- 1Core Unit Data Integration Center, University Medicine Greifswald, Greifswald, Germany
- 2Department of Systems Biology and Bioinformatics, University of Rostock, Rostock, Germany
- 3Department of Safety and Radiation Protection, Forschungszentrum Jülich, Jülich, Germany
- 4Medical Informatics Laboratory, Institute for Community Medicine, University Medicine Greifswald, Greifswald, Germany
- 5Leibniz-Institute for Food Systems Biology at the Technical University of Munich, Munich, Germany
MicroRNAs (miRNAs) and long non-coding RNAs (lncRNAs) are involved in the modulation of the DNA-damage response (DDR) and upon exposure to ionizing radiation (IR), their expression fluctuates. In this study, we propose a workflow that enables the creation of regulatory networks by integrating transcriptomics data as well as regulatory data in order to better understand the interplay between genes, transcription factors (TFs), miRNAs, and lncRNAs in the cellular response to IR. We preprocessed and analyzed publicly available gene expression profiles and then applied our consensus and integration approach using open source data and tools. To exemplify the benefits of our proposed workflow, we identified a total of 32 differentially expressed transcripts corresponding to 20 unique differentially expressed genes (DEGs) and using these DEGs, we constructed a regulatory network consisting of 106 interactions and 100 nodes (11 DEGs, 78 miRNAs, 1 DEG acting as a TF, and 10 lncRNAs). Overrepresentation analyses (ORAs) furthermore linked our DEGs and miRNAs to annotations pertaining to the DDR and to IR. Our results show that MDM2 and E2F7 function as network hubs, and E2F7, miR-25-3p, let-7a-5p, and miR-497-5p are the four nodes with the highest betweenness centrality. In brief, our workflow, that is based on open source data and tools, and that generates a regulatory network, provides novel insights into the regulatory mechanisms involving miRNAs and lncRNAs in the cellular response to IR.
1 Introduction
Our genome is continuously exposed to exogenous and endogenous agents that cause DNA damage. Consequently, several mechanisms have evolved to detect and counteract DNA damage, collectively termed the DNA-damage response (DDR) (Jackson and Bartek, 2009). Ionizing radiation (IR) induces various types of DNA damage e.g. single-strand breaks (SSBs), double-strand breaks (DSBs), oxidized bases and abasic sites (Ward, 1994) with the DSB considered the most critical DNA lesion for the cell. IR induces DNA damage through direct ionization and through radiation-induced reactive oxygen species (ROS), which damage the DNA indirectly (Ward, 1988). DSBs are mainly repaired by two mechanisms: non-homologous end joining (NHEJ) (Lieber, 2008) or homologous recombination (HR) (San Filippo et al., 2008). The failure to repair DSBs or an incorrect repair can lead to mutations, chromosomal rearrangements, genomic instability, driving oncogenesis or lead to cell death (Pampfer and Streffer, 1989; Morgan et al., 1996; Ceccaldi et al., 2016; Murashko et al., 2021).
In recent years, non-coding RNAs (ncRNAs) have emerged as a crucial component of the DDR. Different RNA species, including but not limited to, microRNAs (miRNAs) and long non-coding RNAs (lncRNAs) have been shown to modulate the DDR, for example, in repairing DSBs (Thapar, 2018; Ketley and Gullerova, 2020). Mature miRNAs are single-stranded endogenous RNAs approximately 22 nucleotides in length that posttranscriptionally regulate messenger RNAs (mRNAs) (O’Brien et al., 2018). On the other hand, lncRNAs are considered to be longer than 200 nucleotides and are often involved in transcriptional regulation or occasionally function as a competing endogenous RNA (ceRNA), an RNA transcript that sequesters miRNAs and therefore inhibits their effects (St.Laurent et al., 2015; Ma et al., 2019). The importance of miRNAs and lncRNAs in the DDR is well established (Zhang and Peng, 2015; Liu et al., 2016; Thapar, 2018; Shaw and Gullerova, 2021) and several studies have already investigated changes in gene/mRNA, miRNA, and lncRNA expression after IR exposure (Lacombe et al., 2018; May et al., 2021; Jia and Wang, 2022). However, their interplay following IR exposure has yet to be elucidated.
Many tools have already been developed to integrate different types of omics data such as transcriptomics, proteomics, metabolomics, and methylomics (Graw et al., 2021). Furthermore, the use of network-based approaches to better understand regulatory mechanisms behind complex diseases has repeatedly been employed (Boroń et al., 2022; Noble et al., 2022; Vahabi and Michailidis, 2022). Nonetheless, bioinformatics approaches in combination with omics data have seldom been used in the context of ionizing radiation to create regulatory networks. Using a mouse model of radiation-induced lung injury, Li et al. (2019) identified a lncRNA-, microRNA- and mRNA-associated ceRNA network. The analysis of lncRNAs and mRNAs of mouse peripheral blood mononuclear cells (PBMCs) exposed to low-dose IR also revealed lncRNA-mRNA coexpression networks (Qi et al., 2020). Therefore, regulatory networks incorporating mRNA-, miRNA-, and lncRNA-derived data are needed to understand the interplay following IR exposure.
In this study, we developed a generic workflow, that is applied to a specific used case. Particularly, our workflow is based on the analysis of transcriptomics data, where peripheral blood is subjected to IR, and our workflow then incorporates regulatory information pertaining to TFs, miRNAs, and lncRNAs to create a regulatory network. Due to the fact that the generation of omics data using cells subjected to IR is very expensive and time-consuming, our methodology focuses on using open source data and tools. Namely, we obtained publicly available raw microarray datasets along with their corresponding platform specifications from the Gene Expression Omnibus (GEO) database (Edgar et al., 2002). We then preprocessed and analyzed the transcriptomics data and applied a consensus approach to uncover differentially expressed mRNAs, which we refer to as DEGs throughout this manuscript. In order to construct the regulatory network, we then incorporated TF, miRNA, and lncRNA regulatory data that we retrieved from publicly available databases. The construction, visualization and analysis of the network were performed using open source tools. We hereby uncovered 20 radio-responsive DEGs that have the potential to be used as IR biomarkers in terms of exposure, absorbed dose or dose rate. We also suggested that MDM2, E2F7, miR-26b-5p, and GADD45A may play an important role in the DDR since these nodes had the highest degree. The degree of a node corresponds to the total number of incoming and outgoing edges associated to this node. Nodes with high degrees are often referred to as hubs and are more likely to play an essential role (He and Zhang, 2006). Similarly, we revealed that E2F7, miR-25-3p, let-7a-5p, and miR-497-5p are the nodes with the highest betweenness centrality. Nodes with the highest betweenness centrality most frequently control information flows in the network (Abbasi et al., 2012). Moreover, overrepresentation analyses (ORAs) further supported the involvement of our uncovered molecules in the DDR in response to IR. In brief, we suggest that the identified regulatory network containing DEGs, TFs, miRNAs, and lncRNAs reveals insightful regulation mechanisms behind the cellular response to IR.
2 Materials and methods
The workflow developed in this study is depicted in Figure 1.
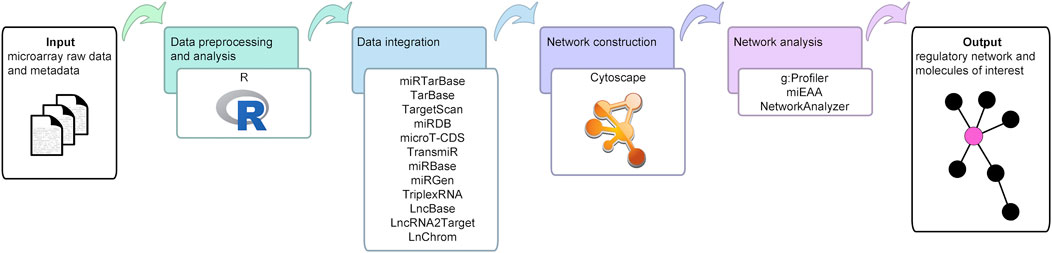
FIGURE 1. Bioinformatics workflow of the current study. This figure illustrates the open source data, tools, and databases employed to uncover the molecules and interactions of interest, and to create and analyze the final network.
2.1 Microarray data acquisition and preprocessing
We downloaded publicly available raw microarray datasets along with their corresponding platform specifications from the Gene Expression Omnibus (GEO) database, www.ncbi.nlm.nih.gov/geo/, see Table 1. In order to be included in this study, the original investigations needed to satisfy all of our following inclusion criteria. Namely, they needed to 1) make use of peripheral blood from healthy human volunteers; 2) include non-irradiated control samples; 3) use ex vivo radiation dose that can be found in the range of 0–8 Gy and that include doses greater than 1 Gy; and 4) perform the microarray data acquisition 24 h post-irradiation. We retained the following datasets: GSE8917, GSE65292, GSE90909, and GSE102971 (Paul and Amundson, 2008; Ghandhi et al., 2015; Broustas et al., 2017; Park et al., 2017). These studies are based on the Agilent platform (Whole Human Genome Microarrays with One-Color (Cyanine-3) labelled cRNA), thus making the studies especially comparable. Within GSE8917, we kept the samples where the microarray data acquisition was performed 24 h post-irradiation. Within GSE65292, we kept the samples that were irradiated with an acute radiation dose-rate and removed those that were irradiated with a low dose-rate since the acute dose-rate resembled the dose-rates used in the other studies. Within GSE90909, we kept the samples that were irradiated via X-rays and removed those that were irradiated using neutrons since the other studies used X-rays or γ-rays. Within GSE102971, we retained the samples where human peripheral blood was used. All subsequent preprocessing steps as well as the gene expression analysis, the permutation test, the target analyses, and the functional enrichment analyses were performed using R, version 4.1.1 (R Core Team, 2013).

TABLE 1. Microarray gene expression profiles used for the differential gene expression analysis. GEO dataset: Gene Expression Omnibus dataset (series) are represented by a series accession number beginning with the letters GSE; Platform: a platform beginning with the letters GPL, provides the physical setup of an assay such as an array and is linked to a GEO platform accession number; Number of samples: total number of control and irradiated samples; Radiation doses (Gy): the samples where irradiated with doses given in Gray (Gy), doses irradiated with 0Gy correspond to control samples.
The remaining samples within a dataset were preprocessed in the following manner. Within a sample, the control features of the raw data were removed. The background-corrected expression values of features sharing the same probe name were averaged. The Boolean values indicating if a feature’s expression value is a non-uniformity outlier were then averaged for features sharing the same probe name. Features for which the non-uniform outlier value was greater than 0.5 were assigned an expression value equivalent to “NA”. Likewise, features for which the average positive and significant value was smaller than 0.5 were assigned an expression value equivalent to “NA”. For the remaining samples within a dataset, a feature was completely removed from further analyses if it was associated with at least one expression value equivalent to “NA”. Additionally, the remaining data within a dataset was afterwards log2-transformed and quantile normalized using the function normalizeBetweenArrays in limma (Ritchie et al., 2015). These rigid filtering criteria were chosen to ensure a high quality of the preprocessed data. For each dataset, boxplots as well as density curves of the expression values, before and after quantile normalization, were created in order to eliminate outliers (data not shown). Upon visual inspection, the samples GSM2417372, GSM2417385, and GSM2417432 pertaining to GSE90909 were removed.
