- 1Department of Biosystems Engineering, University of Manitoba, Winnipeg, MB, Canada
- 2International Institute for Sustainable Development (IISD), Winnipeg, MB, Canada
- 3Department of Soil Science, University of Manitoba, Winnipeg, MB, Canada
Developing strategies for the prevention of antimicrobial resistance genes (ARG) in wastewater treatment facilities, such as municipal wastewater sewage lagoons, is important to prevent ARG introduction and proliferation to the environment. While previous studies have shown promising results in reducing ARGs through biological and phytoremediation systems like Constructed Wetlands, the underlying mechanisms and the impact of water chemistry on ARG reduction remain poorly understood. This study used metagenomics to investigate the effect of three different biological wastewater treatments on the microbial community and resistome. The results indicated that each biological treatment significantly reduced ARGs and metal resistance genes (MRGs). A network analysis identified eight bacterial genera strongly associated with resistance genes, six of which belonged to the phylum Pseudomonadota and two to Campylobacteria. Of the six genera in Pseudomonadota, four were facultative anaerobes. A strong correlation was observed between iron (Fe) concentration in water and total ARG abundance (r = 0.95), as well as between total metal concentration (across eight measured metals) and ARGs (r = 0.93). Additionally, there was a significant correlation between ARG abundance and Fe resistance (r = 0.86). This implies that high Fe and metal concentrations may favor ARG-harboring facultative bacteria by providing an electron donor for anaerobic respiration. This study suggests that phytoextraction of metals, including Fe, may play a significant role in reducing ARG abundance in treated water columns. These findings offer valuable insights into how metal removal and phytoremediation can effectively reduce ARG levels in wastewater.
1 Introduction
Antimicrobial resistance is one of the greatest threats to human health in the 21st century (WHO, 2023). Before the discovery and use of antimicrobials, communicable diseases were the leading cause of death worldwide (Adedeji, 2016). Thanks to the success of antibiotics, communicable diseases are no longer the leading cause of death; today, non-communicable diseases such as cancer, heart disease, and stroke have taken their place (Adedeji, 2016). From the time antibiotics were first discovered in 1928 to the end of the golden era of antibiotic discovery in 1970 (Adedeji, 2016), the average life expectancy of a baby born in the USA rose from 57.5 to 71 years. This significant improvement in human health is now jeopardized by the development and spread of antimicrobial resistance in pathogenic bacteria.
Wastewater is known to promote antimicrobial resistance and horizontal gene transfer in microbial communities (Sambaza and Naicker, 2023). Antimicrobial resistance genes (ARGs) may occur in bacteria through natural evolution or acquired processes. The presence of xenobiotic contaminants such as antimicrobials, heavy metals, and biocides can promote the development of ARGs through forced selection and suppression of non-resistant bacteria (Murray et al., 2024). Horizontal gene transfer (HGT) via mobile genetic elements (MGEs), such as transposons, plasmids, integrons, and viral elements, can facilitate the transfer and acquisition of ARGs from one bacterium to another. Pollution from sources such as wastewater from human and animal waste contributes to the proliferation of antibiotic-resistant bacteria (ARB) in the environment. Once in the environment, these ARBs pose a risk of exposure and infection to human and animal populations (Larsson and Flach, 2022). Therefore, it is crucial to understand how to control and reduce the release of ARBs and ARGs in wastewater effluents. Conventional methods such as chlorination, UV disinfection, or oxidation can result in bacterial regrowth and do not reduce the relative abundance of ARGs (Mosaka et al., 2022), potentially leading to ARG selection rather than removal. Hence, it is imperative to develop biotic treatment strategies that control ARG development and dissemination through evolutionary processes, reducing ARG selection and relative abundance in wastewater.
Constructed wetlands have been investigated as a treatment strategy for removing antimicrobials and ARGs from wastewater (Liu et al., 2019; McCorquodale-Bauer et al., 2023). While the treatment of antimicrobials in wastewater is important, antimicrobial concentrations are often below minimum inhibitory concentrations (MICs) and minimum selection concentrations (MSCs) in wastewater effluents and the environment (Larsson and Flach, 2022). Although antimicrobial concentrations are often correlated with ARG abundance, this is likely due to the presence of both in pollution sources and the development of ARGs within the microbial community of a treated host, rather than the selection forces enacted by wastewater antimicrobial concentration (Larsson and Flach, 2022). Factors such as metal concentration, pH, dissolved oxygen (DO), and nutrients canplay a significant role in the promotion or removal of ARBs and ARGs due to their influence on the microbial community (Jampani et al., 2024).
The co-occurrence and co-selection of ARGs and metal resistance genes (MRGs) are well-studied phenomena (Li et al., 2016; Amarasekara et al., 2023; Li et al., 2022). This is partly due to genetic linkage and chromosomal proximity (Li et al., 2016). When ARGs and MRGs are clustered on MGEs, the spread and acquisition of these genes will proliferate throughout the microbial community if the acquired genes provide molecular machinery that confers a competitive advantage to the receiving microorganism. Additionally, similar mechanisms of resistance, such as efflux pumps and reduced permeability, are common between antimicrobial resistance and metal resistance (Edet et al., 2023). Heavy metals have also been found to interact with antimicrobials, increasing the development of ARGs in bacteria (Sutradhar et al., 2023).
Biological treatments such as phytoremediation and algae-remediation are well suited for the uptake and removal of metal ions in wastewater (Mandal et al., 2024; Sarma et al., 2024). Furthermore, biological treatments can change water quality factors, such as nutrients, biological oxygen demand (BOD), chemical oxygen demand (COD), and dissolved oxygen (DO) (Blandford et al., 2024; Wibowo et al., 2023), influencing biotic factors such as microbial resistance. This study investigated the role of biological treatments on wastewater resistomes (complete set of ARGs and MRGs) and mobilomes (complete set of MGEs) via heavy metal remediation and water chemistry effects. The correlation between heavy metals, MRGs, ARGs, and specific bacterial genera was investigated to explore and expound on the sources of ARGs in wastewater and the treatment mechanisms affected by biological treatments.
2 Methodology
2.1 Experimental design
The current study investigated the resistome and taxonomy of the microbial community in municipal wastewater and the effects of biological treatment on these factors. The Village of Dunnottar Wastewater Treatment Facility (50° 26′ 39.3″N, 97° 01′ 04.0″W), located near the west shore of Lake Winnipeg’s south basin (approximately 1 h north of Winnipeg, Manitoba, Canada), is a municipal sewage lagoon servicing 989 permanent residents and a summertime (cottage) population of approximately 3,000. The lagoon consists of a primary, secondary and tertiary treatment cell (Supplementary Figure S2). The lagoon works through continuous flow and raw sewage is delivered to the primary cell by septic truck. The Dunnottar Wastewater Treatment Facility was used to study microbial resistome through both in situ sampling and as the source influent for a mesocosm experiment described below.
Supplementary Table S1 provides an outline of all samples taken throughout the study along with their location, material composition, date of collection and analysis performed.
2.1.1 Lagoon sampling
Samples for metagenomic analysis were taken from the primary lagoon and secondary lagoon along with total phosphorus (TP), soluble reactive phosphorus (SRP), COD, total organic carbon (TOC), DO, and total metals. In the current study, these results were used to provide background to the mesocosm study and toward the correlation and network analysis presented in the results section. Samples were taken on day 0 and day 45 of the study. In addition to water column samples, sediment samples were also taken from the secondary lagoon.
There were five in-situ full-scale floating treatment wetlands (FTWs) in the secondary sewage lagoon. The FTWs were arranged in a square, creating a corral in the middle. Samples were taken from inside the FTW corral (Secondary lagoon Sample 1 and Sample 2) and from the in-situ plant rhizome biofilm (JR1 and JR2 in Supplementary Material). These samples were used for the correlation and network analysis presented in the results and discussion section.
2.1.2 Mesocosm experiment
Wastewater from the secondary sewage lagoon was pumped into 9 mesocosms (2 m diameter, 600 L). Each mesocosm was treated with either transplanted Lemna (from the secondary sewage lagoon), emerged macrophytes on a FTW mat, or algae from the secondary lagoon. Samples for metagenomic analysis were taken on day 45 of the experiment (15th August) from each mesocosm. The source water from the secondary lagoon was also sampled on day 0 as described in the previous section. A sample from the secondary lagoon [Secondary (August 15)] was taken on day 45 (15th August), the same day as the mesocosms were sampled. This sample served as a control for the treatment occurring in the lagoon over the same period as the mesocosm experiment. The objective of the mesocosm experiment was to investigate the effect of each biological treatment (described below) on the microbial community and the resistome. The experimental design was a randomized complete block (Figure 1) with a one-way treatment (Lemna, macrophytes, algae) layout and three replicates (blocks) per treatment.
