of motor deficits after stroke. Neuroimage 36, 164–173. doi: 10.1016/j.neuroimage.2007.03.043
Fadiga, L., Fogassi, L., Pavesi, G., and Rizzolatti, G. (1995). Motor facilitation during action observation: a magnetic stimulation study. J. Neurophysiol. 73, 2608–2611. doi: 10.1152/jn.1995.73.6.2608
Ferreira dos Santos, L., Christ, O., Mate, K., Schmidt, H., Krüger, J., and Dohle, C. (2016). Movement visualisation in virtual reality rehabilitation of the lower limb: a systematic review. Biomed. Eng. Online 15, 75–88. doi: 10.1186/s12938-016-0289-4
Fiorio, M., Mariotti, C., Panzeri, M., Antonello, E., Classen, J., and Tinazzi, M. (2014). The role of the cerebellum in dynamic changes of the sense of body ownership: a study in patients with Cerebellar degeneration. J. Cogn. Neurosci. 24, 712–721. doi: 10.1162/jocn
French, B., Thomas, L. H., Coupe, J., McMahon, N. E., Connell, L., Harrison, J., et al. (2016). Repetitive task training for improving functional ability after stroke (Review). Cochrane Database Syst. Rev. 11, CD006073.
Fu, M. J., Knutson, J., and Chae, J. (2015). Stroke rehabilitation using virtual environments. Phys. Med. Rehabil. Clin. N. Am. 26, 747–757. doi: 10.1016/j.pmr.2015.06.001.Stroke
Furlan, L., Conforto, A. B., Cohen, L. G., and Sterr, A. (2016). Upper limb immobilisation: a neural plasticity model with relevance to poststroke motor rehabilitation. Neural Plast. 2016:8176217. doi: 10.1155/2016/8176217
Galea, J. M., Mallia, E., Rothwell, J., and Diedrichsen, J. (2015). The dissociable effects of punishment and reward on motor learning. Nat. Neurosci. 18, 597–602. doi: 10.1038/nn.3956
Gallivan, J. P., and Culham, J. C. (2015). Neural coding within human brain areas involved in actions. Curr. Opin. Neurobiol. 33, 141–149. doi: 10.1016/j.conb.2015.03.012
Gauggel, S., and Fischer, S. (2001). The effect of goal setting on motor performance and motor learning in brain-damaged patients. Neuropsychol. Rehabil. 11, 33–44. doi: 10.1080/09602010042000150
Gendolla, G. H. E. (1999). Self-relevance of performance, task difficulty, and task engagement assessed as cardiovascular response. Motiv. Emot. 23, 45–66. doi: 10.1023/A:1021331501833
Gentile, A. M. (1972). A working model of skill acquisition with application to teaching. Quest 17, 3–23. doi: 10.1080/00336297.1972.10519717
Gentile, G., Petkova, V. I., and Ehrsson, H. H. (2011). Integration of visual and tactile signals from the hand in the human brain?: an fMRI study. J Neurophysiol. 105, 910–922. doi: 10.1152/jn.00840.2010
Gerbier, E., and Toppino, T. C. (2015). The effect of distributed practice: neuroscience, cognition, and education. Trends Neurosci. Educ. 4, 49–59. doi: 10.1016/j.tine.2015.01.001
Ghai, S. (2018). Effects of real-time (sonification) and rhythmic auditory stimuli on recovering arm function post stroke: a systematic review and meta-analysis. Front. Neurol. 9:488. doi: 10.3389/fneur.2018.00488
Ghazanfar, A. A., and Schroeder, C. E. (2006). Is neocortex essentially multisensory? Trends Cogn. Sci. 10, 278–285. doi: 10.1016/j.tics.2006.04.008
Gomez-Rodriguez, M., Peters, J., Hill, J., Schölkopf, B., Gharabaghi, A., and Grosse-Wentrup, M. (2011). Closing the sensorimotor loop: haptic feedback facilitates decoding of motor imagery. J. Neural Eng. 8:036005. doi: 10.1088/1741-2560/8/3/036005
Grahn, J. A. (2012). Neural mechanisms of rhythm perception: current findings and future perspectives. Top. Cogn. Sci. 4, 585–606. doi: 10.1111/j.1756-8765.2012.01213.x
Guadagnoli, M. A., and Lee, T. D. (2004). Challenge point: a framework for conceptualizing the effects of various practice conditions in motor learning. J. Mot. Behav. 36, 212–224. doi: 10.3200/JMBR.36.2.212-224
Gur, R. C., Turetsky, B. I., Loughead, J., Waxman, J., Snyder, W., Ragland, J. D., et al. (2007). Hemodynamic responses in neural circuitries for detection of visual target and novelty: an event-related fMRI study. Hum. Brain Mapp. 28, 263–274. doi: 10.1002/hbm.20319
Hamzei, F., Läppchen, C. H., Glauche, V., Mader, I., Rijntjes, M., and Weiller, C. (2012). Functional plasticity induced by mirror training: the mirror as the element connecting both hands to one hemisphere. Neurorehabil. Neural Repair 26, 484–496. doi: 10.1177/1545968311427917
Han, C. E., Arbib, M. A., and Schweighofer, N. (2008). Stroke rehabilitation reaches a threshold. PLoS Comput. Biol. 4:e1000133. doi: 10.1371/journal.pcbi.1000133
Hardwick, R. M., Caspers, S., Eickhoff, S. B., and Swinnen, S. P. (2018). Neural correlates of action: comparing meta-analyses of imagery, observation, and execution. Neurosci. Biobehav. Rev. 94, 31–44. doi: 10.1016/j.neubiorev.2018.08.003
Harmsen, W. J., Bussmann, J. B. J., Selles, R. W., Hurkmans, H. L. P., and Ribbers, G. M. (2015). A mirror therapy-based action observation protocol to improve motor learning after stroke. Neurorehabil. Neural Repair 29, 509–516. doi: 10.1177/1545968314558598
