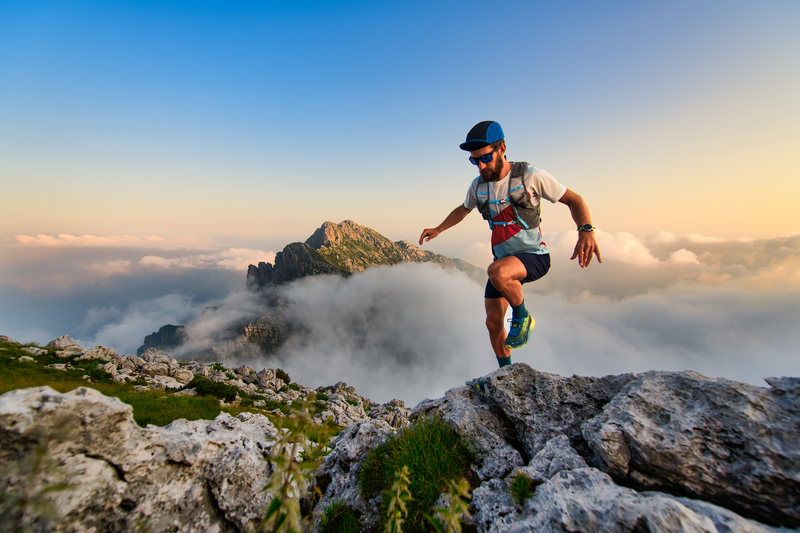
94% of researchers rate our articles as excellent or good
Learn more about the work of our research integrity team to safeguard the quality of each article we publish.
Find out more
ORIGINAL RESEARCH article
Front. Syst. Biol. , 27 February 2023
Sec. Data and Model Integration
Volume 3 - 2023 | https://doi.org/10.3389/fsysb.2023.1136999
Introduction: As people living with HIV age, the increasing burden of multimorbidity poses a significant health challenge. The aims of this study were to identify common patterns of multimorbidity and examine changes in their burden, as well as their associations with risk factors, over a 3–5 year period in people with HIV, enrolled in the Pharmacokinetic and clinical Observations in PeoPle over fiftY (POPPY) study.
Methods: Common multimorbidity patterns were identified in POPPY participants with HIV using principal component analysis, based on Somers’ D statistic. Multimorbidity burden scores were calculated for each participant/pattern at study entry/follow-up and were standardised relative to the mean in the sample at baseline (scores >0 thus reflect a greater number of comorbidities relative to the mean). Two multivariable linear regression models were fitted to examine the associations between risk factors and burden z-scores at baseline and change in z-scores over a 3–5 year period.
Results: Five patterns were identified among the 1073 POPPY participants with HIV {median age [interquartile range (IQR)], 52 (47–59) years; 85% male and 84% white}: Cardiovascular diseases (CVDs), Sexually transmitted diseases (STDs), Neurometabolic, Cancer and Mental-gastro-joint. The multivariable linear regression showed that older age, behavioural factors (i.e., body mass index (BMI), history of injection drug use, current recreational drug use and sex between men), and HIV-specific factors (i.e., duration since HIV diagnosis and a prior AIDS diagnosis) were associated with higher multimorbidity burden at baseline. However, only three of the factors (age, BMI and duration since HIV diagnosis) were significantly associated with an increase in burden across specific patterns over time.
Discussion: Key modifiable and non-modifiable factors contributing to an increase in burden of multimorbidity were identified. Our findings may inform the development of more targeted interventions and guidelines to effectively prevent and manage the rising burden of multimorbidity in people with HIV.
The widespread use of antiretroviral therapy (ART) has dramatically reduced the risk of AIDS-related morbidity and mortality and improved the overall survival of people with HIV (May et al., 2014). As a result, those living with HIV are experiencing a similar life expectancy to that of HIV-negative individuals. Currently, around one in three individuals accessing HIV care in the UK are aged ≥ 50 years (Kirwan et al., 2016), a proportion that is projected to increase to 54% by 2028 (Yin et al., 2015). As people with HIV are growing older, multimorbidity, defined as the co-existence of two or more health conditions (Boyd and Fortin, 2010), is becoming increasingly prevalent. Several studies have reported that people with HIV have a greater risk of developing comorbidities traditionally associated with aging, including cardiovascular, metabolic, bone and neurodegenerative disorders, compared with their HIV-negative counterparts (Guaraldi et al., 2011; Althoff et al., 2015). Multimorbidity presents a significant health challenge with far-reaching implications for people with HIV, particularly as current guidelines are often tailored to address the management of individual comorbidities (EACS, 2022; Waters et al., 2022) and rarely address the complex needs of individuals with multiple morbidities and their associated impacts, which include polypharmacy, drug-drug interactions and adverse therapeutic effects. Consequently, people with HIV may be at a higher risk of adverse health outcomes associated with multimorbidity, such as poorer quality of life and higher healthcare costs/utilisation (Gebo, 2008; Nachega et al., 2012; Rodriguez-Penney et al., 2013; Van Duin et al., 2017).
Certain health conditions are more likely to co-occur among people with HIV due to shared causes and risk factors, with likely contributions from HIV-mediated inflammatory processes (e.g., chronic inflammation and systemic immune activation) and long-term exposure to ART (Deeks and Phillips, 2009; Althoff et al., 2015; Guaraldi et al., 2017; Wong et al., 2018). Behavioural and/or environmental factors may also play an important role. For example, a higher prevalence of smoking is reported in people with HIV compared to the general population, a behaviour that is recognised as a risk factor for multiple conditions including lung cancer and obstructive lung disorders (Rossouw et al., 2015). Additionally, chronic inflammation has been linked to the development of both cardiovascular disease and neurocognitive decline (Deeks et al., 2013; Babu et al., 2019).
