- 1Département des sciences animales, Université Laval, Québec, QC, Canada
- 2Institut sur la nutrition et les aliments fonctionnels, Université Laval, Québec, QC, Canada
- 3Centre de recherche en infectiologie porcine et avicole, Faculté de médecine vétérinaire, Université de Montréal, Saint-Hyacinthe, QC, Canada
- 4Département de biochimie, microbiologie et bio-Informatique, Université Laval, Québec, QC, Canada
- 5Olymel S.E.C./L.P., Boucherville, QC, Canada
Introduction: A thorough understanding of the microbial ecology within the swine value chain is essential to develop new strategies to optimize the microbiological quality of pork products. To our knowledge, no study to date has followed the microbiota through the value chain from live farm animals to the cuts of meat obtained for market. The objective of this study is to evaluate how the microbiota of pigs and their environment influence the microbial composition of samples collected throughout the value chain, including the meat plant and meat cuts.
Method and results: Results from 16S rDNA sequencing, short-chain fatty acid concentrations and metabolomic analysis of pig feces revealed that the microbiota from two farms with differing sanitary statuses were distinctive. The total aerobic mesophilic bacteria and Enterobacteriaceae counts from samples collected at the meat plant after the pre-operation cleaning and disinfection steps were at or around the detection limit and the pigs from the selected farms were the first to be slaughtered on each shipment days. The bacterial counts of individual samples collected at the meat plant did not vary significantly between the farms. Alpha diversity results indicate that as we move through the steps in the value chain, there is a clear reduction in the diversity of the microbiota. A beta diversity analysis revealed a more distinct microbiota at the farms compared to the meat plant which change and became more uniform as samples were taken towards the end of the value chain. The source tracker analysis showed that only 12.92% of the microbiota in shoulder samples originated from the farms and 81% of the bacteria detected on the dressed carcasses were of unknown origin.
Discussion: Overall, the results suggest that with the current level of microbial control at farms, it is possible to obtain pork products with similar microbiological quality from different farms. However, broader studies are required to determine the impact of the sanitary status of the herd on the final products.
1 Introduction
Pork makes up a major portion of global meat consumption and up until 2015, it was the world’s most consumed meat (OECD, 2021). Like all muscles from healthy animals, with the exception of lymph nodes, pork harbours a small number of microorganisms (Huffman, 2002). Meat and meat products are often identified as causing foodborne illnesses (Painter et al., 2013; Ramsay and Delisle, 2017). Meat is rich in nutrients and water, which supports microbial growth (Remenant et al., 2015; Zagorec and Champomier-Vergès, 2017). As such, contamination of swine carcasses and the resulting cuts of meat can lead to spoilage or pathogen growth throughout the shelf life of the product. Contamination of the meat can occur through microorganisms that are present in different parts of the animal (digestive tract, skin, respiratory tract, saliva, etc.; Zweifel and Stephan, 2014). Hence, pork quality relies on a combination of effective biosecurity measures, herd health management at the farm, hygienic slaughter and cutting practices and risk management measures throughout the value chain (HACCP plans and similar measures).
The sanitary status or health status of a farm is determined by a veterinarian through the monitoring of current and historical diseases as well as the current sanitation conditions of the farm. This status is thought to influence the final microbial quality of the product (Hurd et al., 2008; Vigors et al., 2020). Proper health management and other sanitary measures, including on-farm biosecurity, reduce the risk of infection from major swine pathogens such as pathogenic serovars of Mycoplasma hyopneumoniae (enzootic pneumonia) and Actinobacillus pleuropneumoniae (pleuropneumonia; Stärk et al., 2008). Other pathogens, including Clostridium perfringens, Salmonella enterica and Yersinia enterocolitica have been linked with carcass contamination (Fosse et al., 2008). While modern pig farms generally maintain excellent sanitary status, there is a certain level of variability between farms (Cameron, 2000). Improving the sanitary conditions at the farm can also improve animal health and behaviour (Meer et al., 2017). To properly evaluate the impact of the sanitary status of farms on the microbiota throughout the different steps of the value chain, precise information about the microbial populations in swine, from farm to the packaging of meat, must be obtained. To date, the only information available is limited to studies where samples were randomly or sporadically taken at specific steps of the value chain.
Several 16S rRNA amplicon sequencing studies have focused on key areas of the swine value chain. The microbial ecosystem found in the air of the housing building (Nehme et al., 2008; Kumari et al., 2016; Kraemer et al., 2019; Yan et al., 2021), the swine gut (Kim et al., 2015; Kim and Isaacson, 2015; Zhao et al., 2015; Holman et al., 2017; Crespo-Piazuelo et al., 2018; Quan et al., 2018; Xiao et al., 2018; Adhikari et al., 2019; Mark Welch et al., 2019; Vigors et al., 2020), the respiratory tract, mouth and saliva (Wang et al., 2018; Huang et al., 2019; Mou et al., 2019; Murase et al., 2019), the meat plant environment (Bridier et al., 2019; Zwirzitz et al., 2020) and the carcass (Mann et al., 2016; Zwirzitz et al., 2019; Zwirzitz et al., 2020) have been examined. Swine gut microbiota is by far the most studied of these environments, enough so that the concept of a “core” microbiota (bacteria present in over 90% of samples) was put forward by Holman et al. (2017). These authors identified the genera Clostridium, Blautia, Lactobacillus, Prevotella, Ruminococcus, Roseburia, RC9 “gut group” and Subdoligranulum as the main members of that “core”.
To our knowledge, no other study covers all of these environments and follows the same live animals from farm to meat cuts. Two commercial swine farms with different sanitary statuses were selected, we characterized the microbiota of the animals and their environments and observed how the microbiota changed at the carcass dressing (slaughter, evisceration) and meat cut preparation steps. This descriptive study presents detailed knowledge of the farm and animal microbiota, and the efficacy with which meat processing plants mitigate the impact of these microbes on the resulting carcasses and meat cuts.
2 Material and methods
2.1 Selected farms
Two commercial farms out of 126 were selected by experienced veterinarians and were located in the province of Quebec. The farms were selected based on their sanitary status, the gastrointestinal health of the animals and the medical history associated with the farms; one farm with a lower sanitary status (farm-L) and one with a higher status (farm-H). Both were finishing farms of similar housing size, with 1,200 and 1,600 animals, respectively. The animals (female (Yorkshire X Landrace) X Duroc male) were fed the same commercial diet and followed the same 4-phase finishing nutrition program. There was one exception at farm-L, where the diet changed from pellets to mash feed 22 days before the first sampling, due to an episode of salmonellosis (see discussion for clinical details). Animals from both farms were sent to the same federally-inspected meat plant when they reached a weight of 115 ± 7 kg. They came from the same production lot where three out of five shipments were followed. Samples were collected at each farm 3 days before the swine were transported to the slaughterhouse; farm-L animals were sent from July to August and farm-H from October to November. Animals were subject to a mandatory 18-h feed withdrawal before slaughter as required by the market agreement on animal welfare issues. All animal care and handling procedures were approved by Université Laval’s Animal Use and Care Committee (2019-329), which strictly adheres to the Guidelines of the Canadian Council on Animal Care (CCAC, 2009).
2.2 Slaughter and carcass breaking
Pigs were separated into groups of five and stunned using CO2. After dressing, the carcasses were blast-chilled for 90 min and then cooled overnight (24 h, 2°C) before being split into retail cuts (Supplementary Figure S1). Inspection data were collected, including frequency of demerits (abscess, lymphadenitis, bruises, etc.) and number of carasses that were condemned or retained for further examination (dead animal at reception, large and widespread abscesses, peritonitis, icterus, etc.), stomach size (in terms of feed withdrawal efficacy) and presence of bloated viscera.
2.3 Sampling and sample processing
Multiple types of samples were collected along the value chain (Supplementary Figure S1). All samples were stored on ice until they were processed. At the farms, sample types included air (Ar), feces (Fc), saliva (Sa), and feed (Fe). Fc and Sa samples were collected from 16 pens for all three shipments. Air was sampled using a SASS 3100 dry air sampler (Research International, Monroe, WA, United States) with a welded Standard electret filter cartridge. A volume of 10 m3 was collected at 300 mL/min for 33 min, three times a day (8:00, 11:00, and 14:00). The apparatus was placed on a table (1-m high) in the middle of the alley in the room where the pigs were housed. The filters were then preserved at −20°C until particle extractions were performed using the SASS 3010 particle extractor (Research International) with the recovery buffer (138 mM NaCl, 2.7 mM KCl, 0.05% Triton X-100, <0.1% NaN3 10 mM Na3PO4, pH 7.4). Extractions were performed according to manufacturer instructions. In order to ensure sufficient amounts of material for subsequent DNA purification, particle solutions were pooled in equal volumes by sampling date, and then centrifuged at 4°C, 14 000 × g for 20 min (Sorvall legend XTR centrifuge, Thermo Fisher Scientific, Waltham, MA, United States). Excess supernatant was removed, and cells were stored at −80°C until DNA extraction.
Fc samples were collected using a PERFORMAbiome•GUT | PB-200 sampling kit following manufacturer instructions (DNAgenotek, Ottawa, Ontario, Canada). Freshly defecated feces from 16 random animals, one per pen, were collected every sampling day. Additional Fc samples were collected for metabolomic and short chain/volatile fatty acid analyses (see sections below). A 500-µL aliquot from each PB-200 tube was aseptically pooled according to sampling date. Individual and pooled samples were then stored at −80°C until DNA extraction. Saliva was collected using a P-151 kit (DNAgenotek) as suggested by the manufacturer. An aliquot of 250 µL from each P-151 tube was pooled by sampling date and then stored at −80°C until DNA extraction. Feed was aseptically sampled directly from eight feeders and stored in sterile 4-oz Whirlpack bags (Nasco, Madison, WI, United States). The samples were pooled to form a composite by mixing 2.5 g of feed from each feeder and then stored at −20°C until DNA extraction.
At the meat plant, pre-operational procedures were performed to ensure a clean processing line. On each processing day, the animals under study were the first to be slaughtered and their carcasses were the first to be split into retail cuts the next day. This allowed us to properly assess what contamination originated from the animals from each farm. Environmental samples were aseptically collected for each shipment before and after the animals under study were processed on the dressing line. Surface samples were obtained using a sterile sponge (Whirl-Pak® Speci-Sponge® Environmental Surface Sampling Bags, Nasco) that was humidified with 10 mL of sterile 2% buffered peptone water (Peptone Water, phosphate-buffered, Milipore Sigma, Oakville, Canada) and a 10 × 10 cm sterile template (3 M cattle template, USDA100, 3 M Canada, London, Malaysia). In collaboration with the quality control team, we selected sampling sites according to their HACCP plan. At evisceration, water samples were collected from a central drain under the viscera conveyer (Dev; 150 mL). As well, surface samples from a gutter post-evisceration (Gev; 300 cm2) and from a conveyor before the first carcass shower wash (Cev; 300 cm2) were obtained. Twenty-five blast chilled carcasses were sampled using surface swabs (Dc). Swabs were collected from a 100-cm2 surface from the hind leg near the anus, the belly and the jowl (300 cm2 in total), according to the Guidelines for Escherichia coli Testing for Process Control Verification in Cattle and Swine Slaughter Establishments (FSIS-GD-1996-0001, 2005, FDA). A second group of 25 carcasses, were sampled the following day after overnight refrigeration at 2°C (Cc). In the area where carcasses were split, water samples were collected from a central drain (Dcu; 150 mL) and surface samples at the end of the conveyor (Ccu; 300 cm2). During this part of the process, 25 shoulders (S) were randomly selected for sampling (Supplementary Figure S1) and a total of 450 cm2 was swabbed from the surface of each shoulder and from the inside section where the shoulder bone was removed.
At the laboratory, 10 mL of 2% buffered peptone water was added to each of Whirl-Pak bags containing the sponge. The content of the bag was then homogenized using a Stomacher 400C (Seward Laboratory Systems Inc., London, United Kingdom) for 2 min, at 230 rpm. An aliquot of 2 mL from each set of carcasses and shoulder samples was pooled and thoroughly mixed for microbial enumeration. Pooling was deemed necessary for some samples in order to obtain enough DNA for downstream applications. Samples were stored at −80°C until DNA extraction.