Harvey, R. L. (2009). Improving poststroke recovery: neuroplasticity and task-oriented training. Curr. Treat. Options Cardiovasc. Med. 11, 251–259. doi: 10.1007/s11936-009-0026-4
Hasan, S. M. M., Rancourt, S. N., Austin, M. W., and Ploughman, M. (2016). Defining optimal aerobic exercise parameters to affect complex motor and cognitive outcomes after stroke?: a systematic review and synthesis. Neural Plast. 2016, 1–12. doi: 10.1155/2016/2961573
Hasselmo, M. E., Wyble, B. P., and Wallenstein, G. V. (1996). Encoding and retrieval of episodic memories: role of cholinergic and GABAergic modulation in the hippocampus. Hippocampus 6, 693–708. doi: 10.1002/(sici)1098-1063(1996)6:6<693::aid-hipo12>3.0.co;2-w
Hauptmann, B., Reinhart, E., Brandt, S. A., and Karni, A. (2005). The predictive value of the leveling off of within-session performance for procedural memory consolidation. Cogn. Brain Res. 24, 181–189. doi: 10.1016/j.cogbrainres.2005.01.012
Hayward, K. S., Barker, R. N., Carson, R. G., and Brauer, S. G. (2014). The effect of altering a single component of a rehabilitation programme on the functional recovery of stroke patients: a systematic review and meta-analysis. Clin. Rehabil. 28, 107–117. doi: 10.1177/0269215513497601
Henson, R., Shallice, T., and Dolan, R. (2000). Neuroimaging evidence for dissociable forms of repetition priming. Science 287, 1269–1272. doi: 10.1126/science.287.5456.1269
Henson, R. N., Rylands, A., Ross, E., Vuilleumeir, P., and Rugg, M. D. (2004). The effect of repetition lag on electrophysiological and haemodynamic correlates of visual object priming. Neuroimage 21, 1674–1689. doi: 10.1016/j.neuroimage.2003.12.020
Henson, R. N. A. (2003). Neuroimaging studies of priming. Prog. Neurobiol. 70, 53–81. doi: 10.1016/S0301-0082(03)00086-8
Hinder, M. R., Tresilian, J. R., Riek, S., and Carson, R. G. (2008). The contribution of visual feedback to visuomotor adaptation: how much and when? Brain Res. 1197, 123–134. doi: 10.1016/j.brainres.2007.12.067
Hird, J. S., Landers, D. M., Thomas, J. R., and Horan, J. J. (1991). Physical practice is superior to mental practice in enhancing cognitive and motor task performance. J. Sport Exerc. Psychol. 8, 281–293. doi: 10.1123/jsep.13.3.281
Horak, F. B. (1991). “Assumptions underlying motor control for neurologic rehabilitation,” in Proceedings of the Contemporary Management of Motor Problems: Proceedings of the II Step Conference, (Alexandria, VA: Foundation for Physical Therapy), 11–27.
Huang, H., Wolf, S. L., and He, J. (2006). Recent developments in biofeedback for neuromotor rehabilitation. J. Neuroeng. Rehabil. 3:11. doi: 10.1186/1743-0003-3-11
Hubbard, I. J., Parsons, M. W., Neilson, C., and Carey, L. M. (2009). Task-specific training: evidence for and translation to clinical practice. Occup. Ther. Int. 16, 190–203. doi: 10.1002/oti
Hylin, M. J., Kerr, A. L., and Holden, R. (2017). Understanding the mechanisms of recovery and/or compensation following injury. Neural Plast. 2017:7125057. doi: 10.1155/2017/7125057
Jang, S. H., Cho, S., Lee, J., and Park, J. (2003). Cortical reorganization induced by task-oriented training in chronic hemiplegic stroke patients. Mot. Syst. 14, 7–11. doi: 10.1097/01.wnr.0000051544.96524.f2
Jang, S. H., You, S. H., Hallett, M., Cho, Y. W., Park, C.-M., Cho, S.-H., et al. (2005). Cortical reorganization and associated functional motor recovery after virtual reality in patients with chronic stroke: an experimenter-blind preliminary study. Arch. Phys. Med. Rehabil. 86, 2218–2223. doi: 10.1016/j.apmr.2005.04.015
Janssen, H., Ada, L., Bernhardt, J., McElduff, P., Pollack, M., Nilsson, M., et al. (2014). An enriched environment increases activity in stroke patients undergoing rehabilitation in a mixed rehabilitation unit: a pilot non-randomized controlled trial. Disabil. Rehabil. 36, 255–262. doi: 10.3109/09638288.2013.788218
Jeannerod, M. (2001). Neural simulation of action: a unifying mechanism for motor cognition. Neuroimage 14, 103–109. doi: 10.1006/nimg.2001.0832
Johansen-Berg, H., Dawes, H., Guy, C., Smith, S. M., Wade, D. T., and Matthews, P. M. (2002). Correlation between motor improvements and altered fMRI activity after rehabilitative therapy. Brain 125, 2731–2742. doi: 10.1093/brain/awf282
Johansson, B. B., and Ohlsson, A. L. (1996). Environment, social interaction, and physical activity as determinants of functional outcome after cerebral infarction in the rat. Exp. Neurol. 139, 322–327. doi: 10.1006/exnr.1996.0106
Johnson, S. H. (2000). Imagining the impossible: intact motor representations in hemiplegics. Neuroreport 11, 729–732. doi: 10.1097/00001756-200003200-00015
Joo, H. W., Hyun, J. K., Kim, T. U., Chae, S. H., Lee, Y. I., and Lee, S. J. (2012). Influence of constraint-induced movement therapy upon evoked potentials in rats with cerebral infarction. Eur. J. Neurosci. 36, 3691–3697. doi: 10.1111/ejn.12014
Kalaria, R. (2002). Similarities between Alzheimer’s disease and vascular dementia. J. Neurol. Sci. 20, 29–34. doi: 10.1016/S0022-510X(02)00256-3
