- 1Department of Biosystems Engineering, University of Manitoba, Winnipeg, Canada
- 2Department of Microbiology, University of Manitoba, Winnipeg, Canada
There is a growing concern about the potential dissemination of antimicrobial resistance into agricultural fields due to the application of manure as crop fertilizer. While reducing the usage of antibiotics in livestock production stands as the first alternative to prevent this problem, there is evidence that this may not be enough to eliminate antimicrobial resistance elements already present in manure microbiomes. This study employed a metagenomic approach to investigate the impact of common manure treatments, including aerobic storage, mesophilic anaerobic digestion (MAD), and solid-liquid separation, on the presence and abundance of antimicrobial resistance genes (ARGs), bactericides, and heavy metal resistance genes (BacMet), and mobile genetic elements (MGEs) in manure from three different farms, including one operating in an antibiotic-free environment. The results indicated that MAD was the best method to reduce the numbers of ARGs, BacMet, and MGEs, achieving reduction rates greater than 40%, 89%, and 68%, respectively. Manure storage significantly reduced BacMet levels (over 30%) and MGEs (28%) but had no significant effect on total ARG levels. Solids recovered through solid-liquid separation exhibited elevated levels of ARGs, BacMet, and MGEs, while the liquid fraction displayed levels similar to untreated manures. Correlation and co-occurrence modeling analyses indicated that changes in microbial communities, particularly fluctuations in aerobic and facultative communities belonging to Bacillota, Actinomycetota, and Pseudomonadota phyla, played a significant role in driving changes in ARGs, BacMet, and MGEs. The results also showed the presence of toxin-antitoxin and transposon systems near different ARGs. Overall, the results confirmed that genes conferring resistance to various antimicrobials and MGE capable of mobilizing them are widely spread in dairy farms; that even under the absence of antibiotics, the use of heavy metals and disinfectants may promote the maintenance of ARGs and MGEs, and; that treatment such as anaerobic digestion could reduce the risk of the spread of antimicrobial resistance.
1 Introduction
The widespread use of antimicrobials for human and veterinary applications has caused the rapid emergence and dissemination of antimicrobial-resistant bacteria (ARB) that threaten not only human health but also animal welfare and the food supply (WHO, 2018b). Livestock production is one of the main sources of antibiotics in the environment, as significant amounts are used as treatment and prophylactic therapies for infectious diseases (Kumar et al., 2012). Antibiotics used in veterinary applications include aminoglycosides, cephalosporins, fluoroquinolones, lincosamides, macrolides, cephalosporins, penicillin, sulfas, and tetracyclines, many of which are critically important antimicrobials and are essential to treat human diseases (WHO, 2018a; FDA, 2018). When used in animals, these antibiotics are generally poorly metabolized and are secreted in urine and feces (Jjemba, 2002), making animal manure a hotspot for the development and dissemination of antimicrobial resistance (Lima et al., 2020).
The presence of relatively high levels of residual antibiotics, ARB and ARGs in animal manures has been well documented (Wohde et al., 2016; Liu et al., 2019; Flores-Orozco et al., 2022). Since manure is commonly applied in agricultural soils as a crop fertilizer, there is a growing concern about the potential enrichment of ARGs and ARBs in manured soils and the ultimate effects this could have on human and animal health. For instance, some studies have found that manured soils tend to have higher levels of ARGs and ARBs than conventional agricultural soils (Hu et al., 2016; Xu et al., 2019; Wang et al., 2020; Zhang et al., 2021). Therefore, there is an urgent need to find alternatives to reduce the levels and diversity of ARGs and ARBs in manures before they reach the environment (Stoica and Cox, 2021).
One of the first options to reduce the risk of dissemination of antimicrobial resistance is limiting the use of antibiotics in animal husbandry. However, while more poultry, swine, and dairy farms are opting to limit the use of antibiotics, there are still concerns about animal health and welfare (Singer et al., 2019). Moreover, there is growing evidence of the presence of various ARGs in manure from organic farms where antibiotics are not used (Smith et al., 2013; Pitta et al., 2020). ARGs are often found on mobile genetic elements (MGEs) that can accumulate genes conferring resistance to different compounds (e.g., heavy metals, disinfectants) and other catabolic genes (e.g., for toluene/xylene, benzoate, and naphthalene degradation) (Shintani and Nojiri, 2013). Thus, selective pressures other than antibiotics, such as disinfection regimes and feed additives, can cause the indirect selection of ARGs and ARBs (Vats et al., 2022). All this implies that limiting the usage of antibiotics may not be enough to reduce the risk of the spread of antimicrobial resistance.
In the last decade, several studies have evaluated the fate of various ARGs in different types of animal manures during common manure treatments, including composting (Cui et al., 2016; Cheng et al., 2019), aerobic and anaerobic storage (Duriez and Topp, 2007; Wallace et al., 2018), and anaerobic digestion (AD) (Flores-Orozco et al., 2022). Although all these studies have shown that manure treatments can influence the levels of ARGs and MGEs in manure thanks to the specific microbial successions involved (Cheng et al., 2019; Zhang et al., 2020), it has not been possible to draw solid conclusions due to the variability of the results. Also, the great majority of studies have focused on a handful of genes, so it is not clear if the fate of a few genes represents the fate of the whole set of ARGs (resistome) and MGEs (mobilome). Additionally, the fate and evolution of ARGs and MGEs in novel manure treatments, such as the separation of solids from liquid manure (often used in cattle farms for recovering solids for animal bedding), have not been investigated. As the adoption of these treatments is expected to grow due to their various economic and environmental benefits, including increasing the value of manure and reducing the costs related to bedding materials and the reduction of odors and nutrients (Wicks and Keener, 2017), it is important to understand the dynamics of ARGs and MGEs in these contexts.
The use of metagenomics to study entire resistomes and mobilomes offers several benefits compared to traditional approaches based solely on qPCR techniques. For example, metagenomics can provide a holistic view of the relationships between the microbial communities and ARGs, allow for the quantification of the abundance of known and unknown resistance genes, and provide the genetic context of ARGs and their mobility potential (de Abreu et al., 2021). This approach has facilitated the identification of community-based antimicrobial resistance, sources of resistance, co-resistance, and potential pathways for transmission in natural environments and agricultural, municipal, and clinical settings (Zhang et al., 2011; Chen et al., 2017; Ma et al., 2020). However, the costs and complexity of the data analysis, combined with the lack of standard guidelines, have limited the use of metagenomics to study antimicrobial resistance in animal husbandry settings.
In this study, we used metagenomics to evaluate the fate of resistomes, mobilomes, and bactericides and heavy metals resistance (BacMet) genes in different manure treatments, including storage in an open (aerobic) tank, solid-liquid separation as part of a bedding-recovery unit (BRU), and mesophilic anaerobic digestion (MAD). One of the surveyed farms was operating in an antibiotic-free environment, so it provided insights into the effects of limiting the use of antibiotics on resistomes and mobilomes and the potential impact of other antimicrobials, such as foot bathing solutions, on them. The study also evaluated the co-occurrence of microbial groups, ARGs, and MGEs, to determine the microbial successions potentially driving the changes in resistomes and mobilomes under various manure management strategies. The results provide insights that could be applied to design treatments that may reduce the risk of the spread of antimicrobial resistance via manure applications.
2 Materials and methods
2.1 Sampling and DNA isolation
Untreated bovine manures were collected from the temporary manure pit of three dairy farms located in Manitoba, Canada, as follows: Two samples (4 months apart) of untreated manure (F1M1, F1M2) from a medium-size farm (∼200 milking cows, Farm 1); Two samples (1 year apart) of manure from a small antibiotic-free dairy farm (<100 milking cows, Farm 2); two samples of manure from a large farm (>500 milking cows, Farm 3). Treated manures were sampled as follows: Untreated manures from Farm 1 were digested in a bench-scale mesophilic anaerobic digester as described in Flores-Orozco et al. (2023). Hence, Farm 1 treated manures were the corresponding digestates (F1D1, F1D2). Farm 2 treated manures corresponded to manure stored in the open collection tank sampled in two consecutive fall seasons (F2S1, F2S2). This tank was 5.5 m in height and 28 m in diameter with no roof (aerobic) and was usually emptied twice a year during the spring and fall seasons. Farm 3 treated manures were the liquid (F3L1, F3L2) and solid (F3S1, F3S2) fractions of the BRU operated in the farm. In such systems, the solids are separated through a tumbling drum composter for approximately 24 h, reaching temperatures over 60°C. A detailed description of the operation of the BRU on this farm as well as the general physicochemical properties of the solids and liquids can be found in Ackerman et al. (2018). Farm 3 untreated manures were also digested (F3D1, F3D2) in a lab-scale mesophilic anaerobic digester similar to Farm 1 samples. A scheme of the sampling points manures can be found in the Supplementary Figure S1. All three farms reported the use of acidified copper sulfate solutions for foot bathing, typically composed of 80%–90% copper sulfate mixed with 10%–20% citric acid.