2.1.3 Biological treatments
2.1.3.1 Emerged macrophyte
The emerged macrophyte system is known as a Floating Treatment Wetland (FTW) and is a specific type of constructed wetland that grows through primarily hydroponic mechanisms. In the current study, the emerged macrophytes were established over the previous summer (2022) and consisted of a variety of wetland species initially transplanted from a local ditch located near the sewage lagoon. Each mesocosm treated with emerged macrophytes held one FTW consisting of 40 pots. Each pot (diameter = 70 mm, depth = 80 mm) contained one plant. The plant species were mostly Typha sp., Carrix sp., and Phragmites sp.
2.1.3.2 Lemna
Mesocosms treated with Lemna received 100 g of wet Lemna (washed) from the secondary sewage lagoon. Due to the still water conditions provided by the mesocosm and the high nutrient levels from the lagoon, Lemna grew and spread across the entire mesocsom surface.
2.1.3.3 Algae
Algae from the secondary sewage lagoon were pumped into each mesocosm. In the absence of plants the algae grew and proliferated in the unplanted mesocosms and dominated the surface of the water column. Algae species included Tetradesmus obliquus, Pseudococcomyxa simplex, Pseudopediastrum integrum, Pediastrum duplex, and Cosmarium regnellii.
TP, SRP, COD, DO, pH, temperature, and metal ion concentrations were measured throughout the 100-day experiment period. Samples for metagenomic analysis were taken from the source water (secondary lagoon) on day 0 and from the mesocosms on day 45. These samples were compared to corresponding samples from the secondary and primary lagoons.
2.2 Trace metal analysis
Water and plant tissue were analyzed for metal concentration using an Agilent 7850 inductively coupled plasma mass spectrometer (ICP-MS following sample digestion with HCl and HNO3 according to U.S. EPA SW-846 Method 3010A and Thermo Scientific AN 4344620). Along with measuring metal concentrations in the mesocosms, metal concentrations were measured at each metagenomic sampling point in the primary and secondary lagoon on the corresponding day.
2.3 Metagenomic shotgun sequencing
2.3.1 DNA extraction
DNA was isolated from wastewater, lagoon sludge, and root biofilm samples as follows: Samples were centrifuged at 12,400 rpm (13,600 × g) for 15 min using a Microcentrifuge with a 4-978-241F 20 Place Rotor (Fisher Scientific). The pellets were washed twice with deionized water to remove residual components (e.g., minerals) that could interfere with the subsequent steps. DNA was extracted from 350 mg of the centrifuged pellets using the PowerSoil DNA extraction kit (Omega Bio-tek, United States), according to the manufacturer’s instructions. For water samples, the PowerWater kit (QIAGEN, United States) was used. DNA concentration was measured with a NanoDrop 1000 (Thermo Fisher Scientific, Canada). DNA samples were then stored at −20°C in 2 mL nuclease-free microcentrifuge tubes until they were sent for sequencing.
2.3.2 Sequencing
DNA samples were sent to the McGill University Genome Quebec Innovation Centre in Montréal, QC, Canada, where shotgun metagenomic sequencing was performed using an Illumina NovSeq PE150 platform. The paired-end sequences generated were then provided by the institution. These sequences were deposited in the Bioproject PRJNA1165796.
2.3.3 Metagenomic analysis
This study employed a comprehensive workflow to process and analyze DNA sequences, consisting of four main steps: raw data processing, classification, assembly, and alignment. The workflow is outlined in Supplementary Figure S3 and described below.
The initial step involved processing the raw DNA sequence data using Trimmomatic v.039 (Bolger et al., 2014). The process included trimming four bases from both the start (leading) and the end (trailing) of a read if they fell below the set quality threshold. Additionally, sliding window trimming with a window size of four and a minimum quality score of 20 was utilized to excise low-quality segments of reads.
Quality-controlled sequences were classified using Kraken v2.1.2 (Wood et al., 2019) in the fast operation mode (60% confidence, a minimum of two k-mer group hits) and its standard database (10 October 2023). The OTU’s relative abundances in each sample were estimated with Bracken v2.7 (Lu et al., 2017).
The quality-controlled sequences were assembled into contigs with MEGAHIT v1.2.9 (Li et al., 2015) using the default parameters. These contigs were aligned against the CARD v3.1 (Alcock et al., 2020), BacMet2-experimentally confirmed (Pal et al., 2014), MobileOG v1.5 (Brown et al., 2022), and the Sulfur Metabolizing Database (SMDB) (Mo et al., 2023) using DIAMOND blastx v2.0.15 (Buchfink et al., 2015) in n sensitive mode with the following parameters and thresholds: e-values < 1e-10; identity percentage >90%; maximum number of query hits <3; and a minimum of 25 amino acids. All detected genes were classified according to the description of their respective databases. The abundance of each gene was normalized to the number of contigs in each sample and the length of the gene and reported as reads per kilobase million (rpkm).
2.4 Data analysis and visualization
The results derived from the metagenomic analyses were imported into R (RStudio Team, 2020) for statistical analysis and data visualization. The average values and the standard deviations (when n > 1) of the different parameters evaluated were reported. A Bayesian-multiplicative replacement was applied to reduce the sparsity of the microbiome data using the R package zCompositions (Palarea-Albaladejo and Antoni Martín-Fernández, 2015). Data visualization was performed using ggplot2 (Wickham, 2016). The R package Metacoder (Foster et al., 2017) was used to generate differential heat-trees of the changes in the resistome and mobilome profiles after the treatments. For this, the log2 of the fold change (log2 FC) of the total abundance of the different genes (ARGs, MRGs, and MGEs) was estimated and then encoded in the color of the plot. The number of genes in each level of the trees was used to define the size of the nodes and edges.
Spearman’s rank correlation analyses were used to evaluate the relationship between heavy metal concentrations, resistomes and mobilomes. These results were then visualized with correlograms created using appropriate R packages. Treatment effects were assessed using non-parametric tests (e.g., Kruskal-Wallis, Wilcoxin Ranked Sum). Only differences where statistical tests had p-values <0.05 were stated as significant.
Network analysis of ARGs, MRGs, biocide resistance genes, and bacterial genera was conducted using ggraph in RStudio (RStudio Team, 2020). The network was based on Bray-Curtis similarity, with minimum requirements for edges set to P = 0.70. The minimum number of connections per node was set to 2, and nodes present in less than 50% of sample locations were excluded from the network. These parameters ensured significant edge connections.
To scale bacterial abundance to reads (RPKM), the Hellinger scaling method was applied using the decostand function in the “vegan” R package (Oksanen et al., 2022). Genera from phyla with no edges (P > 0.70) linked to resistance genes were removed from the network analysis.
3 Results and discussion
3.1 Metal removal and uptake
Total concentrations of each metal measured in wastewater varied over the growing season and the effect of each treatment varied across metals as well (Figure 2). Overall, there was a trend of metal removal throughout the growth season followed by a re-establishment of initial metal concentrations, likely due to biomass die-off and decomposition. Emerged macrophytes (FTW) treatment led to significantly (p < 0.05) greater Cu, Hg, Pb, As, and Cd removal than the other two treatment types, achieving an average metal removal on the final day (across all metal elements measured) of 59.49% (±7.45%) of initial concentration, as opposed to algae treatment [−117.02% (±69.53%)] and Lemna treatment [−32.51% (±8.31%)], and were the least affected by end of season metal release. Total metal concentration increased at the end of the growing season to levels higher than the initial concentration for mesocosms treated by algae. It is likely that this is due to the death and decomposition of the algae biomass releasing metals into the water column that were not initially suspended (Deng et al., 2016).
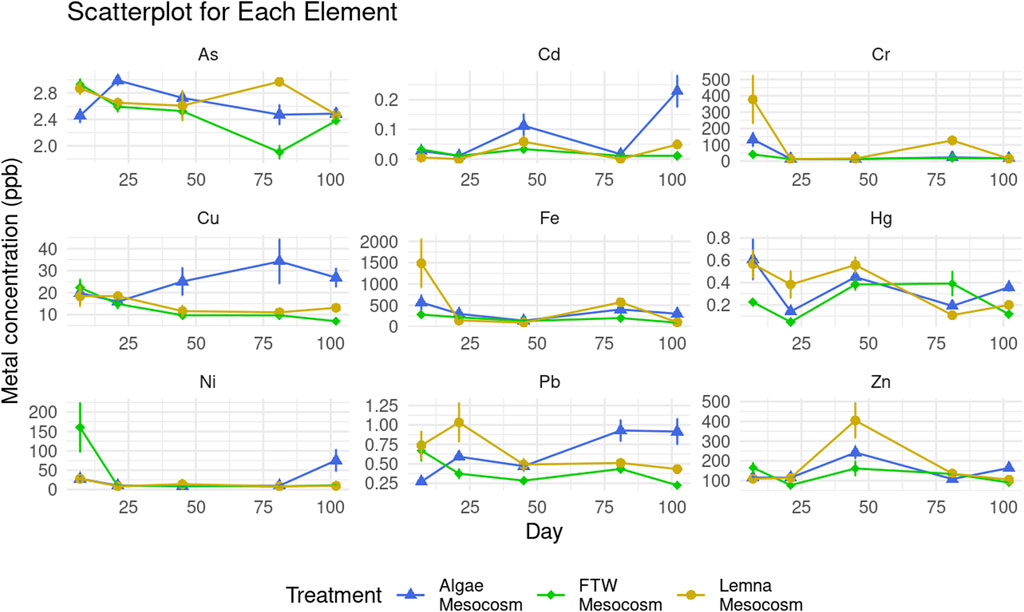
Figure 2. Metal concentration in wastewater per mesocosm treatment. Bars indicate standard deviation.