Kawagoe, R., Takikawa, Y., and Hikosaka, O. (1998). Expectation of reward modulates cognitive signals in the basal ganglia. Nat. Neurosci. 1, 411–416. doi: 10.1038/1625
Keetch, K. M., Lee, T. D., Schmidt, R. A., and Young, D. E. (2005). Especial skills: their emergence with massive amounts of practice. J. Exp. Psychol. Hum. Percept. Perform. 31, 970–978. doi: 10.1037/0096-1523.31.5.970
Kitazawa, S., Kimura, T., and Yin, P.-B. (1998). Cerebellar complex spikes encode both destinations and errors in arm movements. Nature 392, 494–497. doi: 10.1038/33141
Kleim, J. A., and Jones, T. A. (2008). Principles of experience-dependent neural plasticity: implications for rehabilitation after brain damage. J. Speech Lang. Hear. Res. 51:S225. doi: 10.1044/1092-4388(2008/018)
Knill, D. C., and Pouget, A. (2004). The Bayesian brain: the role of uncertainty in neural coding and computation. Trends Neurosci. 27, 712–719. doi: 10.1016/j.tins.2004.10.007
Kollen, B. J., Lennon, S., Lyons, B., Wheatley-Smith, L., Scheper, M., Buurke, J. H., et al. (2009). The effectiveness of the bobath concept in stroke rehabilitation what is the evidence? Stroke 40:e89-97. doi: 10.1161/STROKEAHA.108.533828
Körding, K. P., and Wolpert, D. M. (2006). Bayesian decision theory in sensorimotor control. Trends Cogn. Sci. 10, 319–326. doi: 10.1016/j.tics.2006.05.003
Kwakkel, G. (2009). Intensity of practice after stroke: more is better. Schweizer Arch. fur Neurol. und Psychiatr. 160, 295–298. doi: 10.1080/09638280500534861
Kwakkel, G., van Peppen, R., Wagenaar, R. C., Wood Dauphinee, S., Richards, C., Ashburn, A., et al. (2004). Effects of augmented exercise therapy time after stroke. Stroke 35, 2529–2539. doi: 10.1161/01.str.0000143153.76460.7d
Kwakkel, G., Veerbeek, J. M., van Wegen, E. E. H., and Wolf, S. L. (2015). Constraint-induced movement therapy after stroke. Lancet Neurol. 14, 224–234. doi: 10.1016/S1474-4422(14)70160-7
Làdavas, E. (2008). Multisensory-based approach to the recovery of unisensory deficit. Ann. N. Y. Acad. Sci. 1124, 98–110. doi: 10.1196/annals.1440.008
Lage, G. M., Ugrinowitsch, H., Apolinário-Souza, T., Vieira, M. M., Albuquerque, M. R., and Benda, R. N. (2015). Repetition and variation in motor practice: a review of neural correlates. Neurosci. Biobehav. Rev. 57, 132–141. doi: 10.1016/j.neubiorev.2015.08.012
Lang, C. E., MacDonald, J. R., and Gnip, C. (2007). Counting repetitions: an observational study of outpatient therapy for people with hemiparesis post-stroke. J. Neurol. Phys. Ther. 31, 3–10. doi: 10.1097/01.NPT.0000260568.31746.34
Langhorne, P., Bernhardt, J., and Kwakkel, G. (2011). Stroke rehabilitation. Lancet 377, 1693–1702. doi: 10.1016/S0140-6736(11)60325-5
Langhorne, P., Coupar, F., and Pollock, A. (2009). Motor recovery after stroke? a systematic review. Lancet Neurol. 8, 741–754. doi: 10.1016/S1474-4422(09)70150-4
Laver, K. E., Lange, B., George, S., Deutsch, J. E., Saposnik, G., and Crotty, M. (2017). Virtual reality for stroke rehabilitation. Cochrane Database Syst. Rev. 11:CD008349. doi: 10.1002/14651858.CD008349.pub4
Lee, T. D., and Genovese, E. D. (1988). Distribution of practice in motor skill acquisition: learning and performance effects reconsidered. Res. Q. Exerc. Sport 59, 277–287. doi: 10.1080/02701367.1988.10609373
Levin, M. F., Weiss, P. L., and Keshner, E. A. (2015). Emergence of virtual reality as a tool for upper limb rehabilitation: incorporation of motor control and motor learning principles. Phys. Ther. 95, 415–425. doi: 10.2522/ptj.20130579
Liepert, J., Bauder, H., Miltner, W. H. R., Taub, E., and Weiller, C. (2000). Treatment-induced cortical reorganization after stroke in humans. Stroke 31, 1210–1216. doi: 10.1161/01.STR.31.6.1210
Lilja, M., Bergh, A., Johansson, L., and Nygård, L. (2003). Attitudes towards rehabilitation needs and support from assistive technology and the social environment among elderly people with disability. Occup. Ther. Int. 10, 75–93. doi: 10.1002/oti.178
Lin, C. H., Knowlton, B. J., Chiang, M.-C., Iacoboni, M., Udompholkul, P., and Wu, A. D. (2011). Brain-behavior correlates of optimizing learning through interleaved practice. Neuroimage 56, 1758–1772. doi: 10.1016/j.neuroimage.2011.02.066
Livingston-Thomas, J., Nelson, P., Karthikeyan, S., Antonescu, S., Jeffers, M. S., Marzolini, S., et al. (2016). Exercise and environmental enrichment as enablers of task-specific neuroplasticity and stroke recovery. Neurotherapeutics 13, 395–402. doi: 10.1007/s13311-016-0423-9
Lohse, K. R., Lang, C. E., and Boyd, L. A. (2014). Is more better? Using metadata to explore dose-response relationships in stroke rehabilitation. Stroke 45, 2053–2058. doi: 10.1161/STROKEAHA.114.004695
Lucca, L. F. (2009). Virtual reality and motor rehabilitation of the upper limb after stroke: a generation of progress? J. Rehabil. Med. 41, 1003–1006. doi: 10.2340/16501977-0405
Luft, A. R., McCombe-Waller, S., Whitall, J., Forrester, L. W., Macko, R., Sorkin, J. D., et al. (2004). Repetitive bilateral arm training and motor. JAMA 292, 1853–1862.