Untreated and treated manures were analyzed for total solids (TS), volatile solids (VS), chemical oxygen demand (COD), dissolved COD (dCOD), and alkalinity as described in (Flores-Orozco et al., 2020). These physicochemical properties can be found in the Supplementary Table S1. DNA was isolated from 350 mg centrifugated pellets (10 min at 13,000 x g) of untreated and treated manures using a PowerSoil DNA extraction kit (Omega Bio-tek, United States) following the process suggested by the company. A NanoDrop 1000 (Thermo Fisher Scientific, Canada) was used to determine DNA quality and concentration. DNA samples were sent to the Genome Quebec Innovation Centre (Montreal, Canada) for metagenomic sequencing, where an Illumina MiSeq platform was used to generate 250 bp paired-end metagenomic sequences.
2.2 Bioinformatics
All bioinformatic tasks were performed in the online platform Galaxy (Afgan et al., 2018). Quality control of raw sequence data was performed using FastQC (Andrews, 2010) to ensure data integrity for downstream analyses. The FastQC analysis encompassed assessments of basic statistics, per-base sequence quality, per-sequence quality scores, per-base sequence content, per-base GC content, per-sequence GC content, per-base N content, sequence length distribution, sequence duplication levels, overrepresented sequences, and adapter content. Based on the FastQC reports, sequences were trimmed and filtered to remove adapters and low-quality bases using Trimmomatic v0.38 (Bolger et al., 2014). The post-trimming sequence quality was reassessed to confirm that all remaining reads met the quality thresholds for subsequent analyses. The number of quality-controlled reads ranged from 428,841 to 909,101 in all samples.
Taxonomic labels were assigned to these reads using Kraken2 v2.1.1 (Wood et al., 2019) in fast operation mode (70% confidence, five minimum hit groups) and its standard database (2021, May 17). Then, the OTUs’ relative abundances were estimated using Bracken v2.7 (Lu et al., 2017). Note that the taxonomic nomenclature used in this study corresponded to the NCBI database as of 05 April 2023.
The presence of ARGs, biocides and heavy metals (BacMet) genes, and MGE-like and related module sequences was determined following a read-based and a co-assembly approach. For the read-based approach, quality-controlled reads were aligned against the CARD v3.1 (Alcock et al., 2020), BacMet2–experimentally confirmed (Pal et al., 2014), and the MobileOG v1.5 (Brown et al., 2021) databases to determine ARG-, BacMe-, and MGE-like sequences, respectively, using DIAMOND blastx v2.015 (Buchfink et al., 2014) in sensitive mode (e-value < 1e-10, identity percentage >90%, query coverage >90%, maximum number of query hits = 2). All genes were classified according to the description of their respective databases. Note that the genes listed in the mobileOG database include proteins known to be part of the machinery required for gene mobilization but that are not necessarily within the MGEs themselves. To simplify the analysis, all these genes are referred to as MGEs in the rest of the document. The abundance of all these genes was normalized to the number of quality-controlled sequences and the length of the genes and reported as reads per kilobase million (rpkm).
To examine the co-occurrence of ARGs and MGEs, we aimed to construct longer contigs. Given the insufficient number of metagenomic reads for separate assemblies from each sample, we employed a co-assembly method. This involved creating contigs using the collective sequence data from each sample set (e.g., Farm 1 untreated manure, Farm 1 treated manure, etc.) with Megahit utilizing the default settings. The co-assembled contigs were aligned to the CARD, BacMet, and mobileOG databases using DIAMOND blastx (e-value < 1e-10, identity percentage >90%, subject coverage >60%, maximum number of query hits = 2). The position of MGEs, ARGs, and MGEs was also determined in contigs where these elements co-occurred.
2.3 Data analyses
ARGs were grouped by the mechanism and the antibiotic class they encoded resistance to, as described in the database. ARGs for macrolide, lincosamide, and streptogramin were grouped as mls; ARGs encoding resistance to more than one antibiotic were classified as multidrug. BacMet genes were also grouped by the type of compound they exert resistance to as defined in the database; those genes encoding resistance to more than one different compound type were classified as multi-compound. MGEs were classified based on their molecular mechanisms described in the mobileOG database.
Principal Coordinates Analyses (PCoA) based on Bray-Curtis were used to determine similarities between the different groups of samples in terms of ARGs, BacMet, MGEs, and microbial communities. The Chao, Shannon, and Simpson diversity indices were estimated using the R package vegan. The 95%-confidence intervals of the diversity indices were estimated via bootstrapping (n = 1500, sample size = 133). Mantel tests were used to determine the correlations between the total levels of ARGs, MGEs, and the microbial genera grouped by the type of respiration (i.e., aerobic, anaerobic, and facultative). The relationships between microbial genera and ARGs and MGEs were further explored as follows: First, Pearson’s correlation coefficients of ARGs, MGEs, and microbial genera were calculated. Second, in order to reduce spurious correlations and false positives, a probabilistic model of species co-occurrence (Veech, 2013) was applied using the R package cooccur. Then, microbial genera that co-occurred at a significantly (p-value <0.025) higher frequency with ARGs and MGEs and had positive and significant correlation coefficients (r > 0.50, p-value <0.05) were defined as genera potentially carrying ARGs. Statistically significant differences in the different parameters evaluated in this study were determined using t-tests with an alpha of 0.05.
3 Results and discussion
3.1 Microbiomes in untreated and treated manures
3.1.1 Microbiomes in untreated manures
The microbial communities at the phylum and genus levels of untreated and treated manures from the three different farms are shown in Figures 1A, B, respectively. The dominant phyla in untreated manure from Farm 1 (F1M1, F1M2) were Bacillota (51%), Actinomycetota (33%), Euryarchaeota (7.6%), Pseudomonadota (5.1%), and Bacteroidota (2.5%); in Farm 2 (F2M1, F2M2) Bacillota (49%), Actinomycetota (15%), Pseudomonadota (12.3%), Bacteroidota (11%), and Euryarchaeota (8.4%); and in Farm 3 (F3M1, F3M2) Bacillota (37%), Actinomycetota (22%), Pseudomonadota (17%), Bacteroidota (15.6%), and Euryarchaeota (24.8%). Although the dominant phyla were similar in all untreated manures, those from Farm 1 were characterized by higher levels of Actinomycetota (e.g., Bifidobacterium, Corynebacterium) and lower levels of Bacteroidota (e.g., Sphingobacterium, Phocaeeicola, Bacteroides). The higher levels of Mycoplasmatota (e.g., Haploplasma, Paracholeplasma, Acholeplasma) were distinctive of untreated manures from Farm 2. Also, the microbiomes in manures from Farm 2 were more diverse than the other untreated manures (Figure 1C), although they also had the lowest microbial richness indices (Figure 1D). On the other hand, Farm 3 was characterized by a higher abundance of Pseudomonadota (e.g., Acinetobacter, Pseudoxanthomonas) and Bacteroidota (e.g., Sphingobacterium, Bacteroides). The diversity indices indicated that the microbiomes in manures from Farm 3 were less diverse than those in Farm 2 but similar to those in Farm 1 (Figure 1C), although they had higher microbial richness indices (Figure 1D).