2.4 Short-chain fatty acid analysis of feces
Short-chain fatty acid (SCFA; acetic, propionic, butyric, isobutyric, valeric and isovaleric acids) concentrations were measured in the 16 individual Fc samples from each sampling day (96 total samples). Fc samples were stored at −80°C until analysis. Fecal suspensions were then prepared from thawed Fc samples. Samples were divided into 500 mg aliquots of feces that were dissolved in 10 times the volume of water and homogenized for 2 min with a Bead Ruptor 12 (Omni international, Kennesaw, GA, United States). The suspensions were then centrifuged at 4°C, 5,500 × g for 30 min and SCFAs were extracted from the supernatant by liquid-liquid extraction and analyzed by gas chromatography coupled to a flame ionization detector (GC-FID Shimadzu, Kyoto, Japan), as described in Roussel et al. (2022).
2.5 Metabolomic analysis of feces
Untargeted metabolomics was also performed on the individual Fc samples using a liquid chromatography coupled to a mass spectrometer (LC-MS). Feces were thawed and divided into 900 mg aliquots. Samples were then lyophilized with a Lyovapor L-300 (BÜCHI Labortechnik AG, Flawil, Suisse) for 72 h. A volume of 12.5 µL of 50% MeOH in water per mg of dry matter was added. Samples were then mixed with a bead beater (Bead ruptor 12) for 2 min and then agitated with a multi-tube vortex (Vx-2500, VWR international, Wayne, NJ, USA) for 5 min. Next, samples were sonicated in an ultrasonic bath at 25°C for 30 min, agitated with a multi-tube vortex for 5 min and centrifuged at 4°C, 2 795 × g for 30 min. A volume of 250 µL of supernatant was collected and mixed with 250 µL of 50% MeOH in water containing 10 internal standards (2 ppm trihydroxybenzoic acid-d2, 2 ppm caffeine-methyl-d3, 10 ppm succinic acid-d6, 0.2 ppm N-dodecylphosphocholine-d38, 10 ppm trans-cinnamic acid-d5, 2 ppm L-tryptophane-d5, 2 ppm glycocholic acid-d4, 10 ppm L-leucine-d7, 2 ppm 4-hydroxybenzoic acid-d4 and 2 ppm methyl 4-hydroxybenzoate-d4; CDN isotope). Finally, samples were filtered with spin filters (InnoSep Spin, NY, 0.2 µm, Canadian Life Science, Peterborough, Canada) prior to LC-MS analysis.
LC-MS analyses were performed on a system consisting of a Vanquish ultra-high-performance liquid chromatography (UHPLC) and a Fusion Tribrid mass spectrometer (Thermo Fisher Scientific, Waltham, MA, United States). Mobile phases were water (A) and acetonitrile (B), each with 0.1% formic acid. The following elution gradient was used: 0 min 2% of B, 0.5 min 2% of B, 9 min 45% of B, 9.5 min 80% of B, 15.5 min 80% of B, 16 min 2% of B and 22 min 2% of B, at a flow rate of 0.3 mL/min. A volume of 5 µL was injected on an Acquity UPLC HSS T3 Column (100 Å, 1.8 µm, 2.1 mm × 100 mm; Waters, Milford, MA, United States) and kept at 30°C. Samples were maintained at 4°C in the auto-sampler. A Quality Control (QC) pooled sample consisting of an equal volume of each sample was analyzed every 10 samples.
An electronebulizer heated to 350°C was used as an ionization source with a capillary voltage of 3.5 kV in positive mode and 2.5 kV in negative mode. Mass spectroscopy (MS) acquisitions were performed on an orbitrap at a resolution of 120 000 in profile mode using Easy-IC for mass correction. All other parameters were set at their default values. MS2 spectra for the QC pooled sample were acquired using the AcquireX method. Data were analyzed with the Compound Discovered 3.2 and MetFrag Web 2.1 software packages (Ruttkies et al., 2016).
2.6 Microbial analysis
For enumeration on agar plates, tenfold serial dilutions were carried out using sterile 0.1% peptone water (BD Biosciences, Franklin Lakes, NJ, United States). Total aerobic mesophilic (TAM) counts (Health Canada, 2020) were performed on Plate Count Agar (BD Biosciences; 35°C for 48 h). Enterobacteriaceae (EB) counts (Health Canada, 1997) were performed on Violet Red Bile Glucose Agar (BD Biosciences; 35°C for 24 h). Measurements were performed in duplicate. For environmental samples (drains, conveyor and gutter), contamination originating from the animals was measured by subtracting the value obtained after the pre-operation procedures from the value obtained right after the passage of animals under study. The number of animals sent to the meat plant varied over the six shipments, therefore basic statistical weighting was used to adjust the results to a lot size of 300 animals (Kalton and Flores-Cervantes, 2003). All bacterial counts were transformed to a Log10 value of colony forming units per 300 cm2 or 300 mL prior to statistical analysis (Gill, 2000). When no colonies were observed, the level of detection was used in statistical analyses.
2.7 DNA extraction and 16S rDNA sequencing
Frozen samples were thawed at 4°C. Liquid samples were centrifuged (Sorvall legend) at 4°C, 24 000 × g for 10 min for the 50 mL samples, and 14 000 × g for 20 min for the 10 mL samples. The supernatant was removed, and the cell pellets were used for DNA extraction. Fe samples were hydrated in 2% buffered peptone water in a 9:1 ratio of water to feed for 30 min at 4°C in filtered stomacher bags. The hydrated pellets were homogenized using a Stomacher 400C for 2 min at 230 rpm. A total volume of 2.5 mL was recovered and centrifuged at 14 000 × g for 10 min at room temperature. The pelleted cells were used for DNA extraction.
DNA extraction kits were chosen based on their extraction efficiency with the various samples collected. A DNeasy PowerSoil Kit (QIAGEN, Toronto, Canada) was used for air, feed, conveyor, gutter, and carcass samples. A QIAamp Fast DNA Stool Mini Kit (QIAGEN) was used for the stabilized feces, the DNeasy PowerWater Kit (QIAGEN) for the liquid collected from the drains and a QIAamp BiOstic Bacteremia DNA Kit (QIAGEN) was used for S samples. A MasterPure Complete DNA and RNA Purification Kit (Lucigen, Teddington, United Kingdom) was used for Sa samples. All kits were used following the manufacturer protocols. Extracted DNA samples were quantified using a NanoDrop One spectrophotometer (Thermo Fisher Scientific, Ottawa, ON, Canada).
2.8 Amplification of the 16S DNA
During the preliminary set up of the experiment, metagenomic shotgun sequencing was performed to analyze the microbial communities. Unfortunately, pig DNA was in such abundance (>95%) in the samples collected that the analysis was losing depth. Therefore, amplification and sequencing of the 16S gene was deemed more appropriate. Library preparation and sequencing were performed at the Plateforme d’analyse génomique (Institut de Biologie Intégrative et des Systèmes, Université Laval, Quebec City, Canada). Amplification of the 16S V3-V4 region was performed as described in Klindworth et al. (2012) in a long oligo PCR approach. The PCR reactions were purified using an Axygen PCR cleanup kit (Axygen, New York, NY, USA). The quality of the purified PCR product was confirmed with a DNA7500 BioAnalyzer chip (Agilent, Santa Clara, CA, United States) and quantified using a NanoDrop One spectrophotometer. Barcoded amplicons were pooled in equimolar concentrations and sequenced on an Illumina MiSeq (paired-end 300 bp with two index reads). Samples collected after pre-operational cleaning and disinfection procedures with insufficient read quantity were discarded.
2.9 Sequence processing
The first step in read processing was to inspect the quality plots generated by FastQC (version 0.11.5; Andrews, 2010). Amplicon sequence variants (ASVs) were generated using the DADA2 workflow package (version 1.22.0; R version 4.1.1; Callahan et al., 2016). During filtration, the first 17 nucleotides of the forward reads and the first 21 of the reverse reads were trimmed to remove primers. Sequences containing ambiguous nucleotides (N) were discarded. According to the findings of Prodan et al. (2020), the expected error filter was not used to avoid bias towards bacteria with an error-prone genome. Dereplication, sample inference, chimera identification and merging of the paired-end reads were performed using the default parameters, with the exception that samples were pooled during inference step. Taxonomic assignment was done using the SILVA rRNA database (release 138.1; Pruesse et al., 2007) with the naive Bayesian classifier method (the assignTaxonomy command of the DADA2 package). Species were then added with the add species function. A phylogenetic tree was built based on a multiple alignment (DECIPHER R package version 2.22.0; Wright, 2016). Then a neighbor-joining tree was built and used as a basis for the GTR + G + I maximum likelihood tree (phangorn, R package version 2.8.1; Schliep, 2010). Counts, taxa, study metadata and phylogenectic trees were then combined into a phyloseq object (version 1.38.0; McMurdie and Holmes, 2013). Contaminants were removed using blank samples and the prevalence method (threshold of 0.5; decontam, R package version 1.14.0; Davis et al., 2018). Data were filtered by removing non-bacterial ASVs (Kingdom Eukaryota and Archaea, Order Chloroplast and Family Mitochondria), then ASVs without phylum identification followed by any low prevalence phylum (less than five ASVs per phylum), and finally low prevalence ASVs (present in less than 5% of samples). Filtered ASVs were searched against the NCBI nr/nt 16S curated database (Bioproject 33175 or 33317; excluding archea; downloaded 14-01-2022) in GenBank using BLASTN (version 2.12.0; Altschul et al., 1990). When the query ASV had more than 97% identity with the sequences in the GenBank database, the same genus level identification as in the SILVA database and a clearly defined species assignment (no ambiguous same percentage identity yet different species identification; those were left as NA) was manually reassigned to that ASV. The same procedure was followed for genus reassignment with a 90% identity threshold and family consensus. The remaining taxa were grouped in the “remaining taxa” row. The ASV counts were normalized into relative abundance for heatmap visualisation (ampvis2, R package version 2.7.13; Andersen et al., 2018). A heatmap graph was produced using a subset of bacterial families known to impact meat (Campylobacteraceae, Carnobacteriaceae, Enterobacteriaceae, Lactobacillaceae and Staphylococcaceae; Baer et al., 2013; Saucier, 2016; Møretrø and Langsrud, 2017). Sequences can be found in DDBJ/ENA/GenBank under the BioProject PRJNA923296.
2.10 Statistical analysis
A Shapiro-Wilk test was used to confirm normality and a Student T test to compare each sample type between farms and for both TAM and Enterobacteriaceae counts. T tests were also used for samples collected before and immediately after the animals under study were processed as well as carcass inspection data collected for each shipment and SCFAs in feces. These tests were also performed without separating them by farm in order to determine whether the animal’s presence had an effect on the contamination level. A non-parametric analysis was also performed on the basis that when a specific microbial concentration threshold is used to determine the end of shelf life, cell counts closer to that threshold limit represent a greater microbial food safety risk. For that, a Wilcoxon test was performed to compare the two farms instead of a Student T test because the distributions were not normal when all sample type were pooled together.
Metabolomics data integrity was first validated by measuring the %CV on different internal standards detected in positive (caffeine-d3, dodecylphosphochloline-d38, tryptophan-d5 and leucine-d7) and negative (succinic acid-d6, trans-cinnamic acid-d5 and tryptophan-d5) ionization. The %CV was below 20% for all these compounds. Then, untargeted metabolomic data quality was assessed using a principal component analysis (PCA) and showed a strong clustering of QC-pool injections. One sample was considered an outlier and was removed. Metabolic features were filtered based on the following strict criteria: present in all the QC pool injections (3183 and 542 features left in positive and negative mode), and %CV < 20% in its own group (farm-L or farm-H, 251 and 71 features left in each ionization mode). Features without a MS2 spectra were also removed from the analysis (240 and 64 features left). A differential analysis (Log2 fold change >1 and a p-value <0.05) was performed on the remaining features. Selected metabolites were then putatively identified using the Compound Discoverer FiSH score as the scoring metric and then confirmed using MetFrag Web.
The 16S rDNA sequences were categorized based on farm, sample type (Ar, Fc, Sa, Fe, Dev, Cev, Gev, Dc, Cc, Dcu, Ccu, and S), sampling location (farm, evisceration, cut-out) and shipment week (weeks 1, 2, and 3). Differential abundance analyses were performed also for a subset of genera and families important for meat safety (Campylobacteraceae, Carnobacteriaceae, Enterobacteriaceae, Lactobacillaceae, and Staphylococcaceae), between farms, using the Analysis of Compositions of Microbiomes with Bias Correction (ANCOMBC) methodology. This method was performed on the raw read counts of each family and their genus (ANCOMBC R package version 1.4.0; Lin and Peddada, 2020). Several parameters were used to control the false discovery rate (FDR) and increase the robusnes of the analyses. These included: a zero cut of 1 (no genera excluded), 1,000 iterations, a conservative variance estimate, FDR adjustment of p values, structural zeros and negative lower-bound zeros.