Lemna treatment was effective for certain elements (Cr, Cu, Fe, Ni, Pb, and Zn), even at the end of the growth season. It out-performed FTW treatment in iron (Fe) and chromium (Cr) removal, achieving 93.22% (±2.63%) and 95.97% (±1.59%), respectively. Metals were also measured in macrophyte root, shoot, and Lemna tissue at the end of the growth season (see Supplementary Figure S1). These results corroborate the findings of the water-metal analysis as greater concentrations of both iron and chromium were found in Lemna tissue (Fe = 2006.28 ± 229.77, Cr = 19.67 ± 1.16 mg/kg) than in macrophyte roots (Fe = 1574.11 ± 329.29 mg/kg, Cr = 8.86 ± 1.04 mg/kg) or shoots (Fe = 383.68 ± 53.97 mg/kg, Cr = 5.12 ± 3.18 mg/kg). With the exception of Hg, macrophyte roots accumulated greater concentrations of metals than the shoots.
3.2 Resistomes and mobilomes in lagoon system
The resistome and mobilomes within the microbial communities of the different samples are shown in Figure 3. A total of 138 different ARGs were found in the primary lagoon. ARGs encoding resistance to beta-lactams (47 genes, 25.1 rpkm), multidrug (24 genes, 30.5 rpkm) and aminoglycosides (22 genes, 6.78 rpkm) were the most abundant and diverse in this sample (Figure 3A). In terms of MRGs, chromium (Cr, 23.96 rpkm), multi-metals (11.1 rpkm), and mercury (Hg, 9.1 rpkm) resistance were the dominant in the primary lagoon sample (Figure 3B). The highest diversity and total levels of MGEs were also observed in the primary lagoon sample (981 genes, 75.5 rpkm), confirming the potential for the transmission of ARGs and MRGs, and the emergence of ARB in wastewater systems.
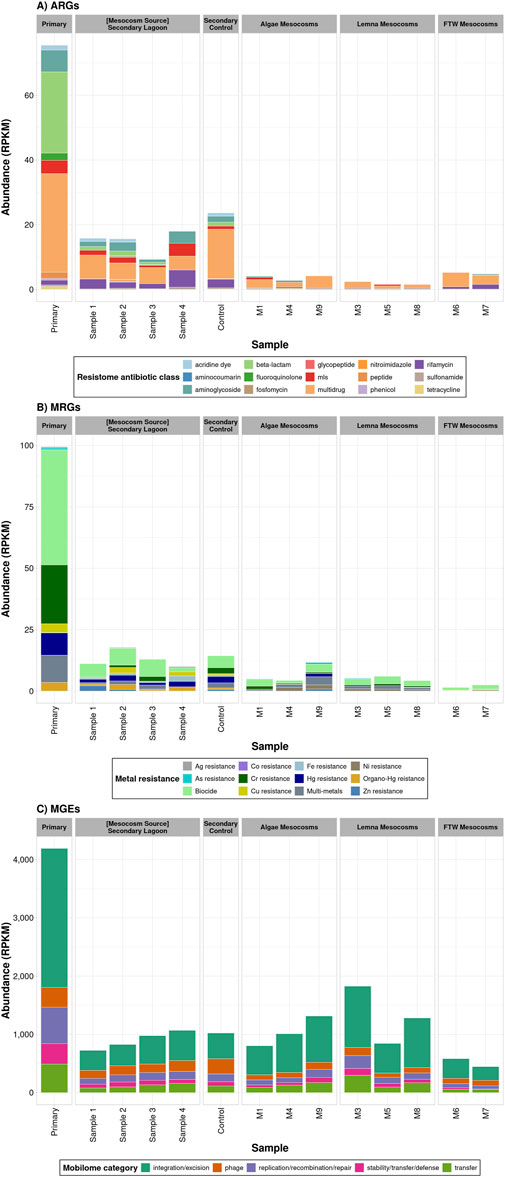
Figure 3. Resistome and mobilome profiles in each treatment. (A) Total levels of ARGs grouped by antibiotic class. (B) Total levels of MRGs grouped according to the type of resistant described in BacMet2. Biocide resistance includes genes which confer resistance to Quaternary Ammonium Compounds (QACs), Paraquat and diaquats, and Acids. These compounds were grouped generally as Biocides and treated separately from the metal specific MRGs throughout the manuscript. (C) Total levels of MGEs classified according to type of function listed in the mobileOG database.
Interestingly, both the diversity (100 genes) and the total levels of ARGs (14.7 rpkm) and MRGs were substantially lower in the secondary lagoon, suggesting that the biological activity and environmental conditions in the lagoon systems resulted in the reduction of these genes. The most abundant ARGs in this sample were multidrug (34 genes, 5.4 ± 1.2 rpkm), beta-lactams (25 genes, 1.1 ± 0.35 rpkm), and aminoglycosides (17 genes, 2.2 ± 1.2 rpkm). The total levels of MRGs in the secondary lagoon (8.1 ± 2.2 rpkm) were 86% lower than those in the primary lagoon (59.4 rpkm), although a total of 46 different genes were detected. In terms of MGEs, the mobilome was more diverse (1,299 genes) but showed less abundance (899 ± 153 rpkm) in the secondary lagoon (Figure 3C). Cumulatively, these results suggest the capability of the biological activity in this lagoon system to potentially reduce the risk of spreading antimicrobial resistance (AMR).
3.3 Effects of treatments on resistomes and mobilomes
The three biological treatments evaluated in this study reduced the diversity and total levels of ARGs (p = 0.05) and MRGs (p = 0.02) present in the secondary lagoon, which served as the wastewater source for the experiments. Mesocosms with floating macrophyte mats exhibited the lowest abundance of MRGs (p = 0.04) and MGEs (p = 0.04), while mesocosms treated with Lemna had the lowest abundance of ARGs (p = 0.10).
In the FTW mesocosms, only 12 distinct ARGs were detected, with total ARG levels (5.0 ± 0.33 rpkm) reduced to 34% of those in the secondary lagoon (Figure 2A). ARGs conferring resistance to aminoglycosides, MLS, and fluoroquinolones were the most reduced groups. This treatment also decreased the diversity of MRGs (9 genes) and their total abundance (1.0 ± 0.6 rpkm, p = 0.01) by 20% and 88%, respectively (Figure 2B). Specifically, MRGs encoding resistance to Ag, As, Zn, Hg, and Fe showed lower levels, while resistance to Cu and multi-metal elements increased (Figure 3B). A total of 578 MGEs were detected in the FTW Mesocosm, representing a 55% reduction compared to the source. Although total MGE levels were also reduced (515 ± 96.2 rpkm), the decrease was not statistically significant (p = 0.15, Figure 2C). MGEs related to transfer mechanisms and phages exhibited the highest reductions (Figure 3C). In contrast, the abundance of MGEs involved in integration and excision functions increased, suggesting the presence and proliferation of microbial groups carrying these MGEs in this treatment.
In the Lemna mesocosms, only 12 ARGs were detected, reflecting an 88.5% reduction from the secondary lagoon. Furthermore, total ARG levels decreased by 87.5%, with beta-lactam, aminoglycoside, and MLS ARGs being the most reduced groups (Figure 4A). The diversity of MRGs (9 genes) decreased by 80%, and their total abundance (4.2 ± 1.0 rpkm) was reduced by 48%, although this reduction was not statistically significant (p = 0.11). MRGs encoding resistance to Hg, Ag, As, Cu, Fe, and Zn were lower in abundance (Figure 4B). Fewer MGEs (677 genes) were detected compared to the secondary lagoon, though total MGE levels (1,318 ± 492 rpkm) tended to be higher. This increase, while not statistically significant, appeared to result from the enrichment of MGEs related to integration and excision (Figure 4C).