Over the last decade, the clustering of comorbidities has been increasingly explored using data-driven approaches which, compared to other measures (e.g., simple counts and weighted indices), can identify non-random co-occurrence of conditions (De Francesco et al., 2020) and improve our understanding of common clusters (patterns), associations and interactions between comorbidities. This, in turn, will allow us to identify patterns that pose the greatest burden where targeted prevention and treatment interventions may be most appropriate. To our knowledge, only four studies have investigated multimorbidity patterns in people with HIV using a data-driven approach. A recent review highlighted that certain patterns among HIV populations were also generally reported in the general population, such as those including CVDs, metabolic disorders and mental health problems (De Francesco et al., 2020). In addition, novel patterns, including STDs and conditions related to substance use (alcohol, recreational drugs, tobacco) or their complications, were also identified. We previously identified six common patterns among people with HIV receiving clinical care in the UK and Ireland (enrolled in the Pharmacokinetic and clinical Observations in PeoPle over fiftY (POPPY) study between 2013 and 2016) (De Francesco et al., 2018). However, the list of comorbidities (65 in total) was selected using a prevalence threshold of ≥ 1.5% in the study population. Use of this threshold may have failed to accurately capture key morbidities in this population, such as rarer and/or complex conditions that pose a greater multimorbidity burden. Furthermore, how the burden of specific patterns evolves over time remains unclear. Here we aim to: 1) re-identify multimorbidity patterns in POPPY participants with HIV, using an updated wider list of comorbidities; 2) examine changes in the burden of multimorbidity patterns over a 3–5-year follow up period; and 3) investigate the relative contributions of socio-demographic, lifestyle and clinical factors to these changes in each pattern over time.
The POPPY study is a prospective cohort study that includes three groups: “older” (aged ≥50 years) and “younger” (18–49 years) groups of people with HIV (the latter being frequency-matched to the older group on gender, ethnicity, sexual orientation, and participating clinic), and a group of HIV-negative controls aged ≥ 50 years. The present analyses were restricted to participants with HIV as a control group was not directly relevant to our aims here. Participants with HIV (n = 1,073) were recruited from 8 outpatient clinics (7 in England and 1 in Ireland) between April 2013 and January 2016 (Bagkeris et al., 2018). Briefly, participants eligible for inclusion were selected using the following criteria to reflect the UK HIV population in clinical care: 1) documented presence of HIV infection; 2) white or black-African ethnicity; 3) likely route of HIV acquisition via sexual exposure; and 4) the ability to comprehend the study information leaflet. All study participants provided written informed consent. The study was approved by the UK National Research Ethics Service (NRES; Fulham, London, United Kingdom, number 12/LO/1409).
At baseline (April 2013–January 2016) and follow-up (May 2015–February 2018), information on the presence/absence of comorbidities was collected using self-reported medical history supplemented using information on concomitant medication (non-antiretroviral) and healthcare utilisation data (including visits to general practitioners, hospitals, psychiatrists/psychologists, specialists, and use of ambulance/hospital transport). Participants were asked to report whether they had ever developed any of 52 health conditions (from 17 pathophysiological systems) from a detailed list and to add any other conditions experienced (using free-text) that were not included in the list. Conditions that were reported by < 1.0% of participants were excluded, giving a total of 73 comorbidities for the present analysis (Supplementary Table S1).
A range of covariates (demographic, lifestyle and clinical) recorded at baseline were included based on previous literature on the determinants of multimorbidity identified among cohorts of people with HIV: Age (per 10-year increment), sex (male vs. female), race (black African vs. white ethnicity), sexual orientation (men who have sex with men (MSM) or heterosexual), body mass index (BMI, per 1-kg/m2 increment), current smoking (current smoker, ex- and non-smoker) and alcohol consumption (current, ex- and no-use), current recreational drug use (yes or no within the 6 months preceding study visit) and history of injection drug use (IDU, yes or no). The following HIV-specific factors, which were obtained via linkage with the UK CHIC study [16] and the Mater Misericordiae University Hospital Infectious Diseases cohort for participants recruited in Ireland, were also included: nadir CD4+ T-cell count (per 100 cells/μL increment), years since HIV diagnosis (per 5-year increment) and any prior AIDS-related event (yes or no). Further information on the statistical properties of the included variables can be found in our previous publication (De Francesco et al., 2018).
Baseline demographic, clinical and lifestyle characteristics of included participants were described. Categorical data are presented as frequencies and proportions (%) and continuous data as median and interquartile range (IQR).
As previously described (De Francesco et al., 2018), common multimorbidity patterns were identified among all POPPY participants with HIV (n = 1,073) using principal component analysis (PCA) (Jolliffe, 2014). Briefly, Somers’ D statistic was used to assess pairwise associations between the 73 comorbidities at baseline, as current evidence suggests that this approach is more accurate in detecting non-random associations between comorbidities compared to other agreement measures (Ng, 2015; De Francesco et al., 2018). PCA was then applied to the matrix with the pairwise associations to reduce the original set of variables to a smaller set of principal components (PCs), whilst retaining as much of the variability in the dataset as possible. These PCs can be interpreted as patterns of multimorbidity, i.e., non-random groups of comorbidities. In order to allow multiple patterns to be present within one individual, an oblimin rotation was used in the PCA. To be consistent with our previous publication, a correlation ≥ 0.40 was used to determine comorbidities that were significantly associated with a pattern and were subsequently used to determine the label of that pattern.