Alpha diversity (within-sample) was calculated on non-normalized data (phyloseq version 1.30.0; McMurdie and Holmes, 2013). The species richness was evaluated with an Observed and Chao1 index and evenness was evaluated with the Shannon and Simpson index. Theses indices were selected to evaluate large population changes as they give both a number for the total ASV (Observed and Chao1) and abundance distribution amongst these ASV (Shannon and Simpson). A Student T test was used to compare each sample type between farms. A FDR correction was used to control false positives. To evaluate differences between sample type diversity, a Tukey HSD test was performed between all the samples (agricolae, R package version 1.3–5; Mendiburu and Yaseen, 2021).
Beta diversity (between samples) was calculated for the normalized ASV counts using unweighted and weighted UniFrac distances (Lozupone and Knight, 2005) and Bray-Curtis dissimilarities (Phyloseq R package version 1.38.0; McMurdie and Holmes, 2013). Data were normalized by performing a Hellinger transformation (decostand function of the vegan R package version 2.5–7; Legendre and Gallagher, 2001). Principal coordinate analysis (PCoA) was used to visualize the distances between samples (Ampvis2, R package version 2.7.13; Andersen et al., 2018). Permutational analysis of multivariate dispersions (PERMDISP) was used to test the homogeneity of dispersion for each metadata category (betadisper function of the vegan R package). Since heterogeneity of dispersion was confirmed, analysis of similarities (ANOSIM; function of the vegan R package) was performed.
The linear discriminant analysis effect size (LEfSe) method was performed on non-normalized data (raw ASV counts) using the microbiome analyst platform (Dhariwal et al., 2017). Genera with higher relative abundances in the different sampling sites of the value chain were identified by LEfSe. The size effect of each of these genera was calculated using linear discriminant analysis (LDA; Segata et al., 2011). An LDA score (Log10) of 1.0 was used as the cut-off for identifying biomarkers. LEfSe was also used to compare the Fc samples between farms in order to identify bacteria that could be linked to short-chain fatty acid production. Here, a LDA of 2 was used as the cut-off value.
Microbial source tracking was achieved for Dc, Cc and S samples using the SourceTracker software package (version 1.0.1) with the default parameters (Knights et al., 2011). A rarefaction value of 1,000 reads and an alpha 1 and 2 of 0.001 were used. Farm (Ar, Fc, Sa, Fe) and environment (Dev, Cev, Gev, Dcu, Ccu) samples were considered source samples and those from meat (Dc, Cc, S) were considered to be sink samples.
3 Results
3.1 Characterization of the sampled farms
Farm microbiota were characterized by analyzing Ar, Fc, Sa, and Fe samples using 16S rRNA gene amplicons. At the genera level, samples from Ar were similar to Fc, while Sa samples were similar to Fe (Figure 1). When comparing the two farms for each sample type, the Fe microbiota, and to a lesser extent Sa, exhibited noticeable differences. Interestingly, at the phyla level, Proteobacteria represented 90.7% of the reads in Fe from farm-L and 57.6% from farm-H. Proteobacteria were two times more abundant in Sa samples from farm-H compared to farm-L (Supplementary Figure S2).
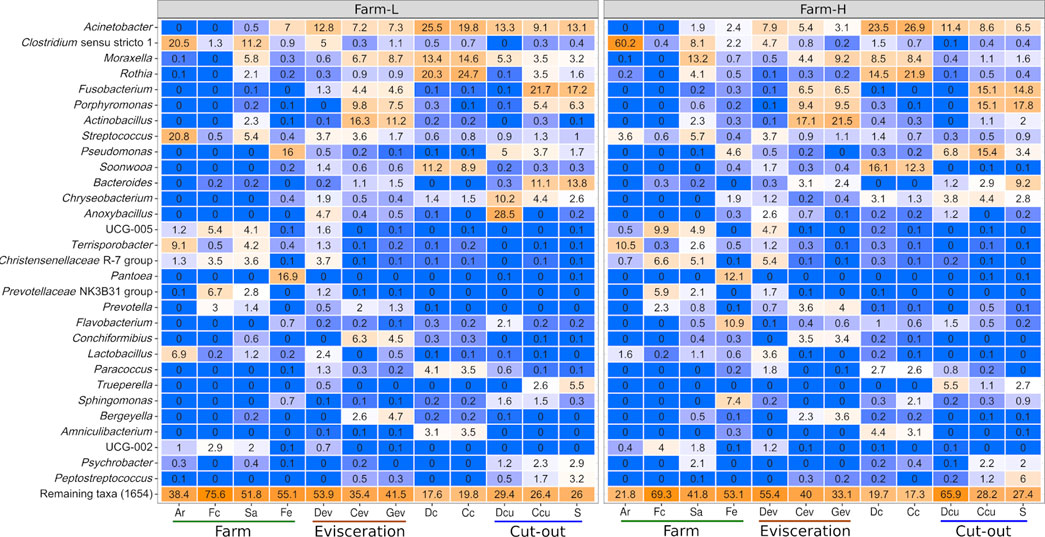
FIGURE 1. Top 30 genera based on relative abundance (%) identified in each of the sample types for both farms. Genera were identified using the SILVA database. Colour gradients range from blue = 0% to orange = 100%. Samples were collected from air (Ar), feces (Fc), saliva (Sa), feed (Fe), drain at evisceration (Dev), conveyor at evisceration (Cev), blood collection gutter (Gev), dressed carcasses (Dc), cold carcasses (Cc), drain at cut-out (Dcu), conveyor at cut-out (Ccu) and shoulder (S). Farm-L with a lower and farm-H with a higher sanitary status, respectively.
Short-chain fatty acid concentrations in the feces of animals from both farms were measured and compared to assess microbial activity (Table 1). Concentration of total SCFAs was significantly higher in samples from farm-L than farm-H (p = 0.02) as well as for each individual SCFAs (all p < 0.01). Furthermore, the results from the LEfSe analysis indicate a higher abundance (p < 0.05, LDA score cutoff of 2; data not shown) of specific genera known to produce SCFAs in farm-L Selenomonas (Wang et al., 2012), Anaerovibrio (Holman et al., 2021), Roseburia (Puertollano et al., 2014; Wang et al., 2019; Markowiak-Kopeć and Śliżewska, 2020), Akkermansia (Li et al., 2021) and Clostridium (Puertollano et al., 2014; Wang et al., 2019; Markowiak-Kopeć and Śliżewska, 2020).
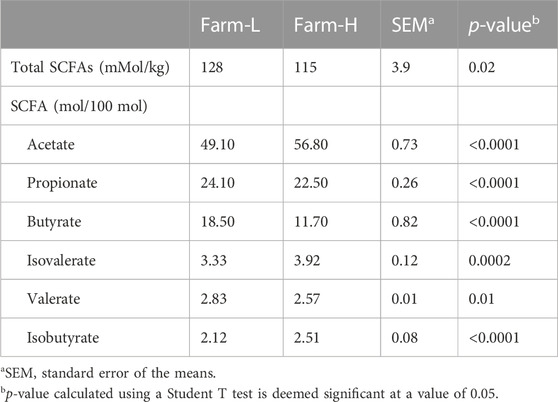
TABLE 1. Average short-chain fatty acid concentrations (SCFAs) in the feces of swine from two farms with different sanitary statuses.
Metabolomic analysis was performed on feces to identify potential metabolic markers related to the farm of origin and their different sanitary statuses. There was a clear separation of data from the two farms on the first principal component of the PCA score plot for both positive (27.1%) and negative ionization (28.5%; Supplementary Figure S3). This indicates that the farm environment and characteristics had an important influence on the metabolite composition of feces. Discriminant analysis through Volcano plots provide another clear indication of the different metabolites characterizing each farm. Under positive ionization, 85 ions were significantly more abundant in the feces collected from either farm (Figure 2A). Under negative ionization, 16 ions were significantly more abundant (Figure 2B). Putative identification based on molecular mass and fragmentation spectra was obtained for 21 metabolites in positive ionization and six metabolites in negative ionization (Supplementary Table S1). Since the farms fed animals different diet form (pellet vs. mash) prior to slaughter and only two farms were sampled, we could not confidently ascribe specific biomarkers to the health status of the animals. Nonetheless, they represent potential candidates for further studies. Overall, our results indicate that the microbiota of animals were different between the two farms.
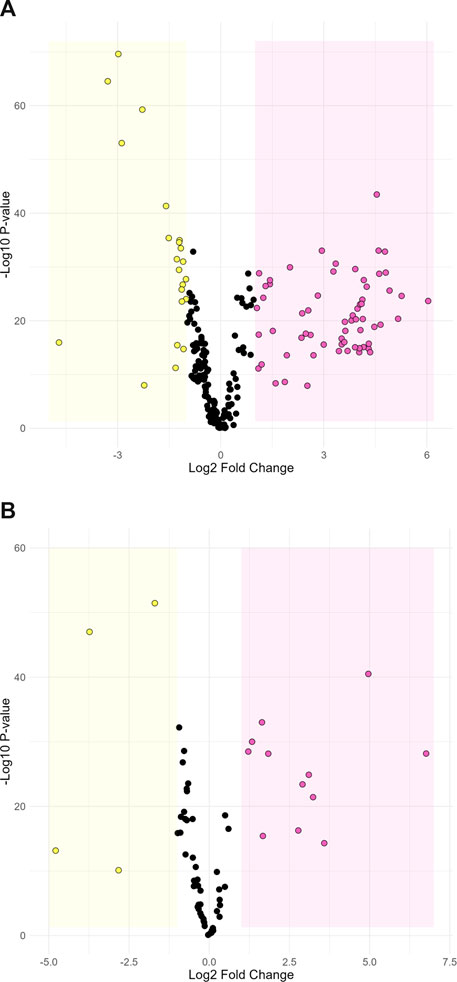
FIGURE 2. Volcano plot comparing metabolite abundance between farm-L and farm-H under positive (A) and negative (B) ionization. Dots in the yellow section are ions that are significantly more abundant in farm-H samples and dots in the pink section are significantly more abundant in farm-L samples. The highlighted sections represent a Log2-fold change greater than 1 and a p-value less than 0.05.
3.2 Characterization of the meat plant prior to operations
At the beginning of each slaughter and carcass breaking day, before the animals enter the processing line, most of the samples from the meat plant environment (Cev, Gev, Dcu, Ccu) had microbial counts (TAM and EB) around or below the detection level (<2.48 Log10 CFU/300 mL or cm2). This was true for all sampling weeks for both farms. Furthermore, there was no significant difference (t-test and Wilcoxon; p > 0.05) in the initial contamination of the meat plant on days that animals from either farm were processed. For many of the samples, DNA could not be extracted in sufficient quantity to be analyzed due to low levels of contamination. A sampling area much larger than 300 cm2 would have been necessary to obtain enough DNA. Despite the similarities described above, Dev samples had TAM count means of 5.17 ± 1.58 and 2.96 ± 0.24 Log10 CFU/300 mL and EB means of 3.93 ± 1.35 and 2.88 ± 0.70 Log10 CFU/300 mL for farm-L and farm-H, respectively. Initial counts for Dev appear to vary substantially between sampling days after the pre-operation procedures.
3.3 Microbial contamination from presence of animals on the processing line
Unsurprisingly, as the animals went through the processing line, the CFU in environmental samples rose signficantly. For TAM counts, the increase was significant (p < 0.05) for every sample. The EB counts in the Gev (p = 0.08) and the drain at cut-out (Dcu;p = 0.4) samples were not significantly different from those collected from the clean processing line.
To evaluate contamination of the processing line due to animals, the microbial counts from environmental samples collected after animals under study were processed were subtracted from those measured at the end of the pre-operation procedures. No significant difference (t-test and Wilcoxon) was detected between the farms for any of the microbial counts (Figure 3). There was only a marginal tendency for the cold carcass TAM counts (p = 0.067; t-test), where farm-L had a mean of 4.48 ± 0.24 Log10 CFU/300 cm2 and farm-H had a mean of 4.96 ± 0.24 Log10 CFU/300 cm2, but the difference was below 1 Log10 unit. For Dc, Cc and S samples, TAM counts diminished as the samples were collected further along the processing lines. Means decreased from 4.74 (Dc) and 4.48 (Cc) to 3.08 (S) Log10 CFU/300 cm2 for farm-L, and 4.76 (Dc) and 4.96 (Cc) to 3.39 (S) Log10 CFU/300 cm2 for farm-H. However, EB counts did not follow this trend. Samples increased from a mean of 1.59 (Dc) and 1.33 (Cc) to 2.52 (S) Log10 CFU/300 cm2 for farm-L and 1.71 (Dc) and 1.43 (Cc) to 1.97 (S) Log10 CFU/300 cm2 for farm-H.