Maier, M., Ballester Rubio, B., Duff, A., Duarte Oller, E., and Verschure, P. F. M. J. (2019). Effect of specific over nonspecific VR-based rehabilitation on poststroke motor recovery: a systematic meta-analysis. Neurorehabil. Neural Repair 33, 112–129. doi: 10.1177/1545968318820169
Martens, R., Burwitz, L., and Zuckerman, J. (1976). Modeling effects on motor performance. Res. Quarterly. Am. Alliance Heal. Phys. Educ. Recreat. 47, 277–291. doi: 10.1080/10671315.1976.10615372
Mattar, A. A. G., and Gribble, P. L. (2005). Motor learning by observing. Neuron 46, 153–160. doi: 10.1016/j.neuron.2005.02.009
Mazzoni, P., and Krakauer, J. W. (2006). An implicit plan overrides an explicit strategy during visuomotor adaptation. J. Neurosci. 26, 3642–3645. doi: 10.1523/JNEUROSCI.5317-05.2006
McCracken, H. D., and Stelmach, G. E. (1977). A test of the schema theory of discrete motor learning. J. Mot. Behav. 9, 193–201. doi: 10.1080/00222895.1977.10735109
McGann, M. (2010). perceptual modalities: modes of presentation or modes of interaction? J. Conscious. Stud. 17, 72–94.
Meredith, M. A., and Stein, B. E. (1986). Visual, auditory, and somatosensory convergence on cells in superior colliculus results in multisensory integration. J. Neurophysiol. 56, 640–662. doi: 10.1152/jn.1986.56.3.640
Middleton, E. L., and Schwartz, M. F. (2012). Errorless learning in cognitive rehabilitation: a critical review. Neuropsychol. Rehabil. 22, 138–168. doi: 10.1016/j.neuroimage.2013.08.045
Molier, B. I., Van Asseldonk, E. H. F., Hermens, H. J., and Jannink, M. J. A. (2010). Nature, timing, frequency and type of augmented feedback; Does it influence motor relearning of the hemiparetic arm after stroke? A systematic review. Disabil. Rehabil. 32, 1799–1809. doi: 10.3109/09638281003734359
Molinari, M., Leggio, M. G., De Martin, M., Cerasa, A., and Thaut, M. (2003). Neurobiology of rhythmic motor entrainment. Ann. N. Y. Acad. Sci. 999, 313–321. doi: 10.1196/annals.1284.042
Moore, E., Schaefer, R. S., Bastin, M. E., Roberts, N., and Overy, K. (2017). Diffusion tensor MRI tractography reveals increased fractional anisotropy (FA) in arcuate fasciculus following music-cued motor training. Brain Cogn. 116, 40–46. doi: 10.1016/j.bandc.2017.05.001
Mulder, T. (2007). Motor imagery and action observation: cognitive tools for rehabilitation. J. Neural Transm. 114, 1265–1278. doi: 10.1007/s00702-007-0763-z
Mulder, T., and Hochstenbach, J. (2001). Adaptability and flexibility of the human motor system: implications for neurological rehabilitation. Neural Plast. 8, 131–140. doi: 10.1155/NP.2001.131
Mulligan, M., Guess, D., Holvoet, J., and Brown, F. (1980). The individualized curriculum sequencing model (I): implications from research on massed, distributed, or spaced trial training. Res. Pract. Pers. Sev. Disabil. 5, 325–336. doi: 10.1177/154079698000500403
Naito, E., Kochiyama, T., Kitada, R., Nakamura, S., Matsumura, M., Yonekura, Y., et al. (2002). Internally simulated movement sensations during motor imagery activate cortical motor areas and the cerebellum. J. Neurosci. 22, 3683–3691. doi: 10.1523/jneurosci.22-09-03683.2002
Nathan, D. E., Prost, R. W., Guastello, S. J., Jeutter And, D. C., and Reynolds, N. C. (2012). Investigating the neural correlates of goal-oriented upper extremity movements. Neuro Rehabil. 31, 421–428. doi: 10.3233/NRE-2012-00812
Nezafat, R., Shadmehr, R., and Holcomb, H. H. (2001). Long-term adaptation to dynamics of reaching movements: a PET study. Exp. Brain Res. 140, 66–76. doi: 10.1007/s002210100787
Nielsen, J. B., Willerslev-Olsen, M., Christiansen, L., Lundbye-Jensen, J., and Lorentzen, J. (2015). Science-based neurorehabilitation: recommendations for neurorehabilitation from basic science. J. Mot. Behav. 47, 7–17. doi: 10.1080/00222895.2014.931273
Nombela, C., Hughes, L. E., Owen, A. M., and Grahn, J. A. (2013). Into the groove: can rhythm influence Parkinson’s disease? Neurosci. Biobehav. Rev. 37, 2564–2570. doi: 10.1016/j.neubiorev.2013.08.003
Nudo, R. J., Milliken, G. W., Jenkins, W. M., and Merzenich, M. M. (1996). Use-dependent primary motor alterations of movement representations cortex of adult squirrel monkeys. J. Neurosci. 16, 785–807. doi: 10.1523/jneurosci.16-02-00785.1996
Okamoto, T., Endo, S., Shirao, T., and Nagao, S. (2011). Role of cerebellar cortical protein synthesis in transfer of memory trace of cerebellum-dependent motor learning. J. Neurosci. 31, 8958–8966. doi: 10.1523/JNEUROSCI.1151-11.2011
Park, H., Kim, S., Winstein, C. J., Gordon, J., and Schweighofer, N. (2016). Short-duration and intensive training improves long-term reaching performance in individuals with Chronic stroke. Neurorehabil. Neural Repair 30, 551–561. doi: 10.1177/1545968315606990