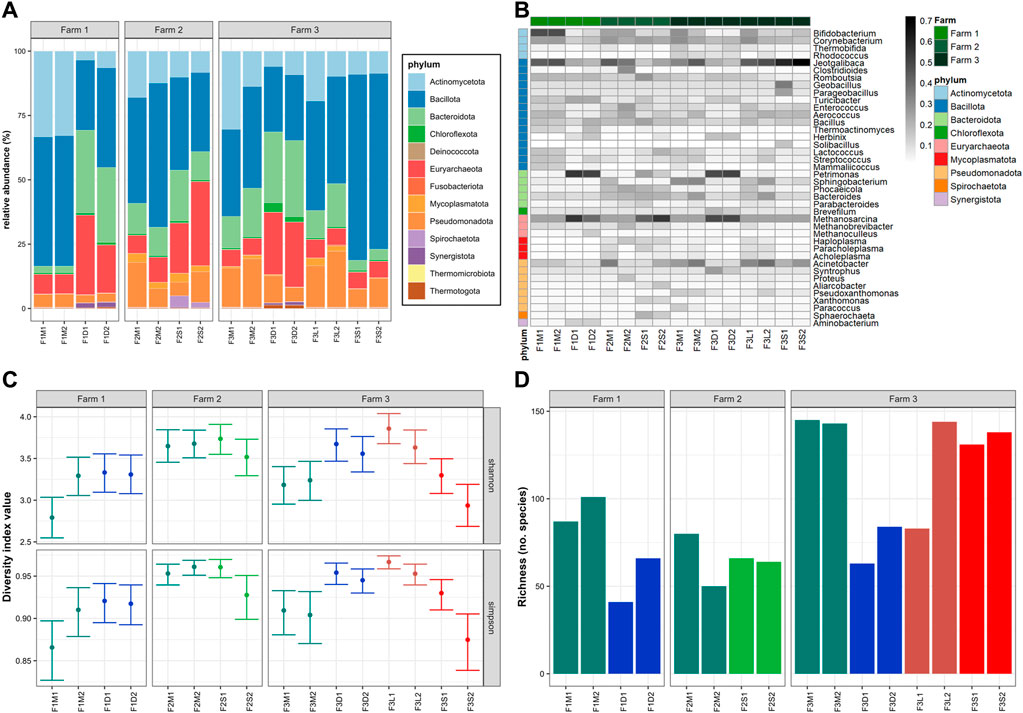
FIGURE 1. Microbiomes: (A) Microbial communities at phylum; (B) Top-40 microbial genera; (C) 95% confidence interval of Shannon and Simpson diversity indices; (D) Chao index. The color in the heatmap represents the squared root of relative abundance. Colors in C and D represent the different treatments.
To evaluate the overall similarity and dissimilarity of microbial communities among the different farms and manure samples, a PCoA was performed based on the phylum-level composition, as depicted in Figure 2A. The PCoA analysis explained 64.1% of the variation, with PC1 accounting for 48.6% and PC2 accounting for 15.5%. The PCoA plot revealed distinct clustering patterns, indicating that untreated manures from the same farm tended to group together and were distinguishable from manures obtained from the other farms. Notably, untreated manures from Farm 2 exhibited greater variability in the structure of their microbial communities. Specifically, F2M1 displayed a closer resemblance to the microbiome structure of manure from Farm 3, while F2M2 displayed a closer resemblance to the microbiomes of manures from Farm 1. These differences were primarily attributed to variations in the levels of Bacillota and Pseudomonadota between F2M1 and F2M2.
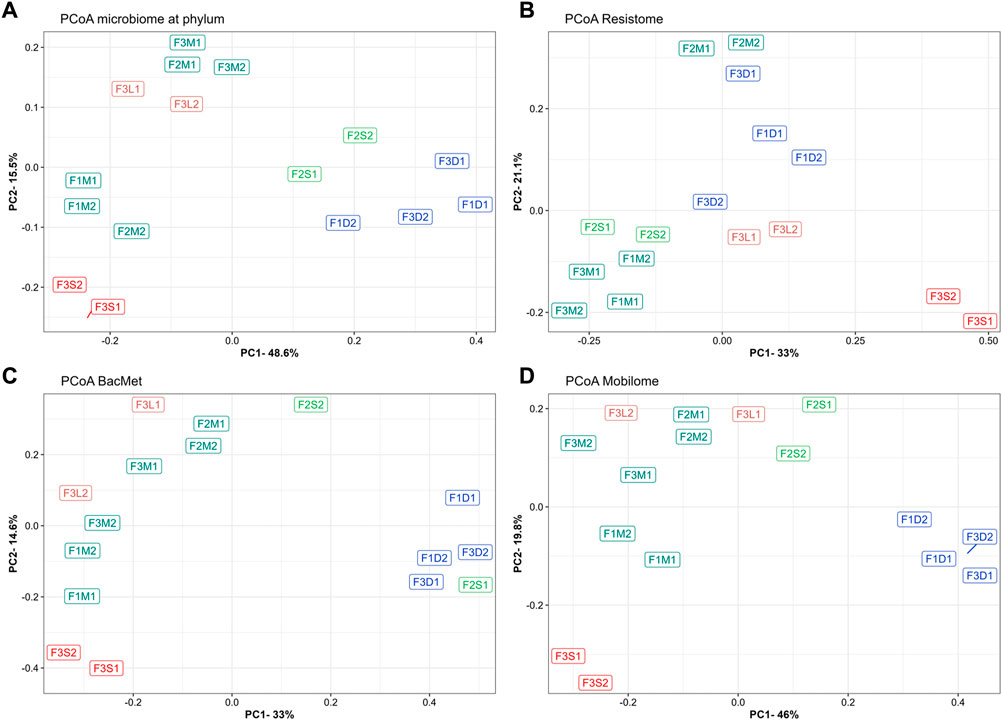
FIGURE 2. Principal coordinate analyses of microbiomes at phylum (A), ARGs (B), BacMet (C), and MGEs (D). Bray-Curtis distance was used to ordinate samples. Colors indicate the farm where the manure samples were collected.
The observed differences in the structures of microbial communities across the farms are likely influenced by a combination of factors, including each farm’s specific manure management strategies, unique farm operations such as feeding practices and disinfection regimes. Additionally, environmental and physicochemical variables like temperature, humidity, nitrogen, and oxygen levels in the manure storage areas could also have significant impacts (Sun et al., 2020). In the case of Farm 3, the recirculation of solids from the BRU may have influenced the microbiomes in the temporary manure pit where the samples were collected. Previous studies have reported the enrichment of specific bacteria, such as Streptococcus and Klebsiella, in farms utilizing BRUs (Harrison et al., 2008). However, there is still limited knowledge regarding the impact of such practices on microbial communities in manure pits and the potential consequences they may have. In contrast, the microbiome of manures from the antibiotic-free farm (Farm 2) exhibited higher diversity but lower microbial richness compared to the other farms (Figures 1C, D). Previous studies have reported that antibiotics can reduce the microbial diversity in complex microbiomes (Liu et al., 2020; Mazzurco Miritana et al., 2020). This suggests that the absence of residual antibiotics allowed natural competition that ultimately led to the establishment of more evenly distributed microbial communities.
Overall microbiome structure in untreated manures of the three survey farms corresponded to common manure microbiomes adapted to environmental conditions (e.g., higher O2) and not gut-adapted manure microbiomes (Sukhum et al., 2021). Untreated bovine manures are commonly rich in Pseudomonadata and Bacteroidota (Pitta et al., 2020), whereas in this study, Bacillota stood as the dominant phyla. Also, the results highlight the potential influence of manure management strategies, such as the use of BRUs or the absence of antimicrobials, on the microbial composition of manures. Further research is necessary to gain a deeper understanding of how these practices affect microbial communities in manure pits and the potential implications for overall ecosystem health and stability.
3.1.2 Microbiomes in treated manures
The impact of manure treatments on microbial communities was evident, leading to distinct changes in composition. For instance, MAD samples (F1D1, F1D2, F3D1, F3D2) exhibited higher abundances of Euryarchaeota (e.g., Methanosarcina), Bacteroidota (e.g., Petrimonas), Synergistota (e.g., Aminobacterium), and Thermotogota (e.g., Mesotoga), and lower levels of Actinobacteria (e.g., Bifidobacterium), Bacillota (e.g., Enterococcus, Aerococcus, Lactococcus, Streptococcus), and Pseudomonadota (e.g., Acinetobacter) (Figures 1A, B). As expected, the digested manures had elevated levels of anaerobic microorganisms and reduced levels of facultative and aerobic species (Supplementary Figure S2A). Also, these samples had lower levels of Gram-positive and higher levels of Gram-negative species (Supplementary Figure S2B). Moreover, the results showed that various microbial groups (e.g., Bifidobacterium, Enterococcus, Streptococcus) known to host pathogenic species were reduced in MAD (Figure 1B), indicating that digested manure would pose lower health risks. The microbial diversity in the digested manures tended to be higher but less rich compared to untreated manures (Figures 1C, D). The PCoA plot (Figure 2A) demonstrated that despite originating from different farms, the microbial communities in the digested manures exhibited similarities. Overall, the microbial communities in the MAD samples aligned with those typically observed in healthy mesophilic anaerobic digesters (Khafipour et al., 2020).