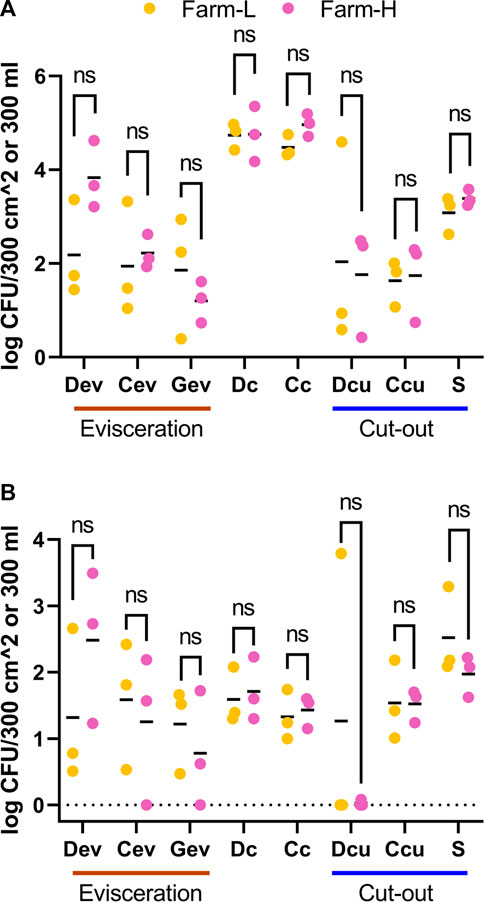
FIGURE 3. Total aerobic mesophilic bacteria (A) and Enterobacteriaceae (B) counts in Log10 CFU/300 cm2 or 300 mL for samples associated with farm-L (yellow) or farm-H (pink), with lower and higher sanitary statuses, respectively. Samples were collected from air (Ar), feces (Fc), saliva (Sa), feed (Fe), drain at evisceration (Dev), conveyor at evisceration (Cev), blood collection gutter (Gev), dressed carcasses (Dc), cold carcasses (Cc), drain at cut-out (Dcu), conveyor at cut-out (Ccu) and shoulder (S) after the last animal under study was processed. The results are the contamination from animals, calculated by subtracting the values obtained for the clean production line. The detection level for drain samples was ≤2.48 Log10 CFU/300 mL for TAM and EB. For the other samples, the detection level was ≤1.22 Log10 CFU/300 cm2 for TAM and ≤0.70 Log10 CFU/300 cm2 for EB.
Various non-microbiological data were collected by inspectors to assess carcass weight and other potential defects (see above for description; data not shown). No significant differences were observed between the two farms for any of the inspection data collected.
3.4 Analysis of microbiota variations across the swine value chain using 16S rDNA amplicons
Overall, Acinetobacter was the most abundant genus across all samples except for in Ar and Fc (Figure 1). These bacteria were found mostly on the surface of dressed (Dc) and cold carcasses (Cc; between 20% and 27% of relative abundance) and were detected at lower abundances across all meat plant environmental samples. Clostridium (Clostiridum_sensu_stricto_1) was particularly abundant in Ar samples (20.5% and 60.2% for farm-L and H, respectively). Fusobacterium, Porphyromonas and Bacteroides were mainly detected on equipment surfaces (Cev, Gev, and Ccu) and S samples. Results varied for Ccu and S samples depending on the farm. Streptococcus was the only genus found across the whole value chain. This genus generally had low abundances (<5.7%) except for farm-L air samples (20.8%). Pseudomonas distribution varied greatly between samples. In Fe samples, Pseudomonas was more abundant at farm-L (16.0%) than farm-H (4.6%). The opposite was found for Ccu (3.7% at farm-L, 15.4% at farm-H). Anoxybacillus abundance was different between the two farms for Dcu (28.5% at farm-L and 1.2% at farm-H). The Anoxybacillus high mean relative abundance at farm-L was caused by one sample with extremely high abundance. This result was consistent with the abnormally high plate counts for TAM and EB in the same contaminated sample (Figure 3; Dcu).
Certain bacterial families have a large impact on the pork value chain from a meat safety and hygiene perspective. Some cause meat spoilage while others increase meat shelf life. Five of those families, Campylobacteraceae, Carnobacteriaceae, Enterobacteriaceae, Lactobacillaceae and Staphylococcaceae, were examined as a subset of the total data. The relative abundances of the genera within this subsample are presented in Figure 4. No Listeriaceae were detected. The abundances of these families were similar between the two farms. Campylobacteraceae were found mostly in Fc, Cev, Gev, and S samples. Carnobacteriaceae were mostly detected at the cut-out area, but were also found across the value chain. Only traces were found in Fc and Fe. Enterobacteriaceae were present only in trace amounts in Ar samples. High abundances of Lactobacillaceae were identified in Ar, Fc, Sa, Dev and on carcass surfaces. Staphylococcaceae were found across the value chain, but only in trace amounts in the Fc and Dev. At the genus level, Allicoccus, Atlantibacter, Companilactobacillus, Corticicoccus, Cronobacter, Mamallicoccus, Serratia, Salinicoccus, Salmonella, and Weisella (p < 0.001) were significantly more abundant in farm-L samples. The only genus that was more abundant in the farm-H samples was Lacticigenium (p < 0.001; Supplementary Figure S4). Escherichia/Shigella were detected for both farms mainly in Sa and Fc, and in Dev, Cev and Gev. The same Escherichia/Shigella ASV detected in Sa and Fc was also found in the meat plant environment (ASV168; Supplementary Table S2). Contrary to Campylobacter, only traces of Escherichia/Shigella were detected on carcasses and the resulting shoulder meat cuts.
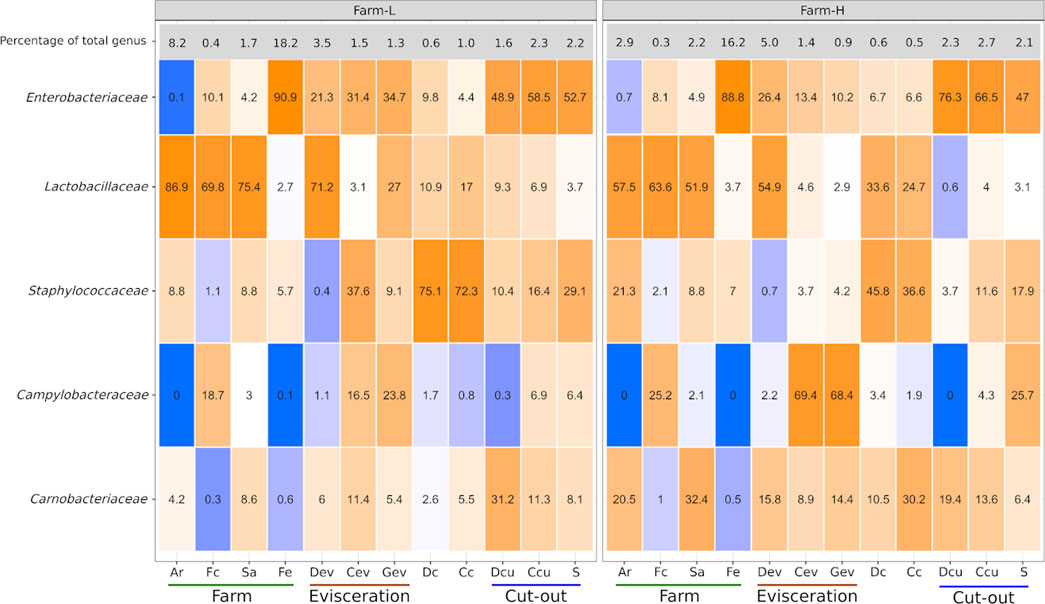
FIGURE 4. Campylobacteraceae, Carnobacteriaceae, Enterobacteriaceae, Lactobacillaceae, and Staphylococcaceae relative abundances (%) to one another calculated for each of the sample types for both farms. The top row indicates the percentage that these five families represented in the total microbiota of each sample type. Colour gradients range from blue = 0% to orange = 100%. Samples were collected from air (Ar), feces (Fc), saliva (Sa), feed (Fe), drain at evisceration (Dev), conveyor at evisceration (Cev), blood collection gutter (Gev), dressed carcasses (Dc), cold carcasses (Cc), drain at cut-out (Dcu), conveyor at cut-out (Ccu) and shoulder (S). Farm-L with a lower and farm-H with a higher sanitary status, respectively.
Alpha diversity was determined for each sample type to evaluate microbial diversity in terms of richness (Observed and Chao1) and both richness and evenness (Shannon and Simpson; Figure 5). There were significant differences between farms for Ar and Fe samples (p < 0.05). Diversity was lowest in Fe samples and highest in Sa samples (p < 0.05; Observed and Chao1). In terms of evenness, the microbiota in the Ar was more uniform for farm-L than for farm-H, while the reverse was observed for the Fe samples. The Simpson index for the Ar samples was distinctive from the other sample types and indicated a clear prevalence of a few taxa, namely, Clostridium in farm-H and Clostridium and Streptococcus in farm-L (Figure 1). As samples were obtained along the different locations and steps in the value chain, there was a clear reduction in microbial diversity. However, there was a large variation in the Shannon and Simpson indexes for farm-L suggesting that the microbiota varied substantially between the three shipment weeks.
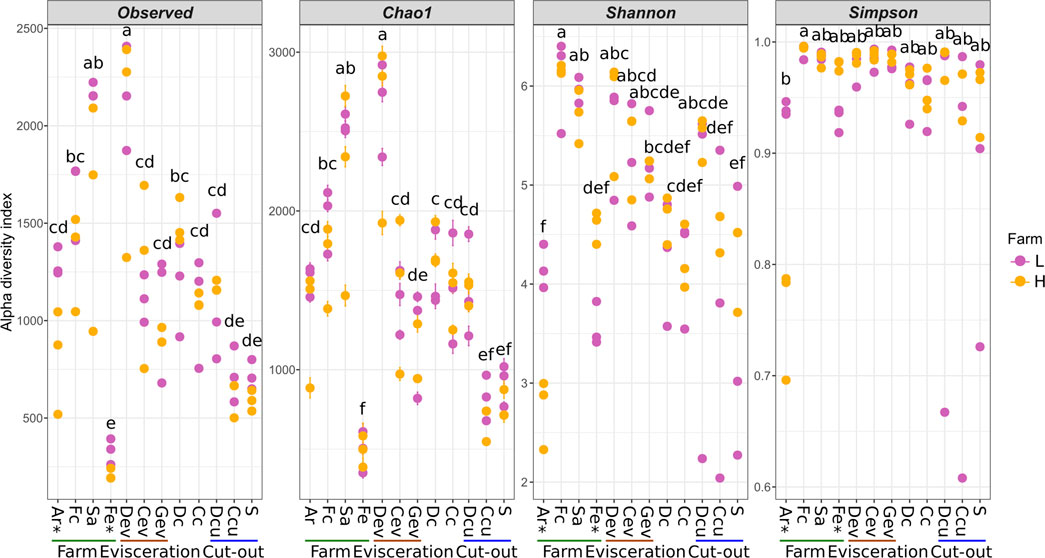
FIGURE 5. Alpha diversity of the samples along the value chain calculated with different indexes for richness (Observed, Chao1) and both richness and evenness (Shannon, Simpson). Significant differences between the two farms according to a Student T test (p < 0.05) are identified with an asterisk (*) at the bottom of the graph. Significantly different samples are identified with different letters according to a Tukey HSD test (p < 0.05). Samples were collected from air (Ar), feces (Fc), saliva (Sa), feed (Fe), drain at evisceration (Dev), conveyor at evisceration (Cev), blood collection gutter (Gev), dressed carcasses (Dc), cold carcasses (Cc), drain at cut-out (Dcu), conveyor at cut-out (Ccu) and shoulder (S). Farm-L (yellow) or farm-H (pink) with lower and higher sanitary statuses, respectively.