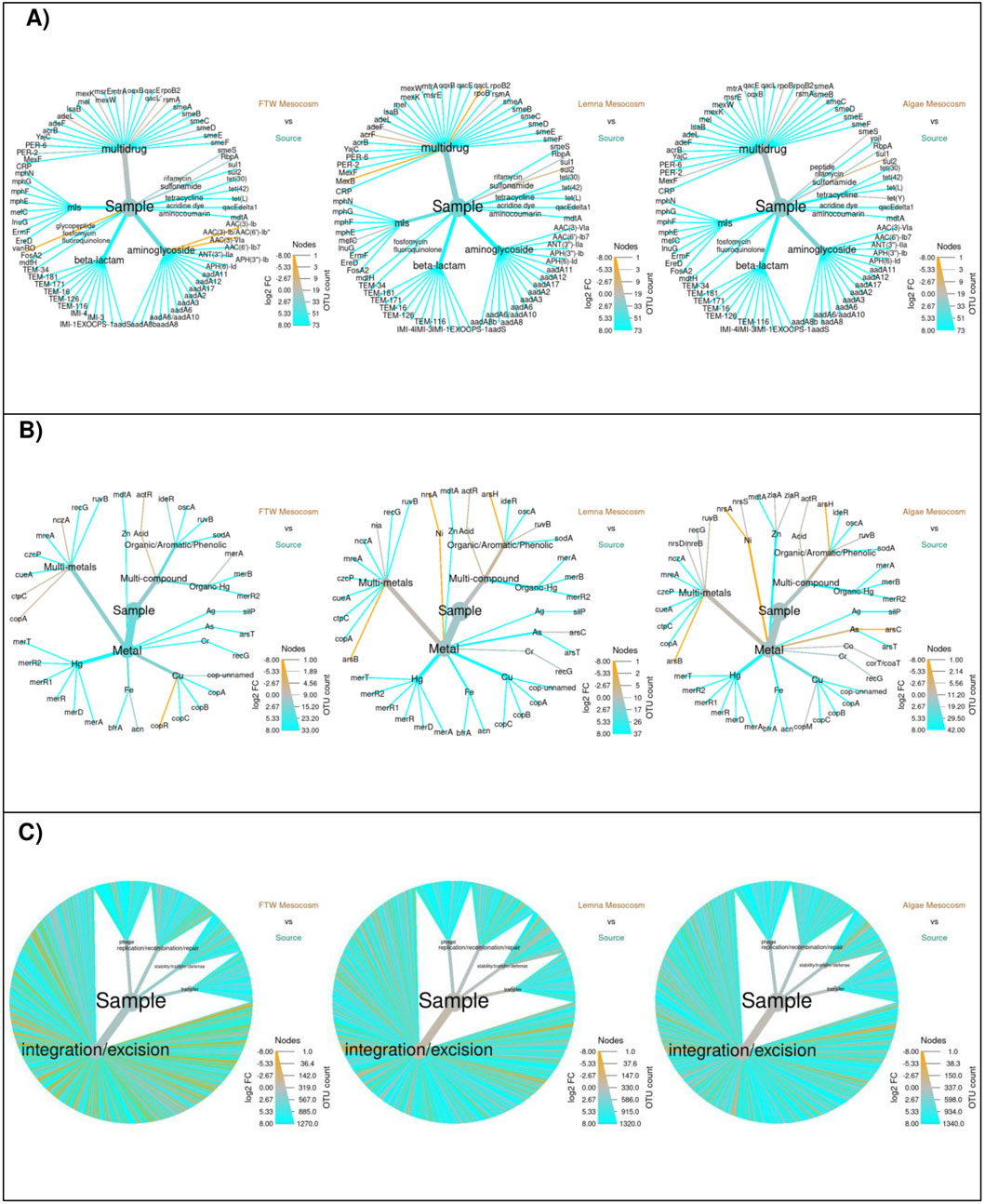
Figure 4. Differential heat trees of the changes in the total abundance of ARGs (A), MRGs (B), and MGEs (C) in the mesocosm treatments. The color encodes the log2 of the fold change (FC) defined as the total abundance in the secondary source influent divided by the total abundance after treatment. Blue (cyan) and orange indicate reduction and increases, respectively. Grey indicates no difference. Edge size indicates the number of ARGs in each group.
The Algae mesocosms had 20 detectable ARGs, reducing total levels by 70.5%. ARGs encoding resistance to MLS, beta-lactams, and aminoglycosides were found in lower concentrations (Figure 4A). For MRGs, 18 genes were detected, with total levels trending lower (6.18 ± 4.3 rpkm), although this was not statistically significant (p = 0.26). MGE diversity (756 genes) was reduced by 42%, but total MGE levels (1,043 ± 257 rpkm) showed a 14% increase, though this change was also not statistically significant (p = 0.545). Phage-associated MGEs and those involved in replication, recombination, and repair were generally reduced, while integration/excision MGEs showed enrichment (Figure 4C).
Overall, the results showed that the total levels of ARGs and MRGs in all three treatments were lower than those observed in the secondary lagoon control, suggesting that the treatments effectively reduced the risk of AMR transmission by decreasing the abundance of these resistance genes. While past research has shown the statistical comparability of metagenomics and qPCR (Flores-Orozco et al., 2022), further research should be conducted to validate and expand on these findings using qPCR and antibiotic susceptibility testing.
3.4 Microbial populations
The microbial communities at the phylum levels are shown in Figure 5, whereas the abundance of the top 40 most abundant genera is displayed in the Supplementary Figure S4. The most abundant phylum in the primary lagoon was Pseudomonadota (91.5%), which was mainly composed of the genera Aeromonas (68.5%), Pseudomonas (6.8%), Shewanella (4.6%), and Escherichia (1.9%). The microbiomes of the secondary lagoon samples were also dominated by the Pseudomonadota (61.2%), although Bacteroidota (13.7% ± 10.4%), Cyanobacteriota (8.9%), and Actinomycetota (8.5%) also represented a significant part of the community. At the genus level, Flavobacterium (11.3%) had the highest abundance, followed by Acidovorax (6.5%), Sphingopyxis (6.5% ± 6.0%), and Enterobacter (5.9%).
The microbial communities showed distinct shifts during the different treatments, especially an increase in Cyanobacteriota at the expense of Pseudomonadota. For example, the microbiome in the Algae mesocosms was characterized by high levels of the phylum Cyanobacteriota (58.2%), mainly composed of Cyanobium (23.9%), Nostoc (17.8%), and Trichormus (8.5%). Pseudomonadota was the second most abundant phylum, accounting for about 30.3% of the microbial communities, and Aeromonas (7.1%) and Delftia (3.7%) were the dominant genera in this phylum. In the Lemna mesocosms, the phyla Cyanobacteriota (67.9% ± 11.3%) and Pseudomonadota (23.7%) also dominated the microbial communities. However, in this treatment the genus Nostoc (35.3% ± 12.3%) was the most abundant, followed by Trichormus (16.9%) and Cyanobium (9.2%). On the other hand, Pseudomonadota (55.4%), Bacillota (16.4%), and Actinomycetota (15.3%) were the most abundant phyla in the FTW treatment samples. At the genus level, Delftia (13.5%), Cutibacterium (10.4%), and Staphyloccocus (7.9%) had the highest abundance in this treatment.
3.5 Correlation of MRGs and ARGs
Across all the metagenomic samples in this study a Spearman’s correlation between the abundance of ARGs and MRGs was r = 0.70 (including rhizome biofilm samples). Co-occurrence and co-selection of ARGs and MRGs have been found in many past studies (e.g., Li et al., 2016; Amarasekara et al., 2023; Li et al., 2022). There are three accepted models that explain ARG/MRG co-selection, which are co-resistance, cross-resistance, and co-regulation (Baker-Austin et al., 2006; Gillieatt and Coleman, 2024). Cross resistance refers to ARGs and MRGs that confer resistance to both antimicrobials and metals, co-resistance refers to ARGs and MRGs that share close genetic linkages, and co-regulation refers to resistance genes that share the same regulatory systems (Gillieatt and Coleman, 2024). Co-resistance may lead to resistance genes that are maintained in clusters as adjacent genetic elements (Li et al., 2016). The resulting co-residence or clustering of ARGs and MRGs on MGEs (such as integrons, transposons, and plasmids) allow for HGT and further explain the widespread co-occurrence observed across the microbial population (Amarasekara et al., 2023). In the current study, a significant positive correlation (r = 0.62, p < 0.05) was observed between MRGs and MGEs, suggesting that MRGs were to some extent present and carried on MGEs. There was strong correlation between specific ARGs and MRGs-most notably: Fe resistance genes and antibiotic target replacement (r = 0.90, p < 0.05) and antibiotic target alteration (r = 0.86, p < 0.05) genes; Hg resistance genes and antibiotic efflux pumps genes (r = 0.89, p < 0.05); and Organic-mercury (Hg) resistance genes and antibiotic inactivation (r = 0.89, p < 0.05), antibiotic target replacement, (r = 0.90, p < 0.05) and antibiotic target alteration genes (r = 0.91, p < 0.05).