2.2 Differential gene expression analysis and consensus approach
To detect significant radiation-induced transcript expression changes, we applied a one-way analysis of variance (ANOVA). We adjusted for multiple testing using the Bonferroni method (Bonferroni, 1936). Transcripts with an adjusted p < 0.05 were considered statistically significant. We also did not perform Tukey’s honestly significance difference test since we were not interested in identifying pairwise differences in transcript expression between radiation doses. The probe names of the transcripts were then converted to HUGO Gene Nomenclature Committee (HGNC) and RefSeq symbols using biomaRt, version 2.50.3 (Durinck et al., 2005). All Venn diagrams were plotted with the ggvenn R package (version 0.1.9; https://CRAN.R-project.org/package=ggvenn). DEGs, genes that were statistically significantly differentially expressed (Bonferroni-adjusted p < 0.05), in at least three of the four datasets were selected for further analysis.
2.3 Permutation test of consensus approach
To show that our selected transcripts are not a result of a random selection, we performed a permutation test. For each dataset, we randomly selected a number of transcripts, selected from the complete list of transcripts available after preprocessing without replacements, corresponding to the number of statistically significantly differentially expressed (Bonferroni-adjusted p < 0.05) per dataset. We then determined the number of transcripts that were common in at least three of the four datasets. We repeated this process 100,000 times and subsequently calculated a p-value.
2.4 Database integration and network construction
Since we are interested in regulatory interactions between DEGs, TFs, miRNAs, and lncRNAs, we made use of the following databases to construct our regulatory network. Figure 2 illustrates the different possible types of regulatory interactions that we searched for, and ultimately, the regulatory network configuration. Five databases were utilized to find miRNA-gene interactions. The interaction pairs pertaining to each database were downloaded in bulk from their respective website. MiRTarBase, release 8.0 (Hsu et al., 2011), and TarBase, v8 (Huang et al., 2020), were used to obtain validated miRNA-gene interactions. We only kept the interactions that were validated in both databases. TargetScan, release 8.0 (Lewis et al., 2005), miRDB, v6.0 (Chen and Wang, 2020), and microT-CDS, v4 (Reczko et al., 2012), were on the other hand used to uncover predicted miRNA-gene interactions. In TargetScan, interactions with a context score smaller than −0.48 were retained. In miRDB, interactions with a score greater to 94 were likewise withheld. Similarly in microT-CDS, interactions with a miTG score greater than 0.905 were kept. We only made use of interactions that were predicted in all three databases after setting the respective cutoffs and filtering restrictions in an attempt to filter out false positives. Since no regulation type was provided in these databases, we assumed that the miRNAs repress their target genes. We next uncovered TF-miRNA interactions using TransmiR, v2.0 (Tong et al., 2019), and miRGen, v3 (Georgakilas et al., 2016). Similarly, the interaction pairs pertaining to these databases were downloaded in bulk from their respective website. TransmiR contains validated TF-miRNA interactions, namely we made use of the interactions curated from the literature. We withheld interactions where the interaction type exactly matched “Activation” or “Repression” and searched for interactions where our uncovered DEGs acted as TFs. Primary miRNA names were converted to mature miRNAs names using the hsa.gff3 file provided by the miRBase, Release 22.1, website (https://www.mirbase.org/ftp.shtml) (Griffiths-Jones, 2004). In contrast, miRGen contains predicted TF-miRNA interactions and we kept predicted interactions with a p-value < 0.05. Similarly, the primary miRNA names were converted to mature miRNAs names using miRBase, Release 22.1. Since the regulation type in this database was not given, we assumed that the TFs activated their target miRNAs. We also made use of TriplexRNA, v2.0 (Schmitz et al., 2014) to expose predicted RNA triplexes composed of two miRNAs and their mutual target mRNA.
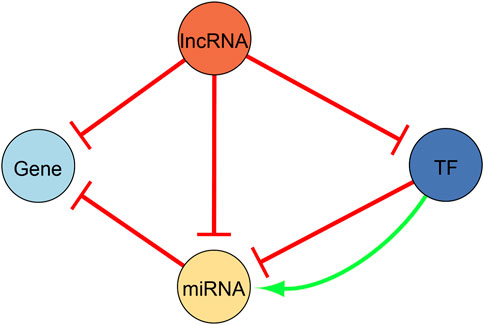
FIGURE 2. Illustration of the regulatory network configuration. We used this configuration to construct the regulatory network presented in this study. Yellow nodes represent microRNAs (miRNA), light blue nodes represent differentially expressed genes, dark blue nodes are differentially expressed genes that act as transcription factors (TFs), and orange nodes are long non-coding RNAs (lncRNA). All edges are directed. Repressing edges are red whereas activating edges are green.
We afterwards identified experimentally supported lncRNA-target interactions using the following databases. The interaction pairs pertaining to each database were downloaded in bulk from their respective website. lncRNA-miRNA interactions were found via LncBase, v2 (Paraskevopoulou et al., 2016), lncRNA-target (miRNAs or DEGs) interactions though LncRNA2Target v3.0 (Wang et al., 2019), and lncRNA-chromatin interactions (affecting DEG or miRNA expression, therefore lncRNA-target interactions) through LnChrom (Yu et al., 2018). We included the latter since chromatin remodeling is relevant to the DDR. We first filtered the LncBase database and removed interactions whose detection type matched “INDIRECT”. We then kept interactions whose category corresponded to “Normal/Primary” and we finally kept interactions validated in “Bone Marrow” tissue since this tissue in the database is the closest to peripheral blood. We also assumed that the lncRNAs inhibit their miRNA targets. For the LncRNA2Target database, we made use of the low throughput experiments to avoid obtaining too many results. We retained interactions associated to “DNA damage” and “normal” disease states, and we removed interactions where experiments were performed using murine tissue. In LnChrom, we used the interactions discovered via high- and low-throughput methods. Since the regulation type in these databases was not given, we assumed that the lncRNAs repressed their targets. For the construction of our regulatory network, we kept all interactions uncovered via LncRNA2Target and LnChrom. We also kept the interactions common to both LncBase and LncRNA2Target, and to LncBase and LnChrom, a conservative approach with the goal of maintaining a moderate regulation network size.
We finally made use of Cytoscape v.3.9.1 (Shannon et al., 2003) to visualize the resulting regulatory networks, which included miRNA-DEG, TF-miRNA, lncRNA-miRNA, and lncRNA-target interactions.
2.5 Differentially expressed genes and network analysis
We used the gprofiler2 R package, version 0.2.1 (Raudvere et al., 2019), in combination with R, version 4.1.1 (R Core Team, 2013) to perform an ORA first using the DEGs and then the network nodes. We limited the annotations to “Homo sapiens” and all data sources were selected except for the electronic GO annotations. The p-values where corrected via the false discovery rate (FDR) method (Benjamini and Hochberg, 1995) and annotations with an adjusted p-value < 0.05 were selected. The same query was run directly in g:GOSt on the g:Profiler website, https://biit.cs.ut.ee/gprofiler/gost version e105_eg52_p16_e84549f database updated on 03/01/2022, to retrieve the DEGs associated to the enriched annotations. We furthermore carried out an ORA on the miRNAs present in the networks using the miRNA Enrichment and Annotation tool (miEAA), v.2.0 (Backes et al., 2016). In the additional parameters, we selected all the available categories and kept the annotations with an FDR adjusted p-value < 0.05. The p-values were adjusted for each category independently and we set the minimum of required hits per subcategory to 5, a fairly stringent cutoff. The annotations were also limited to Homo sapiens. We utilized g:Profiler in combination with miEAA to uncover annotations pertaining to the DDR and thereby demonstrated that our DEGs and network nodes (DEGs, TFs, miRNAs, and lncRNAs) are relevant to the DDR. We specifically searched for overrepresented annotations directly containing either “damage”, “ionization”, or “radiation” and filtered out irrelevant annotations. Furthermore, we made use of NetworkAnalyzer, v.4.4.8, a Cytoscape plugin, to carry out a network analysis (analyzed as a directed graph) to determine topological parameters such as the degree of the nodes and the betweenness centrality (Assenov et al., 2008). Nodes with the highest degrees or with the highest betweenness centrality may thus play biologically significant roles in the regulation of the cellular response to irradiation.
3 Results
3.1 Identification of differentially expressed genes
Supplementary Table 1 shows the differentially expressed transcripts (Bonferroni-adjusted p < 0.05), that is, the probe names of the transcripts and their converted names to RefSeq and HGNC symbols using biomaRt. Namely, we uncovered 32 unique transcripts corresponding to 20 unique HGNC symbols which we refer to as DEGs. These transcripts were differentially expressed in at least three of the four GEO datasets, see Figure 3. Namely, we found 986, 45, 112, and 0 differentially expressed transcripts in GSE102971, GSE65292, GSE90909, GSE8917, respectively. A permutation test supports the notion that these 32 common differentially expressed transcripts are radio-responsive transcripts (p-value = 0). None of the 32 transcripts were differentially expressed in all four datasets. An ORA using g:Profiler revealed that 310 annotations with an FDR-corrected p-value < 0.05 were enriched. Of these, 17 annotations were directly related to DNA damage or radiation, see Supplementary Table 2. Nine genes were associated to these enriched annotations: ASCC3, DDB2, E2F7, GADD45A, LIG1, MDM2, PCNA, POLH, and RPS27L. Furthermore, these 9 genes were associated to the gene ontology term “cellular response to DNA damage stimulus”, term ID GO:0006974. These results imply that our uncovered DEGs are affected by IR.
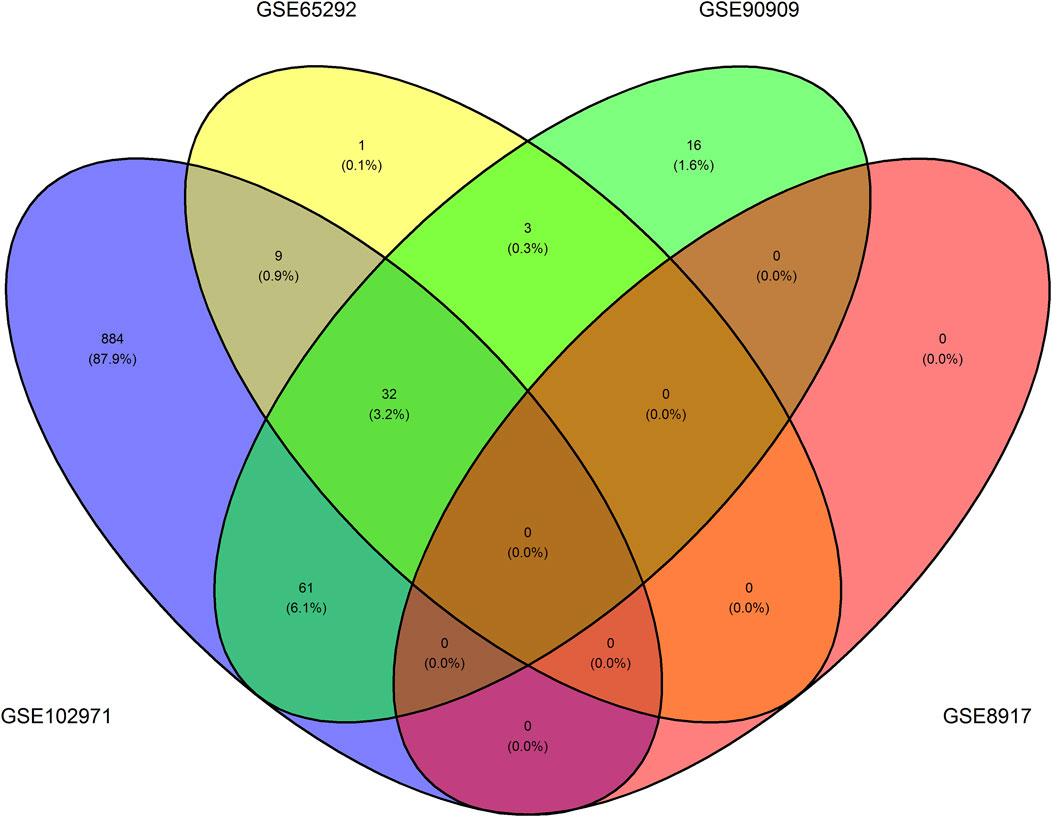
FIGURE 3. Venn diagram illustrating the number of overlapping differentially expressed transcripts per dataset. One can observe the number of overlapping differentially expressed transcripts that have a Bonferroni-adjusted p < 0.05 between the GSE102971, GSE65292, GSE8917, and GSE90909 datasets.