Beta diversity analysis was conducted to visualize the between-sample differences in diversity and to identify which factors impact the changes in the microbiota along the value chain (Table 2). Unweighted and weighted UniFrac distances and Bray-Curtis dissimilarities were visualized using PCoA (Figure 6) and an ANOSIM test (p < 0.05) on the distance matrices. Across all distance measures, sample type and location (farms, evisceration area and cut-out area samples) were significant (p < 0.0001) while farms (L and H) and shipment weeks (1, 2, and 3) were not. Sample type had the highest R value indicating that it explained the largest amount of variation. Each sample type had a unique microbiota. They then regrouped themselves based on their position along the value chain (Figure 6). This secondary grouping is defined by the unique microbiota at each farm, the evisceration area, and the cut-out area. In all distance metrics, samples were mostly distributed along the principal coordinate 1 (PCo1) according to their location, indicating that the microbiota at the farms are replaced and became less diverse further down the value chain. The only exceptions were Dev and Fe samples. The Dev samples varied between farms and were distant from the other samples from the meat plant. The Fe samples were more similar to samples from the meat plant than other samples from the farms. Principal coordinates 2 (PCo2) and 3 (PCo3) appear mostly linked to dissimilarities between the different sample types. When considering only abundance (Bray-Curtis dissimilarities; Figures 6A, B), the PCo2 microbiota were mostly influenced by the dissimilarities between Cev and Gev, which is maximal when compared to Dcu. For PCo3, dissimilarities between carcass samples (Dc and Cc) and the rest of the meat plant samples were apparent. When only considering phylogeny (unweighted UniFrac distances; Figures 6C, D) along the PCo2, samples were distributed mostly based on the dissimilarity between Fe and the meat plant samples. On the PCo3 axis, dissimilarities between the Dcu and the rest of the samples were evident. When both abundance and phylogeny is considered (weighted UniFrac distances; Figures 6E, F), the samples were distributed mostly along the PCo2 according to their similarities to Ar or Cev and Gev samples. Along the PCo3, smaller variations are illustrated (axis is narrower) through minor differences between Fe and Ar samples.
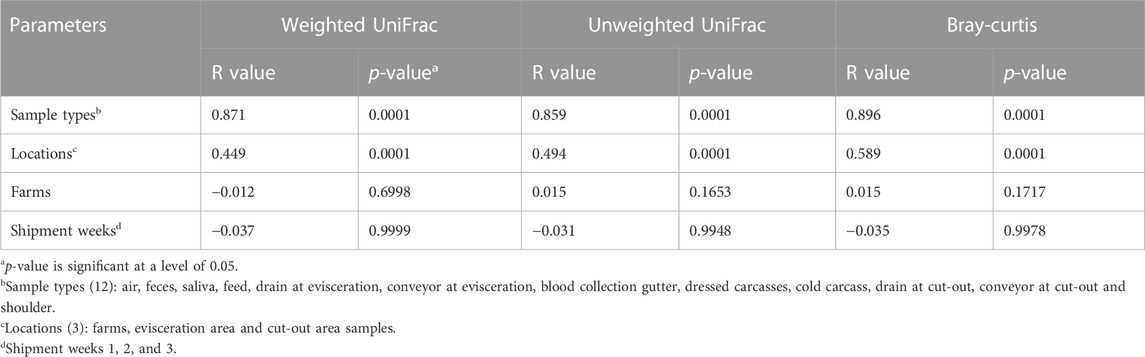
TABLE 2. Factors associated with the microbiota community structure for samples collected along the swine value chain as measured using ANOSIM of the weighted and unweighted UniFrac distances and Bray-Curtis dissimilarities (R value).
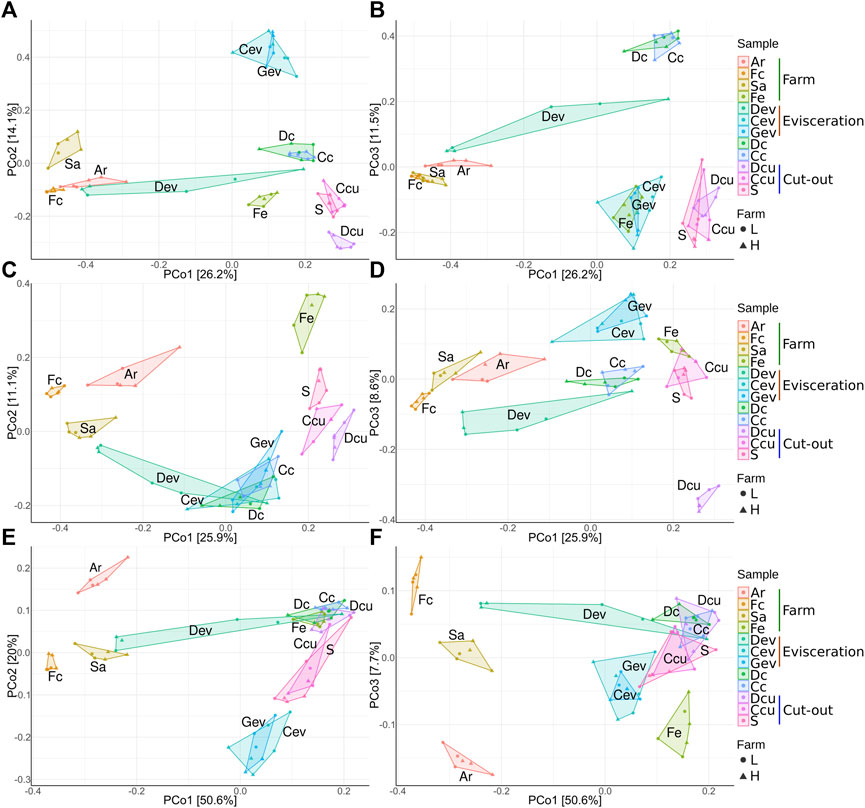
FIGURE 6. Principal-coordinate analysis plots of Bray-Curtis dissimilarities (A,B), unweighted UniFrac distances (C,D), and weighted UniFrac distances (E,F) classified by sample type. The right side (A,C,E) are axes 1 and 2 and the left side (B,D,F) are axes 1 and 3. Samples were collected from air (Ar), feces (Fc), saliva (Sa), feed (Fe), drain at evisceration (Dev), conveyor at evisceration (Cev), blood collection gutter (Gev), dressed carcasses (Dc), cold carcasses (Cc), drain at cut-out (Dcu), conveyor at cut-out (Ccu) and shoulder (S). Farm-L or farm-H with lower and higher sanitary statuses, respectively.
LEfSe analysis was performed in an effort to identify genera that could be used as biomarkers of the farm of origin. No genera were strongly associated with either farm using standard LEfSe parameters (p < 0.05 for the Kruskal-Wallis test and LDA score cut-off of 2). When the severity of the LDA score cutoff was lowered to 1, 12 genera were associated with a specific farm (Figure 7). Flavobacterium and Eubacterium_saphenum_group were associated with farm-H. Anaevibrio, Mageeibacillus, Micrococcus, Megasphaera, Akkermansia, Selenomonas, Ewingella, Deinococcus, Bifidobacterium and Mistsuokella were associated with farm-L. The genera associated with farm-L were found across the entire value chain, whereas the biomarkers for farm-H were mostly present in the meat plant samples. These genera represented a small percentage (<4.5%) of the total relative abundance in all sample types. The one exception is the Fe samples where they make up a larger percentage (8.3% at farm-L and 10.9% at farm-H; Supplementary Figure S5). Of the genera identified, only Anaevibrio, Mageeibacillus, Micrococcus, Megasphaera, Akkermansia and Flavobacterium were over the threshold of 1.5 LDA score. None of the genera identified here had an LDA score of 2 or higher. This indicates that while the Kruskal-Wallis test lists these bacteria as significantly more abundant at one of the farms, linear discriminant analysis shows that they had low relevancy as biomarkers of the farm origin. Inversely, this means that the bacteria that impact the value chain were not affected by which farm they were associated with. This finding is consistent with the results from the ANOSIM analysis (Table 2). However, since only two farms were processed in one plant, that does not mean it is possible, for all commercial organizations, to produce similar meat microbial quality products from animals coming from two different farms.
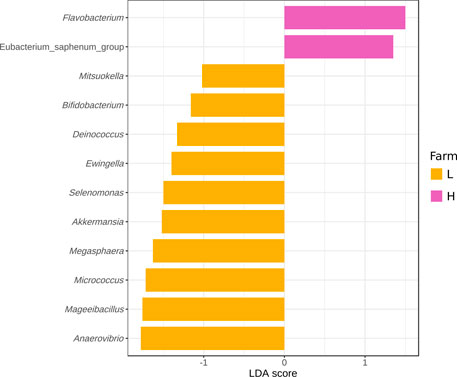
FIGURE 7. Differentially abundant genera across all samples as assessed using linear discriminant analysis (LDA) with effect size (LEfSe) measurements for farm-L (yellow) and farm-H (pink) with lower and higher sanitary statuses, respectively. Only those genera with an LDA score (log10) of >1.0 are displayed.
A source tracking analysis was used to identify possible sources of microbial contamination on the carcasses and shoulder cuts. As shown in Figure 8A, most microorganisms found (as ASVs) in Dc samples did not likely originate from the farm since there were limited ASVs ascribed to them. Farm samples (Ar, Fc, Sa, and Fe) are the source of only 2.2% of the ASVs also found in Dc samples. The contamination sources remain mostly unknown (81%), but 14.4% came from the Dev samples. Figure 8B shows that overnight refrigeration after the initial blast chill did not change the microbiota of carcasses in a major way, since 89.9% of the ASVs were the same in Dc and Cc samples. Figure 8C indicates that most of the microorganisms detected in S samples likely originated from Cc (79.6%). The farm samples (Ar, Fc, Sa, and Fe) represented a total of 3.52% shared ASVs and most of the meat plant samples (Dev, Cev, Gev, Dcu, and Ccu) represent 9.39%.
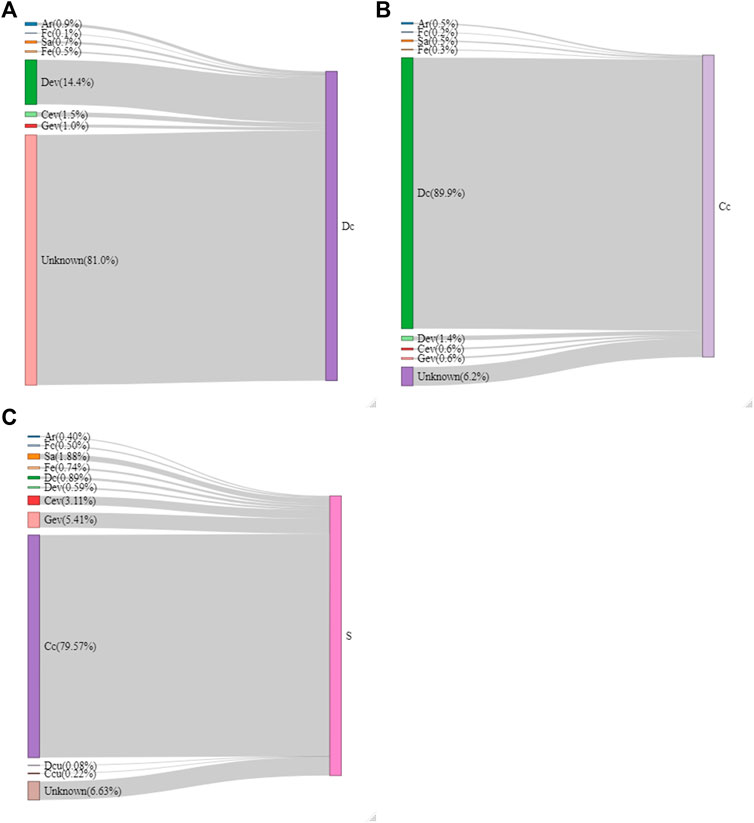
FIGURE 8. Flow diagram of the sources of microorganisms on the dressed carcass [Dc; (A)], cold carcass [Cc; (B)] and the shoulder [S; (C)] samples generated by the SourceTracker software. The proportion (%) that each source represents on the selected meat sample is indicated in parentheses. Samples were collected from air (Ar), feces (Fc), saliva (Sa), feed (Fe), drain at evisceration (Dev), conveyor at evisceration (Cev), blood collection gutter (Gev), drain at cut-out (Dcu), conveyor at cut-out (Ccu).
4 Discussion
In this study, differences in the microbiota in samples taken from multiple steps along the value chain (from farm to meat cut-out area) were compared for two farms with different sanitary statuses. One aim was to measure the contribution of the farm and the meat plant to the microbiota found in the cuts of meat. The strength of our experimental design comes from the fact that the same animals that were raised on commercial farms were followed throughout the entire value chain.