The multimorbidity patterns were assessed over time by calculating burden scores for each participant and each pattern at baseline and follow-up. The analysis excluded those who were lost to follow-up (n = 238) or who had missing clinical data (BMI (n = 7); nadir CD4+ T-cell count (n = 36); years since HIV diagnosis (n = 4)). Descriptive characteristics were compared between those who were included (n = 788) and those excluded (n = 285) from analyses (Supplementary Table S2) with no large differences in the characteristics of the two groups.
Multimorbidity burden scores were determined using data on the comorbidities (presence/absence) and the positive coefficients (loadings) returned by the PCA (Supplementary Figures S1–S4) on baseline data. The scores were standardised relative to the mean in the sample at baseline, with negative z-scores denoting scores that were lower than the average from the baseline population. Scores are proportional to the number of comorbidities included within a pattern, with higher scores representing a greater number of comorbidities in an individual. All comorbidities (except STDs) were assumed to be chronic in nature and therefore were assessed cumulatively, i.e., any comorbidities reported by individuals at baseline were carried forward to follow-up. Changes in the burden of the ‘STD’ pattern over follow-up was not considered since this includes comorbidities of acute nature and were not of clinical interest/significance for the present analyses. Negative PCA loadings were excluded as they were relatively small in magnitude and were judged by authors to lack clinical relevance when compared with the comorbidities that were significantly associated with the pattern (i.e., ≥0.4 loading); this ensured that follow-up scores could not decrease due to the new onset of a condition with a negative weighting. We did not re-run the PCA at follow-up as our aim was to investigate changes in the burden scores over time and thus needed comparability of the scores at the two timepoints.
Two multivariable linear regression models were fitted to examine the associations between the previously described risk factors and burden z-scores at baseline and change in z-scores over the 3–5 year period. An additional analysis was also conducted in a subset of the included participants who had available data on CD4/CD8 ratio (n = 770), to explore its association with baseline and changes in burden z-scores. All associations are reported as coefficients with associated 95% confidence intervals (CI). All analyses were conducted using R (version 4.2.4). A significance level of p < .05 guided statistical interpretation.
The socio-demographic and HIV-related characteristics of the 788 participants with complete data are summarised in Table 1. Participants were predominantly male (85.9%), of white ethnicity (85.7%), MSM (77.4%) with a median (IQR) age of 53 (47, 59) years. The majority of participants (91.4%) had an undetectable viral load (<50 copies/mL). The median (IQR) nadir CD4+ T-cell count and years since HIV diagnosis were 205 (108, 310) cells/μL and 13.2 (7.7, 20.4), respectively. Current smoking was reported by 23.9% of participants, current recreational drug use by 27.2% and a history of IDU by 9.5%.
TABLE 1. Demographic, lifestyle and HIV-related characteristics of POPPY participants with HIV (n = 788).
Among the 788 participants, the prevalence of comorbidities ranged from 1% (pancreatitis) to 42% (gonorrhoea) (Supplementary Table S3). Other prevalent comorbidites included dyslipidemia (31%), clinical depression (30%) and syphilis (30%). In addition, 97.7% of all participants reported ≥ 1 comorbidity (median [IQR] per individual: 6 [3–8]) at baseline. At follow-up, 98.9% reported ≥1 comorbidity with a median [IQR] of 7 [4–10] comorbidities per individual (Figure 1). Most comorbidities demonstrated a similar small increase in prevalence, with the exception of the following conditions: joint/back pain (increased by 16%), chest infections (9%), osteopenia/osteoporosis (6.6%) and eye problems (6%) which had increased over the 3–5 year period (Supplementary Table S3).
FIGURE 1. The number (%) of comorbidities reported by POPPY participants with HIV at baseline (n = 1,073), baseline (returned for follow-up, n = 788) and follow-up (n = 788), with the median (IQR) number of comorbidities per individual also reported.
Five common patterns were identified, accounting for 21.1% of the total variance in the 73 comorbidities. The comorbidities with a correlation (loading) ≥ 0.4 with each distinguished pattern are reported in Table 2. Three patterns were previously identified (De Francesco et al., 2018): ‘CVDs’ (hypertension, coronary artery bypass(CABG)/percutaneous transluminal coronary angioplasty (PCTA)), ‘STDs’ (gonorrhoea, chlamydia, syphilis, Lymphogranuloma venereum and herpes simplex virus) and ‘Cancer’ (haematological cancer and solid organ cancer). In addition, two novel patterns were identified: ‘Neurometabolic’ (pancreatic insufficiency, peripheral neuropathy, Pneumocystis pneumonia, pruritis, type II diabetes and hypothyroidism) and ‘Mental-gastro-joint’ pattern (clinical depression, joint inflammation/arthritis, pancreatitis, persistent bowel disorders and joint replacement).
TABLE 2. Patterns identified using principal component analysis in all POPPY participants with HIV (n = 1,073).
The distribution of burden z-scores for the patterns at baseline and follow-up are shown in Figure 2. The highest median (IQR) z-score was reported in the Mental-gastro-joint pattern at both baseline (−0.21 [−0.83,0.58]) and follow-up (0.04 [−0.68,1.01]), with the largest median (IQR) change also observed for this pattern (0.09 [0.00,0.35]). There was considerable variation in the median distribution of both the CVD (baseline: −0.28 [−0.81,0.49]; follow-up: −0.06 [−0.72,0.77]) and Neurometabolic (baseline: −0.28 [−0.78,0.52]; follow-up: −0.07 [−0.63,0.77]) z-scores at baseline and follow-up. In contrast, the majority of participants reported extremely low z-scores in the Cancer pattern at both time points, with a very small change in this z-score: 0.00 [0.00–0.15].