3.2 MiRNAs targeting the differentially expressed genes
We next identified validated and predicted miRNA-DEG interactions using the 20 previously determined DEGs. In total, we detected 89 validated miRNA-DEG interactions and three predicted miRNA-DEG interactions, see Figure 4. The validated and predicted interactions did not overlap thus bringing the total of uncovered miRNA-DEG interactions to 92. A complete list of these 92 miRNA-DEG interactions can be found in Supplementary Table 3. A total of 12 DEGs (ASCC3, CCL27, DDB2, E2F7, FDXR, FHL2, GADD45A, GLS2, LIG1, MDM2, PCNA, and PHPT1) were targeted by 77 miRNAs. MDM2 was targeted the most, that is, 44 miRNAs targeted this DEG, followed by E2F7, ASCC3, GADD45A, and PCNA, that were targeted 17, 7, 7, and 6 times, respectively. The most involved miRNAs were miR-26b-5p, miR-124-3p, miR-16-5p, miR-26a-5p, miR-27a-3p, miR-30a-5p, and miR-542-3p targeting a total 8, 3, 3, 2, 2, 2, and 2 DEGs, respectively. Furthermore, out of the 9 DEGs that were associated to DNA damage or radiation enriched annotations, see previous section, 7 were targeted by miRNAs: ASCC3, DDB2, E2F7, GADD45A, LIG1, MDM2, and PCNA. We did not uncover any RNA triplexes using TriplexRNA. Altogether, this demonstrates that our uncovered miRNAs may play a regulatory role in the cellular response to IR.
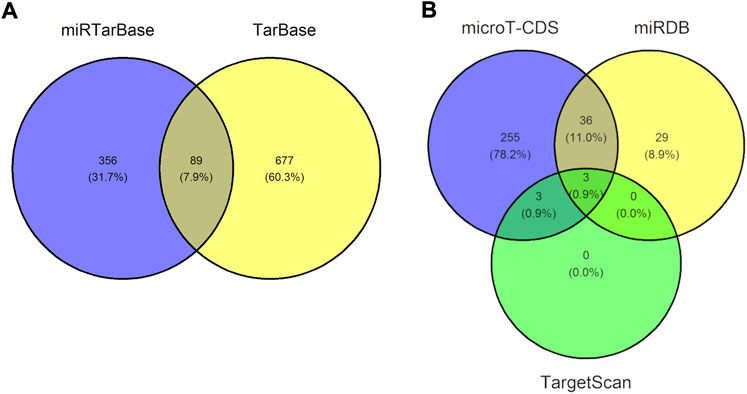
FIGURE 4. Venn diagram showing the number of uncovered microRNA-gene interactions. (A) Venn diagram illustrating the 89 overlapping micorRNA-gene interaction pairs uncovered using miRTarBase and TarBase, two databases containing validated microRNA-gene interactions. (B) Venn diagram illustrating the three overlapping microRNA-gene interaction pairs found using microT-CDS, miRDB, and TargetScan, three databases containing predicted microRNA-gene interactions. The validated and predicted interactions did not overlap, therefore we uncovered a total of 92 unique miRNA-gene interaction pairs.
3.3 Transcription factors targeting the miRNAs
We subsequently uncovered regulatory interactions containing TFs. Namely, TransmiR and miRGen were then used to identify TFs regulating miRNAs, thus DEGs acting as TFs. We did not identify any TF-miRNA interactions using miRGen, however using TransmiR, we did find that E2F7 targets miR-25-3p and miR-25-5p. These findings therefore imply that E2F7 may contribute to the complexity of the cellular response to IR.
3.4 lncRNAs targeting the miRNAs
In order to finalize the network, we afterward uncovered lncRNA-target interactions, where the targets could either be DEGs or miRNAs, which were exposed in the previous sections. We identified 779 lncRNA-miRNA interactions using LncBase, five lncRNA-target interactions using LncRNA2Target, and seven lncRNA-target interactions using LnChrom, see Figure 5. Notably, we did not uncover interactions in LnChrom when using high-throughput methods. Since our main focus was to demonstrate the establishment of a workflow that generates a regulation network using open source data and tools, we used a conservative approach to determine lncRNA-target interactions. With this in mind, we excluded 778 interactions identified via LncBase only, and we retained a total of 12 lncRNA-target interactions, see Figure 5; Table 2. Namely, 10 lncRNAs accounted for the 12 interactions and they targeted 9 different DEGs or miRNAs. DDB2 and let-7a-5p were each targeted by 2 different lncRNAs whereas the other targets were only targeted by a single lncRNA. These results support the implication of lncRNAs in the context of cellular damage brought about by irradiation.
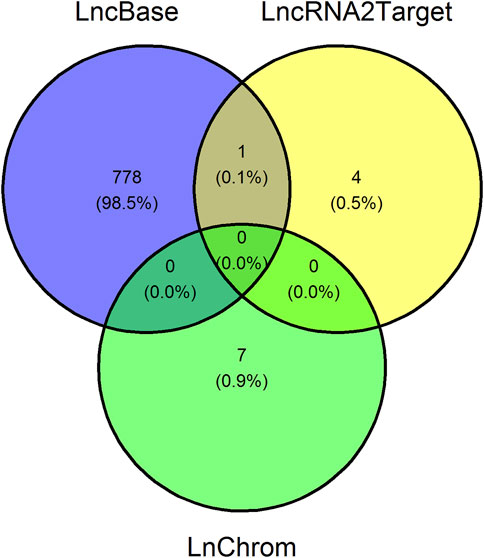
FIGURE 5. Venn diagram showing the number of uncovered long non-coding RNA-target interactions. Venn diagram illustrating the number of overlapping long non-coding RNA (lncRNA)-target interaction pairs between LncBase, LncRNA2Target, and LnChrom, three databases containing lncRNA-target interactions. The lncRNA targets are either genes or microRNAs. We retained 12 interaction pairs, namely those uncovered using LncRNA2Target and LnChrom.
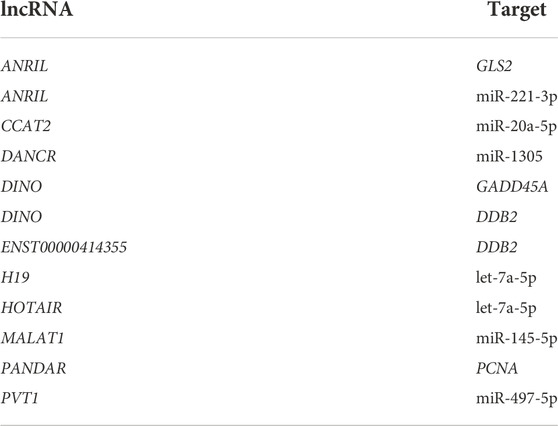
TABLE 2. Long non-coding RNAs and their targets. Long non-coding RNAs (lncRNA) and their targets, either genes or microRNAs, uncovered in our study are tabulated here.
3.5 Network construction and analysis
Utilizing the complete list of interactions, see Supplementary Table 4 that we discovered in the previous sections, we assembled the final regulatory network. Specifically, we employed Cytoscape to visualize the final regulation network, see Figure 6. This network consists of a total of 106 interactions and 100 nodes (11 DEGs, 78 miRNAs, 1 DEG acting as a TF, and 10 lncRNAs). Using NetworkAnalyzer, we carried out a network topology analysis to determine which nodes have the highest degree, and therefore function as hubs, or the highest betweenness centrality. The network topology table generated by NetworkAnalyzer is given in Supplementary Table 5. The list of selected nodes with the highest degree are tabulated in Table 3. MDM2, E2F7, miR-26b-5p, and GADD45A are of degree 44, 19, 8, and 8, respectively. These are the four nodes with the highest degree. On the other hand, E2F7, miR-25-3p, let-7a-5p, and miR-497-5p are the four nodes with the highest betweenness centrality with E2F7 having the highest, see Table 3. All node types (DEG, miRNA, TF, and lncRNA) are connected to the nodes that have the highest degree or betweenness centrality. This suggests that the regulation processes in response to IR are complex and involve different layers of regulation, for example, by miRNAs, TFs, and lncRNAs. Furthermore, E2F7 is among the nodes having the highest degree and the highest betweenness centrality. This gene may therefore play a particularly important part in the regulation of DNA damage.