Pascual-Leone, A., Grafman, J., and Hallett, M. (1994). Modulation of cortical motor output maps during development of implicit and explicit knowledge. Science 263, 1287–1289. doi: 10.1126/science.8122113
Pauwels, L., Chalavi, S., Gooijers, J., Maes, C., Albouy, G., Sunaert, S., et al. (2018). Challenge to promote change: the neural basis of the contextual interference effect in young and older adults. J. Neurosci. 38, 3333–3345. doi: 10.1523/JNEUROSCI.2640-17.2018
Pavlides, C., Miyashita, E., and Asanuma, H. (1993). Projection from the sensory to the motor cortex is important in learning motor skills in the monkey. J. Neurophysiol. 70, 733–741. doi: 10.1016/j.neuron.2011.07.029
Penhune, V. B., Zatorre, R. J., and Evans, A. C. (1998). Cerebellar contributions to motor timing: a PET study of auditory and visual rhythm reproduction. J. Cogn. Neurosci. 10, 752–765. doi: 10.1162/089892998563149
Plautz, E. J., Milliken, G. W., and Nudo, R. J. (2000). Effects of repetitive motor training on movement representations in adult squirrel monkeys: role of use versus learning. Neurobiol. Learn. Mem. 74, 27–55. doi: 10.1006/nlme.1999.3934
Praag, H., Van Kempermann, G., and Gage, F. H. (1999). Running increases cell profileration and neurogenesis in the adult mouse dentate gyrus. Nat. Neurosci. 2, 266–270. doi: 10.1038/6368
Proffitt, R., and Lange, B. (2015). Considerations in the efficacy and effectiveness of virtual reality interventions for stroke rehabilitation: moving the field forward. Phys. Ther. 95, 441–448. doi: 10.2522/ptj.20130571
Rajkowski, J., Majczynski, H., Clayton, E., and Aston-Jones, G. (2004). Activation of monkey Locus Coeruleus neurons varies with difficulty and performance in a target detection task. J. Neurophysiol. 92, 361–371. doi: 10.1152/jn.00673.2003
Ramachandran, V. S., and Rogers-Ramachandran, D. (1996). Synaesthesia in Phantom Limbs induced with mirrors. Proc. Biol. Sci. 263, 377–386. doi: 10.1098/rspb.1996.0058
Redcay, E., Dodell-Feder, D., Pearrow, M. J., Mavros, P. L., Kleiner, M., Gabrieli, J. D. E., et al. (2010). Live face-to-face interaction during fMRI; a new tool for social cognitive neuroscience. Neuroimage 50, 1639–1647. doi: 10.1038/jid.2014.371
Redgrave, P., and Gurney, K. (2006). The short-latency dopamine signal: a role in discovering novel action. Nat. Rev. Neurosci. 7, 967–975. doi: 10.1038/nrn2022
Reinkensmeyer, D. J., Burdet, E., Casadio, M., Krakauer, J. W., Kwakkel, G., Lang, C. E., et al. (2016). Computational neurorehabilitation: modeling plasticity and learning to predict recovery. J. Neuroeng. Rehabil. 13:42. doi: 10.1186/s12984-016-0148-3
Renton, T., Tibbles, A., and Topolovec-Vranic, J. (2017). Neurofeedback as a form of cognitive rehabilitation therapy following stroke: a systematic review. PLoS One 12:e0177290. doi: 10.1371/journal.pone.0177290
Ridderinkhof, K. R., van den Wildenberg, W. P. M., Segalowitz, S. J., and Carter, C. S. (2004). Neurocognitive mechanisms of cognitive control: the role of prefrontal cortex in action selection, response inhibition, performance monitoring, and reward-based learning. Brain Cogn. 56, 129–140. doi: 10.1016/j.bandc.2004.09.016
Rizzolatti, G., and Sinigaglia, C. (2010). The functional role of the parieto-frontal mirror circuit: interpretations and misinterpretations. Nat. Rev. Neurosci. 11, 264–274. doi: 10.1038/nrn2805
Ronsse, R., Puttemans, V., Coxon, J. P., Goble, D. J., Wagemans, J., Wenderoth, N., et al. (2011). Motor learning with augmented feedback: modality-dependent behavioral and neural consequences. Cereb. Cortex 21, 1283–1294. doi: 10.1093/cercor/bhq209
Rosenkranz, K., and Rothwell, J. C. (2006). Differences between the effects of three plasticity inducing protocols on the organization of the human motor cortex. Eur. J. Neurosci. 23, 822–829. doi: 10.1111/j.1460-9568.2006.04605.x
Ross, J. M., and Balasubramaniam, R. (2014). Physical and neural entrainment to rhythm: human sensorimotor coordination across tasks and effector systems. Front. Hum. Neurosci. 8:576. doi: 10.3389/fnhum.2014.00576
Rossignol, S., and Jones, G. M. (1976). Audio-spinal influence in man studied by the H-reflex and its possible role on rhythmic movements synchronized to sound. Electroencephalogr. Clin. Neurophysiol. 41, 83–92. doi: 10.1016/0013-4694(76)90217-0
Rossiter, H. E., Boudrias, M.-H., and Ward, N. S. (2014). Do movement-related beta oscillations change after stroke? J. Neurophysiol. 112, 2053–2058. doi: 10.1152/jn.00345.2014
Rubin, K. H., Bukowski, W., and Parker, J. G. (2006). “Peer interactions, relationships, and groups,” in Handbook of Child Psychology: Vol. 3. Social, Emotional, and Personality Development, eds W. Damon, M. Lerner, and N. Eisenberg (New York, NY: Wiley).