FIGURE 2. Distribution of multimorbidity burden z-scores at baseline (red) and follow-up (blue) among POPPY participants with HIV (n = 788), with median change [interquartile range; IQR] also reported.
A longer duration since HIV diagnosis (range: 0.09–0.17, all p < 0.01) and a prior AIDS diagnosis (range: 0.19–0.83, all p < 0.01) were significantly associated with higher z-scores (1 standard deviation (SD) higher score) across all patterns at baseline (Table 3). Older age (0.37 [0.30, 0.44]), being male (0.35 [0.07, 0.63]) and a higher BMI (0.03 [0.01, 0.05] per 1-kg/m2 increment) were independently associated with higher CVD z-scores. In contrast, participants of white ethnicity had a lower z-score compared to those of Black-African ethnicity (−0.33 [−0.62, −0.05]). Similarly, older age (0.16 [0.09, 0.22]), sex between men (0.29 [0.05, 0.54], a higher BMI (0.02 [0.01, 0.03] per 1-kg/m2 increment) and current recreational drug use (0.14 [0.00, 0.28]) were associated with higher Neurometabolic z-scores. Older age (0.16 [0.08, 0.23]) was also associated with higher Cancer z-scores. Men had lower Mental-gastro-joint z-scores compared to women at baseline (−0.44 [−0.73, −0.15]). On the other hand, sex between men (0.32 [0.04, 0.59]), a higher BMI (0.02 [0.01, 0.04] per 1-kg/m2 increment), current smoking (0.25 [0.07, 0.42]), and a history of IDU (0.46 [0.23, 0.68]) were associated with higher Mental-gastro-joint z-scores. Furthermore, in the sub-analysis (n = 770), CD4:CD8 ratio was not significantly associated with any of the patterns at baseline (Supplementary Table S4).
TABLE 3. The relative contributions of demographic, lifestyle and clinical factors to the burden of multimorbidity patterns at baseline (n = 788) assessed using multivariable linear regression models, with regression coefficients (95% confidence intervals) and associated p-value reported.
A longer time since HIV diagnosis was significantly associated with an increase in z-scores (1 SD) across all patterns (range: 0.02–0.03; all p < 0.01) (Table 4). None of the other risk factors were significantly associated with an increase in Cancer or Mental-gastro-joint z-scores. In contrast, older age per 10-year increment (0.04 [0.01, 0.06], p < 0.001) and a higher BMI (0.01 [0.01, 0.02] per 1-kg/m2, p = 0.02) were independently associated with an increase in CVD z-scores over time. Similarly, older age (0.03 [0.01, 0.05], p = 0.01) and a higher BMI (0.01 [0.00, 0.01] per 1-kg/m2, p = 0.01) were significantly associated with an increase in Neurometabolic z-scores. Furthermore, in the sub-analysis, CD4:CD8 ratio was not found to be associated with an increase in scores across any of the patterns over time (Supplementary Table S5).
TABLE 4. The relative contributions of demographic, lifestyle and clinical factors to an increase in burden of multimorbidity patterns (n = 788) assessed using multivariable linear regression models, with regression coefficients (95% confidence intervals) and associated p-value reported.
This study identified five common multimorbidity patterns in a cohort of people living with HIV receiving clinical care in the United Kingdom and Ireland and found that their burden has increased over a 3–5 year period. We also characterised the relative contributions of HIV- and non-HIV-related risk factors, and report key modifiable (e.g., BMI and recreational/injection drug use) and non-modifiable (e.g., age and time since HIV diagnosis) factors associated with the rise in multimorbidity burden.
Existing studies on multimorbidity patterns among people with HIV are scarce and vary considerably in terms of their methodological approach. In particular, a small number of comorbidities (typically 14–24) are considered, which may not reflect the wider spectrum of health conditions typically reported in people with HIV (Morales et al., 2022). We re-identified patterns using a wide spectrum of comorbidities reflecting the complexity of multimorbidity in this population. In doing so, two novel patterns were identified (Neurometabolic and Mental-gastro-joint), along with three patterns (CVDs, STDs and Cancer) that were previously identified among this cohort (De Francesco et al., 2018), and are consistent with those reported by other studies in people with HIV (Goulet et al., 2005; Yang et al., 2021). However, it is difficult to compare our results with those of previous studies due to differences in statistical methods, data sources, study populations and the comorbidities included. In both general populations and people with HIV, pancreatic insufficiency, hypothyroidism, pruritis and peripheral neuropathy have been linked to type 2 diabetes (Evans et al., 2012; Piciucchi et al., 2015; Ogbonna and Ezeani, 2019; Pillay et al., 2020; Stefaniak et al., 2021; Kuka et al., 2022). However, the potential shared underlying biological pathways associated with these comorbidities merits further investigation. For example, exploring whether the comorbidities in the Neurometabolic pattern are more likely to co-occur due to contributions from HIV-mediated inflammatory processes such as persistent inflammation. The biological link between mental health and joint disorders has been supported by other clinical studies that suggest a bidirectional relationship in which local and systemic inflammation play an important role (Lu et al., 2019; Nikiphorou et al., 2019; Lwin et al., 2020). HIV-associated systemic inflammation may also explain the co-occurrence of gastrointestinal disorders within this pattern (Brenchley et al., 2008).