Manure storage reduced the abundance of Bacillota (e.g., Jeotgalibaca, Clostridioides), Actinomycetota (e.g., Bifidobacterium, Enterococcus, Aerococcus), and Pseudomonadota (e.g., Acinetobacter) and enriched Bacteroidota (e.g., Petrimonas), Euryarchaeota (e.g., Methanosarcina), and Spirochaetota (e.g., Sphaerochaeta) (Figures 1A, B). Some Bacillota genera, such as Rombustia, Lactococcus, and Streptococcus, and some Pseudomonadota, such as Aliarcobacter, Xanthomonas, and Sphaerochaeta, also tended to be higher in stored manure (Figure 1B). Although the microbial diversity of untreated and stored manures in the antibiotic-free farm was not significantly different (Figures 1C, D), the second stored batch (F2S2) tended to be less diverse and rich than the untreated batch (F2M2). The PCoA plot (Figure 2A) revealed that the microbial communities in stored manures exhibited greater similarity to those of digested manures, suggesting that manure storage facilitated the establishment of certain anaerobic communities commonly observed in anaerobic digesters (Supplementary Figure S2A).
Some differences between the microbial communities in untreated manure (F3M1, F3M2) and the streams of the BRU system (solids and liquids) were observed. For example, the microbiomes in the liquid stream (F3L1, F3L2) tended to have a higher abundance of Mycoplasmatota (e.g., Haploplasma, Paracholeplasma, Acholeplasma), a slightly higher abundance of some Bacillota (i.e., Jeotgalicoccus, Aerococcus) and Psuodomonadota (i.e., Acinetobacter), and lower levels of Actinomycetota (e.g., Thermobifida, Rhodococcus) (Figures 1A, B). However, the microbial richness and diversity indices were similar between the BRU-liquids and untreated manures. This similarity was further supported by the PCoA plot (Figure 2A), which demonstrated that these samples were closer to untreated manure (F3M1, F3M2). In a previous study, Ackerman et al. (2018) reported that the untreated manure and the liquids from the BRU of this farm had similar physicochemical properties, which could explain why the microbiomes were similar. In contrast, the microbiomes of the solids stream from the BRU (F3S1, F3S2) had a much higher abundance of Bacillota (e.g., Jeotgalibaca, Geobacillus, Parageobacillus, Aerococcus, Solibacillus), which represented 70% ± 3% of the microbial population. The levels of phyla, such as Actinomycetota (e.g., Bifidobacterium, Corynebacterium, Thermobifida, Rhodococcus), Pseudomonadota (e.g., Pseudoxanthomonas), and Bacteroidota (e.g., Sphingobacterium, Bacteroides), were found at lower concentrations in the BRU-solids samples compared to the levels in untreated manures. Additionally, while the microbial richness of the BRU-solids samples was comparable to that of untreated manures (Figure 1D), they exhibited significantly lower evenness (Figure 1C). These differences were evident in the PCoA plot, where the BRU-solids samples clustered distinctly apart from untreated manures and the BRU-liquids (Figure 2A). Overall, these results indicate that the microbial populations in the solids obtained from BRU systems can substantially differ from those found in untreated dairy manures and in the BRU-liquid stream.
3.2 Resistomes and mobilomes in untreated bovine manures
3.2.1 Resistomes in untreated manures
The read-based analysis revealed the presence of a substantial number of ARG-like sequences in untreated manures obtained from the three surveyed farms. In Farm 1, 52 different ARGs were identified, while Farm 2 and Farm 3 had 77 and 86 different ARGs, respectively (Supplementary Table S2). Antibiotic inactivation, efflux pumps, and antibiotic target protection were the predominant modes of action of ARGs observed in all untreated manures. Regarding specific antibiotic resistances, the most abundant ARGs were associated with tetracycline, aminoglycoside, macrolide-lincosamide-streptogramin (mls), beta-lactams, and multidrug resistance. Additionally, ARGs related to sulfonamides (e.g., sul1, sul2) were detected in all manure samples. These findings confirm that untreated bovine manures serve as reservoirs for numerous ARGs, including those for critically important antibiotics, as previously reported (Lima et al., 2020).
In terms of total levels of ARGs (Figures 3A, B), untreated manure from Farm 1 (666 ± 60 rpkm) tended to be slightly higher than those in manures from Farm 2 (519 ± 24 rpkm) and Farm 3 (558 ± 86 rpkm). These results suggested that the recovery and recirculation of solids in Farm 3 did not affect the total ARG levels, as they were similar to those from the other farms. Interestingly, the ARG levels in manures from the antibiotics-free farm (Farm 2) tended to be slightly lower, but they were not significantly different (p-value >0.05). This was unexpected because one would anticipate lower ARG levels in the absence of selective pressure from antibiotics. For example, a previous study reported a lower abundance and diversity of ARGs in manure from farms not using antibiotics compared to conventional farms (Pitta et al., 2020). This discrepancy may be the result of the different microbial communities as Pseudomonadota was the main phylum in manure microbiomes (accounting for over 55%), whereas in our study, the dominant phylum in the antibiotic-free farm was Bacillota (49%), and Pseudomonadota only accounted for around 12% of the microbiome. Given the co-location of ARGs and heavy metal resistance genes (Shintani and Nojiri, 2013), it is possible that heavy metals from foot baths, like copper, may indirectly select for ARGs, as suggested by prior research (Vats et al., 2022). Overall, the results confirmed the presence of relatively high levels of ARGs, potentially conferring resistance to tetracyclines, mls, and aminoglycosides in manure from antibiotic-free dairy farms. The resistome PCoA (Figure 2B), explaining 54% of the variation (PC1 = 33%; PC2 = 21.1%), revealed distinct resistome profiles in manure from Farm 2 (F2M1, F2M2), indicating differences in ARG diversity. Overall, these findings suggest that limiting the use of antibiotics may not be sufficient to minimize the risk of antibiotic resistance dissemination in manures. More in-depth studies on the long-term evolution of ARGs in antibiotic-free farms are required since other practices, such as the use of disinfectants or feed ingredients, could possibly cause the co-selection of ARGs (Mulder et al., 2018).
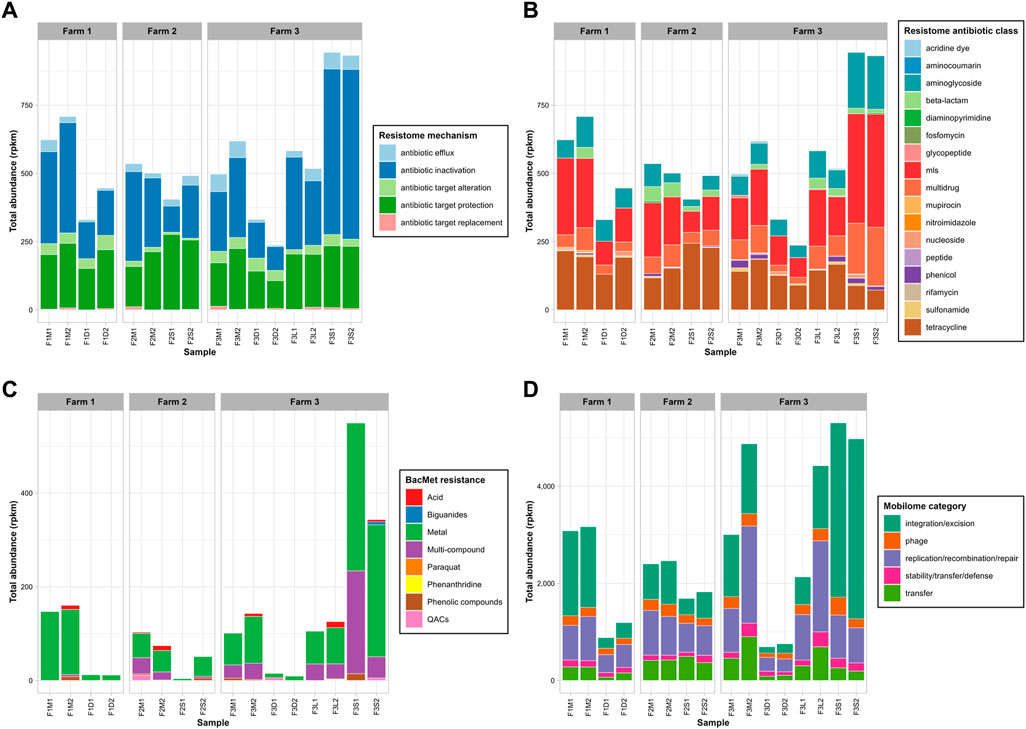
FIGURE 3. Total abundance of ARGs by mechanism (A) and antibiotic class (B), BacMet genes by compound class (C), and MGEs by category (D). On panel (B), mls: macrolide-lincosamide-streptogramin ARGs.