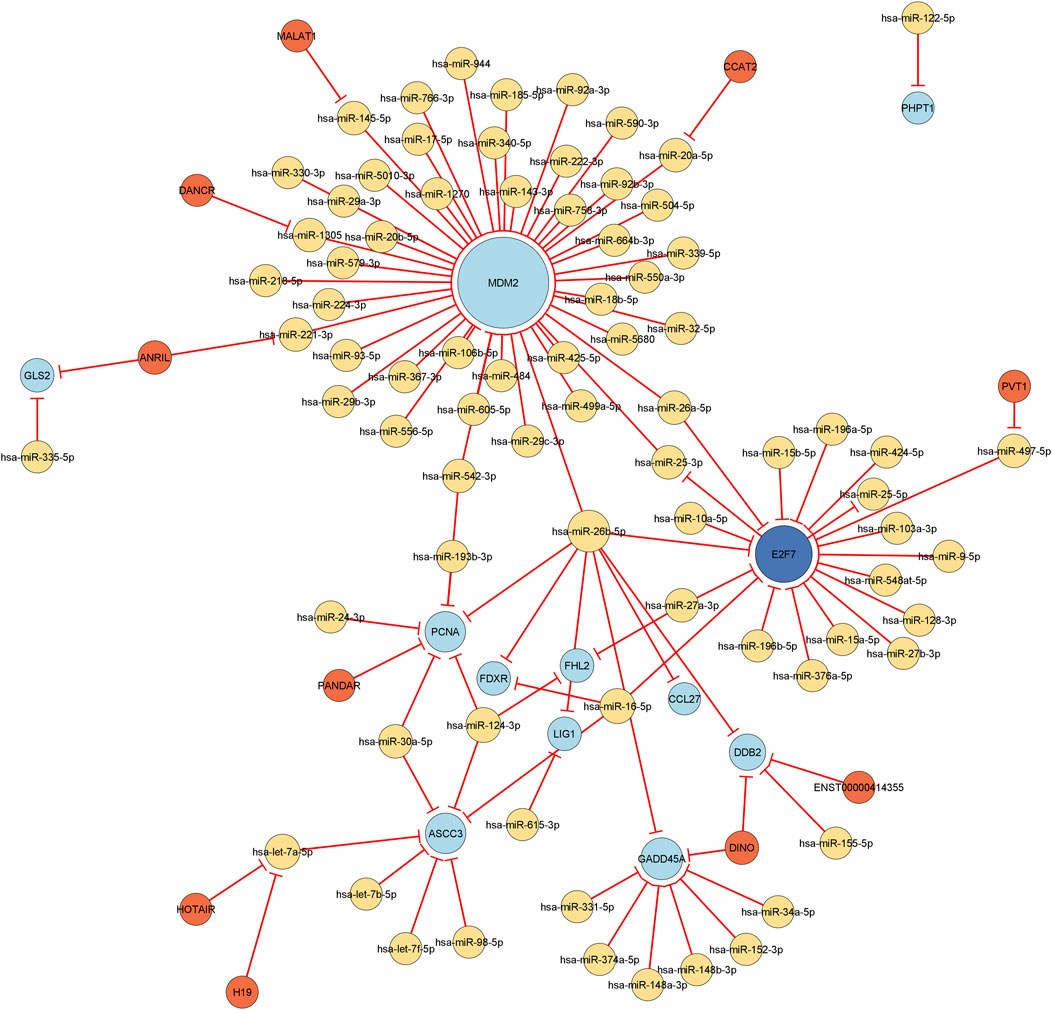
FIGURE 6. Visualization of the final regulatory network. All edges are repressing edges (red) and are directed. The yellow nodes represent microRNAs, the light blue nodes represent differentially expressed genes, the dark blue node corresponds to a differentially expressed gene that acts as transcription factor, and the orange nodes are long non-coding RNAs. The size of the node is proportional to its degree, i.e., the bigger the node, the more incoming and outgoing edges the node has.
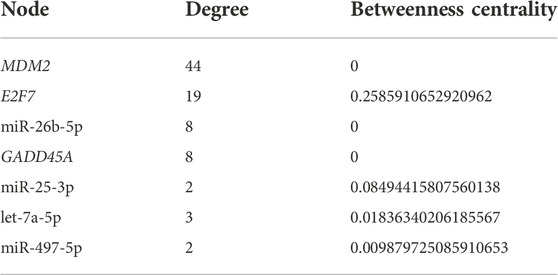
TABLE 3. Selected nodes with the highest degree or betweenness centrality. The name of the nodes (microRNAs or differentially expressed genes) is given in the first column. The second and third column respectively represent the degree of the node or its betweenness centrality measurement.
An ORA in g:Profiler using the network nodes also revealed that 271 annotations, with an FDR-corrected p-value < 0.05, were enriched. Moreover, 14 of these annotations were directly related to DNA damage or radiation, see Supplementary Table 6. Seven genes were connected to these enriched annotations: ASCC3, DDB2, E2F7, GADD45A, LIG1, MDM2, and PCNA. These genes were also mainly responsible for the enriched annotations directly related to DNA damage or radiation in section 3.1. Additionally, 12 of these 14 enriched annotation terms were already uncovered in section 3.1. Despite 76 nodes that could not be included in the g:Profiler query, the network was associated to enriched annotations directly related to DNA damage or radiation showing that our network is relevant in the context of radiation.
We also carried out an ORA on the network miRNAs using miEAA. 4852 annotations with an FDR-corrected p-value < 0.05, were enriched. The high number of enriched terms arises from the fact that we selected all parameters available (28 in total). Of these overrepresented annotations, 22 were directly related to DNA damage or radiation, see Supplementary Table 7. Specifically, 52 of our 78 network miRNAs were related to these 22 annotations. MiR-26a-5p, miR-185-5p, miR-34a-5p, miR-124-3p, miR-26b-5p, miR-615-3p, and miR-92a-3p were present in 10, 8, 8, 6, 6, 6, and 6 DNA damage- or radiation-related annotations, respectively. This demonstrates that our network miRNAs are indicative of DDR-regulation processes and that they may be important DDR modulators. We also examined if other types of our network nodes were comprised in the overrepresented miEAA annotations. Specifically, we inspected annotations pertaining to NPInter, a database of ncRNAs and biomolecule interactions (Teng et al., 2020). We examined if any of our network lncRNAs were comprised in these overrepresented miEAA annotations. Upon inspection, we observed that 2 of our 10 network lncRNAs, PVT1 and DANCR, were also found in NPInter associated annotations and functioned as ncRNAs regulating miRNAs. This additionally strengthens the concept that our network lncRNAs are involved in regulating DNA damage responses. Overall, the complex interplay between genes, TFs, miRNAs, and lncRNAs in response to exposure to IR may give rise to diverse regulatory mechanisms behind the DDR and should further be investigated.
4 Discussion
The interplay between genes, TFs, miRNAs, and lncRNAs in response to exposure to IR brings about specific regulatory mechanisms in the DDR that can be studied using openly available datasets and tools when following the proposed workflow. In this specific study, we demonstrated how to analyze microarray transcriptomics and integrate miRNA, TF, and lncRNA regulatory information such as to create and investigate a regulatory network regarding the radiation-induced DDR in response to IR.
Our workflow revealed that our differentially expressed transcripts originated from three of the four GEO datasets. We did not uncover any transcripts of interest in GSE8917. This may be because our preprocessing methods in combination with the Bonferroni p-value adjustment method were too stringent for this particular dataset. We opted for a consensus approach in conjunction with strict preprocessing and data analysis conditions to uncover relevant DEGs related to radiation and to overcome variations in the different datasets. Accordingly, a consensus approach that incorporates several expression profile studies is likely to improve the accuracy in identifying relevant transcripts as well as to reduce the probability of identifying false positives (Freiesleben et al., 2016). Furthermore, the integration of data from multiple cohorts is essential to generate robust and reproducible results (Beuchel et al., 2021). Here, we detected 20 radio-responsive genes and 17 of these 20 genes (i.e., APOBEC3H, ASCC3, DDB2, E2F7, FDXR, FHL2, GADD45, LIG1, MDM2, PCNA, PHPT1, POLH, RPS27L, SLC4A11, TNFSF4, VWCE, and ZNF79) have been previously identified as IR responsive genes (Kang et al., 2003; Dressman et al., 2007; Fachin et al., 2007; Gruel et al., 2008; Meadows et al., 2008; Paul and Amundson, 2008; Henríquez Hernández et al., 2009; Kabacik et al., 2011; Mayer et al., 2011; Paul and Amundson, 2011; Paul et al., 2011; Pogosova-Agadjanyan et al., 2011; Templin et al., 2011; Wen et al., 2011; Boldt et al., 2012; Knops et al., 2012; El-Saghire et al., 2013; Nosel et al., 2013; Paul et al., 2013; Versteyhe et al., 2013; Beer et al., 2014; Vinoth et al., 2014; Ghandhi et al., 2015; Macaeva et al., 2016; Rouchka et al., 2016; Broustas et al., 2017; Lacombe et al., 2018; Piotto et al., 2018; Ghandhi et al., 2019; Cruz-Garcia et al., 2020a; Cruz-Garcia et al., 2020b; Li et al., 2022). Two of the three remaining DEGs (i.e., CCL27 and GLS2) have also been described in the context of DNA damage or of oxidative stress, which is also related to radiation as radiation-induced reactive oxygen species (ROS). For example, the increased secretion of CCL27 in the human keratinocyte cell line HaCaT following X-ray irradiation, was triggered by the boosted generation of ROS, which was accompanied by the release of TNF-α (Zhang et al., 2017). Similarly, the expression of GLS2 was induced in response to DNA damage or oxidative stress in a p53-dependent manner, and elevated GLS2 lowered intracellular ROS levels, thus resulted in an overall decrease in DNA oxidation (Hu et al., 2010; Suzuki et al., 2010). Moreover, SLC4A11 has also been described in the context of oxidative stress (Roy et al., 2015). Upon exposure to oxidative stress, HEK 293 cells transfected with mutant SLC4A11 were more vulnerable to oxidative and mitochondrial damage compared to cells containing wild-type SLC4A11 (Roy et al., 2015). Ultimately, using publicly available expression profiles jointly with this consensus approach may be an alternative method to identify transcripts of interest when the production of experimental data is not possible or limited.
Moreover, we observed that MDM2 and E2F7 function as network hubs. Nodes with a high degree, also known as hubs, are more likely to play an essential role (He and Zhang, 2006). In line with this, multiple review articles have described the key role of MDM2 in the p53-MDM2-signaling axis as part of the DDR (Nag et al., 2013; Haronikova et al., 2019; Chen et al., 2020; Gnanasundram et al., 2021; Okazaki, 2022). In addition, we found that MDM2 is targeted by 44 different miRNAs. For example, we showed that MDM2 is targeted by miR-26b-5p, the miRNA with the highest degree. It was exposed that this miRNA directly targeted MDM2 (Benderska et al., 2015). Furthermore, we found that MDM2 is targeted by miR-25-3p, the node with the second highest betweenness centrality, and by miR-32-5p. In agreement with this, it was confirmed that the direct silencing of MDM2 by miR-25 and miR-32 led to an accumulation of p53, and the overexpression of these miRNAs in transfected glioblastoma multiforme cells hampered the growth of glioblastoma multifome cells in mouse brains (Suh et al., 2012). Our workflow also showed that MDM2 is targeted by miR-605-5p. In human breast cancer cells lines, Suh et al. (2012) reported that miR-605 interrupted the p53:MDM2 interaction by posttranscriptionally repressing MDM2 (Xiao et al., 2011). The authors further observed that these molecules create a positive feedback loop that facilitates the response to stress (Xiao et al., 2011). We furthermore uncovered that miR-143-3p and miR-145-5p, miRNAs belonging to the same cluster, repressed MDM2. Correspondingly, it was shown in head and neck squamous cell carcinomas that these miRNAs negatively modulated the expression of their target gene, MDM2 (Zhang et al., 2013). The authors additionally described that these miRNAs create a feedback loop with MDM2 and p53, and this feedback loop gives rise to the regulation of cellular apoptosis in response to DNA damage stress (Zhang et al., 2013). We also mentioned that E2F7 functions as network hub. It is well established that the E2F-family of transcriptional regulators are important controllers of the eukaryotic cell cycle (Emanuele et al., 2020). In addition to controlling the timely expression of genes necessary for G1/S transition and DNA replication, a study further found that E2F7 contributed to the regulation of DNA repair and genomic integrity by regulating genes that control DNA repair pathways (Mitxelena et al., 2018). We revealed that E2F7 is targeted by 17 miRNAs and that it acts as a TF by regulating two miRNAs. Namely, we uncovered that E2F7 regulates both miR-25-3p and miR-25-5p. It has been described in human U2OS osteosarcoma cells that E2F7 is required for the timely repression of a set of miRNAs including miR-25, which promote cell proliferation (Mitxelena et al., 2016). Moreover, we suggested that E2F7 is targeted by miR-424-5p, the node with the fourth highest betweenness centrality. This interaction has been confirmed in human hepatocellular carcinoma (HCC) cells and the targeting of E2F7 by miR-424-5p regulated the cell cycle and inhibited the proliferation of HCC cells (Zhao et al., 2020). Our results underline the substantial importance of studying the relationship between miRNAs and genes in the cellular response to IR in order to better understand regulatory mechanisms behind the DDR. We also exposed 59 miRNAs that were associated to DNA damage- or radiation-related overrepresented annotations. The most prominent miRNA, miR-26b-5p, was the miRNA with the highest degree and was associated to six overrepresented annotations relating to radiation and DNA damage. In breast cancer tissue samples of Chernobyl radiation-exposed female clean-up workers, it was demonstrated that the expression of miR-26b-5p was increased (Wilke et al., 2018). Han et al. (2020) reported that miR-26b-5p was the most downregulated miRNA in lung adenocarcinoma (LUAD) and that the miR-26b-5p overexpression in LUAD cells exposed to X-ray radiation led to enhanced radiosensitivity (Han et al., 2020). Furthermore, they showed that exosomal miR-26b-5p derived from A549 cells, and transferred to irradiation-resistant LUAD cells inhibited the expression of ATF2, a miR-26b-5p target, and promoted DNA damage, apoptosis, and radiosensitivity (Han et al., 2020). Although we did not identify the lncRNA DUXAP8 since we used restrictive lncRNA-target identification methods, the anti-apoptotic function of DUXAP8 in H1975 and A549 cell was partially reversed by miR-26b-5p, a target of this lncRNA (Liu et al., 2021). This study, like ours, shows that the interplay between miRNAs and lncRNAs is important when investigating regulatory responses. Therefore in order to create a more accurate and complete regulatory network involving miRNAs and lncRNAs, one should particularly pay attention to the filtering criteria used in publicly available databases when creating such a network.