In the present study, we found that the contributions of HIV- and non-HIV-related risk factors to the burden at baseline and over time varied across patterns. With the demographic/lifestyle factors, older age and a higher BMI were associated with higher CVDs, Neurometabolic and Cancer burden at baseline. However, these factors were only associated with an increase in CVDs and Neurometabolic burden over time. With the behavioural factors, history of IDU and current recreational drug use were associated with higher Mental-gastro-joint and Neurometabolic burden at baseline, respectively, which is consistent with previous studies linking IDU/recreational drug use to the development of mood disorders and metabolic complications(Henry, 2000; Williams et al., 2017). However, none of the behavioural factors were identified as predictors for an increase in burden of any of the patterns. One possible explanation for this may be that a longer follow-up period may be required to reveal their impact over time. Alternatively, this could reflect reverse causation as the majority of comorbidities preceded the assessment of the behavioural factors, and thus people may already have made changes to their lifestyles after a previous diagnosis of a comorbidity. In terms of the HIV-related factors, a prior AIDS diagnosis and a longer duration since HIV diagnosis were associated with higher scores across all the patterns at baseline. However, only the latter was identified as a predictor for an increase in burden of these patterns over time. Nevertheless, these findings suggest that biological effects of HIV infection, including sustained immune activation and chronic inflammation, may play a central role in the burden of multimorbidity among people with HIV.
To our knowledge, this is the first study to assess the burden of multimorbidity patterns, and the relative contributions of risk factors, over time. However, there are some limitations to our study that need to be considered. First, there is no uniform list of comorbidities to define multimorbidity among people with HIV, so the list considered here may be debated. However, we used a large list to ensure we could capture the diverse/wide spectrum of comorbidities typically reported in this population. Second, data on comorbidities were collected using self-reported medical history, and were thus subject to recall bias. However, we ensured that, where possible, information was collected/validated using healthcare utilisation and concomitant medication data. Third, multimorbidity burden over time was examined using patterns identified at baseline. We acknowledge that the loadings of comorbidities may have changed after a follow-up period of 3–5 years, but examining scores based on baseline patterns allowed us to compare both time points. Fourth, the exploration of alternative methodologies such as Actionable Explainable Artificial intelligence (AxAI) (a machine learning approach)(Saranti et al., 2022), as well as a longer follow-up period, may have been advantageous to assess the change in multimorbidity burden and to elucidate the causal link with risk factors. The POPPY study has two additional study visits planned for data collection, which will allow us to reexamine the burden of multimorbidity over a longer follow-up period. Finally, the POPPY cohort is predominantly men of white ethnicity (representative of the UK HIV population seen in clinical care); therefore, our findings are less generalizable to cohorts in different HIV settings, i.e., populations that are predominately women and/or of non-white ethnicity.
This study represents one of the first efforts to describe multimorbidity burden over time among people with HIV. Our work highlights an increasing need for the development of targeted interventions, particularly focusing on modifiable risk factors, and guidelines to address the complexities and management of multimorbidity in the context of HIV. Our findings also highlight the need for further work to elucidate the role of HIV-mediated immunological pathways (using biomarker data) to the burden of multimorbidity. Future planned work will also explore the impact of multimorbidity burden on health/treatment outcomes over time.
The original contributions presented in the study are included in the article/Supplementary Material, further inquiries can be directed to the corresponding author.
The studies involving human participants were reviewed and approved by The UK National Research Ethics Service (NRES; Fulham London, United Kingdom number 12/LO/1409). The patients/participants provided their written informed consent to participate in this study.
LS, DD, and CS designed the study. LS performed the analyses, with support from DD, and drafted the manuscript. CS supervised the project. All authors critically revised, edited and approved the final draft of the manuscript. All authors had final responsibility for the decision to submit for publication. The corresponding author confirms that all listed authors meet authorship criteria.
The POPPY study waves 1–3 was funded by investigator-initiated grants from BMS, Gilead Sciences, Janssen, Merck and ViiV Healthcare (EudraCT Number: 2012- 003581-40; Sponsor Protocol Number: CRO1992). The POPPY study waves 4 and 5 is funded by investigator-initiated grants from Gilead Sciences, ViiV Healthcare and Merck Sharp & Dohme (UK) Limited (Sponsor protocol number: 19SM5112). We acknowledge the use of the National Institute for Health Research (NIHR)/Wellcome Trust Clinical Research Facility at King’s College Hospital. The study is also supported by the National Institute for Health Research (NIHR) Biomedical Research Centre based at Imperial College Healthcare NHS Trust and Imperial College London and by an NIHR Senior Investigator Award to CS. LS was funded through the National Institute for Health and Care Research Health Protection Research Unit (NIHR HPRU) in Blood Borne and Sexually Transmitted Infections at University College London in partnership with the UK Health Security Agency (HPRU Grant no: NIHR200911). The funders were not involved in the study design, collection, analysis, interpretation of data, the writing of this article or the decision to submit it for publication.