3.2.2 Bactericides and heavy metal resistance in untreated manures
Genes encoding resistance to biocides and heavy metals (BacMet) were also found in untreated manures from the three farms. A total of 18 BacMet genes were found in Farm 1, 31 in Farm 2, and 37 in Farm 3 (Supplementary Table S3). Genes encoding resistance to heavy metals (i.e., copA, tcr, acn) and multi-compounds (i.e., ade) were prevalent in all untreated manures. Additionally, genes linked to resistance to acids like sodium deoxycholate (e.g., evgS, mdt, yeg), and hydrochloric acid (e.g., gad) were also persistent in untreated manures. Farm 1 exhibited the highest overall levels of BacMet genes (154 ± 9 rpkm), while Farm 2 had the lowest values (89 ± 20 rpkm) (Figure 3C). However, the overall levels did not exhibit significant differences across the three farms. Among the BacMet genes, those associated with heavy metals, particularly copper (e.g., copA, tcrA, tcrB, tcrY), and iron (e.g., acn), were the most abundant across all three farms. The use of copper-based solutions for foot-bathing is a common practice in dairy farms to prevent hoof diseases (Cook, 2017). The farms surveyed in this study reported using copper sulphate solutions for this purpose. Therefore, it is plausible that this practice exerts selective pressure favoring the presence of heavy metal resistance genes in the microbiomes of manure. Similarly, using disinfectants like quaternary ammonium compounds (QACs) in daily farm operations may also contribute to selecting specific resistance genes (Mulder et al., 2018).
The PCoA based on BacMet-like genes revealed that untreated manures from Farm 1 and Farm 3 clustered closely together, while Farm 2 samples showed some differences (Figure 2C). Overall, these findings indicate that resistance genes associated with heavy metals and other compounds are prevalent in dairy farms. Further investigations are necessary to understand the impact of routine farm practices such as foot bathing and disinfection on the prevalence and spread of this type of resistance.
3.2.3 Mobilomes in untreated bovine manures
Untreated manures were also rich in MGEs-like sequences. A total of 167, 169, and 231 different genes were detected in untreated manures from Farm 1, Farm 2, and Farm 3, respectively (Supplementary Table S4). On average, the total MGE levels in untreated manures were 3,123 ± 61 rpkm in Farm 1, 2,432 ± 46 rpkm in Farm 2, and 3,939 ± 1,322 rpkm in Farm 3 (Figure 3D). The most common and abundant MGE groups in all untreated manures were replication/recombination/repair, integration/excision, and transfer. These results confirmed that bovine manures are important reservoirs of MGEs, as previous studies have suggested (Buta-Hubeny et al., 2022). The PCoA (Figure 2D), which explained 65.8% (PC1 = 46%; PC2 = 19.8%) of the variability of the MGE profiles, showed that manure samples from different farms tended to cluster apart and exhibit distinct profiles. Although the total MGE levels of the three farms were not significantly different, some patterns were observed. For example, the antibiotic-free farm (Farm 2) tended to have lower total MGE levels. Specifically, the integration/excision MGEs were significantly lower in Farm 2, whereas the phage group tended to be higher than in Farm 1 (Figure 3D). On the other hand, Farm 3, which utilized the BRU system, exhibited higher total MGE levels and richness. Specifically, phage-related MGEs were significantly more abundant, while transfer and replication/recombination/repair MGEs also tended to be higher. These findings suggest that the utilization of BRU systems may lead to an enrichment of microbial communities carrying MGEs. A more detailed discussion of putative MGE carriers is presented in subsequent sections.
Overall, the analysis of resistomes and mobilomes in the different farms confirmed the presence of various ARGs and MGE in animal manures, confirming that manure from dairy farms could provide favorable conditions for horizontal gene transfer of antimicrobial resistance elements. Also, the small difference in the total ARG and MGE levels in manure from the antibiotic-free farm (Farm 2) suggested that limiting the use of antibiotics may not be sufficient to minimize the levels of these antimicrobial resistance markers and associated risks.
3.3 Effect of manure treatments on resistomes
Mesophilic anaerobic digestion achieved the highest reduction rates of total ARG levels, reaching over 40% reduction in manures from Farm 1 (−42% ± 7%) and Farm 3 (−47.5% ± 20%). The PCoA (Figure 2B) indicated that MAD samples were similar between them and substantially different from untreated manures and other treated samples. The storage treatment showed no significant effect on the total ARG levels in untreated manures, although there was a slight decrease compared to the untreated samples. Similarly, the total ARG levels remained unchanged in the liquid stream of the BRU system, which is consistent with the minimum changes in microbial communities observed in this condition (Figure 1B). In contrast, the solids stream of the BRU system saw a 70% increase in the total ARG levels. This increase was primarily observed in antibiotic inactivation mechanisms (+145% ± 46%) (Figure 3A), as well as genes encoding resistance to aminoglycosides (+173% ± 28%), macrolide-lincosamide-streptogramin (mls) antibiotics (+131% ± 42%), and multidrug resistance (+135% ± 29%) (Figure 3B). The PCoA analysis indicated that these samples (F3S1, F3S2) exhibited significant differences in ARG diversity compared to the other samples (Figure 2B). The rise in ARG levels in the BRU solids samples was likely associated with an increase in the abundance of Bacillota (Figure 1B), a phylum known to harbor various species carrying ARGs (Flores-Orozco et al., 2023).
In terms of BacMet genes, MAD reduced over 80% of the total BacMet levels in untreated manures from Farm 1 (−92% ± 1%) and Farm 3 (−89% ± 6%). The remaining genes were primarily heavy metal resistance genes, present at low concentrations. Storage treatment (Farm 2) showed varying reduction rates of BacMet genes, ranging from 31% to 96%. While the majority of BacMet groups declined during storage, heavy metal resistance genes persisted in F2S2. The liquid fraction of the BRU system in Farm 3 exhibited reductions ranging from 12% to 5.12% in total BacMet levels. In contrast, the BRU-solids experienced a significant enrichment of BacMet genes, ranging from 140% to 440%. Notably, genes encoding resistance to heavy metals (273% ± 129%) were the main group enriched in the solid fraction. This may have been the result of the concentration of solids and the ultimately increase in the microbial density in the solids or the relative enrichment of some resistance genes due to water removal, or both.
Overall, all these findings emphasize MAD as the most effective method for reducing BacMet genes, followed by storage, although with variable outcomes. Conversely, the BRU system appeared to enrich genes conferring resistance to heavy metals and other compounds in the solid fraction. Further studies are warranted to assess the impact of solid recovery from manure on the evolution of these antimicrobial resistance markers in dairy farms, as this practice may be enriching different resistance genes.
The analysis of ARG and BacMet profiles in the treated manures revealed distinct clustering patterns in the PCoA plot (Figures 2B, C), indicating that different manure treatments had specific influences on ARGs. These findings support previous studies suggesting that the microbial dynamics associated with each treatment process play a significant role (Cheng et al., 2019; L; Zhang et al., 2020). Further sections provide detailed information on putative ARGs and BacMet carriers and the microbial dynamics involved.