Another miRNA of interest is let-7a-5p, the miRNA with the second highest degree and the node with the third highest betweenness centrality, and it was also associated to two DNA damage-related overrepresented annotations. Its expression level was highly upregulated in hematopoietic humanized NSG-SGM3 mice after radiation exposure (Tsogbadrakh et al., 2022). A miRNA profiling of human carcinoma cell lines HONE1 and CNE2 revealed that let-7a-5p, along with miR-26a-5p, let-7f-5p, and miR-20a-5p, other miRNAs also identified through our study, were differentially expressed after X-ray radiation (Luo et al., 2022). It was additionally revealed that miR-26a-5p contributed to X-ray radiation resistance (Luo et al., 2022). A recent study by Li et al. (2021) showed that the lncRNA OTDU6B-AS1 maintained the expression of MTDH by downregulating miR-26a-5p and thereby promoted autophagy and DNA damage (Li et al., 2021). Similarly, although we did not identify OTDU6B-AS1 and MTDH, our findings demonstrate that the complex interplay between lncRNAs and miRNAs may be behind certain aspects of the DDR.
We furthermore identified miR-124-3p as candidate miRNA involved in the cellular response to radiation as it was associated to six overrepresented annotations relating to DNA damage and radiation. Conducting experiments in CD2F1 mice using lethal radiation doses, Chakraborty et al. (2020) observed that the anti-inflammatory miR-124-3p was consistently inhibited across all phases post-total body irradiation (TBI) (Chakraborty et al., 2020). We likewise identified miR-27a-3p and miR-30a-5p, and these miRNAs may also be part of the cellular response to radiation. One study found that miR-27a-3p, however along miR-30a-3p and not miR-30a-5p as identified through our analysis, were among a serum miRNA signature group of five miRNAs able to distinguish between C57BL/6J mice exposed to sublethal and lethal radiation doses (Acharya et al., 2015). Another investigation exposed 25 significantly up- or downregulated miRNAs in the irradiated blood serum of macaques (Fendler et al., 2017). Of these 25, we also identified six: miR-122-5p, miR-16-5p, miR-30a-5p, miR-34a-5p, miR-424-5p, and miR-93-5p. It is evident that miRNAs play a role in regulating the response of cells exposed to irradiation. However, more studies are needed to understand what role miRNAs play in the context of a regulatory cellular network regarding the cellular response to radiation, for instance a radio-protective, anti-oxidant, anti-inflammatory, or pro-apoptotic role.
As previously mentioned, we purposely used restrictive methods to detect possible interaction pairs. More permissive methods, i.e., using the complete results generated by LncBase, resulted in the identification of 208 additional lncRNAs corresponding to 778 extra interactions (Figure 5). It is consequently very difficult to filter through these results in order to detect lncRNAs relevant to irradiation. Moreover, as stated by Yang et al. (2019), the information demonstrating the role of lncRNAs in the radiation-induced DDR is highly limited (Yang et al., 2019). Nonetheless, some of our identified lncRNAs (ANRIL, DINO, ENST00000414355, MALAT1, and HOTAIR) have been shown to participate in the DDR. For instance, following DNA damage, ANRIL was transcriptionally upregulated, and elevated levels of ANRIL suppressed the expression of NK4a, INK4b and ARF at the late-stage of DNA damage response, thus allowed cells to return to normal after DNA repair completion (Wan et al., 2013). Another study found that DINO was transcriptionally activated via p53 after DNA damage and regulated the p53-dependent DNA damage response (Schmitt et al., 2016). It was observed that the bone marrow plasma cells from patients with monoclonal gammopathy of undetermined significance or multiple myeloma expressed elevated MALAT1, which was involved in alternative non-homologous end joining (A-NHEJ) pathway (Hu et al., 2018). Furthermore, the knockdown of MALAT1 induced DNA damage and apoptosis in multiple myeloma (Hu et al., 2018). Additionally, it was demonstrated that ENST00000414355 negatively modulated the expression DDB2, a lncRNA-target pair also uncovered in our investigation (Zhou et al., 2015). The siRNA-mediated knockdown of ENST00000414355 furthermore inhibited the growth of DNA-damaged cells (Zhou et al., 2015). The interplay between another of our lncRNA-target pair, HOTAIR and miR-218-5p, was described elsewhere (Hu et al., 2019). Namely in response to IR, the knockdown of HOTAIR inhibited cell survival and increased cell apoptosis, and this radiosensitizing effect was attributed to the upregulation of miR-218 (Hu et al., 2019). Although the information concerning lncRNAs in response to IR is limited, we showed that their interplay with miRNAs or DEGs could help understand complex regulatory events regarding the DDR in response to IR.
There is also one main issue in our methodology to be considered. For instance, we made use of biomaRt to convert our transcript IDs to up-to-date HGNC symbol. However, biomaRt did not convert the following probe names of the transcripts: A_23_P113283, A_24_P88921, A_33_P3214501, A_33_P3232277, A_33_P3248227, A_33_P3252834, A_33_P3258452, A_33_P3258612, A_33_P3267410, A_33_P3345031, A_33_P3357748, A_33_P3361891, A_33_P3397763. When looking at these transcripts the GPL10332 platform associated to GSE102971, these could respectively be associated to the following genes or pseudogenes: ZMAT3, XPC, AEN, CCNG1, PVT1, PHLDA3, C12orf5, PCNA, KILLIN, LOC645978, PVT1, TMPRSS7, TNFSF9. In platform GPL13497 associated to GSE65292 and GSE90909, all transcripts, except for A_33_P3267410 and A_33_P3345031, corresponded to the same gene symbols. In this platform, A_33_P3267410 was associated to KLLN and A_33_P3345031 could only be associated to a chromosomal location and a sequence. However, when we tried finding these transcripts that biomaRt could not convert to up-to-date HGNC symbols in GPL1708, the platform associated to GSE8917, except for A_23_P113283 and A_24_P88921 that corresponded to ZMAT3 and XPC respectively, all other transcript IDs could not be converted. Although biomaRt is often used for mapping relevant sets of probe and target molecules, another tool should be considered to map Agilent Whole Human Genome Microarray transcript IDs to up-to-date HGNC symbols. Another caveat that we noticed is that there is a poor overlap in the validated miRNA-DEG interactions uncovered using miRTarBase and TarBase. This is further exemplified when looking at the predicted miRNA-DEG interactions uncovered using TargetScan, miRDB, and microT-CDS. This may be due to the fact that these three aforementioned databases use different algorithms to identify novel interaction pairs. In general, we decided to use overlapping results to minimize the identification of inaccurate interactions.
In conclusion, the workflow we established in this study was constructed to uncover regulatory mechanisms behind the cellular response to IR. To this end, we revealed insightful molecules such as DEGs, TFs, miRNAs, and lncRNAs, as well as potential regulation mechanisms involved in the DDR. We also suggested that our 20 radio-responsive DEGs have the potential to be used as IR biomarkers in terms of exposure, absorbed dose or dose rate. A specificity of our work is the stringent use of open source data and tools. We hope to motivate the reuse of existing resources, which can save time and effort for generating own data and building own tools. At the same time, we wish to stress the importance of making own project data available to domain-specific data bases in high quality and semantically enriched, for example, using GO terms. We conclude that our meaningful combination of open source data and tools is a valuable method to gain a new understanding in the cellular response to IR.
Data availability statement
The datasets presented in this study can be found in online repositories. The names of the repository/repositories and accession number(s) can be found in the article/Supplementary Material.
Author contributions
SF conceptualized and designed the study. SF performed the data preprocessing, data analysis and the regulatory data integration as well as the network analysis. SF generated all visualizations and wrote the manuscript. All authors revised the manuscript, read and approved the final manuscript.
Funding
This work was supported by the German Federal Ministry of Education and Research (FKZ 02NUK043C and FKZ 01ZZ 1801M).
Conflict of interest
The authors declare that the research was conducted in the absence of any commercial or financial relationships that could be construed as a potential conflict of interest.
Publisher’s note
All claims expressed in this article are solely those of the authors and do not necessarily represent those of their affiliated organizations, or those of the publisher, the editors and the reviewers. Any product that may be evaluated in this article, or claim that may be made by its manufacturer, is not guaranteed or endorsed by the publisher.