Salmoni, A. W., Schmidt, R. A., and Walter, C. B. (1984). Knowledge of results and motor learning: a review and critical reappraisal. Psychol. Bull. 95, 355–386. doi: 10.1037/0033-2909.95.3.355
Sánchez, A., Millán-Calenti, J. C., Lorenzo-López, L., and Maseda, A. (2013). Multisensory stimulation for people with Dementia. Am. J. Alzheimer’s Dis. 28, 7–14. doi: 10.1177/1533317512466693
Sato, A., and Yasuda, A. (2005). Illusion of sense of self-agency: discrepancy between the predicted and actual sensory consequences of actions modulates the sense of self-agency, but not the sense of self-ownership. Cognition 94, 241–255. doi: 10.1016/j.cognition.2004.04.003
Savion-Lemieux, T., and Penhune, V. B. (2005). The effects of practice and delay on motor skill learning and retention. Exp. Brain Res. 161, 423–431. doi: 10.1007/s00221-004-2085-9
Schaechter, J. D. (2004). Motor rehabilitation and brain plasticity after hemiparetic stroke. Prog. Neurobiol. 73, 61–72. doi: 10.1016/j.pneurobio.2004.04.001
Schaefer, R. S. (2014). Auditory rhythmic cueing in movement rehabilitation: findings and possible mechanisms. Philos. Trans. R. Soc. Lond. B. Biol. Sci. 369:20130402. doi: 10.1098/rstb.2013.0402
Scharf, M. T., Woo, N. H., Lattal, K. M., Young, J. Z., Nguyen, P. V., and Abel, T. (2002). Protein synthesis is required for the enhancement of long-term potentiation and long-term memory by spaced training. J. Neurophysiol. 87, 2770–2777. doi: 10.1177/0093854806288066
Schmidt, R. A. (1975). A schema theory of discrete motor skill learning. Psychol. Rev. 82, 225–260. doi: 10.1037/h0076770
Schmidt, R. A., and Lee, T. D. (2011). Motor Control and Learning: A Behavioral Emphasis, 5th Edn. Champaign, IL?: Human Kinetics.
Shadmehr, R., Smith, M. A., and Krakauer, J. W. (2010). Error correction, sensory prediction, and adaptation in motor control. Annu. Rev. Neurosci. 33, 89–108. doi: 10.1146/annurev-neuro-060909-153135
Shams, L., and Seitz, A. R. (2008). Benefits of multisensory learning. Trends Cogn. Sci. 12, 411–417. doi: 10.1016/j.tics.2008.07.006
Shea, C. H., and Kohl, R. M. (1991). Composition of practice: influence on the retention of motor skills. Res. Q. Exerc. Sport 62, 187–195. doi: 10.1080/02701367.1991.10608709
Shea, J. B., and Morgan, R. L. (1979). Contextual interference effects on the acquisition, retention, and transfer of a motor skill. J. Exp. Psychol. Hum. Learn. Mem. 5, 179–187.
Singer, B., Vallence, A.-M., Cleary, S., Cooper, I., and Loftus, A. (2013). The effect of EMG triggered electrical stimulation plus task practice on arm function in chronic stroke patients with moderate-severe arm deficits. Restor. Neurol. Neurosci. 31, 681–691. doi: 10.3233/RNN-130319
Sisti, H. M., Glass, A. L., and Shors, T. J. (2007). Neurogenesis and the spacing effect: learning over time enhances memory and the survival of new neurons. Learn. Mem. 14, 368–375. doi: 10.1101/lm.488707
Slater, M., Spanlang, B., Sanchez-Vives, M. V., and Blanke, O. (2010). First person experience of body transfer in virtual reality. PLoS One 5:e0010564. doi: 10.1371/journal.pone.0010564
Smania, N., Gandolfi, M., Paolucci, S., Iosa, M., Ianes, P., Recchia, S., et al. (2012). Reduced-intensity modified constraint-induced movement therapy versus conventional therapy for upper extremity rehabilitation after stroke: a multicenter trial. Neurorehabil. Neural Repair 26, 1035–1045. doi: 10.1177/1545968312446003
Smith, M. A., and Shadmehr, R. (2005). Intact ability to learn internal models of arm dynamics in Huntington’s disease but not cerebellar degeneration. J. Neurophysiol. 93, 2809–2821. doi: 10.1152/jn.00943.2004
Sober, S. J., and Sabes, P. N. (2003). Multisensory integration during motor planning. J. Neurosci. 23, 6982–6992. doi: 10.1523/jneurosci.23-18-06982.2003
Subramanian, S., Knaut, L. A., Beaudoin, C., McFadyen, B. J., Feldman, A. G., and Levin, M. F. (2007). Virtual reality environments for post-stroke arm rehabilitation. J. Neuroeng. Rehabil. 4, 1–5. doi: 10.1186/1743-0003-4-20
Subramanian, S. K., Massie, C. L., Malcolm, M. P., and Levin, M. F. (2010). Does provision of extrinsic feedback result in improved motor learning in the upper Limb Poststroke? a systematic review of the evidence. Neurorehabil. Neural Repair 24, 113–124. doi: 10.1177/1545968309349941
Sun, L., Yin, D., Zhu, Y., Fan, M., Zang, L., Wu, Y., et al. (2013). Cortical reorganization after motor imagery training in chronic stroke patients with severe motor impairment: a longitudinal fMRI study. Neuroradiology 55, 913–925. doi: 10.1007/s00234-013-1188-z
Tanaka, H., Sejnowski, T. J., and Krakauer, J. W. (2009). Adaptation to visuomotor rotation through interaction between posterior parietal and motor cortical areas. J. Neurophysiol. 102, 2921–2932. doi: 10.1152/jn.90834.2008
Taub, E. (1976). Movement in nonhuman primates deprived of somatsosensory feedback. Exerc. Sport Sci. Rev. 4, 335–374.