3.4 Effect of manure treatments on mobilomes
MAD was the best alternative to reduce the total MGE levels from untreated manures, achieving a reduction of 68% ± 6% in Farm 1% and 81% ± 5% in Farm 2 (Figure 3D). The MGE groups that saw the greatest reduction in MAD were integration/excision (−86% ± 4%), transfer (−73% ± 19%), and replication/recombination/repair (−63% ± 19). Storage treatment resulted in a 28% ± 3% reduction in total MGE-like sequences. Integration/excision, phage-like, and replication/recombination/repair MGEs were reduced by 47% ± 10%, 31% ± 11%, and 30% ± 8%, respectively. The liquids of the BRU (F3L1, F3L2) saw a reduction of 19% ± 14% in MGE-like sequences, whereas they increased between 2% and 77% in the solids of the BRU (F3S1, F3S2). The variability of the results observed in Farm 3 was attributed to the high levels observed in F3M2, which was 60% higher than in F3M1. It is possible that this difference resulted from the differences in the microbial communities (i.e., Pseudomonadota) between the two samples. Despite this variability, some patterns emerged. For example, MGE-like sequences classified as integration/excision were the group that saw the greatest increase (169% ± 16%) in BRU-solids samples (Figure 3D). Interestingly, MGEs directly associated with transfer processes (e.g., conjugation, secretion, and transformation machinery) decreased by around 61% ± 25% in BRU solid samples. This observation, combined with the PCoA (Figure 2D) suggested a substantial change in the mobilome of these samples, which likely corresponded to the substantial changes in the microbial communities.
Overall, the results indicate that MAD was the best alternative to reduce the total levels of MGE-like sequences, ARGs, and BacMet genes in bovine dairy manure, which suggests that digested manure would pose a much lower risk of spread of antimicrobial resistance than untreated and stored manures, and the streams of BRU systems. The results also indicated that the solids obtained via BRU had higher levels of total MGEs, raising concerns about the potential increased risk of antimicrobial dissemination on farms with this type of system. Previous studies have observed increased levels of residual antimicrobials in the solid fraction of manures (Wallace et al., 2018), thus, it is possible that the increased levels of MGEs and ARGs are the results of a stronger selective pressure in the solid fraction of the BRU. However, it is worth noting that the solids analyzed in this study corresponded to the solids obtained directly from the BRU, which are known to have more easily digestible carbon, hence, more biological activity, than solids that have been piled and composted for several weeks (Ackerman et al., 2018). It is possible that the microbiomes, resistomes, and mobilomes of the untreated solids may be quite different from those in more mature solids. Further research is needed to gain a better understanding of the fate and evolution of ARGs in farms employing such manure recirculation systems.
3.5 Relationship between microbial groups, ARGs, and MGEs
3.5.1 Correlation between microbiomes, resistomes, and mobilomes
Mantel tests were used to evaluate the relationship between the resistome and mobilome profiles with the microbial communities. The results suggested that the correlation between the microbial communities at the phylum level and ARGs (R = 0.43, p-value = 0.002) and MGEs (R = 0.63, p-value = 0.001) was significantly positive. The microbiome profile at the genera level was also positively and significantly correlated with ARGs (R = 0.44, p-value = 0.001) and MGEs (R = 0.63, p-value = 0.001). Interestingly, the Mantel test also revealed that the relative abundance of aerobic and anaerobic communities was significantly correlated with resistomes (aerobes: R = 0.36, p-value = 0.003; anaerobes: R = 0.25, p-value = 0.04) and mobilomes (aerobes: R = 0.54, p-value = 0.001; anaerobes: R = 0.41, p-value = 0.003). Furthermore, a significant positive correlation was observed between the mobilomes and resistomes (R = 0.84, p-value = 0.008), indicating that changes in the mobilome may contribute to variations in ARGs. These findings confirm the substantial influence of microbial communities on the dynamics of ARGs and MGEs in manure-related environments, as previous studies have indicated (Zhang et al., 2019; Liu et al., 2020).
3.5.2 Microbial groups potentially carrying ARGs and MGEs
A model of co-occurrence combined with Pearson Correlation analyses was used to determine significant associations between microbial genera and ARGs, MGEs, and BacMet genes (Figure 4A). The results indicated that 34 different ARGs were associated with 69 genera, primarily belonging to the phyla Bacillota (22 genera), Pseudomonadota (11), Actinomycetota (7), and Bacteroidota (6). Bacillota were the main group significantly associated with all different ARG mechanisms, including efflux pumps (e.g., efrB, adeJ, tet(40), fexA, mefB, floR, pp-flo), target alteration (e.g., ileS, Erm), target protection (e.g., tetS, tet44, optrA, poxtA), target replacement (i.e., sul2), and ARGs for mls (i.e., lnuG, ErmF, mefB), tetracyclines (i.e., tetS, tet44, tet40), and sulfonamides (i.e., sul2). On the other hand, Pseudomonadota stood as the primary phylum associated with ARGs for beta-lactams (e.g., CARB), phenicol (e.g., floR, pp-flo, fexA), and multidrug (e.g., cfr(D), poxtA, smeE, efrB, optrA).
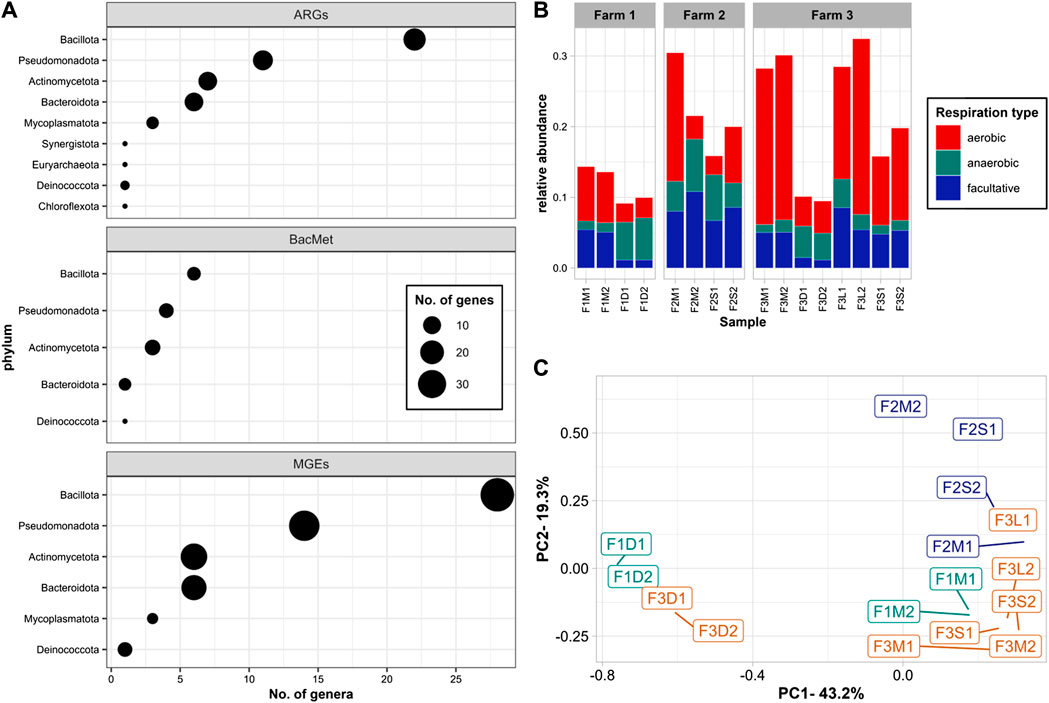
FIGURE 4. Co-occurrence: (A) Number of microbial genera significantly associated with ARGs, BacMet, and MGEs; (B) relative abundance of genera associated with ARGs, BacMet, and MGEs by respiration type; (C) PCoA of genera associated with ARGs, BacMet, and MGEs. Significant associations were defined as co-occurrence with a frequency higher than expected (p-values < 0.025) and positive Pearson’s correlation coefficient (R > 0.50). The size of the figure in panel A indicates the number of different genes associated with genera of the given phylum.
In terms of BacMet, 12 genes were significantly associated with 28 different genera. Bacillota (e.g., Amylolactobacillus, Carnobacterium, Erysipelothrix, Limosilactobacillus, Mammaliicoccus, and Staphylococcus) was the main group associated with genes conferring resistance to heavy metals, including iron (i.e., acn), copper (i.e., tcrY), and Arsenic (i.e., arsB). Pseudomonadota (e.g., Comamonas, Halopseudomonas) was the main group associated with genes conferring resistance to multi compounds (e.g., ruvB, rpoS, smeE, srpB).