POPPY Management Team: MB, PM, FP, CS, MS, AW, Christina Prechtl. POPPY Scientific Steering Committee: JA, David Asboe, MB, Lucy Garvey, PM, FP, Anton Pozniak, CS, MS, JV, IW, and AW. POPPY Sites and Trials Unit: Caldecot Centre, King’s College Hospital (FP, Lucy Campbell, Selin Yurdakul, Sara Okumu, Louise Pollard, Beatriz Santana Suárez, Luella Hanbury) Department of Infection and Population Health, UCL (IW, Damilola Otiko, Laura Phillips, Rosanna Laverick, Michelle Beynon, Anna-Lena Salz, Abigail Severn, Michelle Beynon, Gosala Gopalakrishnan) Clinical Research Facility Allan at St Mary’s, Brighton and Sussex University Hospital (Martin Fisher, Amanda Clarke, JV, Andrew Bexley, Celia Richardson, Sarah Kirk, Rebecca Gleig, Leigh Greenland) HIV Molecular Research Group, School of Medicine, UCD (PM, Alan Macken, Bijan Ghavani-Kia, Joanne Maher, Maria Byrne, Ailbhe Flaherty, Aoife McDermott, Riya Negi, Alejandro Garcia-Leon and Aoife Cotter) Homerton Sexual Health Services, Homerton University Hospital (JA, Sifiso Mguni, Rebecca Clark, Rhiannon Nevin-Dolan, Sambasivarao Pelluri, Tracey Fong) Ian Charleson Day Centre, Royal Free Hospital (MJ, Nnenna Ngwu, Nargis Hemat, Anne Carroll, Sabine Kinloch, Mike Youle, Sara Madge and Katie Spears) Imperial Clinical Trials Unit, Imperial College London (Daphne Babalis, Jodi Meyerowitz, Christina Prechtl) St. Mary’s Hospital London, Imperial College Healthcare NHS Trust (AW, Lucy Garvey, Merle Henderson, Claire Peterson, Wilbert Ayap, Allan Lisenco and Ian McGuinness) St Stephen's Centre, Chelsea and Westminster Hospital (MB, David Asboe, Anton Pozniak, Margherita Bracchi, Nicole Pagani, Maddalena Cerrone, Daniel Bradshaw, Francesca Ferretti, Chris Higgs, Elisha Seah, Stephen Fletcher, Michelle Anthonipillai, Ashley Moyes, Katie Deats, Irtiza Syed, Clive Matthews, Peter Fernando, Cherry Colcol). POPPY methodology/statistics: CS, Nicholas Bakewell, Hajra Okhai, Luxsena Sukumaran. NIHR HPRU Steering Committee: Professor CA Sabin (HPRU Director), Dr J Saunders (UKHSA Lead), Professor C Mercer, Dr H Mohammed, Professor G Rait, Dr R Simmons, Professor W Rosenberg, Dr T Mbisa, Professor R Raine, Dr S Mandal, Dr R Yu, Dr S Ijaz, Dr F Lorencatto, Dr R Hunter, Dr K Foster and Dr M Tahir.
CS has received funding from Gilead Sciences, ViiV Healthcare, MSD and Janssen-Cilag for membership of Advisory Boards and for preparation of educational materials. AW has been an investigator on studies sponsored by, received research grants from and received speaker fees or honoraria from ViiV Healthcare, Janssen, Gilead Sciences and MSD. FP reports grants and personal fees from Gilead Sciences, ViiV Healthcare and MS; all outside of the work reported here. PM has received honoraria and/or travel grants from Gilead Sciences, MSD, Bristol-Myers Squibb, and ViiV Healthcare, and has been awarded grants by Science Foundation Ireland, outside the submitted work. JA reports personal fees from Gilead Sciences and ViiV; all outside of the work reported here. MB has acted as a speaker or adviser to, has been an investigator for, or has received grants to her institution from Gilead, ViiV, Janssen, BS, Teva, Cipla, Mylan, and MSD; all outside the work presented here. JV reports travel, research grants, and personal fees from Merck, Janssen Cilag, Piramal Imaging, ViiV Healthcare, and Gilead; all outside the work presented here. The funders were not involved in the study design, collection, analysis, interpretation of data, the writing of this article or the decision to submit it for publication.
The remaining authors declare that the research was conducted in the absence of any commercial or financial relationships that could be construed as a potential conflict of interest.
All claims expressed in this article are solely those of the authors and do not necessarily represent those of their affiliated organizations, or those of the publisher, the editors and the reviewers. Any product that may be evaluated in this article, or claim that may be made by its manufacturer, is not guaranteed or endorsed by the publisher.
The views expressed are those of the author(s) and not necessarily those of the NHS, the NIHR, the Department of Health or the funders.