Based on metataxonomic results, the concentration of SCFAs and metabolomic analysis of feces, the two farms were distinctive in terms of their microbiota. This is consistent with the observations made by the veterinarians who selected the farms. The hygiene conditions at farms have been shown to impact the microbiota in the gut of swine (Le Floc’h et al., 2014), their feed (Maciorowski et al., 2007) and the air (Duchaine et al., 2000). Results indicate that the bacterial genera identified in Ar and Fc samples were closely related as expected, since dried fecal particles are a major source of air contamination (Hamscher et al., 2003). Mechanical problems with the ventilation system in farm-H could explain some of the variation observed in the air microbiota. Inefficient ventilation is known to support higher concentrations of total microbes and higher percentages of fecal microbes in the air (Kim and Ko, 2019). These microbes, of which Clostridium are a large part of (Nehme et al., 2008; Song et al., 2021), clearly distinguished the two farms (Figure 1). High alpha diversity in the air in buildings where swine are raised has been linked to higher incidences of antibiotic resistance genes and opportunistic pathogens (Kumari et al., 2016; Yan et al., 2021). In our study, ASV counts in Ar samples were higher than those reported in the literature (Kraemer et al., 2019; Yan et al., 2021). The high observed/ChaO1 alpha diversity we observed may be due, in part, to the pooling of all samples at the sample inference step of the bioinformatic pipeline, which can increase sensitivity to rare ASVs.
Because the feed comes into contact with saliva, it is expected that their bacterial composition might overlap and change with the type of feed. A month prior to sampling, farm-L experienced a salmonellosis outbreak, necessitating a switched to mash feed to control the diarrhea caused by the outbreak (Lo Fo Wong et al., 2004; Mikkelsen Lene et al., 2004; Longpré et al., 2016; Lebel et al., 2017; O'Meara et al., 2020). Mash feed is known to induce higher levels of SCFA in feces, which in turn improve hematological profile of pigs and reduce ulceration, diarrhea levels, as well as Salmonella and E. coli shedding (Lo Fo Wong et al., 2004; Mikkelsen Lene et al., 2004; Longpré et al., 2016; Lebel et al., 2017; O'Meara et al., 2020). Our Lefse analysis confirmed that the mash feed promoted well-known SCFA-producing bacteria, likely causing the higher concentration of SCFAs in the feces of animals from farm-L. This may also have contributed to stabilizing the microbiota, resulting in an alpha diversity similar to that of the feces from farm-H. Mash feed is also known to harbor higher bacterial counts than traditional hot pelleted feed (Mikkelsen Lene et al., 2004; Paramithiotis et al., 2009; O'Meara et al., 2020). Hence, the type of feed was an important factor in determining the differences between the two farms in our study.
The meat plant was clean before any of the animals under study were processed, allowing us to assess the contamination that came from the animals. Environmental samples of various types have been reported to range from 2 to 6 Log10 of CFU/cm2 for TAM and from undetected to 5 Log10 of CFU/cm2 for EB (Warriner et al., 2002; Bridier et al., 2019; Maes et al., 2019). Our microbial counts for TAM and EB were below those values in Cev, Gev, Ccu, and Dcu samples. Only the Dev samples exhibited highly variable levels of contamination over time. This is consistent with the literature which suggests that processing plant drains are a source of contamination, notably through aerosol formation (Byrne et al., 2008), and they are a main contributor to the contamination variation and evolution of a processing plant over time (ICMSF, 2018).
Overall, contamination level of the meat plant was similar after the animals under study were processed for both farms. However, season is an important contributing factor of contamination in swine raising environments (Kumari et al., 2016) and its effect should be addressed over a longer period of time. Carcass (Dc and Cc; Figure 2) and drain samples (Dev and Dcu; Figure 3) had similar numbers of TAM and EB compared to the range reported in the literature (Spescha et al., 2006; Gill and Badoni, 2010; Zwirzitz et al., 2020), whereas Cev, Gev and Ccu samples (Figure 3) were lower (Warriner et al., 2002; Bridier et al., 2019; Zwirzitz et al., 2020). Total aerobic mesophilic, Enterobacteriaceae and coliform counts increased on the processing equipment over time (Warriner et al., 2002). The low counts observed may be explained by the fact that the animals examined were the first of the day to be slaughtered. They spent less than an hour on the processing line, which processed 500 animals per hour. Gev and Dcu samples had a significant increase in TAM after the animals were processed (Figure 3). This was not the case for the EB counts. Gev mainly collected blood and if swine were healthy, their blood contained a limited number of microbes (Hyun et al., 2021). This may explain why EB counts did not change over the short sampling period in Gev samples. Hot water flowed through Dcu during operation. Because enterobacteria do not form spores (Brenner and Farmer, 2015), they likely could not survive in those conditions. Cell counts suggest that contamination risks are similar for both farms. The slightly higher relative abundance of Salmonella in samples from farm-L might suggest otherwise, although only trace amounts were detected (0.036% relative abundance in Fe and 0.0045% on Cev).
Alpha diversity decreased in samples along the value chain, suggesting that the microbiota become less complex especially for Dc, Cc, and S samples. This progressive diversity reduction is partially explained by the heat treatment (scalding and singeing) that is part of processing the animal, and the subsequent dehairing and polishing procedures. These steps remove a large portion of the skin microbiota which could be recontaminated by the spreading of resident bacteria from the meat plant onto the carcasses (Gill and Bryant, 1993; Gill, 2000; Wheatley et al., 2014; Zwirzitz et al., 2020). In Zwirzitz et al. (2020), alpha diversity decreased between the arrival of animals at the slaughterhouse and singeing, increased again at the polishing step, and then decreased continuously until carcasses were shipped to a meat cutting facility. This indicates that polishing is a critical step in replacing the animal microbiota on the surface of the carcasses with resident bacteria from the slaughterhouse. Clearly, the processing steps prior to the overnight refrigeration warrant further investigation. In our study, this process could explain how the beta diversity for carcasses from both farms were not significantly different. The sample type and location (farm vs. evisceration area vs. cut-out area) significantly affected the structure of the microbiota community (Table 2).
There are indications that some of the bacteria from the farms were still present on the carcasses and the shoulder samples. Escherichia/Shigella were detected mainly in Sa and Fe samples at the farms and in Dev, Cev, and Gev samples at the evisceration area. The same Escherichia/Shigella ASV detected in Sa and Fc samples were also found in all meat plant samples (ASV168; Supplementary Table S2) and traces were detected in Cc and S samples. Campylobacter, the most common pathogen in the swine value chain (Farzan et al., 2010; Baer et al., 2013), was detected in Sa, Fe, and S samples. The data revealed that the ASVs detected in the S samples were not the same as those found in Sa, Fe, Sa, Dev, and Dcu (Supplementary Table S2) and were instead the same as Cev, Gev, and Ccu. This suggests that a portion of the detected Campylobacter might be from meat plant surfaces. Furthermore, Carnobacterium were detected almost exclusively at the cut-out location, indicating that these bacteria are from the meat plant. These bacteria are nearly absent on carcasses but were identified on S samples. Interestingly, this genus, along with many Enterobacteriaceae (Supplementary Figure S2; Enterobacter, Citrobacter, Buttilauxella, Lelliottia and Kluyvera), replaced Lactobacillus, Moraxella and Staphylococcus on S samples. This could explain why Enterobacteriaceae counts increased on the shoulders instead of following the general downward trend of the total aerobic mesophilic counts after carcass breakdown. Multiple studies (Gill and Bryant, 1993; Gill et al., 1999; Gill, 2000; Gill and Sofos, 2005) investigated the impact of carcass breakdown on meat microbiota. Multiple types of bacteria are transferred from the equipment, workers, etc. to the resulting meat cuts during the processing steps in the value chain, further replacing any bacteria from the farm. The source tracking analysis confirms that only 12.92% of the microbiota on S samples originated from the farms and the meat plant environment (Figure 8C).
Overall, 81% of the bacteria detected in the Dc samples were of unknown origin (Figure 8A) indicating that much remains to be investigated to understand the sources of contamination found in the final cuts of meat. Dehairing and polishing processes would be valuable steps to consider in determining sources of contamination (Gill, 2000). Once carcasses were blast chilled, the microbiota was less susceptible to variation between Dc, Cc and S samples; 89.9% of the bacteria found in the Cc samples came from the Dc and 79.6% of the bacteria found on S samples came from Cc. Refrigeration favors the development of a more psychrotrophic microbiota (Zwirzitz et al., 2020) and on S samples some cold-tolerant bacteria were detected, as the cut-out area was maintained at 7°C at all times (Figure 1; Pseudomonas, Psychrobacter; Juni, 2015; Palleroni, 2015).
5 Conclusion
The variability in the microbiota observed in samples along the value chain were more associated with the location (farm, evisceration area, cut-out area) and sample type rather than the farm of origin or the shipment week. The microbes identified on each farm were largely controlled by in-house operational procedures. This is evidenced by the fact that less than 4% of bacteria identified in the S samples, the last step in the value chain that was examined, were determined to be from the farms. In fact, a more homogeneous and less complex microbiota was observed as we moved forward along the value chain. However, it would be presumptuous to claim that meat plants completely decontaminate animals from their initial microbiota acquired at the farm regardless of their sanitary status since Salmonella was found in low relative abundance with Fe and Cev samples associated to farm-L. The results rather suggest that the microbial control that is presently achieved by farms makes it possible to produce pork products with adaquate microbiological quality. Nevertheless, a broader study that includes more farms with different sanitary statuses is needed to accurately determine the impact and magnitude of farm sanitary status on the final commercial pork products knowing that many factors such as season, feed form and composition, housing conditions, preslaughter management, etc., are also contributing factors.
Data availability statement
The datasets presented in this study can be found in online repositories. The names of the repository/repositories and accession number(s) can be found in the article/Supplementary Material.
Ethics statement
The animal study was reviewed and approved by the Université Laval’s Animal Use and Care Committee (2019-329). Written informed consent was obtained from the owners for the participation of their animals in this study.
Author contributions
Conceptualization, LS and SF; methodology, PL, CD, ÉP, and PF; software, AV, AD-F, P-LP, and PL; validation, LS, P-LP, and AV; investigation, PL; resources, LS and CD; data curation, PL, AD-F, P-LP, and AV; writing‒original draft preparation, PL; writing‒review and editing, LS and AV; visualization, PL; supervision and project administration, LS, ÉP, and SF; funding acquisition, LS and SF. All authors contributed to the article and approved the submitted version.
Funding
This research is part of the Canadian Food Innovation Network (CFIN; ASC-14) science cluster and funded in part by the Government of Canada under the Canadian Agricultural Partnership’s AgriScience Program, which is a federal, provincial, and territorial initiative. It was also funded by the Ministère de l’Agriculture, des Pêcheries et de l’Alimentation du Québec (MAPAQ) as part of the Chaire de recherche MAPAQ sur la qualité et la salubrité de la viande et des produits de viande transformée (Musculo; PPIA13). Sollio Cooperative Group was a partner in this project.
Acknowledgments
We acknowledge Dr. B. Boucher and Dr. J. Brochu for their valuable contributions as veterinarians. The authors thank the Institute of Nutrition and Functional Foods platforms and the Institute of Integrative Biology and Systems for their technical assistance.
Conflict of interest
The authors AV and LS declared that they were editorial board members of Frontiers at the time of submission. This had no impact on the peer review process and the final decision. Authors ÉP and SF were employed by the company Olymel S.E.C./L.P.
The remaining authors declare that the research was conducted in the absence of any commercial or financial relationships that could be construed as a potential conflict of interest.
Publisher’s note
All claims expressed in this article are solely those of the authors and do not necessarily represent those of their affiliated organizations, or those of the publisher, the editors and the reviewers. Any product that may be evaluated in this article, or claim that may be made by its manufacturer, is not guaranteed or endorsed by the publisher.
Supplementary material
The Supplementary Material for this article can be found online at: https://www.frontiersin.org/articles/10.3389/fsysb.2023.1183868/full#supplementary-material
References
Adhikari, B., Kim, S. W., and Kwon, Y. M. (2019). Characterization of microbiota associated with digesta and mucosa in different regions of gastrointestinal tract of nursery pigs. Int. J. Mol. Sci. 20 (7), 1630. doi:10.3390/ijms20071630
Altschul, S. F., Gish, W., Miller, W., Myers, E. W., and Lipman, D. J. (1990). Basic local alignment search tool. J. Mol. Biol. 215 (3), 403–410. doi:10.1016/S0022-2836(05)80360-2
Andersen, K. S., Kirkegaard, R. H., Karst, S. M., and Albertsen, M. (2018). ampvis2: an R package to analyse and visualise 16S rRNA amplicon data. bioRxiv 1, 299537. doi:10.1101/299537
Andrews, S. (2010). FastQC: A quality control tool for high throughput sequence data. available at: http://www.bioinformatics.babraham.ac.uk/projects/fastqc/.