A total of 96 different MGEs were significantly associated with 58 genera, of which 28 were Bacillota, 14 Pseudomonadota, 6 Actinomycetota, and 6 Bacteroidota. Staphylococcus, Amylolactobacillus, and Carnobacterium were the top genera associated with integration/excision genes; Comamonas and Glutamicibacter with replication/recombination/repair; Glutamicibacter and Psychrobacter with transfer; Myroides and Comamonas with stability/transfer/defense; and Carnobacterium, Comamonas, Erysipelothrix, and Jeotgalicoccus with phage related MGEs.
A table with the list of bacterial genera linked to both ARGs and MGEs can be found in the Supplementary Table S5. Glutamicibacter, Comamonas, Psychrobacter, Empedobacter, Advenella, Macrococcus, Carnobacterium, Moraxella, Petrocella, and Staphylococcus stood as the genera associated with the highest number of ARGs and MGEs. Many of these genera are known to host important pathogenic species with high antimicrobial resistance potential (CDC, 2019), confirming that livestock manure is an important reservoir of pathogenic bacteria with the genetic machinery to disseminate antimicrobial resistance.
3.5.3 Co-occurrence of ARGs and MGEs in co-assembled contigs
The co-occurrence of ARGs and MGEs was investigated by analyzing co-assembled contigs. Intriguingly, these analyses revealed the presence of structures resembling the transposon family Tn554, which encompassed the transposition genes tnpB and tnpC, along with different ARGs such as ANT(9)-la, ErmA, LnuG, and LnuP in samples obtained from Farm 2 and Farm 3 (Figure 4). These transposon structures are commonly associated with Bacillota genera, including Staphylococcus, Enterococcus, Listeria, and Bacillus (Li et al., 2019). Remarkably, these transposon structures were also observed in the antibiotic-free farm, indicating the widespread distribution of horizontal gene transfer machinery for various antimicrobial resistance genes in dairy farms. Previous studies have proposed that ruminant guts could exert selective pressure favouring certain tet ARGs (Sabino et al., 2019). Therefore, it is possible that even in the absence of antibiotics, other selective pressures may exist, promoting the prevalence of mls and aminoglycoside ARGs.
Genes encoding the Zeta toxin and Epsilon antitoxin, which are part of a type II toxin-antitoxin (TA) system, were identified in a contig derived from a MAD sample obtained from Farm 1, in close proximity to the ARG ErmB (Figure 4). Type II TA systems are genetic elements present in low-copy plasmids and bacterial and archaeal chromosomes and are involved in different biological roles, including stress responses, and plasmid and genome stabilization (Fraikin et al., 2020). TA systems function by producing a toxin and its corresponding antitoxin, which neutralizes the toxin, ensuring the survival of cells containing the system. These systems are often associated with MGEs and have been linked to ARGs conferring resistance to erythromycin (Zielenkiewicz and Cegłowski, 2005). Interestingly, ARGs encoding resistance to this type of antibiotic are persistently found in manure anaerobic digesters (Tran et al., 2021; Flores-Orozco et al., 2023), where there should be minimum selective pressure in favor of these ARGs. These results suggested that Erm genes may be preserved due to the indirect effect of nearby TA systems present in microbial communities in anaerobic digester-related environments. According to the mobileOG, the Zeta toxin and Epsilon antitoxin found in the co-assembled contig belonged to the Lactobacilli genera (i.e., Streptococcus, Enterococcus). Since the abundance of Lactobacilli was reduced during MAD, but Erm ARGs persisted, it is possible that these elements were transferred to another group of closely related microorganisms (e.g., Bacilli) that remained in the anaerobic digester.
Overall, the analysis of co-assembled contigs provided further confirmation of the findings obtained from the co-occurrence model, highlighting that genera from the phylum Bacillota are major carriers of ARGs and MGEs. These results suggest that the persistence of ARGs in untreated and treated manure, even in the absence of antimicrobial selective pressure, could be attributed to their proximity to systems such as type II TA systems that indirectly contribute to their maintenance. The identification of transposon genes in the vicinity of various ARGs (e.g., Ant(9)-la, lnuP, lnuG) provides additional evidence of the potential transmissibility of these genes in manure-related environments. However, further metagenomic studies with higher sequencing depths (e.g., tens of millions of reads per sample) are needed to comprehensively assess how these systems influence the preservation and dissemination of ARGs and antibiotic resistance in manure-related environments.
3.6 Microbiome shifts potentially influencing resistomes and mobilomes in manure treatments
Several studies have emphasized the significant role of microbial communities in driving changes in ARGs and MGEs levels. These investigations have shown that shifts in microbial populations with distinct resistomes and mobilomes can occupy ecological niches and influence resistance profiles (Sun et al., 2016; Liu et al., 2020; Wu et al., 2020; Flores-Orozco et al., 2023). For example, during manure composting, the reduction in ARG levels has been associated with the establishment of thermophilic communities at the expense of original mesophilic consortia (Liu et al., 2020). Similarly, studies on pig manure have indicated that thermophilic anaerobic digestion is more effective than mesophilic digestion in reducing ARGs due to the elimination of mesophilic bacteria, particularly Bacteroidetes, and Proteobacteria (Sun et al., 2016). Another study linked the reduction of ARGs to the decline of facultative anaerobes, especially Lactobacilli, during MAD (Flores-Orozco et al., 2023). In our study, the decline in ARG levels corresponded to the decrease in aerobic and facultative communities observed in untreated and digested manures, with a more pronounced effect observed in the digested sample (Figure 5B).
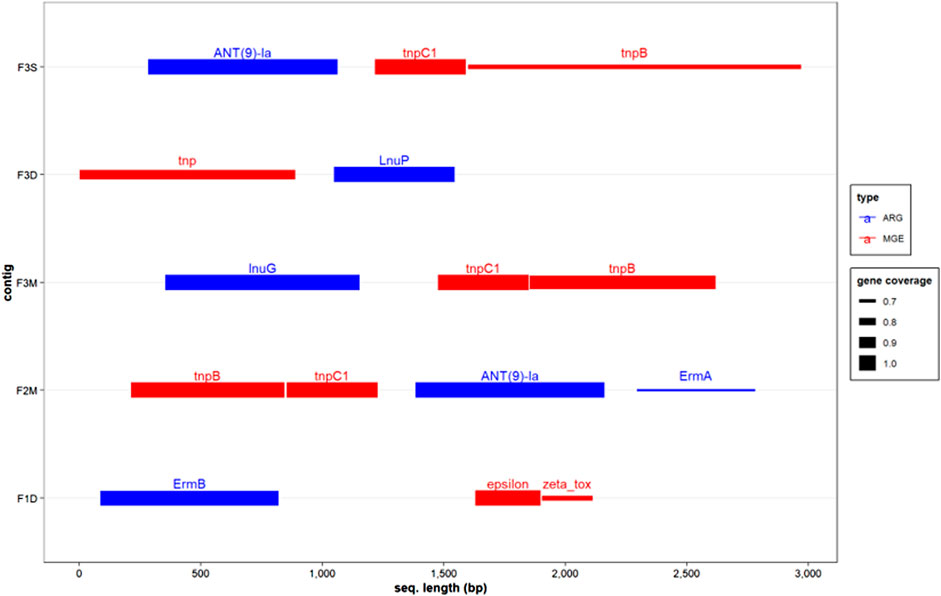
FIGURE 5. Co-occurrence of ARGs and MGEs in co-assembled contigs. The pair of metagenomic samples of untreated and treated manures from each farm were used to generate co-assembled contigs. The size of the segment indicates the percentage of the genes detected in the contig. F1D: digested manure from Farm 1; F2M: untreated manure from Farm 2; F3M untreated manure from Farm 3; F3D: digested manure from farm 3; F3S: BRU-solids from farm 3.
On the other hand, the increase of the aerobic communities in the solids recovered from the BRU (Supplementary Figure S2A) appeared to be associated with increased levels of ARGs (Figures 3A, B). Notably, although the relative abundance of the aerobic groups significantly associated with ARGs and MGEs was lower in the BRU-solids compared to those in the untreated samples (Figure 5B), the total ARG and MGE levels were higher (Figure 3). Since the resistome and mobilome profiles were different from those dominated by anaerobic communities (e.g., F3D1 and F3D2), it is possible that the co-occurrence analysis was not able to detect aerobic groups carrying ARGs and MGEs exclusively present in the BRU-solids samples. Further investigations are necessary to comprehensively assess the dynamics of resistomes and mobilomes in manure solids recovery units like BRUs, as this practice may potentially contribute to elevated levels of various ARGs and MGEs in dairy farm settings.