Taub, E., Crago, J. E., Burgio, L. D., Groomes, T. E., Cook, E. W., DeLuca, S. C., et al. (1994). An operant approach to rehabilitation medicine: overcoming learned nonuse by shaping. J. Exp. Anal. Behav. 61, 281–293. doi: 10.1901/jeab.1994.61-281
Taub, E., and Uswatte, G. (2003). Constraint-Induced Movement therapy: bridging from the primate laboratory to the stroke rehabilitation laboratory. J. Rehabil. Med. 35, 34–40. doi: 10.1080/16501960310010124
Taub, E., Uswatte, G., Mark, V. W., and Morris, D. M. M. (2006). The learned nonuse phenomenon: implications for rehabilitation. Eura. Medicophys. 42, 241–256.
Taub, E., Uswatte, G., and Pidikiti, R. (1999). Constraint-induced movement therapy: a new family of techniques with broad application to physical rehabilitation - a clinical review. J. Rehabil. Res. Dev. 36, 237–251.
Taylor, J. A., Krakauer, J. W., and Ivry, R. B. (2014). Explicit and implicit contributions to learning in a sensorimotor adaptation task. J. Neurosci. 34, 3023–3032. doi: 10.1523/JNEUROSCI.3619-13.2014
Thaut, M. H., and Abiru, M. (2010). rhythmic auditory stimulation in rehabilitation of movement disorders: a review of current research. Muisc Percept. 27, 263–270.
Thaut, M. H., McIntosh, G. C., Rice, R. R., Miller, R. A., Rathbun, J., and Brault, J. M. (1996). Rhythmic auditory stimulation in gait training for Parkinson’s disease patients. Mov. Disord. 11, 193–200. doi: 10.1002/mds.870110213
Thieme, H., Mehrholz, J., Pohl, M., Behrens, J., and Dohle, C. (2012). Mirror therapy for improving motor function after stroke (Review). Cochrane Database Syst. Rev. 14:CD008449. doi: 10.1002/14651858.CD008449
Thomas, L. H., French, B., Coupe, J., Mcmahon, N., Connell, L., Harrison, J., et al. (2017). Repetitive task training for improving functional ability after stroke. Stroke 48, 102–104. doi: 10.1161/STROKEAHA.117.016503
Tsakiris, M., Schütz-Bosbach, S., and Gallagher, S. (2007). On agency and body-ownership: phenomenological and neurocognitive reflections. Conscious. Cogn. 16, 645–660. doi: 10.1016/j.concog.2007.05.012
Tseng, Y., Diedrichsen, J., Krakauer, J. W., Shadmehr, R., and Bastian, A. J. (2007). Sensory prediction errors drive cerebellum-dependent adaptation of reaching. J. Neurophysiol. 98, 54–62. doi: 10.1152/jn.00266.2007
van Dijk, H., Jannink, M. J. A., and Hermens, H. J. (2005). Effect of augmented feedback on motor function of the affected upper extremity in rehabilitation patients: a systematic review of randomized controlled trials. J. Rehabil. Med. 37, 202–211. doi: 10.1080/16501970510030165
van Wegen, E., de Goede, C., Lim, I., Rietberg, M., Nieuwboer, A., Willems, A., et al. (2006). The effect of rhythmic somatosensory cueing on gait in patients with Parkinson’s disease. J. Neurol. Sci. 248, 210–214. doi: 10.1016/j.jns.2006.05.034
Vankov, A., Hervé-Minvielle, A., and Sara, S. J. (1995). Response to novelty and its rapid habituation in Locus Coeruleus neurons of the freely exploring rat. Eur. J. Neurosci. 7, 1180–1187. doi: 10.1111/j.1460-9568.1995.tb01108.x
Veerbeek, J. M., van Wegen, E., van Peppen, R., van der Wees, P. J., Hendriks, E., Rietberg, M., et al. (2014). What is the evidence for physical therapy poststroke? a systematic review and meta-analysis. PLoS One 9:e0087987. doi: 10.1371/journal.pone.0087987
Venna, V. R., Xu, Y., Doran, S. J., Patrizz, A., and McCullough, L. D. (2014). Social interaction plays a critical role in neurogenesis and recovery after stroke. Transl. Psychiatry 4:e351. doi: 10.1038/tp.2013.128
Verschure, P. F. M. J. (2011). “Neuroscience, virtual reality and neurorehabilitation: brain repair as a validation of brain theory,” Paper Presented at the Annual International Conference of the IEEE Engineering in Medicine and Biology Society (EMBC), Boston, 2254–2257. doi: 10.1109/IEMBS.2011.6090428
Wadden, K. P., Asis, K., De Mang, C. S., Neva, J. L., Peters, S., Lakhani, B., et al. (2017). Predicting motor sequence learning in individuals with chronic stroke. Neurorehabil. Neural Repair 3, 95–104. doi: 10.1177/1545968316662526
Wallace, M. T., and Stein, B. E. (1996). Sensory organization of the superior colliculus in cat and monkey. Prog. Brain Res. 112, 301–311. doi: 10.1016/s0079-6123(08)63337-3
Wexler, B. E., Fulbright, R. K., Lacadie, C. M., Skudlarski, P., Kelz, M. B., Constable, R. T., et al. (1997). An fMRI study of the human cortical motor system response to increasing functional demands. Magn. Reson. Imaging 15, 385–396. doi: 10.1016/S0730-725X(96)00232-9
Whitall, J., Waller, S. M., Silver, K. H. C., and Macko, R. F. (2000). Cueing improves motor function in chronic Hemiparetic stroke. Stroke 31, 2390–2395. doi: 10.1161/01.STR.31.10.2390