Baer, A. A., Miller, M. J., and Dilger, A. C. (2013). Pathogens of interest to the pork industry: A review of research on interventions to assure food safety. Compr. Rev. Food Sci. Food Saf. 12 (2), 183–217. doi:10.1111/1541-4337.12001
Brenner, D. J., and Farmer, J. J. (2015). Enterobacteriaceae. Bergey's Man. Syst. Archaea Bact. 24, 1. doi:10.1002/9781118960608.fbm00222
Bridier, A., Le Grandois, P., Moreau, M.-H., Prénom, C., Le Roux, A., Feurer, C., et al. (2019). Impact of cleaning and disinfection procedures on microbial ecology and Salmonella antimicrobial resistance in a pig slaughterhouse. Sci. Rep. 9 (1), 12947. doi:10.1038/s41598-019-49464-8
Byrne, B., Lyng, J., Dunne, G., and Bolton, D. J. (2008). An assessment of the microbial quality of the air within a pork processing plant. Food control 19 (9), 915–920. doi:10.1016/j.foodcont.2007.08.016
Callahan, B. J., McMurdie, P. J., Rosen, M. J., Han, A. W., Johnson, A. J. A., and Holmes, S. P. (2016). DADA2: High-resolution sample inference from Illumina amplicon data. Nat. Methods 13 (7), 581–583. doi:10.1038/nmeth.3869
Cameron, R. D. A. (2000). A review of the industrialisation of pig production worldwide with particular reference to the Asian region. Animal Health and Area-wide Integration. Brisbane, Australia: FAO.
Crespo-Piazuelo, D., Estellé, J., Revilla, M., Criado-Mesas, L., Ramayo-Caldas, Y., Óvilo, C., et al. (2018). Characterization of bacterial microbiota compositions along the intestinal tract in pigs and their interactions and functions. Sci. Rep. 8 (1), 12727. doi:10.1038/s41598-018-30932-6
Davis, N. M., Proctor, D. M., Holmes, S. P., Relman, D. A., and Callahan, B. J. (2018). Simple statistical identification and removal of contaminant sequences in marker-gene and metagenomics data. Microbiome 6 (1), 226. doi:10.1186/s40168-018-0605-2
Dhariwal, A., Chong, J., Habib, S., King, I. L., Agellon, L. B., and Xia, J. (2017). MicrobiomeAnalyst: A web-based tool for comprehensive statistical, visual and meta-analysis of microbiome data. Nucleic Acids Res. 45 (W1), W180–W188. doi:10.1093/nar/gkx295
Duchaine, C., Grimard, Y., and Cormier, Y. (2000). Influence of building maintenance, environmental factors, and seasons on airborne contaminants of swine confinement buildings. Aihaj 61 (1), 56–63. doi:10.1080/15298660008984515
Farzan, A., Friendship, R. M., Cook, A., and Pollari, F. (2010). Occurrence of Salmonella, Campylobacter, Yersinia enterocolitica, Escherichia coli O157 and Listeria monocytogenes in swine. Zoonoses Public Health 57 (6), 388–396. doi:10.1111/j.1863-2378.2009.01248.x
Fosse, J., Oudot, N., Laroche, M., Rossero, A., Seegers, H., H., and Magras, C. (2008). Infection of pigs batches by five bacterial food-borne zoonotic hazards: Variability on farm and at slaughter. Epidémiologie Santé Animale (53), 57–71.
Gill, C. O. (2000). “Chapter 5 - HACCP in primary processing: Red meat, p 81-122,” in HACCP in the meat industry (Duxford, United Kingdom: Woodhead Publishing).
Gill, C. O., and Sofos, J. N. (2005). “Chapter 11 - sources of microbial contamination at slaughtering plants,” in Improving the safety of fresh meat (Cambridge, United Kingdom: Woodhead Publishing Limited), 231–243.
Gill, C. O., and Badoni, M. (2010). Effects of experience with swabbing procedures on the numbers of bacteria recovered from carcasses by swabbing with sponges. J. Food Prot. 73 (4), 747–751. doi:10.4315/0362-028x-73.4.747
Gill, C. O., Badoni, M., and McGinnis, J. C. (1999). Assessment of the adequacy of cleaning of equipment used for breaking beef carcasses. Int. J. Food Microbiol. 46 (1), 1–8. doi:10.1016/S0168-1605(98)00181-0
Gill, C. O., and Bryant, J. (1993). The presence of Escherichia coli, Salmonella and Campylobacter in pig carcass dehairing equipment. Food Microbiol. 10 (4), 337–344. doi:10.1006/fmic.1993.1039
Hamscher, G., Pawelzick, H. T., Sczesny, S., Nau, H., and Hartung, J. (2003). Antibiotics in dust originating from a pig-fattening farm: A new source of health hazard for farmers? Environ. Health Perspect. 111 (13), 1590–1594. doi:10.1289/ehp.6288
Health Canada (2020). Determination of the aerobic colony counts in foods, MFHPB-18. Compend. Anal. methods 2, 1.
Holman, D. B., Gzyl, K. E., Mou, K. T., Allen, H. K., and Cotter, P. D. (2021). Weaning age and its effect on the development of the swine gut microbiome and resistome. mSystems 6(6), 00682211-e100621. doi:10.1128/mSystems.00682-21
Holman, D., W Brunelle, B., Trachsel, J., and Allen, H. (2017). Meta-analysis to define a core microbiota in the swine gut. mSystems 2 (3), e00004. doi:10.1128/mSystems.00004-17
Huang, T., Zhang, M., Tong, X., Chen, J., Yan, G., Fang, S., et al. (2019). Microbial communities in swine lungs and their association with lung lesions. Microb. Biotechnol. 12 (2), 289–304. doi:10.1111/1751-7915.13353
Huffman, R. D. (2002). Current and future technologies for the decontamination of carcasses and fresh meat. Meat Sci. 62 (3), 285–294. doi:10.1016/S0309-1740(02)00120-1
Hurd, H. S., Brudvig, J., Dickson, J., Mirceta, J., Polovinski, M., Matthews, N., et al. (2008). Swine health impact on carcass contamination and human foodborne risk. Public Health Rep. 123 (3), 343–351. doi:10.1177/003335490812300314
Hyun, H., Lee, M. S., Park, I., Ko, H. S., Yun, S., Jang, D.-H., et al. (2021). Analysis of porcine model of fecal-induced peritonitis reveals the tropism of blood microbiome. Front. Cell. Infect. Microbiol. 11, 676650. doi:10.3389/fcimb.2021.676650
International Commission on Microbiological Specifications for Foods (ICMSF) (2018). “Chapter 12 - sampling to assess control of the environment,” in Microorganisms in foods 7: Microbiological testing in food safety management (Cham, Switzerland: Springer International Publishing), 263–292.
Juni, E. (2015). Psychrobacter. Bergey's manual of systematics of archaea and bacteria. New York, NY: John Wiley & Sons, Inc.
Kalton, G., and Flores-Cervantes, I. (2003). Weighting methods. J. official statistics 19 (2), 81–97.
Kim, H. B., and Isaacson, R. E. (2015). The pig gut microbial diversity: Understanding the pig gut microbial ecology through the next generation high throughput sequencing. Veterinary Microbiol. 177 (3), 242–251. doi:10.1016/j.vetmic.2015.03.014
Kim, J., Nguyen, S. G., Guevarra, R. B., Lee, I., and Unno, T. (2015). Analysis of swine fecal microbiota at various growth stages. Archives Microbiol. 197 (6), 753–759. doi:10.1007/s00203-015-1108-1
Kim, K. Y., and Ko, H. J. (2019). Indoor distribution characteristics of airborne bacteria in pig buildings as influenced by season and housing type. Asian-Australas J. Animal Sci. 32 (5), 742–747. doi:10.5713/ajas.18.0415
Klindworth, A., Pruesse, E., Schweer, T., Peplies, J., Quast, C., Horn, M., et al. (2012). Evaluation of general 16S ribosomal RNA gene PCR primers for classical and next-generation sequencing-based diversity studies. Nucleic Acids Res. 41 (1), e1. doi:10.1093/nar/gks808
Knights, D., Kuczynski, J., Charlson, E. S., Zaneveld, J., Mozer, M. C., Collman, R. G., et al. (2011). Bayesian community-wide culture-independent microbial source tracking. Nat. methods 8 (9), 761–763. doi:10.1038/nmeth.1650
Kraemer, J. G., Aebi, S., Oppliger, A., and Hilty, M. (2019). The indoor-air microbiota of pig farms drives the composition of the pig farmers' nasal microbiota in a season-dependent and farm-specific manner. Appl. Environ. Microbiol. 85 (9), e03038-18–e03018. doi:10.1128/AEM.03038-18
Kumari, P., Woo, C., Yamamoto, N., and Choi, H.-L. (2016). Variations in abundance, diversity and community composition of airborne fungi in swine houses across seasons. Sci. Rep. 6 (1), 37929. doi:10.1038/srep37929
Le Floc’h, N., Knudsen, C., Gidenne, T., Montagne, L., Merlot, E., and Zemb, O. (2014). Impact of feed restriction on health, digestion and faecal microbiota of growing pigs housed in good or poor hygiene conditions. Animal 8 (10), 1632–1642. doi:10.1017/S1751731114001608
Lebel, P., Letellier, A., Longpré, J., Laplante, B., Yergeau, E., and Fravalo, P. (2017). Feed presentation options in swine early fattening mitigates Salmonella shedding and specifically modulates the faecal microbiota. J. Appl. Microbiol. 122 (1), 30–39. doi:10.1111/jam.13305
Legendre, P., and Gallagher, E. D. (2001). Ecologically meaningful transformations for ordination of species data. Oecologia 129 (2), 271–280. doi:10.1007/s004420100716
Li, Z., Hu, G., Zhu, L., Sun, Z., Jiang, Y., Gao, M.-J., et al. (2021). Study of growth, metabolism, and morphology of Akkermansia muciniphila with an in vitro advanced bionic intestinal reactor. BMC Microbiol. 21 (1), 61. doi:10.1186/s12866-021-02111-7
Lin, H., and Peddada, S. D. (2020). Analysis of compositions of microbiomes with bias correction. Nat. Commun. 11 (1), 3514. doi:10.1038/s41467-020-17041-7
Lo Fo Wong, D. M. A., Dahl, J., Stege, H., van der Wolf, P. J., Leontides, L., von Altrock, A., et al. (2004). Herd-level risk factors for subclinical Salmonella infection in European finishing-pig herds. Prev. Veterinary Med. 62 (4), 253–266. doi:10.1016/j.prevetmed.2004.01.001
Longpré, J., Fairbrother, J. M., Fravalo, P., Arsenault, J., LeBel, P., Laplante, B., et al. (2016). Impact of mash feeding versus pellets on propionic/butyric acid levels and on total Escherichia coli load in the gastrointestinal tract of growing pigs1. J. Animal Sci. 94 (3), 1053–1063. doi:10.2527/jas.2015-9617
Lozupone, C., and Knight, R. (2005). UniFrac: A new phylogenetic method for comparing microbial communities. Appl. Environ. Microbiol. 71 (12), 8228–8235. doi:10.1128/AEM.71.12.8228-8235.2005
Maciorowski, K. G., Herrera, P., Jones, F. T., Pillai, S. D., and Ricke, S. C. (2007). Effects on poultry and livestock of feed contamination with bacteria and fungi. Animal Feed Sci. Technol. 133 (1), 109–136. doi:10.1016/j.anifeedsci.2006.08.006
Maes, S., Heyndrickx, M., Vackier, T., Steenackers, H., Verplaetse, A., and Reu, K. D. (2019). Identification and spoilage potential of the remaining dominant microbiota on food contact surfaces after cleaning and disinfection in different food industries. J. Food Prot. 82 (2), 262–275. doi:10.4315/0362-028X.JFP-18-226
Mann, E., Wetzels, S. U., Pinior, B., Metzler-Zebeli, B. U., Wagner, M., and Schmitz-Esser, S. (2016). Psychrophile spoilers dominate the bacterial microbiome in musculature samples of slaughter pigs. Meat Sci. 117, 36–40. doi:10.1016/j.meatsci.2016.02.034
Mark Welch, J. L., Dewhirst, F. E., and Borisy, G. G. (2019). Biogeography of the oral microbiome: The site-specialist hypothesis. Annu. Rev. Microbiol. 73 (1), 335–358. doi:10.1146/annurev-micro-090817-062503
Markowiak-Kopeć, P., and Śliżewska, K. (2020). The Effect of probiotics on the production of short-chain fatty acids by human intestinal microbiome. Nutrients 12 (4), 1107. doi:10.3390/nu12041107
McMurdie, P. J., and Holmes, S. (2013). phyloseq: An R package for reproducible interactive analysis and graphics of microbiome census data. PLOS ONE 8 (4), e61217. doi:10.1371/journal.pone.0061217
Meer, Y. v. d., Gerrits, W. J. J., Jansman, A. J. M., Kemp, B., and Bolhuis, J. E. (2017). A link between damaging behaviour in pigs, sanitary conditions, and dietary protein and amino acid supply. PLOS ONE 12 (5), e0174688. doi:10.1371/journal.pone.0174688
Mendiburu, F., and Yaseen, M. (2021). agricolae: Statistical procedures for agricultural research. Available at https://cran.r-project.org/package=agricolae.