Past research has shown specific correlation of the presence of certain metal class MRGs and antimicrobial class ARGs. These include the co-occurrence of zinc (Zn) resistance with beta-lactam resistance, copper (Cu) resistance with beta-lactam resistance, and arsenic (As) resistance with beta-lactam resistance (Li et al., 2016). Figure 6 presents correlations between specific metal compound resistance and ARG abundance categorized by molecular mechanism. In this study, Cu resistance was significantly correlated with antibiotic inactivation (Figure 6), the main mechanisms by which beta-lactams act, providing more evidence for the co-occurrence of Cu resistance and beta-lactam resistance.
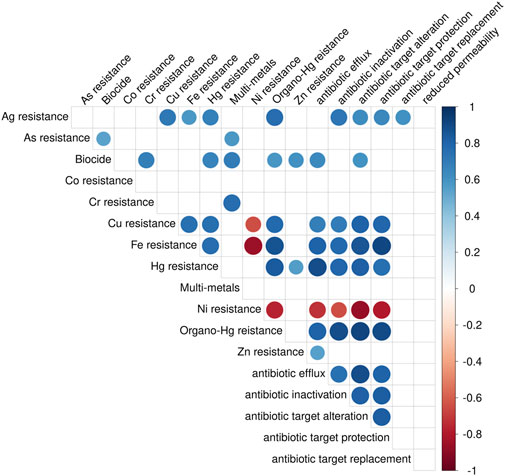
Figure 6. Correlogram of the total abundance (rpkm) of metal resistance and ARG mechanisms. Only correlations with a p < 0.05 from Spearman’s correlation analyses are shown. Correlation coefficients are represented by color and size of the circles.
In the present study, there was a strong correlation between Cu resistance and aminoglycoside resistance (r = 0.76), organo-mercury resistance and aminoglycoside resistance (r = 0.95), mercury resistance and aminoglycoside resistance (r = 0.81), and iron resistance and aminoglycoside resistance (r = 0.79). Beta-lactam resistance was correlated to mercury (r = 0.91) and organo-mercury resistance (r = 0.80). Multi drug resistance was found to be correlated to iron (r = 0.89), mercury (r = 0.85), and organo-mercury resistance (r = 0.79). Additionally, across all samples, iron resistance had a strong correlation to rifamycin resistance (r = 0.96). A strong correlation existed between iron resistance and antibiotic target protection (r = 0.87), and antibiotic target replacement (r = 0.86) (Figure 6). Specific gene correlations exist between iron resistant genes acn and brfA, and antibiotic inactivation genes conferring resistance to aminoglycosides (aaDA12, aaDA17, aaDA2) and macrolides (mphE, mphG). Mercury resistance genes merD, merT, and merR also had strong correlation to antibiotic inactivation genes aaDA12, aaDA17, aaDA2, aaDA3, aaDs, mphE, mphF, mphG, EreD.
3.6 Heavy metals and resistomes
Corelograms were used to visualize Spearman’s rank correlation of heavy metal concnetration in wastewater and resistance gene abundance. Figure 7 shows the correlation of the 6 different heavy metals measured throughout the study to the abundance of total ARGs, MRGs, and MGEs.
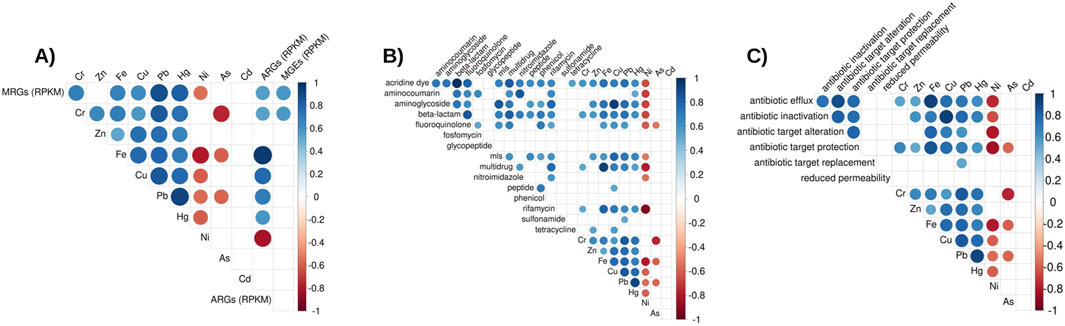
Figure 7. Correlograms: (A) Total abundance of ARGs and MRGs (rpkm) to metal concentration. (B) Total abundance of ARGs by antibiotic class (rpkm) to metal concentration. (C) Total abundance of ARGs by mechanism (rpkm) to metal concentration. Only significant (p < 0.05) Spearman’s correlations are shown. Correlation coefficients are represented by color and size of the circles.
Strikingly, a strong and statistically significant correlation (r = 0.95, p < 0.05) between total ARG abundance and Fe concentration was observed (Figure 6A). This high correlation suggests Fe concentration could influence the presence of ARGs in the microbial communities in these types of environments. Unlike other measured metals, such as Cu, As, Cr, and Hg, Fe does not have a high level of toxicity and is generally innocuous at environmental concentrations. Iron has been found to cause both increased antibiotic resistance and susceptibility in lab experiments, depending on the bacteria and antibiotic (Ezraty and Frédéric, 2016).
Iron plays a role in certain processes related to antimicrobial mechanisms such as efflux pumps, respiration and Fenton process-dependent biocidal activity. Choi et al. (2022) found that Fe supplementation promoted antibiotic resistance in Streptomyces coelicolor and Mycobacterium smegmatis by upregulating genes involved in metabolism and respiration, even under anaerobic conditions. This resistance involves a potassium cyanide-insensitive respiration type, suggesting a strategy that helps bacteria avoid oxidative damage from bactericidal antibiotics. To the best of our knowledge, the present study is the first to look at the effect of Fe concentration on ARGs in an environmental setup. The strength of the correlation suggests that another effect, beyond the previously discussed mechanism, may be responsible for the observed increase in ARG abundance in the presence of higher Fe concentrations. Rather than Fe directly influencing the expression of ARGs, it may promote the growth of bacteria genera and species more likely to harbor and procure resistance, such as certain facultative Pseudomonadota bacteria (further discussed below). Further studies are needed to understand the molecular and evolutionary mechanisms by which Fe influences ARGs.
3.7 Relationships between microorganism, ARGs, MRGs and MGEs
Of all the phyla identified in the study, Pseudomonadota was the most strongly correlated with ARGs (r = 0.77, p < 0.05), followed by campylobacteria (r = 0.66, p < 0.05). To further investigate the relationship between bacteria and ARGs, a network analysis was performed (Figure 8).
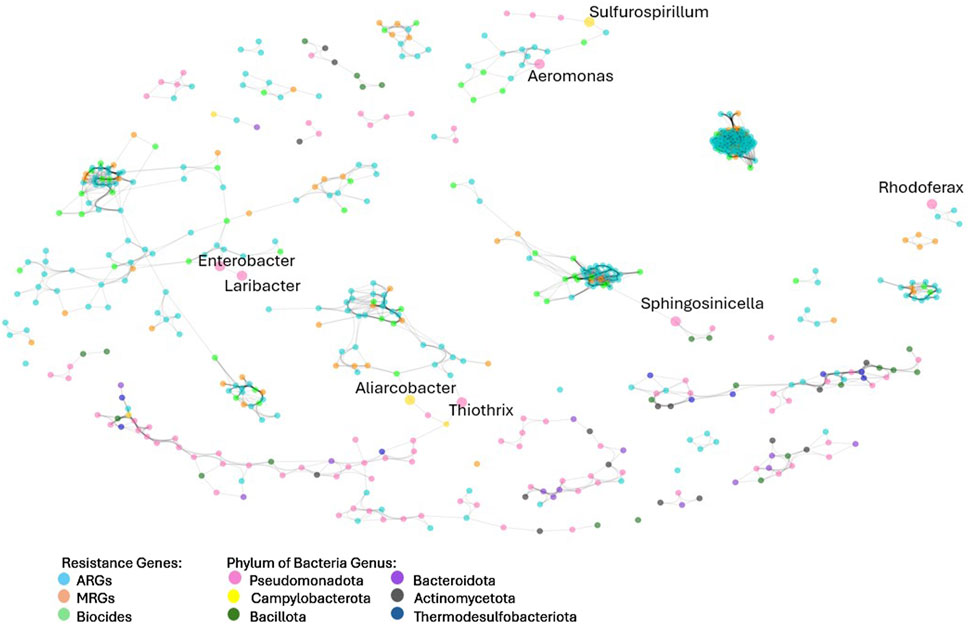
Figure 8. Network analysis of resistance genes and microbial groups at the genus levels. Network analysis included data from all samples in study. Colors indicated the type of resistance encoded by resistance genes and microbial genus.