Wickens, C. D., Hutchins, S., Carolan, T., and Cumming, J. (2013). Effectiveness of part-task training and increasing-difficulty training strategies: a meta-analysis approach. Hum. Factors 55, 461–470. doi: 10.1177/0018720812451994
Wickens, J. R., Reynolds, J. N. J., and Hyland, B. I. (2003). Neural mechanisms of reward-related motor learning. Curr. Opin. Neurobiol. 13, 685–690. doi: 10.1016/j.conb.2003.10.013
Wilkins, K. B., Owen, M., Ingo, C., Carmona, C., Dewald, J. P. A., and Yao, J. (2017). Neural plasticity in moderate to severe chronic stroke following a device-assisted task-specific arm/hand intervention. Front. Neurol. 8:284. doi: 10.3389/fneur.2017.00284
Winstein, C. J. (1991). Knowledge of results and motor learning - Implications for physical therapy. Phys. Ther. 71, 140–149. doi: 10.1093/ptj/71.2.140
Winstein, C. J., Grafton, S. T., and Pohl, P. S. (1997). Motor task difficulty and brain activity: investigation of goal-directed reciprocal aiming using positron emission tomography. J. Neurophysiol. 77, 1581–1594. doi: 10.1152/jn.1997.77.3.1581
Winstein, C. J., Lewthwaite, R., Blanton, S. R., Wolf, L. B., and Wishart, L. (2014). Infusing motor learning research into neurorehabilitation practice: a historical perspective with case exemplar from the accelerated skill acquisition program. J. Neuologic Phys. Ther. 38, 190–200. doi: 10.1097/NPT.0000000000000046.Infusing
Winstein, C. J., Wolf, S. L., Dromerick, A. W., Lane, C. J., Nelsen, M. A., Lewthwaite, R., et al. (2016). Effect of a task-oriented rehabilitation program on upper extremity recovery following motor stroke the ICARE randomized clinical trial. JAMA 315, 571–581. doi: 10.1001/jama.2016.0276
Woldag, H., Stupka, K., and Hummelsheim, H. (2010). Repetitive training of complex hand and arm movements with shaping is beneficial for motor improvement in patients after stroke. J. Rehabil. Med. 42, 582–587. doi: 10.2340/16501977-0558
Wu, C. Y., Trombly, C. A., Lin, K. C., and Tickle-Degnen, L. (2000). A kinematic study of contextual effects on reaching performance in persons with and without stroke: influences of object availability. Arch. Phys. Med. Rehabil. 81, 95–101. doi: 10.1053/apmr.2000.0810095
Wulf, G., and Prinz, W. (2001). Directing attention to movement effects enhances learning: a review. Psychon. Bull. Rev. 8, 648–660. doi: 10.3758/BF03196201
Wulf, G., Chiviacowsky, S., and Lewthwaite, R. (2012). Altering mindset can enhance motor learning in older adults. Psychol. Aging 27, 14–21. doi: 10.1037/a0025718
Yamazaki, T., Nagao, S., Lennon, W., and Tanaka, S. (2015). Modeling memory consolidation during posttraining periods in cerebellovestibular learning. Proc. Natl. Acad. Sci. U.S.A. 112, 3541–3546. doi: 10.1073/pnas.1413798112
Yoo, G. E., and Kim, S. J. (2016). Rhythmic auditory cueing in motor rehabilitation for stroke patients: systematic review and meta-analysis. J. Music Ther. 53, 149–177. doi: 10.1093/jmt/thw003
Yue, Z., Zhang, X., and Wang, J. (2017). Hand rehabilitation robotics on poststroke motor recovery. Behav. Neurol. 2017, 1–20. doi: 10.1155/2017/3908135
Zhang, J., Yu, J., Bao, Y., Xie, Q., Xu, Y., Zhang, J., et al. (2017). Constraint-induced aphasia therapy in post- stroke aphasia rehabilitation?: a systematic review and meta-analysis of randomized controlled trials. PLoS One 12:e0183349. doi: 10.1371/journal.pone.0183349
Zhang, Y., Cai, J., Zhang, Y., Ren, T., Zhao, M., and Zhao, Q. (2016). Improvement in stroke-induced motor dysfunction by music-supported therapy: a systematic review and meta-analysis. Sci. Rep. 6:38521. doi: 10.1038/srep38521
Keywords: neurorehabilitation, motor learning, plasticity, stroke, principles
Citation: Maier M, Ballester BR and Verschure PFMJ (2019) Principles of Neurorehabilitation After Stroke Based on Motor Learning and Brain Plasticity Mechanisms. Front. Syst. Neurosci. 13:74. doi: 10.3389/fnsys.2019.00074
Received: 31 March 2019; Accepted: 19 November 2019;
Published: 17 December 2019.
Edited by:
Olivia Gosseries, University of Liège, BelgiumReviewed by:
Michelle Hook, Texas A&M University, United StatesStephan Patrick Swinnen, KU Leuven, Belgium
Copyright © 2019 Maier, Ballester and Verschure. This is an open-access article distributed under the terms of the Creative Commons Attribution License (CC BY). The use, distribution or reproduction in other forums is permitted, provided the original author(s) and the copyright owner(s) are credited and that the original publication in this journal is cited, in accordance with accepted academic practice. No use, distribution or reproduction is permitted which does not comply with these terms.
*Correspondence: Paul F. M. J. Verschure, pverschure@ibecbarcelona.eu