Supplementary material
The Supplementary Material for this article can be found online at: https://www.frontiersin.org/articles/10.3389/fsysb.2022.928159/full#supplementary-material
References
Abbasi, A., Hossain, L., and Leydesdorff, L. (2012). Betweenness centrality as a driver of preferential attachment in the evolution of research collaboration networks. J. Informetr. 6 (3), 403–412. doi:10.1016/j.joi.2012.01.002
Acharya, S. S., Fendler, W., Watson, J., Hamilton, A., Pan, Y., Gaudiano, E., et al. (2015). Serum microRNAs are early indicators of survival after radiation-induced hematopoietic injury. Sci. Transl. Med. 7 (287), 287ra69. doi:10.1126/scitranslmed.aaa6593
Assenov, Y., Ramírez, F., Schelhorn, S. E. S. E., Lengauer, T., and Albrecht, M. (2008). Computing topological parameters of biological networks. Bioinformatics 24 (2), 282–284. doi:10.1093/bioinformatics/btm554
Backes, C., Khaleeq, Q. T., Meese, E., and Keller, A. (2016). MiEAA: MicroRNA enrichment analysis and annotation. Nucleic Acids Res. 44 (1), W110–W116. doi:10.1093/nar/gkw345
Beer, L., Seemann, R., Ristl, R., Ellinger, A., Kasiri, M. M., Mitterbauer, A., et al. (2014). High dose ionizing radiation regulates micro RNA and gene expression changes in human peripheral blood mononuclear cells. BMC Genomics 15 (1), 814–821. doi:10.1186/1471-2164-15-814
Benderska, N., Dittrich, A. L., Knaup, S., Rau, T. T., Neufert, C., Wach, S., et al. (2015). miRNA-26b overexpression in ulcerative colitis-associated carcinogenesis. Inflamm. Bowel Dis. 21 (9), 2039–2051. doi:10.1097/MIB.0000000000000453
Benjamini, Y., and Hochberg, Y. (1995). Controlling the false discovery rate: A practical and powerful approach to multiple testing. J. R. Stat. Soc. Ser. B 57 (1), 289–300. doi:10.1111/j.2517-6161.1995.tb02031.x
Beuchel, C., Kirsten, H., Ceglarek, U., and Scholz, M. (2021). Metabolite-investigator: An integrated user-friendly workflow for metabolomics multi-study analysis. Bioinformatics 37 (15), 2218–2220. doi:10.1093/bioinformatics/btaa967
Boldt, S., Knops, K., Kriehuber, R., and Wolkenhauer, O. (2012). A frequency-based gene selection method to identify robust biomarkers for radiation dose prediction. Int. J. Radiat. Biol. 88 (3), 267–276. doi:10.3109/09553002.2012.638358
Bonferroni, C. (1936). Teoria statistica delle classi e calcolo delle probabilit `a. Pubbl del Ist Super Sci Econ Commer Firenze 8, 3–62.
Boroń, D., Zmarzły, N., Wierzbik-Strońska, M., Rosińczuk, J., Mieszczański, P., and Grabarek, B. O. (2022). Recent multiomics approaches in endometrial cancer. Int. J. Mol. Sci. 23 (3), 1237. doi:10.3390/ijms23031237
Broustas, C. G., Xu, Y., Harken, A. D., Chowdhury, M., Garty, G., and Amundson, S. A. (2017). Impact of neutron exposure on global gene expression in a human peripheral blood model. Radiat. Res. 187 (4), 433–440. doi:10.1667/RR0005.1
Ceccaldi, R., Rondinelli, B., and D’Andrea, A. D. (2016). Repair pathway choices and consequences at the double-strand break. Trends Cell Biol. 26 (1), 52–64. doi:10.1016/j.tcb.2015.07.009
Chakraborty, N., Gautam, A., Holmes-Hampton, G. P., Kumar, V. P., Biswas, S., Kumar, R., et al. (2020). microRNA and metabolite signatures linked to early consequences of lethal radiation. Sci. Rep. 10 (1), 5424. doi:10.1038/s41598-020-62255-w
Chen, P-H., Tseng, W. H-S., and Chi, J-T. (2020). The intersection of DNA damage response and ferroptosis—a rationale for combination therapeutics. Biology 9 (8), 187. doi:10.3390/biology9080187
Chen, Y., and Wang, X. (2020). MiRDB: An online database for prediction of functional microRNA targets. Nucleic Acids Res. 48 (D1), D127–D131. doi:10.1093/nar/gkz757
Cruz-Garcia, L., O’Brien, G., Sipos, B., Mayes, S., Love, M. I., Turner, D. J., et al. (2020a). Generation of a transcriptional radiation exposure signature in human blood using long-read nanopore sequencing. Radiat. Res. 193 (2), 143–154. doi:10.1667/RR15476.1
Cruz-Garcia, L., O’brien, G., Sipos, B., Mayes, S., Tichý, A., Sirák, I., et al. (2020b). In vivo validation of alternative fdxr transcripts in human blood in response to ionizing radiation. Int. J. Mol. Sci. 21 (21), 78511. doi:10.3390/ijms21217851
Dressman, H. K., Muramoto, G. G., Chao, N. J., Meadows, S., Marshall, D., Ginsburg, G. S., et al. (2007). Gene expression signatures that predict radiation exposure in mice and humans. PLoS Med. 4 (4), e106–e701. doi:10.1371/journal.pmed.0040106
Durinck, S., Moreau, Y., Kasprzyk, A., Davis, S., De Moor, B., Brazma, A., et al. (2005). BioMart and bioconductor: A powerful link between biological databases and microarray data analysis. Bioinformatics 21 (16), 3439–3440. doi:10.1093/bioinformatics/bti525
Edgar, R., Domrachev, M., and Lash, A. E. (2002). Gene expression Omnibus: NCBI gene expression and hybridization array data repository. Nucleic Acids Res. 30 (1), 207–210. doi:10.1093/nar/30.1.207
El-Saghire, H., Thierens, H., Monsieurs, P., Michaux, A., Vandevoorde, C., and Baatou, t. S. (2013). Gene set enrichment analysis highlights different gene expression profiles in whole blood samples X-irradiated with low and high doses. Int. J. Radiat. Biol. 89 (8), 628–638. doi:10.3109/09553002.2013.782448
Emanuele, M. J., Enrico, T. P., Mouery, R. D., Wasserman, D., Nachum, S., and Tzur, A. (2020). Complex cartography: Regulation of E2F transcription factors by cyclin F and ubiquitin. Trends Cell Biol. 30 (8), 640–652. doi:10.1016/j.tcb.2020.05.002
Fachin, A., Mello, S., Sandrin-Garcia, P., Junta, C., Donadi, E., Passos, G., et al. (2007). Gene expression profiles in human lymphocytes irradiated in vitro with low doses of gamma rays. Radiat. Res. 168 (6), 650–665. doi:10.1667/RR0487.1
Fendler, W., Malachowska, B., Meghani, K., Konstantinopoulos, P. A., Guha, C., Singh, V. K., et al. (2017). Evolutionarily conserved serum microRNAs predict radiation-induced fatality in nonhuman primates. Sci. Transl. Med. 9 (379), eaal2408. doi:10.1126/scitranslmed.aal2408
Freiesleben, S., Hecker, M., Zettl, U. K., Fuellen, G., and Taher, L. (2016). Analysis of microRNA and gene expression profiles in multiple sclerosis: Integrating interaction data to uncover regulatory mechanisms. Sci. Rep. 6 (1), 34512–34514. doi:10.1038/srep34512
Georgakilas, G., Vlachos, I. S., Zagganas, K., Vergoulis, T., Paraskevopoulou, M. D., Kanellos, I., et al. (2016). DIANA-miRGen v3.0: Accurate characterization of microRNA promoters and their regulators. Nucleic Acids Res. 44 (1), D190–D195. doi:10.1093/nar/gkv1254
Ghandhi, S. A., Smilenov, L., Shuryak, I., Pujol-Canadell, M., and Amundson, S. A. (2019). Discordant gene responses to radiation in humans and mice and the role of hematopoietically humanized mice in the search for radiation biomarkers. Sci. Rep. 9 (1), 19434. doi:10.1038/s41598-019-55982-2
Ghandhi, S. A., Smilenov, L. B., Elliston, C. D., Chowdhury, M., and Amundson, S. A. (2015). Radiation dose-rate effects on gene expression for human biodosimetry. BMC Med. Genomics 8 (1), 22. doi:10.1186/s12920-015-0097-x
Gnanasundram, S. V., Bonczek, O., Wang, L., Chen, S., and Fahraeus, R. (2021). p53 mRNA metabolism links with the DNA damage response. Genes 12 (9), 1446. doi:10.3390/genes12091446
Graw, S., Chappell, K., Washam, C. L., Gies, A., Bird, J., Robeson, M. S., et al. (2021). Multi-omics data integration considerations and study design for biological systems and disease. Mol. Omics 17 (2), 170–185. doi:10.1039/d0mo00041h
Griffiths-Jones, S. (2004). The microRNA Registry. Nucleic Acids Res. 32, D109–D111. doi:10.1093/nar/gkh023
Gruel, G., Voisin, P., Vaurijoux, A., Roch-Lefevre, S., Grégoire, E., Maltere, P., et al. (2008). Broad modulation of gene expression in CD4+ lymphocyte subpopulations in response to low doses of ionizing radiation. Radiat. Res. 170 (3), 335–344. doi:10.1667/RR1147.1
Han, F., Huang, D., Huang, X., Wang, W., Yang, S., and Chen, S. (2020). Exosomal microRNA-26b-5p down-regulates ATF2 to enhance radiosensitivity of lung adenocarcinoma cells. J. Cell. Mol. Med. 24 (14), 7730–7742. doi:10.1111/jcmm.15402
Haronikova, L., Olivares-Illana, V., Wang, L., Karakostis, K., Chen, S., and Fåhraeus, R. (2019). The p53 mRNA: An integral part of the cellular stress response. Nucleic Acids Res. 47 (7), 3257–3271. doi:10.1093/nar/gkz124
He, X., and Zhang, J. (2006). Why do hubs tend to Be essential in protein networks? PLoS Genet. 2 (6), e88. doi:10.1371/journal.pgen.0020088
Henríquez Hernández, L. A., Lara, P. C., Pinar, B., Bordón, E., Rodríguez Gallego, C., Bilbao, C., et al. (2009). Constitutive gene expression profile segregates toxicity in locally advanced breast cancer patients treated with high-dose hyperfractionated radical radiotherapy. Radiat. Oncol. 4, 17–7. doi:10.1186/1748-717X-4-17
Hsu, S-D., Lin, F-M., Wu, W-Y., Liang, C., Huang, W-C., Chan, W-L., et al. (2011). miRTarBase: a database curates experimentally validated microRNA-target interactions. Nucleic Acids Res. 39, D163–D169. doi:10.1093/nar/gkq1107
Hu, W., Zhang, C., Wu, R., Sun, Y., Levine, A., and Feng, Z. (2010). Glutaminase 2, a novel p53 target gene regulating energy metabolism and antioxidant function. Proc. Natl. Acad. Sci. U. S. A. 107 (16), 7455–7460. doi:10.1073/pnas.1001006107
Hu, X., Ding, D., Zhang, J., and Cui, J. (2019). Knockdown of lncRNA HOTAIR sensitizes breast cancer cells to ionizing radiation through activating miR-218. Biosci. Rep. 39 (4), BSR20181038. doi:10.1042/BSR20181038