There was a close relationship between clusters of ARGs, MRGs and biocides and labelled bacteria genus (Figure 8). Tightly clustered groupings of resistance genes represent genes that were similar (P > 0.70) in presence and abundance across the different samples, indicating that they were related, possibly through chromosomal proximity on MGEs or through presence in certain bacterial species. Bacteria genera in the network analysis that are connected to resistance clusters are also similar in presence and abundance across the different samples, suggesting that they could harbour the resistance genes with which they are clustered. It is noteworthy that the majority of labelled bacteria (those with the strongest similarity to resistome abundance) were genera belonging to Pseudomonadota, followed exclusively by Campylobacteria. These results concur with the relationship identified through Spearman’s rank correlation, despite using different analysis methods (Bray-Curtis’s similarity).
Of the eight genera identified through the network analysis to be clustered with resistance genes, four (Aeromonas, Enterobacter, Laribacter, and Rhodoferax) were facultative Pseudomonadota. Aeromonas, Enterobacter, and Laribacter contain pathogenic bacterial species often associated with resistance, such as A. hydrophila and A. salmonicida (Pintor-Cora et al., 2023), and L. hongkongensis (Lau et al., 2011). Aliarcobacter, belonging to the phylum Campylobacteria, is known to harbour metal and antibiotic resistance genes (Müller et al., 2020). Sulfurosprillum spp., also belonging to the Campylobacteria phylum, are known to reduce sulfur and metal species such as arsenate, and for living in heavy metal-rich environments (Goris and Diekert, 2016).
Neighbour (direct node connections) and next of neighbour (secondary node connections) for each of the eight bacterial connections were identified. These genes represent the resistance genes most similar in abundance and occurrence to each genus. Aeromonas had five neighbours (CEPH-A3, OXA-12, cph-A6, OXA-724, cph-A4) and one next of neighbour gene (cph-A7) belonging to the beta-lactam resistance class. Aeromonas is known to commonly harbour resistance to beta-lactam antimicrobials, including cefepime and other extended-spectrum cephalosporins (Aravena-Román et al., 2012). Six out of 10 neighbour and next of neighbour resistance genes clustered with Aeromonas from the network analysis were beta-lactam ARGs and four of these six confer resistance to cephalosporins.
The congruence between the findings from the present study and known Aeromonas resistance in literature provides an indication of validation for the network analysis. Both Laribacter and Enterobacter bacteria were clustered with a high percentage (6 out of 6 and 5 out of 8, respectively) efflux pump ARGs and MRGs. L. hongkongensis has been found to harbour efflux pump resistance genes, including Acr eflux system genes (Lau et al., 2011), which were present in both Laribacter and Enterobacter resistance clusters. Aliarcobacter was directly connected to only one resistance gene, fetB/ybbM, an MRG conferring resistance to Fe, while its next of neighbours included other MRGs conferring resistance to As (pstB) and to multi-metals (czcA). Aliarcobactor’s next of neighbours also included beta-lactam resistance genes.
Given the strong correlation between Fe concentration and ARGs across the wastewater samples, the relationship between the eight genera connected to resistance clusters identified from the network analysis and Fe was further explored (Table 1).
The three genera most strongly correlated with ARGs (Enterobacter, Laribacter, and Aliarcobacter) were also the three genera most strongly correlated with Fe resistance and Fe concentration (Table 1). The majority of the genera identified by the network analysis were facultative or aerotolerant bacteria that can utilize Fe in their respiration systems. The strong correlation of Fe and ARGs across the wastewater samples (r = 0.95, p < 0.05) and the association of facultative, Fe reducing bacteria with ARGs in this study suggests that Fe promotes the proliferation of Pseudomonadota and Campylobacteria harboring ARGs.
Metal ions are used in anaerobic respiration employed by facultative bacteria. Fe3+ and Mn4+ can be used as alternate electron acceptors (Fenchel et al., 2012). Respiratory Fe reduction has been found to play significant roles in anaerobic carbon transformations in freshwater sediments (Fenchel et al., 2012). Iron reduction [Fe(III) to Fe(II)] pathways have been identified in bacterial species belonging to Aeromonas, Enterobacter, Laribacter and Rhodoferax genera (Table 1).
Sulfur metabolization in sulfur-reducing bacteria is also associated with anaerobic respiration. Sulfur-metabolizing genes (SMGs) were identified utilizing the Sulfur Metabolizing Data Base (SMDB) and were found to be correlated with ARGs (r = 0.63, p < 0.05), and Pseudomonadota (r = 0.76, p < 0.05) and Campylobacteria (r = 0.87, p < 0.05). Additionally, correlations between MRGs (r = 0.51, p < 0.05), and Fe resistance (r = 0.75, p < 0.05), Fe concentration (r = 0.78, p < 0.05), and total metal concentration (of measured metals) (r = 0.83, p < 0.05) exist with sulfur-metabolizing genes. Reduction of ferric iron (Fe3+) and sulfate (SO42−) are the most common electron-accepting processes for anaerobic respiration in anoxic freshwater environments (Berg et al., 2019). Iron also plays a role in Fe(III)-driven sulfide oxidation (Berg et al., 2019). It is therefore probable that Fe provides advantages to each genus identified in Table 1, especially in anoxic or low oxygen environments.
A relationship between facultative and aerotolerant bacteria, and ARGs is strongly suggested by the results of this study. Facultative bacteria have been shown to play a role in ARG dissemination in municipal and livestock wastewater (Hembach et al., 2019; Flores-Orozco et al., 2024). and are overrepresented on the WHO antibiotic-resistant Priority Pathogens list, accounting for 8 of 12 listed pathogens (André et al., 2021).
The use of phytoremediation systems such as emerged floating macrophytes and Lemna corrals may therefore reduce ARGs in wastewater through the removal of metals, including Fe, limiting facultative bacterial growth that are dependent on electron acceptors for anaerobic respiration.
The molecular and evolutionary mechanisms that may explain why facultative bacteria seem more likely to harbor higher-level ARGs than other bacteria remain unknown. Further genomic investigation is recommended to study this relationship.
3.8 Practical implementation
The results of the present study suggest that phytoextraction of metals in wastewater could reduce the abundance of MRGs and ARGs in wastewater effluents. This is of importance to wastewater treatment lagoon and plant operators as phytoremediation systems such as FTWs or Lemna treatment corrals, provide an affordable and practical augmentative treatment to improve lagoon performance (McCorquodale-Bauer et al., 2023). Additionally, the results are relevant beyond the scope of phytoremediation systems, as metal removal through more traditional constructed wetlands or other treatment systems may result in a decrease in MRG and ARG abundance. More research is needed to understand how FTWs would affect metals, MRGs and ARGs at a full-scale level.
4 Conclusion
Each biological treatment system tested in the mesocosms affected microbial diversity and led to a decrease in the total abundance of ARGs and MRGs from the original secondary sewage influent. FTWs, algae and Lemna treatment systems reduced total ARG abundance by 34%, 70.5%, and 88.5%, respectively. Significant correlation between MRGs and ARGs (r = 0.75), and MGEs (r = 0.75) where found. A network analysis revealed strong clustering of ARGs, MRGs and biocide resistance. Through the network analysis, it was found that facultative Pseudomonadota were over-represented in bacteria connected to resistance clusters. Iron concentration was strongly correlated with ARG abundance (r = 0.95, p < 0.05) and evidence presented in Table 1 suggests that Fe facilitates the growth and proliferation of these bacteria. Iron reduction was identified as a non-aerobic respiration pathway in 5 of the 8 genera identified from the resistance network analysis. The presence of Fe may promote the growth of facultative Pseudomonadota and certain Campylobacteria that appear more likely to harbor resistance genes. Therefore, metal phytoextraction from treatments such as FTWs and Lemna may slow the growth of these bacteria and indirectly reduce the abundance of resistance genes.
Data availability statement
The datasets presented in this study can be found in online repositories. The names of the repository/repositories and accession number(s) can be found below: https://www.ncbi.nlm.nih.gov/, PRJNA1031688.