The Supplementary Material for this article can be found online at: https://www.frontiersin.org/articles/10.3389/fsysb.2023.1136999/full#supplementary-material
Althoff, K. N., McGinnis, K. A., Wyatt, C. M., Freiberg, M. S., Gilbert, C., Oursler, K. K., et al. (2015). Comparison of risk and age at diagnosis of myocardial infarction, end-stage renal disease, and non-AIDS-defining cancer in HIV-infected versus uninfected adults. Clin. Infect. Dis. 60 (4), 627–638. doi:10.1093/cid/ciu869
Babu, H., Ambikan, A. T., Gabriel, E. E., Svensson Akusjärvi, S., Palaniappan, A. N., Sundaraj, V., et al. (2019). Systemic inflammation and the increased risk of inflamm-aging and age-associated diseases in people living with HIV on long term suppressive antiretroviral therapy. Front. Immunol. 10, 1965. doi:10.3389/fimmu.2019.01965
Bagkeris, E., Burgess, L., Mallon, P. W., Post, F. A., Boffito, M., Sachikonye, M., et al. (2018). Cohort profile: The Pharmacokinetic and clinical Observations in PeoPle over fiftY (POPPY) study. Int. J. Epidemiol. 47 (5), 1391–1392e. doi:10.1093/ije/dyy072
Boyd, C. M., and Fortin, M. (2010). Future of multimorbidity research: How should understanding of multimorbidity inform health system design? Public Health Rev. 32 (2), 451–474. doi:10.1007/BF03391611
Brenchley, J. M., Douek, D. C., Arminio Monforte, A., Diaz-Cuervo, H., De Luca, A., Maggiolo, F., et al. (2008). Evolution of major non-HIV-related comorbidities in HIV-infected patients in the Italian cohort of individuals, naïve for antiretrovirals (ICONA) foundation study cohort in the period 2004-2014. Mucosal ImmunolHIV Med. 120 (12), 2399–30109. doi:10.1111/hiv.12683
De Francesco, D., Sabin, C. A., and Reiss, P. (2020). Multimorbidity patterns in people with HIV. Curr. Opin. HIV AIDS 15 (2), 110–117. doi:10.1097/coh.0000000000000595
De Francesco, D., Verboeket, S. O., Underwood, J., Bagkeris, E., Wit, F. W., Mallon, P. W. G., et al. (2018). Patterns of co-occurring comorbidities in people living with HIV. Open Forum Infect. Dis. 5 (11), ofy272. doi:10.1093/ofid/ofy272
Deeks, S. G., and Phillips, A. N. (2009). HIV infection, antiretroviral treatment, ageing, and non-AIDS related morbidity. Bmj 338, a3172. doi:10.1136/bmj.a3172
Deeks, S. G., Tracy, R., and Douek, D. C. (2013). Systemic effects of inflammation on health during chronic HIV infection. Immunity 39 (4), 633–645. doi:10.1016/j.immuni.2013.10.001
Evans, S. R., Lee, A. J., Ellis, R. J., Chen, H., Wu, K., Bosch, R. J., et al. (2012). HIV peripheral neuropathy progression: Protection with glucose-lowering drugs? J. Neurovirol 18 (5), 428–433. doi:10.1007/s13365-012-0119-9
Gebo, K. A. (2008). Epidemiology of HIV and response to antiretroviral therapy in the middle aged and elderly. Aging health 4 (6), 615–627. doi:10.2217/1745509x.4.6.615
Goulet, J. L., Fultz, S. L., McGinnis, K. A., and Justice, A. C. (2005). Relative prevalence of comorbidities and treatment contraindications in HIV-mono-infected and HIV/HCV-co-infected veterans. AIDS 19 (Suppl. 3), S99–S105. doi:10.1097/01.AIDS.0000192077.11067.E5
Guaraldi, G., Orlando, G., Zona, S., Menozzi, M., Carli, F., Garlassi, E., et al. (2011). Premature age-related comorbidities among HIV-infected persons compared with the general population. Clin. Infect. Dis. 53 (11), 1120–1126. doi:10.1093/cid/cir627
Guaraldi, G., Zona, S., Menozzi, M., Brothers, T. D., Carli, F., Stentarelli, C., et al. (2017). Late presentation increases risk and costs of non-infectious comorbidities in people with HIV: An Italian cost impact study. AIDS Res. Ther. 14 (1), 8. doi:10.1186/s12981-016-0129-4
Henry, J. A. (2000). Metabolic consequences of drug misuse. BJA Br. J. Anaesth. 85 (1), 136–142. doi:10.1093/bja/85.1.136
Jolliffe, I. (2014). Wiley StatsRef: Statistics reference online. John Wiley & Sons.Principal component analysis
Kirwan, P., Chau, C., Brown, A., Gill, O., and Delpech, V. (2016). HIV in the UK – 2016 report. London: Public Health England.
Kuka, W. P., Shah, J., Alam, U., Shah, R., and Sokhi, D. S. (2022). Clinical characteristics of peripheral neuropathy in Kenyan patients with HIV infection compared with patients with concurrent HIV infection and diabetes mellitus. Diabetes Ther. 13 (3), 441–451. doi:10.1007/s13300-022-01205-3
Lu, H., Surkan, P. J., Irwin, M. R., Treisman, G. J., Breen, E. C., Sacktor, N., et al. (2019). Inflammation and risk of depression in HIV: Prospective findings from the multicenter AIDS cohort study. Am. J. Epidemiol. 188 (11), 1994–2003. doi:10.1093/aje/kwz190
Lwin, M. N., Serhal, L., Holroyd, C., and Edwards, C. J. (2020). Rheumatoid arthritis: The impact of mental health on disease: A narrative review. Rheumatol. Ther. 7 (3), 457–471. doi:10.1007/s40744-020-00217-4
May, M. T., Gompels, M., Delpech, V., Porter, K., Orkin, C., Kegg, S., et al. (2014). Impact on life expectancy of HIV-1 positive individuals of CD4+ cell count and viral load response to antiretroviral therapy. Aids 28 (8), 1193–1202. doi:10.1097/qad.0000000000000243
Morales, D. R., Moreno-Martos, D., Matin, N., and McGettigan, P. (2022). Health conditions in adults with HIV compared with the general population: A population-based cross-sectional analysis. eClinicalMedicine 47, 101392. doi:10.1016/j.eclinm.2022.101392
Nachega, J. B., Hsu, A. J., Uthman, O. A., Spinewine, A., and Pham, P. A. (2012). Antiretroviral therapy adherence and drug-drug interactions in the aging HIV population. Aids 26 (1), S39–S53. doi:10.1097/QAD.0b013e32835584ea