Mikkelsen Lene, L., Naughton Patrick, J., Hedemann Mette, S., and Jensen Bent, B. (2004). Effects of physical properties of feed on microbial ecology and survival of Salmonella enterica serovar Typhimurium in the pig gastrointestinal tract. Appl. Environ. Microbiol. 70 (6), 3485–3492. doi:10.1128/AEM.70.6.3485-3492.2004
Møretrø, T., and Langsrud, S. (2017). Residential bacteria on surfaces in the food industry and their implications for food safety and quality. Compr. Rev. Food Sci. Food Saf. 16 (5), 1022–1041. doi:10.1111/1541-4337.12283
Mou, K. T., Allen, H. K., Alt, D. P., Trachsel, J., Hau, S. J., Coetzee, J. F., et al. (2019). Shifts in the nasal microbiota of swine in response to different dosing regimens of oxytetracycline administration. Veterinary Microbiol. 237, 108386. doi:10.1016/j.vetmic.2019.108386
Murase, K., Watanabe, T., Arai, S., Kim, H., Tohya, M., Ishida-Kuroki, K., et al. (2019). Characterization of pig saliva as the major natural habitat of Streptococcus suis by analyzing oral, fecal, vaginal, and environmental microbiota. PLOS ONE 14 (4), e0215983. doi:10.1371/journal.pone.0215983
Nehme, B., Létourneau, V., Forster, R. J., Veillette, M., and Duchaine, C. (2008). Culture-independent approach of the bacterial bioaerosol diversity in the standard swine confinement buildings, and assessment of the seasonal effect. Environ. Microbiol. 10 (3), 665–675. doi:10.1111/j.1462-2920.2007.01489.x
O'Meara, F. M., Gardiner, G. E., O'Doherty, J. V., and Lawlor, P. G. (2020). The effect of feed form and delivery method on feed microbiology and growth performance in grow-finisher pigs. J. animal Sci. 98 (3), skaa021. skaa021. doi:10.1093/jas/skaa021
Painter, J. A., Hoekstra, R. M., Ayers, T., Tauxe, R. V., Braden, C. R., Angulo, F. J., et al. (2013). Attribution of foodborne illnesses, hospitalizations, and deaths to food commodities by using outbreak data, United States, 1998-2008. Emerg. Infect. Dis. 19 (3), 407–415. doi:10.3201/eid1903.111866
Palleroni, N. J. (2015). Pseudomonas. Bergey's manual of systematics of archaea and bacteria. New York, NY: John Wiley & Sons, Inc.
Paramithiotis, S., Pappa, A. M., Drosinos, E. H., and Zoiopoulos, P. E. (2009). Microbiological, physico-chemical and safety parameters of cereal-based animal diets. Qual. Assur. Saf. Crops Foods 1 (3), 170–178. doi:10.1111/j.1757-837X.2009.00028.x
Prodan, A., Tremaroli, V., Brolin, H., Zwinderman, A. H., Nieuwdorp, M., and Levin, E. (2020). Comparing bioinformatic pipelines for microbial 16S rRNA amplicon sequencing. PLOS ONE 15 (1), e0227434. doi:10.1371/journal.pone.0227434
Pruesse, E., Quast, C., Knittel, K., Fuchs, B. M., Ludwig, W., Peplies, J., et al. (2007). Silva: A comprehensive online resource for quality checked and aligned ribosomal RNA sequence data compatible with ARB. Nucleic Acids Res. 35 (21), 7188–7196. doi:10.1093/nar/gkm864
Puertollano, E., Kolida, S., and Yaqoob, P. (2014). Biological significance of short-chain fatty acid metabolism by the intestinal microbiome. Curr. Opin. Clin. Nutr. Metabolic Care 17 (2), 139–144. doi:10.1097/mco.0000000000000025
Quan, J., Cai, G., Ye, J., Yang, M., Ding, R., Wang, X., et al. (2018). A global comparison of the microbiome compositions of three gut locations in commercial pigs with extreme feed conversion ratios. Sci. Rep. 8 (1), 4536. doi:10.1038/s41598-018-22692-0
Ramsay, D., and Delisle, M-F. (2017). Bilan annuel 2014-2015 - toxi-infections alimentaires. Québec. Available at: https://www.mapaq.gouv.qc.ca/fr/Publications/Bilan_Toxi-infection_2014-2015_Accessible.pdf (Accessed May 08, 2019).
Remenant, B., Jaffrès, E., Dousset, X., Pilet, M.-F., and Zagorec, M. (2015). Bacterial spoilers of food: Behavior, fitness and functional properties. Food Microbiol. 45, 45–53. doi:10.1016/j.fm.2014.03.009
Roussel, C., Chabaud, S., Lessard-Lord, J., Cattero, V., Pellerin, F. A., Feutry, P., et al. (2022). UPEC colonic-virulence and urovirulence are blunted by proanthocyanidins-rich cranberry extract microbial metabolites in a gut model and a 3D tissue-engineered urothelium. Microbiol. Spectr. 10 (5), e0243221. doi:10.1128/spectrum.02432-21
Ruttkies, C., Schymanski, E. L., Wolf, S., Hollender, J., and Neumann, S. (2016). MetFrag relaunched: Incorporating strategies beyond in silico fragmentation. J. Cheminformatics 8 (1), 3. doi:10.1186/s13321-016-0115-9
Saucier, L. (2016). Microbial spoilage, quality and safety within the context of meat sustainability. Meat Sci. 120, 78–84. doi:10.1016/j.meatsci.2016.04.027
Schliep, K. P. (2010). phangorn: phylogenetic analysis in R. Bioinformatics 27 (4), 592–593. doi:10.1093/bioinformatics/btq706
Segata, N., Izard, J., Waldron, L., Gevers, D., Miropolsky, L., Garrett, W. S., et al. (2011). Metagenomic biomarker discovery and explanation. Genome Biol. 12 (6), R60. doi:10.1186/gb-2011-12-6-r60
Song, L., Wang, C., Jiang, G., Ma, J., Li, Y., Chen, H., et al. (2021). Bioaerosol is an important transmission route of antibiotic resistance genes in pig farms. Environ. Int. 154, 106559. doi:10.1016/j.envint.2021.106559
Spescha, C., Stephan, R., and Zweifel, C. (2006). Microbiological contamination of pig carcasses at different stages of slaughter in two European Union–Approved abattoirs. J. Food Prot. 69 (11), 2568–2575. doi:10.4315/0362-028X-69.11.2568
Stärk, K., Miserez, R., Siegmann, S., Ochs, H., Infanger, P., and Schmidt, J. (2008). Erradicación de las enfermedades respiratorias enzoóticas de los cerdos mediante un programa de control nacional en suiza: -EN- A successful national control programme for enzootic respiratory diseases in pigs in Switzerland -fr- un programme réussi de lutte contre les maladies respiratoires enzootiques des porcs en Suisse -es-. Revue Sci. Tech. Int. Office Epizootics) 26, 595–606. doi:10.20506/rst.26.3.1768
Vigors, S., O’ Doherty, J. V., and Sweeney, T. (2020). Colonic microbiome profiles for improved feed efficiency can be identified despite major effects of farm of origin and contemporary group in pigs. Animal 14 (12), 2472–2480. doi:10.1017/S1751731120001500
Wang, M., Wichienchot, S., He, X., Fu, X., Huang, Q., and Zhang, B. (2019). In vitro colonic fermentation of dietary fibers: Fermentation rate, short-chain fatty acid production and changes in microbiota. Trends Food Sci. Technol. 88, 1–9. doi:10.1016/j.tifs.2019.03.005
Wang, Q., Cai, R., Huang, A., Wang, X., Qu, W., Shi, L., et al. (2018). Comparison of oropharyngeal microbiota in healthy piglets and piglets with respiratory disease. Front. Microbiol. 9, 3218. doi:10.3389/fmicb.2018.03218
Wang, X., Li, X., Zhao, C., Hu, P., Chen, H., Liu, Z., et al. (2012). Correlation between composition of the bacterial community and concentration of volatile fatty acids in the rumen during the transition period and ketosis in dairy cows. Appl. Environ. Microbiol. 78 (7), 2386–2392. doi:10.1128/AEM.07545-11
Warriner, K., Aldsworth, T. G., Kaur, S., and Dodd, C. E. R. (2002). Cross-contamination of carcasses and equipment during pork processing. J. Appl. Microbiol. 93 (1), 169–177. doi:10.1046/j.1365-2672.2002.01678.x
Wheatley, P., Giotis, E. S., and McKevitt, A. I. (2014). Effects of slaughtering operations on carcass contamination in an Irish pork production plant. Ir. Veterinary J. 67 (1), 1. doi:10.1186/2046-0481-67-1
Wright, E. S. (2016). Using DECIPHER v2.0 to analyze big biological sequence data in R. R J. 8, 352–359. doi:10.32614/rj-2016-025
Xiao, Y., Kong, F., Xiang, Y., Zhou, W., Wang, J., Yang, H., et al. (2018). Comparative biogeography of the gut microbiome between Jinhua and Landrace pigs. Sci. Rep. 8 (1), 5985. doi:10.1038/s41598-018-24289-z
Yan, H., Li, Y., Zhang, Y., Zhang, H., Guo, Z., and Liu, J. (2021). Deciphering of microbial diversity and antibiotic resistome of bioaerosols in swine confinement buildings. Sci. Total Environ. 781, 147056. doi:10.1016/j.scitotenv.2021.147056
Zagorec, M., and Champomier-Vergès, M-C. (2017). “Chapter 6 - meat microbiology and spoilage,” in Lawrie’s meat science (Duxford, United Kingdom: Woodhead Publishing), 187–203.
Zhao, W., Wang, Y., Liu, S., Huang, J., Zhai, Z., He, C., et al. (2015). The dynamic distribution of porcine microbiota across different ages and gastrointestinal tract segments. PLOS ONE 10 (2), e0117441. doi:10.1371/journal.pone.0117441
Zweifel, C., and Stephan, R. (2014). “Chapter 16 - microbial contamination during slaughter,” in Meat inspection and control in the slaughterhouse (Hoboken, United States: John Wiley & Sons), 423–438.
Zwirzitz, B., Wetzels, S. U., Dixon, E. D., Stessl, B., Zaiser, A., Rabanser, I., et al. (2020). The sources and transmission routes of microbial populations throughout a meat processing facility. npj Biofilms Microbiomes 6 (1), 26. doi:10.1038/s41522-020-0136-z
Keywords: sanitary status, swine value chain, food safety, 16S rDNA, microbiota, microbial ecology, bacteria, meat
Citation: Laforge P, Vincent AT, Duchaine C, Feutry P, Dion-Fortier A, Plante P-L, Pouliot É, Fournaise S and Saucier L (2023) Contribution of farms to the microbiota in the swine value chain. Front. Syst. Biol. 3:1183868. doi: 10.3389/fsysb.2023.1183868
Received: 10 March 2023; Accepted: 15 June 2023;
Published: 12 July 2023.
Edited by:
Helena U. Zacharias, Hannover Medical School, GermanyReviewed by:
Bouabid Badaoui, Mohammed V University, MoroccoChrysovalantou Chatziioannou, International Agency For Research On Cancer (IARC), France
Hassan Zafar, Central European Institute of Technology (CEITEC), Czechia
Wen Chyin Yew, Northumbria University, United Kingdom
Copyright © 2023 Laforge, Vincent, Duchaine, Feutry, Dion-Fortier, Plante, Pouliot, Fournaise and Saucier. This is an open-access article distributed under the terms of the Creative Commons Attribution License (CC BY). The use, distribution or reproduction in other forums is permitted, provided the original author(s) and the copyright owner(s) are credited and that the original publication in this journal is cited, in accordance with accepted academic practice. No use, distribution or reproduction is permitted which does not comply with these terms.
*Correspondence: Linda Saucier, bGluZGEuc2F1Y2llckBmc2FhLnVsYXZhbC5jYQ==