Author contributions
KM-B: Data curation, Formal Analysis, Methodology, Software, Visualization, Writing–original draft, Writing–review and editing, Investigation. DF: Visualization, Writing–review and editing, Data curation, Formal Analysis, Methodology, Writing–original draft. RG: Conceptualization, Writing–review and editing. FZ: Conceptualization, Writing–review and editing. NC: Conceptualization, Funding acquisition, Resources, Supervision, Writing–review and editing.
Funding
The author(s) declare that financial support was received for the research, authorship, and/or publication of this article. The authors declare that they have received fundings, and that this work was possible thanks to Mitacs Accelerate and the International Institute for Sustainable Development (Grant Number IT18386).
Acknowledgments
We would like to thank Mitacs Accelerate and the International Institute for Sustainable Development for supporting this work. We are also grateful to Dr. Mark Hanson for providing direction and insight into the experimental design of this project.
Conflict of interest
The authors declare that the research was conducted in the absence of any commercial or financial relationships that could be construed as a potential conflict of interest.
Generative AI statement
The author(s) declare that no Generative AI was used in the creation of this manuscript.
Publisher’s note
All claims expressed in this article are solely those of the authors and do not necessarily represent those of their affiliated organizations, or those of the publisher, the editors and the reviewers. Any product that may be evaluated in this article, or claim that may be made by its manufacturer, is not guaranteed or endorsed by the publisher.
Supplementary material
The Supplementary Material for this article can be found online at: https://www.frontiersin.org/articles/10.3389/fsybi.2024.1513580/full#supplementary-material
References
Alcock, B. P., Raphenya, A. R., Lau, T. T. Y., Tsang, K. K., Bouchard, M., Edalatmand, A., et al. (2020). CARD 2020: antibiotic resistome surveillance with the comprehensive antibiotic resistance database. Nucleic Acids Res. 48 (D1), D517-D525–25. doi:10.1093/nar/gkz935
Amarasekara, N. R., Mafiz, A. I., Qian, X., Tiedje, J. M., Hao, W., and Zhang, Y. (2023). Exploring the Co-occurrence of antibiotic, metal, and biocide resistance genes in the urban agricultural environment. J. Agric. Food Res. 11, 100474. doi:10.1016/j.jafr.2022.100474
André, A. C., Debande, L., and Marteyn, B. S. (2021). The selective advantage of facultative anaerobes relies on their unique ability to cope with changing oxygen levels during infection. Cell. Microbiol. 23 (8), e13338. doi:10.1111/cmi.13338
Aravena-Román, M., Inglis, T. J. J., Henderson, B., Riley, T. V., and Chang, B. J. (2012). Antimicrobial susceptibilities of Aeromonas strains isolated from clinical and environmental sources to 26 antimicrobial agents. Antimicrob. Agents Chemother. 56 (2), 1110–1112. doi:10.1128/AAC.05387-11
Baker-Austin, C., Wright, M. S., Stepanauskas, R., and McArthur, J. V. (2006). Co-selection of antibiotic and metal resistance. Trends Microbiol. 14 (4), 176–182. doi:10.1016/j.tim.2006.02.006
Berg, J. S., Jézéquel, D., Duverger, A., Lamy, D., Laberty-Robert, C., and Miot, J. (2019). Microbial diversity involved in iron and cryptic sulfur cycling in the ferruginous, low-sulfate waters of Lake pavin. PloS One 14 (2), e0212787. doi:10.1371/journal.pone.0212787
Blandford, N. C., McCorquodale-Bauer, K., Grosshans, R., Hardy, B., Cicek, N., and Vince, P. (2024). Removal of nutrients from aquaculture wastewater using cattail (Typha spp.) constructed wetlands. ’ J. Environ. Qual. 53 (August), 767–775. doi:10.1002/jeq2.20608
Bolger, A. M., Lohse, M., and Usadel, B. (2014). Trimmomatic: a flexible trimmer for Illumina sequence data. Bioinforma. Oxf. Engl. 30 (15), 2114–2120. doi:10.1093/bioinformatics/btu170
Brown, C. L., Mullet, J., Hindi, F., Stoll, J. E., Gupta, S., Choi, M., et al. (2022). MobileOG-Db: a manually curated database of protein families mediating the life cycle of bacterial mobile genetic elements. Appl. Environ. Microbiol. 88 (18), e0099122–22. doi:10.1128/aem.00991-22
Buchfink, B., Xie, C., and Huson, D. H. (2015). Fast and sensitive protein alignment using DIAMOND. Nat. Methods 12 (1), 59–60. doi:10.1038/nmeth.3176
Chaudhary, S., Singh Sindhu, S., Dhanker, R., and Kumari, A. (2023). Microbes-mediated sulphur cycling in Soil: impact on Soil fertility, crop production and environmental sustainability. Microbiol. Res. 271, 127340. doi:10.1016/j.micres.2023.127340
Choi, J.-S., Seok, Y.-J., Cho, Y.-H., and Roe, J.-H. (2022). Iron-induced respiration promotes antibiotic resistance in actinomycete bacteria. MBio 13 (2), e0042522–22. doi:10.1128/mbio.00425-22
Deng, H., Zhang, J., Chen, S., Yang, L., Wang, D., and Yu, S. (2016). Metal release/accumulation during the decomposition of potamogeton crispus in a shallow macrophytic lake. J. Environ. Sci. 42, 71–78. doi:10.1016/j.jes.2015.07.004
Edet, U. O., Bassey, I. U., and Joseph, A. P. (2023). Heavy metal Co-resistance with antibiotics amongst bacteria isolates from an open dumpsite Soil. Heliyon 9 (2), e13457. doi:10.1016/j.heliyon.2023.e13457
Ezraty, B., and Frédéric, B. (2016). The “liaisons dangereuses” between iron and antibiotics. FEMS Microbiol. Rev. 40 (3), 418–435. doi:10.1093/femsre/fuw004
T. G. Fenchel, G. M. King, and T. H Blackburn (2012). “Chapter 7 - Aquatic Sediments,” Bacterial Biogeochemistry. 3rd Edn (Boston: Academic Press), 121–42. doi:10.1016/B978-0-12-415836-8.00007-4
Flores-Orozco, D., Levin, D., Kumar, A., Sparling, R., and Cicek, N. (2022). A meta-analysis reveals that operational parameters influence levels of antibiotic resistance genes during anaerobic digestion of animal manures. Sci. Total Environ. 814, 152711. doi:10.1016/j.scitotenv.2021.152711
Flores-Orozco, D., Levin, D., Kumar, A., Sparling, R., Derakhshani, H., and Cicek, N. (2024). Metagenomic comparison of effects of mesophilic and thermophilic manure anaerobic digestion on antimicrobial resistance genes and mobile genetic elements. Environ. Adv. 15, 100472. doi:10.1016/j.envadv.2023.100472
Foster, Z. S. L., Sharpton, T. J., and Grünwald, N. J. (2017). Metacoder: an R package for visualization and manipulation of community taxonomic diversity data. PLoS Comput. Biol. 13 (2), e1005404. doi:10.1371/journal.pcbi.1005404
Gillieatt, B. F., and Coleman, N. V. (2024). Unravelling the mechanisms of antibiotic and heavy metal resistance Co-selection in environmental bacteria. FEMS Microbiol. Rev. 48 (4), fuae017. doi:10.1093/femsre/fuae017
Goris, T., and Diekert, G. (2016). “The genus sulfurospirillum,” in Organohalide-respiring bacteria. Editors L. Adrian, and F. E. Löffler (Berlin, Heidelberg: Springer Berlin Heidelberg), 209–234. doi:10.1007/978-3-662-49875-0_10
Hembach, N., Alexander, J., Hiller, C., Wieland, A., and Schwartz, T. (2019). Dissemination prevention of antibiotic resistant and facultative pathogenic bacteria by ultrafiltration and ozone treatment at an urban wastewater treatment plant. Sci. Rep. 9 (1), 12843. doi:10.1038/s41598-019-49263-1
Jampani, M., Mateo-Sagasta, J., Chandrasekar, A., Fatta-Kassinos, D., Graham, D. W., Gothwal, R., et al. (2024). Fate and transport modelling for evaluating antibiotic resistance in aquatic environments: current knowledge and research priorities. J. Hazard. Mater. 461, 132527. doi:10.1016/j.jhazmat.2023.132527
Kato, S., and Ohkuma, M. (2021). A single bacterium capable of oxidation and reduction of iron at circumneutral PH. Microbiol. Spectr. 9 (1), e0016121. doi:10.1128/spectrum.00161-21