Ng, S. K. (2015). A two-way clustering framework to identify disparities in multimorbidity patterns of mental and physical health conditions among Australians. Statistics Med. 34 (26), 3444–3460. doi:10.1002/sim.6542
Nikiphorou, E., Lempp, H., and Kohrt, B. A. (2019). Treatment failure in inflammatory arthritis: Time to think about syndemics? Rheumatol. Oxf. 58 (9), 1526–1533. doi:10.1093/rheumatology/kez222
Ogbonna, S. U., and Ezeani, I. U. (2019). Risk factors of thyroid dysfunction in patients with type 2 diabetes mellitus. Front. Endocrinol. (Lausanne) 10, 440. doi:10.3389/fendo.2019.00440
Piciucchi, M., Capurso, G., Archibugi, L., Delle Fave, M. M., Capasso, M., and Delle Fave, G. (2015). Exocrine pancreatic insufficiency in diabetic patients: Prevalence, mechanisms, and treatment. Int. J. Endocrinol. 2015, 595649. doi:10.1155/2015/595649
Pillay, S., Pillay, D., Singh, D., and Pillay, R. (2020). Human immunodeficiency virus, diabetes mellitus and thyroid abnormalities: Should we be screening? South Afr. J. HIV Med. 21 (1), 1116. doi:10.4102/sajhivmed.v21i1.1116
Rodriguez-Penney, A. T., Iudicello, J. E., Riggs, P. K., Doyle, K., Ellis, R. J., Letendre, S. L., et al. (2013). Co-Morbidities in persons infected with HIV: Increased burden with older age and negative effects on health-related quality of life. AIDS Patient Care STDS 27 (1), 5–16. doi:10.1089/apc.2012.0329
Rossouw, T. M., Anderson, R., and Feldman, C. (2015). Impact of HIV infection and smoking on lung immunity and related disorders. Eur. Respir. J. 46 (6), 1781–1795. doi:10.1183/13993003.00353-2015
Saranti, A., Hudec, M., Mináriková, E., Takáč, Z., Großschedl, U., Koch, C., et al. (2022). Actionable explainable ai (AxAI): A practical example with aggregation functions for adaptive classification and textual explanations for interpretable machine learning. Mach. Learn. Knowl. Extr. [Online] 4 (4), 924–953. doi:10.3390/make4040047
Stefaniak, A. A., Krajewski, P. K., Bednarska-Chabowska, D., Bolanowski, M., Mazur, G., and Szepietowski, J. C. (2021). Itch in adult population with type 2 diabetes mellitus: Clinical profile, pathogenesis and disease-related burden in a cross-sectional study. Biol. (Basel) 10 (12), 1332. doi:10.3390/biology10121332
Van Duin, M. J., Conde, R., Wijnen, B., Evers, S. M., Gonzalez-Rodriguez, J. L., Govers, M. J., et al. (2017). The impact of comorbidities on costs, utilities and health-related quality of life among HIV patients in a clinical setting in Bogotá. Expert Rev. Pharmacoecon Outcomes Res. 17 (3), 303–310. doi:10.1080/14737167.2017.1246185
Waters, L., Winston, A., and Reeves, I. (2022). BHIVA guidelines on antiretroviral treatment for adults living with HIV-1 2022.
Williams, S. C., Davey-Rothwell, M. A., Tobin, K. E., and Latkin, C. (2017). People who inject drugs and have mood disorders-A brief assessment of health risk behaviors. Subst. Use Misuse 52 (9), 1181–1190. doi:10.1080/10826084.2017.1302954
Wong, C., Gange, S. J., Moore, R. D., Justice, A. C., Buchacz, K., Abraham, A. G., et al. (2018). Multimorbidity among persons living with human immunodeficiency virus in the United States. Clin. Infect. Dis. 66 (8), 1230–1238. doi:10.1093/cid/cix998
Yang, X., Zhang, J., Chen, S., Weissman, S., Olatosi, B., and Li, X. (2021). Comorbidity patterns among people living with HIV: A hierarchical clustering approach through integrated electronic health records data in South Carolina. AIDS Care 33 (5), 594–606. doi:10.1080/09540121.2020.1844864
Keywords: HIV, human immunodeficiency virus, multimorbidity (MM), comorbidity [MeSH], multimorbidity patterns, longitudinal, principal component analysis, PCA
Citation: Sukumaran L, De Francesco D, Winston A, Mallon PWG, Doyle N, Anderson J, Boffito M, Williams I, Post FA, Vera J, Sachikonye M, Johnson MA and Sabin CA (2023) Changes in multimorbidity burden over a 3–5 year period among people with HIV. Front. Syst. Biol. 3:1136999. doi: 10.3389/fsysb.2023.1136999
Received: 03 January 2023; Accepted: 13 February 2023;
Published: 27 February 2023.
Edited by:
Ljiljana T. Majnaric, University of Osijek, CroatiaReviewed by:
Anna Saranti, University of Natural Resources and Life Sciences Vienna, AustriaCopyright © 2023 Sukumaran, De Francesco, Winston, Mallon, Doyle, Anderson, Boffito, Williams, Post, Vera, Sachikonye, Johnson and Sabin. This is an open-access article distributed under the terms of the Creative Commons Attribution License (CC BY). The use, distribution or reproduction in other forums is permitted, provided the original author(s) and the copyright owner(s) are credited and that the original publication in this journal is cited, in accordance with accepted academic practice. No use, distribution or reproduction is permitted which does not comply with these terms.
*Correspondence: Luxsena Sukumaran, bHV4c2VuYS5zdWt1bWFyYW4uMTlAdWNsLmFjLnVr
Disclaimer: All claims expressed in this article are solely those of the authors and do not necessarily represent those of their affiliated organizations, or those of the publisher, the editors and the reviewers. Any product that may be evaluated in this article or claim that may be made by its manufacturer is not guaranteed or endorsed by the publisher.
Research integrity at Frontiers
Learn more about the work of our research integrity team to safeguard the quality of each article we publish.