Hu, Y., Lin, J., Fang, H., Fang, J., Li, C., Chen, W., et al. (2018). Targeting the MALAT1/PARP1/LIG3 complex induces DNA damage and apoptosis in multiple myeloma. Leukemia 32 (10), 2250–2262. doi:10.1038/s41375-018-0104-2
Huang, H. Y., Lin, Y. C. D., Li, J., Huang, K. Y., Shrestha, S., Hong, H. C., et al. (2020). MiRTarBase 2020: Updates to the experimentally validated microRNA-target interaction database. Nucleic Acids Res. 48 (D1), D148–D154. doi:10.1093/nar/gkz896
Jackson, S. P., and Bartek, J. (2009). The DNA-damage response in human biology and disease. Nature 461 (7267), 1071–1078. doi:10.1038/nature08467
Jia, M., and Wang, Z. (2022). MicroRNAs as biomarkers for ionizing radiation injury. Front. Cell Dev. Biol. 10, 861451. doi:10.3389/fcell.2022.861451
Kabacik, S., Mackay, A., Tamber, N., Manning, G., Finnon, P., Paillier, F., et al. (2011). Gene expression following ionising radiation: Identification of biomarkers for dose estimation and prediction of individual response. Int. J. Radiat. Biol. 87 (2), 115–129. doi:10.3109/09553002.2010.519424
Kang, C-M., Park, K-P., Song, J-E., Jeoung, D-I., Cho, C-K., Kim, T-H., et al. (2003). Possible biomarkers for ionizing radiation exposure in human peripheral blood lymphocytes. Radiat. Res. 159 (3), 312–319. doi:10.1667/0033-7587(2003)159[0312:pbfire]2.0.co;2
Ketley, R. F., and Gullerova, M. (2020). Jack of all trades? The versatility of RNA in DNA double-strand break repair. Essays Biochem. 64 (5), 721–735. doi:10.1042/EBC20200008
Knops, K., Boldt, S., Wolkenhauer, O., and Kriehuber, R. (2012). Gene expression in low- and high-dose-irradiated human peripheral blood lymphocytes: Possible applications for biodosimetry. Radiat. Res. 178 (4), 304–312. doi:10.1667/rr2913.1
Lacombe, J., Sima, C., Amundson, S. A., and Zenhausern, F. (2018). Candidate gene biodosimetry markers of exposure to external ionizing radiation in human blood: A systematic review. PLoS One 13 (6), 01988511. doi:10.1371/journal.pone.0198851
Lewis, B. P., Burge, C. B., and Bartel, D. P. (2005). Conserved seed pairing, often flanked by adenosines, indicates that thousands of human genes are microRNA targets. Cell 120 (1), 15–20. doi:10.1016/j.cell.2004.12.035
Li, P. P., Li, R. G., Huang, Y. Q., Lu, J. P., Zhang, W. J., and Wang, Z. Y. (2021). LncRNA OTUD6B-AS1 promotes paclitaxel resistance in triple negative breast cancer by regulation of miR-26a-5p/MTDH pathway-mediated autophagy and genomic instability. Aging (Albany NY) 13 (21), 24171–24191. doi:10.18632/aging.203672
Li, W., Zhou, S., Jia, M., Li, X., Li, L., Wang, Q., et al. (2022). Early biomarkers associated with P53 signaling for acute radiation injury. Life 12 (1), 99–16. doi:10.3390/life12010099
Li, Y., Zou, L., Yang, X., Chu, L., Ni, J., Chu, X., et al. (2019). Identification of lncRNA, MicroRNA, and mRNA-associated CeRNA network of radiation-induced lung injury in a mice model. Dose. Response. 17 (4), 1559325819891012. doi:10.1177/1559325819891012
Lieber, M. R. (2008). The mechanism of human nonhomologous DNA End joining. J. Biol. Chem. 283 (1), 1–5. doi:10.1074/jbc.R700039200
Liu, Y., Li, Y., and Lu, X. (2016). Regulators in the DNA damage response. Arch. Biochem. Biophys. 594, 18–25. doi:10.1016/j.abb.2016.02.018
Liu, Y., Zhang, G., Chen, H., and Wang, H. (2021). Silencing lncRNA DUXAP8 inhibits lung adenocarcinoma progression by targeting miR-26b-5p. Biosci. Rep. 41 (1), BSR20200884. doi:10.1042/BSR20200884
Luo, H., Zhong, F., Jing, X., Lin, H., and Li, Y. (2022). miRNA profiling of human nasopharyngeal carcinoma cell lines HONE1 and CNE2 after X-ray therapy. Adv. Clin. Exp. Med. 31, 671–687. doi:10.17219/acem/146580
Ma, L., Cao, J., Liu, L., Du, Q., Li, Z., Zou, D., et al. (2019). LncBook: A curated knowledgebase of human long non-coding RNAs. Nucleic Acids Res. 47 (5), D128–D134. doi:10.1093/nar/gky960
Macaeva, E., Saeys, Y., Tabury, K., Janssen, A., Michaux, A., Benotmane, M. A., et al. (2016). Radiation-induced alternative transcription and splicing events and their applicability to practical biodosimetry. Sci. Rep. 6 (1), 19251. doi:10.1038/srep19251
May, J. M., Bylicky, M., Chopra, S., Coleman, C. N., and Aryankalayil, M. J. (2021). Long and short non-coding RNA and radiation response: A review. Transl. Res. 233, 162–179. doi:10.1016/j.trsl.2021.02.005
Mayer, C., Popanda, O., Greve, B., Fritz, E., Illig, T., Eckardt-Schupp, F., et al. (2011). A radiation-induced gene expression signature as a tool to predict acute radiotherapy-induced adverse side effects. Cancer Lett. 302 (1), 20–28. doi:10.1016/j.canlet.2010.12.006
Meadows, S. K., Dressman, H. K., Muramoto, G. G., Himburg, H., Salter, A., Wei, Z. Z., et al. (2008). Gene expression signatures of radiation response are specific, durable and accurate in mice and humans. PLoS One 3 (4), e1912–e1919. doi:10.1371/journal.pone.0001912
Mitxelena, J., Apraiz, A., Vallejo-Rodríguez, J., García-Santisteban, I., Fullaondo, A., Alvarez-Fernández, M., et al. (2018). An E2F7-dependent transcriptional program modulates DNA damage repair and genomic stability. Nucleic Acids Res. 46 (9), 4546–4559. doi:10.1093/nar/gky218
Mitxelena, J., Apraiz, A., Vallejo-Rodriguez, J., Malumbres, M., and Zubiaga, A. M. (2016). E2F7 regulates transcription and maturation of multiple microRNAs to restrain cell proliferation. Nucleic Acids Res. 44 (12), 5557–5570. doi:10.1093/nar/gkw146
Morgan, W. F., Day, J. P., Kaplan, M. I., McGhee, E. M., and Limoli, C. L. (1996). Genomic instability induced by ionizing radiation. Radiat. Res. 146 (3), 247–258. doi:10.2307/3579454
Murashko, M. M., Stasevich, E. M., Schwartz, A. M., Kuprash, D. V., Uvarova, A. N., and Demin, D. E. (2021). The role of RNA in DNA breaks, repair and chromosomal rearrangements. Biomolecules 11 (4), 550. doi:10.3390/biom11040550
Nag, S., Qin, J., Srivenugopal, K. S., Wang, M., and Zhang, R. (2013). The MDM2-p53 pathway revisited. J. Biomed. Res. 27 (4), 254–271. doi:10.7555/JBR.27.20130030
Noble, A. J., Purcell, R. V., Adams, A. T., Lam, Y. K., Ring, P. M., Anderson, J. R., et al. (2022). A final frontier in environment-genome interactions? Integrated, multi-omic approaches to predictions of non-communicable disease risk. Front. Genet. 13, 831866. doi:10.3389/fgene.2022.831866
Nosel, I., Vaurijoux, A., Barquinero, J-F., and Gruel, G. (2013). Characterization of gene expression profiles at low and very low doses of ionizing radiation. DNA Repair (Amst) 12 (7), 508–517. doi:10.1016/j.dnarep.2013.04.021
O’Brien, J., Hayder, H., Zayed, Y., and Peng, C. (2018). Overview of microRNA biogenesis, mechanisms of actions, and circulation. Front. Endocrinol. 9, 402. doi:10.3389/fendo.2018.00402
Okazaki, R. (2022). Role of p53 in regulating radiation responses. Life 12 (7), 1099. doi:10.3390/life12071099
Pampfer, S., and Streffer, C. (1989). Increased chromosome aberration levels in cells from mouse fetuses after zygote X-irradiation. Int. J. Radiat. Biol. 55 (1), 85–92. doi:10.1080/09553008914550091
Paraskevopoulou, M. D., Vlachos, I. S., Karagkouni, D., Georgakilas, G., Kanellos, I., Vergoulis, T., et al. (2016). DIANA-LncBase v2: Indexing microRNA targets on non-coding transcripts. Nucleic Acids Res. 44 (1), D231–D238. doi:10.1093/nar/gkv1270
Park, J. G., Paul, S., Briones, N., Zeng, J., Gillis, K., Wallstrom, G., et al. (2017). Developing human radiation biodosimetry models: Testing cross-species conversion approaches using an ex vivo model system. Radiat. Res. 187 (6), 708–721. doi:10.1667/RR14655.1
Paul, S., and Amundson, S. (2011). Gene expression signatures of radiation exposure in peripheral white blood cells of smokers and non-smokers. Int. J. Radiat. Biol. 87 (8), 791–801. doi:10.3109/09553002.2011.568574
Paul, S., and Amundson, S. A. (2008). Development of gene expression signatures for practical radiation biodosimetry. Int. J. Radiat. Oncol. Biol. Phys. 71 (4), 1236–1244. doi:10.1016/j.ijrobp.2008.03.043
Paul, S., Barker, C. A., Turner, H. C., McLane, A., Wolden, S. L., and Amundson, S. A. (2011). Prediction of in vivo radiation dose status in radiotherapy patients using ex vivo and in vivo gene expression signatures. Radiat. Res. 175 (3), 257–265. doi:10.1667/RR2420.1
Paul, S., Smilenov, L. B., and Amundson, S. A. (2013). Widespread decreased expression of immune function genes in human peripheral blood following radiation exposure. Radiat. Res. 180 (6), 575–583. doi:10.1667/RR13343.1
Piotto, C., Biscontin, A., Millino, C., and Mognato, M. (2018). Functional validation of miRNAs targeting genes of DNA double-strand break repair to radiosensitize non-small lung cancer cells. Biochim. Biophys. Acta. Gene Regul. Mech. 1861 (12), 1102–1118. doi:10.1016/j.bbagrm.2018.10.010
Pogosova-Agadjanyan, E. L., Fan, W., Georges, G. E., Schwartz, J. L., Kepler, C. M., Lee, H., et al. (2011). Identification of radiation-induced expression changes in nonimmortalized human T cells. Radiat. Res. 175 (2), 172–184. doi:10.1667/rr1977.1
Qi, Z., Guo, S., Li, C., Wang, Q., Li, Y., and Wang, Z. (2020). Integrative analysis for the roles of lncRNAs in the immune responses of mouse PBMC exposed to low-dose ionizing radiation. Dose. Response. 18 (1), 1559325820913800. doi:10.1177/1559325820913800
R Core Team (2013). R: A language and environment for statistical computing. Vienna, Austria: R Foundation for Statistical Computing. Available from: http://www.r-project.org.