Kooli, W. M., Junier, T., Shakya, M., Monachon, M., Davenport, K. W., Vaideeswaran, K., et al. (2019). Remedial treatment of corroded iron objects by environmental Aeromonas isolates. Appl. Environ. Microbiol. 85 (3), 020422-e2118. doi:10.1128/AEM.02042-18
Larsson, D. G. J., and Flach, C.-F. (2022). Antibiotic resistance in the environment. Nat. Rev. Microbiol. 20 (5), 257–269. doi:10.1038/s41579-021-00649-x
Lau, S.Kp, Wong, G.Km, Tsang, A.Kl, Teng, J.Ll, Fan, R.Yy, Tse, H., et al. (2011). Virulence determinants, drug resistance and mobile genetic elements of laribacter hongkongensis: a genome-wide analysis. Cell and Biosci. 1 (1), 17. doi:10.1186/2045-3701-1-17
Li, D., Liu, C.-M., Luo, R., Sadakane, K., and Lam, T.-W. (2015). MEGAHIT: An Ultra-Fast Single-Node Solution for Large and Complex Metagenomics Assembly via Succinct de Bruijn Graph. Bioinforma. Oxf. Engl. 31 (10), 1674–1676. doi:10.1093/bioinformatics/btv033
Li, L.-G., Xia, Yu, and Zhang, T. (2016). Co-occurrence of antibiotic and metal resistance genes revealed in complete Genome collection. ISME J. 11 (3), 651–662. doi:10.1038/ismej.2016.155
Li, X., Rensing, C., Vestergaard, G., Arumugam, M., Nesme, J., Gupta, S., et al. 2022. Metagenomic evidence for Co-occurrence of antibiotic, biocide and metal resistance genes in pigs. Environ. Int. 158, 106899. doi:10.1016/j.envint.2021.106899
Liu, X., Guo, X., Liu, Y., Lu, S., Xi, B., Zhang, J., et al. (2019). A review on removing antibiotics and antibiotic resistance genes from wastewater by constructed wetlands: performance and microbial response. Environ. Pollut. (Barking, Essex 1987) 254 (Pt A) 254, 112996. doi:10.1016/j.envpol.2019.112996
Lu, J., Breitwieser, F. P., Thielen, P., and Salzberg, S. L. (2017). Bracken: estimating species abundance in metagenomics data. PeerJ Comput. Sci. 3, e104. doi:10.7717/peerj-cs.104
Mandal, R. R., Bashir, Z., Mandal, J. R., and Raj, D. (2024). Potential strategies for phytoremediation of heavy metals from wastewater with circular bioeconomy approach. Environ. Monit. Assess. 196 (6), 502. doi:10.1007/s10661-024-12680-5
McCorquodale-Bauer, K., Grosshans, R., Zvomuya, F., and Cicek, N. (2023). Critical review of phytoremediation for the removal of antibiotics and antibiotic resistance genes in wastewater. Sci. Total Environ. 870:161876. doi:10.1016/j.scitotenv.2023.161876
Mo, S., Yan, B., Gao, T., Li, J., Kashif, M., Song, J., et al. (2023). Sulfur metabolism in subtropical marine mangrove sediments fundamentally differs from other habitats as revealed by SMDB. Sci. Rep. 13 (1), 8126. doi:10.1038/s41598-023-34995-y
Mosaka, T. B. M., Unuofin, J. O., Daramola, M. O., Tizaoui, C., and Iwarere, S. A. (2022). Inactivation of antibiotic-resistant bacteria and antibiotic-resistance genes in wastewater streams: current challenges and future perspectives. Front. Microbiol. 13, 1100102. doi:10.3389/fmicb.2022.1100102
Müller, E., Abdel-Glil, M. Y., Hotzel, H., Hänel, I., and Herbert, T. (2020). Aliarcobacter butzleri from water poultry: insights into antimicrobial resistance, virulence and heavy metal resistance. Genes 11 (9), 1104. doi:10.3390/genes11091104
Murray, L. M., Hayes, A., Snape, J., Kasprzyk-Hordern, B., Gaze, W. H., and Murray, A. K. (2024). Co-Selection for Antibiotic Resistance by Environmental Contaminants. npj antimicrob. resist. 2 (1). doi:10.1038/s44259-024-00026-7
Oksanen, J., Simpson, G., Guillaume Blanchet, F., Kindt, R., Legendre, P., Minchin, P., et al. (2022). Vegan community ecology package version 2.6-2 april 2022.
Pal, C., Bengtsson-Palme, J., Rensing, C., Kristiansson, E., and Larsson, D. G. J. (2014). BacMet: antibacterial biocide and metal resistance genes database. Nucleic Acids Res. 42 (Database issue), D737–D743. doi:10.1093/nar/gkt1252
Palarea-Albaladejo, J., and Antoni Martín-Fernández, J. (2015). ZCompositions — R package for multivariate imputation of left-censored data under a compositional approach. Chemom. Intelligent Laboratory Syst. 143, 85–96. doi:10.1016/j.chemolab.2015.02.019
Pintor-Cora, A., Tapia, O., Elexpuru-Zabaleta, M., Ruiz de Alegría, C., Rodríguez-Calleja, J. M., Santos, J. A., et al. (2023). Cytotoxicity and antimicrobial resistance of Aeromonas strains isolated from fresh produce and irrigation water. Antibiot. (Basel, Switz.) 12 (3), 511. doi:10.3390/antibiotics12030511
RStudio Team (2020). RStudio. Boston, MA: Integrated Development for R. RStudio, PBC. Available at: http://www.rstudio.com/.
Sambaza, S. S., and Naicker, N. (2023). Contribution of wastewater to antimicrobial resistance: a review article. ’ J. Glob. Antimicrob. Resist. 34 (September), 23–29. doi:10.1016/j.jgar.2023.05.010
Sarma, U., Hoque, Md E., Thekkangil, A., Venkatarayappa, N., and Rajagopal, S. (2024). Microalgae in removing heavy metals from wastewater – an advanced green technology for urban wastewater treatment. J. Hazard. Mater. Adv. 15, 100444. doi:10.1016/j.hazadv.2024.100444
Straub, K. L., and Schink, B. (2004). Ferrihydrite-dependent growth of sulfurospirillum deleyianum through electron transfer via sulfur cycling. Appl. Environ. Microbiol. 70 (10), 5744–5749. doi:10.1128/AEM.70.10.5744-5749.2004
Sutradhar, I., Kalyan, P., Chukwu, K., Abia, A. L. K., Mbanga, J., Essack, S., et al. (2023). Metal ions and their effects on antimicrobial resistance development in wastewater. BioRxiv, 2023.06.16.545339. doi:10.1101/2023.06.16.545339
Wang, D., Jin, C., Jin, A., and Lou, Z. (2020). Characterization of Fe(III)-Reducing enrichment cultures and isolation of Enterobacter sp. Nan-1 from the deep-sea sediment, south China sea. J. Ocean Univ. China 19 (4), 818–826. doi:10.1007/s11802-020-4190-1
WHO, 2023. Antibiotic resistance [WWW document]. World Health Organization, Available at: https://www.who.int/news-room/fact-sheets/detail/antibiotic-resistance. (Accessed 14 February 2024). 252.
Wibowo, G., Andhyka Tyaz Nugraha, Y., and Rohman, A. (2023). Phytoremediation of several wastewater sources using Pistia stratiotes and Eichhornia crassipes in Indonesia. Environ. Nanotechnol. Monit. and Manag. 20, 100781. doi:10.1016/j.enmm.2023.100781
Wickham, H. (2016). Ggplot2: elegant graphics for data analysis. New York: Springer-Verlag. Available at: https://ggplot2.tidyverse.org.
Keywords: heavy metals, bioremediation, antibiotics, metagenomics, bioinformatics, iron, facultative bacteria, constructed wetlands
Citation: McCorquodale-Bauer K, Flores Orozco D, Grosshans R, Zvomuya F and Cicek N (2025) Reduction of antimicrobial resistance genes in wastewater through phytoremediation. Front. Synth. Biol. 2:1513580. doi: 10.3389/fsybi.2024.1513580
Received: 18 October 2024; Accepted: 16 December 2024;
Published: 07 January 2025.
Edited by:
Simona Di Gregorio, University of Pisa, ItalyReviewed by:
Alicia Sanchez-Gorostiaga, Instituto Madrileño de Investigación y Desarrollo Rural, Agrario y Alimentario, SpainJean Damascene Harindintwali, Chinese Academy of Sciences (CAS), China
Copyright © 2025 McCorquodale-Bauer, Flores Orozco, Grosshans, Zvomuya and Cicek. This is an open-access article distributed under the terms of the Creative Commons Attribution License (CC BY). The use, distribution or reproduction in other forums is permitted, provided the original author(s) and the copyright owner(s) are credited and that the original publication in this journal is cited, in accordance with accepted academic practice. No use, distribution or reproduction is permitted which does not comply with these terms.
*Correspondence: Kenton McCorquodale-Bauer, bWNjb3JxdWtAbXl1bWFuaXRvYmEuY2E=