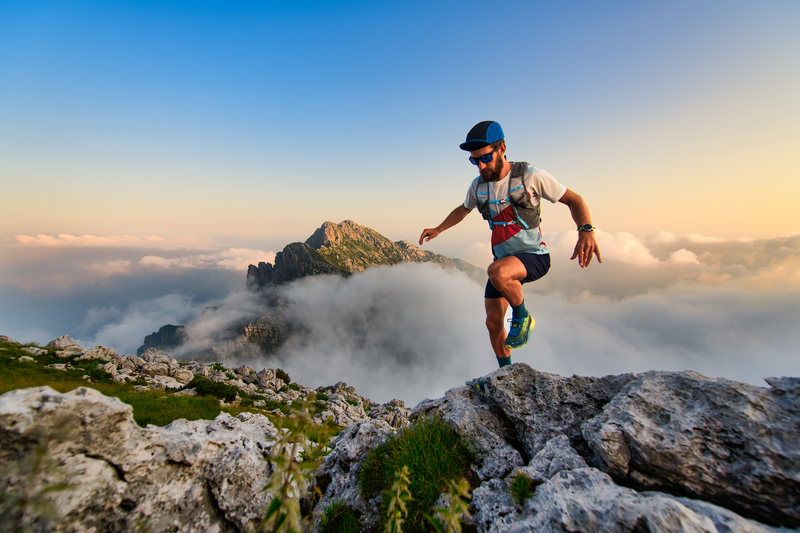
94% of researchers rate our articles as excellent or good
Learn more about the work of our research integrity team to safeguard the quality of each article we publish.
Find out more
REVIEW article
Front. Synth. Biol.
Sec. Ecosystems and Biodiversity Sustainability
Volume 3 - 2025 | doi: 10.3389/fsybi.2025.1532846
This article is part of the Research Topic Harnessing Synthetic Biology for Ecosystem Sustainability and Biodiversity Conservation View all 3 articles
The final, formatted version of the article will be published soon.
You have multiple emails registered with Frontiers:
Please enter your email address:
If you already have an account, please login
You don't have a Frontiers account ? You can register here
Microbial communities are able to carry out myriad functions of biotechnological interest, ranging from the degradation of industrial waste to the synthesis of valuable chemical products. Over the past years, several strategies have emerged for the design of microbial communities and the optimization of their functions. Here we provide an accessible overview of these strategies. We highlight how principles of synthetic biology, originally devised for the engineering of individual organisms and sub-organismal units (e.g., enzymes), have influenced the development of the field of synthetic microbial ecology. With this, we aim to encourage readers to critically evaluate how insights from synthetic biology should guide our approach to community-level engineering.
Keywords: microbial ecology, synthetic ecology, synthetic microbial communities, Synthetic & Systems Biology, microbial commnities
Received: 22 Nov 2024; Accepted: 03 Mar 2025.
Copyright: © 2025 San Román, Arrabal, Benitez-Dominguez, Quirós-Rodríguez and Diaz-Colunga. This is an open-access article distributed under the terms of the Creative Commons Attribution License (CC BY). The use, distribution or reproduction in other forums is permitted, provided the original author(s) or licensor are credited and that the original publication in this journal is cited, in accordance with accepted academic practice. No use, distribution or reproduction is permitted which does not comply with these terms.
* Correspondence:
Juan Diaz-Colunga, Institute of Functional Biology and Genomics, Spanish National Research Council (CSIC), Salamanca, 37007, Spain
Disclaimer: All claims expressed in this article are solely those of the authors and do not necessarily represent those of their affiliated organizations, or those of the publisher, the editors and the reviewers. Any product that may be evaluated in this article or claim that may be made by its manufacturer is not guaranteed or endorsed by the publisher.
Research integrity at Frontiers
Learn more about the work of our research integrity team to safeguard the quality of each article we publish.