Raudvere, U., Kolberg, L., Kuzmin, I., Arak, T., Adler, P., Peterson, H., et al. (2019). g:Profiler: a web server for functional enrichment analysis and conversions of gene lists (2019 update). Nucleic Acids Res. 47 (1), W191–W198. doi:10.1093/nar/gkz369
Reczko, M., Maragkakis, M., Alexiou, P., Grosse, I., and Hatzigeorgiou, A. G. (2012). Functional microRNA targets in protein coding sequences. Bioinformatics 28 (6), 771–776. doi:10.1093/bioinformatics/bts043
Ritchie, M. E., Phipson, B., Wu, D., Hu, Y., Law, C. W., Shi, W., et al. (2015). Limma powers differential expression analyses for RNA-sequencing and microarray studies. Nucleic Acids Res. 43 (7), e47. doi:10.1093/nar/gkv007
Rouchka, E. C., Flight, R. M., Fasciotto, B. H., Estrada, R., Eaton, J. W., Patibandla, P. K., et al. (2016). Transcriptional profile of immediate response to ionizing radiation exposure. Genom. Data 7, 82–85. doi:10.1016/j.gdata.2015.11.027
Roy, S., Praneetha, D., and Vendra, V. (2015). Mutations in the corneal endothelial dystrophy-associated gene SLC4A11 render the cells more vulnerable to oxidative insults. Cornea 34 (6), 668–674. doi:10.1097/ICO.0000000000000421
San Filippo, J., Sung, P., and Klein, H. (2008). Mechanism of eukaryotic homologous recombination. Annu. Rev. Biochem. 77, 229–257. doi:10.1146/annurev.biochem.77.061306.125255
Schmitt, A. M., Garcia, J. T., Hung, T., Flynn, R. A., Shen, Y., Qu, K., et al. (2016). An inducible long noncoding RNA amplifies DNA damage signaling. Nat. Genet. 48 (11), 1370–1376. doi:10.1038/ng.3673
Schmitz, U., Lai, X., Winter, F., Wolkenhauer, O., Vera, J., and Gupta, S. K. (2014). Cooperative gene regulation by microRNA pairs and their identification using a computational workflow. Nucleic Acids Res. 42 (12), 7539–7552. doi:10.1093/nar/gku465
Shannon, P., Markiel, A., Ozier, O., Baliga, N. S., Wang, J. T., Ramage, D., et al. (2003). Cytoscape: A software environment for integrated models of biomolecular interaction networks. Genome Res. 13 (11), 2498–2504. doi:10.1101/gr.1239303
Shaw, A., and Gullerova, M. (2021). Home and away: The role of non-coding RNA in intracellular and intercellular DNA damage response. Genes 12 (10), 1475. doi:10.3390/genes12101475
St.Laurent, G., Wahlestedt, C., and Kapranov, P. (2015). The Landscape of long non-coding RNA classification. Trends Genet. 31 (5), 239–251. doi:10.1016/j.tig.2015.03.007
Suh, S. S., Yoo, J. Y., Nuovo, G. J., Jeon, Y. J., Kim, S., Lee, T. J., et al. (2012). MicroRNAs/TP53 feedback circuitry in glioblastoma multiforme. Proc. Natl. Acad. Sci. U. S. A. 109 (14), 5316–5321. doi:10.1073/pnas.1202465109
Suzuki, S., Tanaka, T., Poyurovsky, M. V., Nagano, H., Mayama, T., Ohkubo, S., et al. (2010). Phosphate-activated glutaminase (GLS2), a p53-inducible regulator of glutamine metabolism and reactive oxygen species. Proc. Natl. Acad. Sci. U. S. A. 107 (16), 7461–7466. doi:10.1073/pnas.1002459107
Templin, T., Paul, S., Amundson, S. A., Young, E. F., Barker, C. A., Wolden, S. L., et al. (2011). Radiation-induced micro-RNA expression changes in peripheral blood cells of radiotherapy patients. Int. J. Radiat. Oncol. Biol. Phys. 80 (2), 549–557. doi:10.1016/j.ijrobp.2010.12.061
Teng, X., Chen, X., Xue, H., Tang, Y., Zhang, P., Kang, Q., et al. (2020). NPInter v4.0: An integrated database of ncRNA interactions. Nucleic Acids Res. 48 (1), D160–D165. doi:10.1093/nar/gkz969
Thapar, R. (2018). Regulation of DNA double-strand break repair by non-coding RNAs. Molecules 23 (11), 2789. doi:10.3390/molecules23112789
Tong, Z., Cui, Q., Wang, J., and Zhou, Y. (2019). TransmiR v2.0: An updated transcription factor-microRNA regulation database. Nucleic Acids Res. 47 (D1), D253–D258. doi:10.1093/nar/gky1023
Tsogbadrakh, B., Jung, J. A., Lee, M., Lee, J. A., and Seo, J. H. (2022). Identifying serum miRNA biomarkers for radiation exposure in hematopoietic humanized NSG-SGM3 mice. Biochem. Biophys. Res. Commun. 599, 51–56. doi:10.1016/j.bbrc.2022.02.010
Vahabi, N., and Michailidis, G. (2022). Unsupervised multi-omics data integration methods: A comprehensive review. Front. Genet. 13, 854752. doi:10.3389/fgene.2022.854752
Versteyhe, S., Driessens, N., Ghaddhab, C., Tarabichi, M., Hoste, C., Dumont, J. E., et al. (2013). Comparative analysis of the thyrocytes and T cells: Responses to H 2O2 and radiation reveals an H2O 2-induced antioxidant transcriptional program in thyrocytes. J. Clin. Endocrinol. Metab. 98 (10), 1645–1654. doi:10.1210/jc.2013-1266
Vinoth, K. J., Manikandan, J., Sethu, S., Balakrishnan, L., Heng, A., Lu, K., et al. (2014). Evaluation of human embryonic stem cells and their differentiated fibroblastic progenies as cellular models for in vitro genotoxicity screening. J. Biotechnol. 184, 154–168. doi:10.1016/j.jbiotec.2014.05.009
Wan, G., Mathur, R., Hu, X., Liu, Y., Zhang, X., Peng, G., et al. (2013). Long non-coding RNA ANRIL (CDKN2B-AS) is induced by the ATM-E2F1 signaling pathway. Cell. Signal. 25 (5), 1086–1095. doi:10.1016/j.cellsig.2013.02.006
Wang, P., Liu, H., Cheng, L., Zhou, W., Jin, X., Xu, Z., et al. (2019). Lncrna2target V3.0: A comprehensive database for target genes of lncRNAs in human and mouse. Nucleic Acids Res. 47 (1), D140–D144. doi:10.1093/nar/gky1051
Ward, J. F. (1988). DNA damage produced by ionizing radiation in mammalian cells: Identities, mechanisms of formation, and reparability. Prog. Nucleic Acid. Res. Mol. Biol. 35, 95–125. doi:10.1016/s0079-6603(08)60611-x
Ward, J. F. (1994). The complexity of DNA damage: Relevance to biological consequences. Int. J. Radiat. Biol. 66 (5), 427–432. doi:10.1080/09553009414551401
Wen, Q., Hu, Y., Zhang, X., Kong, P., and Chen, X. (2011). Gene expression signature of lymphocyte in acute lymphoblastic leukemia patients immediately after total body irradiation. Leuk. Res. 35 (8), 1044–1051. doi:10.1016/j.leukres.2010.12.026
Wilke, C. M., Hess, J., Klymenko, S. V., Chumak, V. V., Zakhartseva, L. M., Bakhanova, E. V., et al. (2018). Expression of miRNA-26b-5p and its target TRPS1 is associated with radiation exposure in post-Chernobyl breast cancer. Int. J. Cancer 142 (3), 573–583. doi:10.1002/ijc.31072
Xiao, J., Lin, H., Luo, X., Luo, X., and Wang, Z. (2011). MiR-605 joins p53 network to form a p53:miR-605:Mdm2 positive feedback loop in response to stress. EMBO J. 30 (3), 524–532. doi:10.1038/emboj.2010.347
Yang, M., Sun, Y., Xiao, C., Ji, K., Zhang, M., He, N., et al. (2019). Integrated analysis of the altered lncRNAs and mRNAs expression in 293T cells after ionizing radiation exposure. Int. J. Mol. Sci. 20 (12), 2968. doi:10.3390/ijms20122968
Yu, F., Zhang, G., Shi, A., Hu, J., Li, F., Zhang, X., et al. (2018). LnChrom: A resource of experimentally validated lncRNA-chromatin interactions in human and mouse. Database (Oxford). 2018, 1–7. doi:10.1093/database/bay039
Zhang, C., and Peng, G. (2015). Non-coding RNAs: An emerging player in DNA damage response. Mutat. Res. Rev. Mutat. Res. 763, 202–211. doi:10.1016/j.mrrev.2014.11.003
Zhang, J., Sun, Q., Zhang, Z., Ge, S., Han, Z. G., and Chen, W. T. (2013). Loss of microRNA-143/145 disturbs cellular growth and apoptosis of human epithelial cancers by impairing the MDM2-p53 feedback loop. Oncogene 32 (1), 61–69. doi:10.1038/onc.2012.28
Zhang, Q., Zhu, L., Wang, G., Zhao, Y., Xiong, N., Bao, H., et al. (2017). Ionizing radiation promotes CCL27 secretion from keratinocytes through the cross talk between TNF-α and ROS. J. Biochem. Mol. Toxicol. 31 (3), e21868. doi:10.1002/jbt.21868
Zhao, Y., Zhu, C., Chang, Q., Peng, P., Yang, J., Liu, C., et al. (2020). MiR-424-5p regulates cell cycle and inhibits proliferation of hepatocellular carcinoma cells by targeting E2F7. PLoS One 15 (11), e0242179. doi:10.1371/journal.pone.0242179
Keywords: DNA damage response, ionizing radiation, regulatory network, data integration, lncRNA, miRNA, transcriptomics, open source
Citation: Freiesleben S, Unverricht-Yeboah M, Gütebier L, Waltemath D, Kriehuber R and Wolkenhauer O (2022) A workflow for the creation of regulatory networks integrating miRNAs and lncRNAs associated with exposure to ionizing radiation using open source data and tools. Front. Syst. Biol. 2:928159. doi: 10.3389/fsysb.2022.928159
Received: 25 April 2022; Accepted: 09 September 2022;
Published: 10 October 2022.
Edited by:
Leda Torres, Instituto Nacional de Pediatría, MexicoReviewed by:
Hugo Tovar, Instituto Nacional de Medicina Genómica (INMEGEN), MexicoLlewelyn Moron-Espiritu, De La Salle University, Philippines
Copyright © 2022 Freiesleben, Unverricht-Yeboah, Gütebier, Waltemath, Kriehuber and Wolkenhauer. This is an open-access article distributed under the terms of the Creative Commons Attribution License (CC BY). The use, distribution or reproduction in other forums is permitted, provided the original author(s) and the copyright owner(s) are credited and that the original publication in this journal is cited, in accordance with accepted academic practice. No use, distribution or reproduction is permitted which does not comply with these terms.
*Correspondence: Sherry Freiesleben, c2hlcnJ5LmZyZWllc2xlYmVuQG1lZC51bmktZ3JlaWZzd2FsZC5kZQ==