Although the changes in the microbial communities during storage did not seem to have influenced the total ARG levels in untreated manure from the antibiotic-free farm, the overall profiles were substantially different. In fact, the resistome profiles of stored manure were more similar to digested manures than to untreated manure from Farm 2 (Figure 2B). For example, the levels of tetracycline ARGs tended to be higher in stored manure, whereas ARGs for mls and multidrug tended to be higher in untreated manures (Figure 3B). These changes in the ARG profile were likely associated with the restructuring of microbial communities within the storage tank, characterized by a decline in aerobic populations and an enrichment of anaerobes (Supplementary Figure S2).
The PCoA of the abundance of genera significantly associated with ARGs and MGEs indicated that despite the differences in microbiomes, resistomes, and mobilomes, treated and untreated manures tended to be similar and clustered together (Figure 5C). This suggested that there were some groups of microorganisms potentially carrying ARGs and MGEs persistent in untreated and treated manures. However, digested manures displayed a distinct profile of microbes associated with ARGs and MGEs, which suggested that MAD allowed the establishment of microbiomes with resistomes and mobilomes that differ from those in untreated manures. The discrepancies in the total levels of ARGs and MGEs and the abundance of microbial groups potentially carrying them demonstrate the complex relationship between antimicrobial resistance markers and microbial communities. These findings emphasize the need for more comprehensive metagenomic studies to identify the specific microbial species carrying ARGs and elucidate the mechanisms underlying their maintenance and mobilization as the major sources for the antibiotic resistance load circulating through the animals and barn has not been determined accurately yet.
4 Conclusion
This study demonstrated that dairy manures are important reservoirs of genes conferring resistance to several different antimicrobials, including antibiotics and heavy metals, and of different MGEs that could potentially disseminate antimicrobial resistance. The results showed that manure storage, a widely used manure management strategy, has little effect on the total ARG and MGE levels, whereas MAD stood as the best alternative to reduce the levels and diversity of these elements. The results also showed that BRU systems caused an enrichment of ARGs and MGEs in the recovered solids, although it had little effect on the liquid stream. Changes in the microbial community structure, particularly the decline of aerobic and facultative genera from Bacillota, Pseudomonadota, and Actinomycetota phyla, were key drivers influencing the changes in ARGs and MGEs across different treatments. Finally, this study suggested that the use of heavy metals and disinfectants in dairy farms may promote the maintenance and possibly the amplification of ARGs and MGEs even in antibiotic free environments.
Data availability statement
The datasets presented in this study can be found in online repositories. The names of the repository/repositories and accession number(s) can be found below: https://www.ncbi.nlm.nih.gov/bioproject/?term=PRJNA1020798.
Author contributions
DF-O: Data curation, Formal Analysis, Investigation, Methodology, Visualization, Writing–original draft, Writing–review and editing. DL: Conceptualization, Writing–review and editing. AK: Conceptualization, Writing–review and editing. RS: Conceptualization, Writing–review and editing. NC: Conceptualization, Funding acquisition, Resources, Supervision, Writing–review and editing.
Funding
The authors declare financial support was received for the research, authorship, and/or publication of this article. The National Sciences and Engineering Research Council of Canada (NSERC) supported this research work financially through the Discovery Grants program RGPIN-2022-03670.
Conflict of interest
The authors declare that the research was conducted in the absence of any commercial or financial relationships that could be construed as a potential conflict of interest.
The authors declared that they were an editorial board member of Frontiers, at the time of submission. This had no impact on the peer review process and the final decision.
Publisher’s note
All claims expressed in this article are solely those of the authors and do not necessarily represent those of their affiliated organizations, or those of the publisher, the editors and the reviewers. Any product that may be evaluated in this article, or claim that may be made by its manufacturer, is not guaranteed or endorsed by the publisher.
Supplementary material
The Supplementary Material for this article can be found online at: https://www.frontiersin.org/articles/10.3389/fsybi.2023.1301879/full#supplementary-material
References
Ackerman, J., Khafipour, E., and Cicek, N. (2018). Sustainable re-use of dairy cow manure as bedding and compost: nutrients and self-heating potential. Can. Biosyst. Eng./ Le. Genie Des. Biosyst. au Can. 60, 61–67. doi:10.7451/CBE.2018.60.6.1
Afgan, E., Baker, D., Batut, B., Van Den Beek, M., Bouvier, D., Ech, M., et al. (2018). The Galaxy platform for accessible, reproducible and collaborative biomedical analyses: 2018 update. Nucleic Acids Res. 46, W537–W544. doi:10.1093/nar/gky379
Alcock, B. P., Raphenya, A. R., Lau, T. T. Y., Tsang, K. K., Bouchard, M., Edalatmand, A., et al. (2020). CARD 2020: antibiotic resistome surveillance with the comprehensive antibiotic resistance database. Nucleic Acids Res. 48, D517–D525. doi:10.1093/nar/gkz935
Andrews, S. (2010). Babraham bioinformatics - FastQC A quality control tool for high throughput sequence data. Available at: https://www.bioinformatics.babraham.ac.uk/projects/fastqc/ (Accessed July 22, 2022).
Bolger, A. M., Lohse, M., and Usadel, B. (2014). Trimmomatic: a flexible trimmer for Illumina sequence data. Bioinformatics 30, 2114–2120. doi:10.1093/bioinformatics/btu170
Brown, C. L., Mullet, J., Hindi, F., Stoll, J. E., Gupta, S., Choi, M., et al. (2021). mobileOG-db: a manually curated database of protein families mediating the life cycle of bacterial mobile genetic elements. bioRxiv. doi:10.1101/2021.08.27.457951
Buchfink, B., Xie, C., and Huson, D. H. (2014). Fast and sensitive protein alignment using DIAMOND. Nat. Methods 12, 59–60. doi:10.1038/NMETH.3176
Buta-Hubeny, M., Korzeniewska, E., Hubeny, J., Zieliński, W., Rolbiecki, D., Harnisz, M., et al. (2022). Structure of the manure resistome and the associated mobilome for assessing the risk of antimicrobial resistance transmission to crops. Sci. Total Environ. 808, 152144. doi:10.1016/j.scitotenv.2021.152144
CDC (2019). Antibiotic resistance threats in the United States, 2019. Atlanta, Georgia: CDC. doi:10.15620/cdc:82532
Chen, B., He, R., Yuan, K., Chen, E., Lin, L., Chen, X., et al. (2017). Polycyclic aromatic hydrocarbons (PAHs) enriching antibiotic resistance genes (ARGs) in the soils. Environ. Pollut. 220, 1005–1013. doi:10.1016/j.envpol.2016.11.047
Cheng, D., Feng, Y., Liu, Y., Xue, J., and Li, Z. (2019). Dynamics of oxytetracycline, sulfamerazine, and ciprofloxacin and related antibiotic resistance genes during swine manure composting. J. Environ. Manage 230, 102–109. doi:10.1016/j.jenvman.2018.09.074
Cook, N. B. (2017). A review of the design and management of footbaths for dairy cattle. Veterinary Clin. N. Am Food Animal Pract. 33, 195–225. doi:10.1016/j.cvfa.2017.02.004
Cui, E., Wu, Y., Zuo, Y., and Chen, H. (2016). Effect of different biochars on antibiotic resistance genes and bacterial community during chicken manure composting. Bioresour. Technol. 203, 11–17. doi:10.1016/j.biortech.2015.12.030
de Abreu, V. A. C., Perdigão, J., and Almeida, S. (2021). Metagenomic approaches to analyze antimicrobial resistance: an overview. Front. Genet. 11, 575592. doi:10.3389/fgene.2020.575592
Duriez, P., and Topp, E. (2007). Temporal dynamics and impact of manure storage on antibiotic resistance patterns and population structure of Escherichia coli isolates from a commercial swine farm. Appl. Environ. Microbiol. 73, 5486–5493. doi:10.1128/AEM.00218-07
FDA (2018). 2018 summary report on antimicrobials sold or distributed for use in food-producing animals. Available at: https://www.fda.gov/media/133411/download (Accessed June 1, 2023).
Flores-Orozco, D., Levin, D., Kumar, A., Sparling, R., and Cicek, N. (2022). A meta-analysis reveals that operational parameters influence levels of antibiotic resistance genes during anaerobic digestion of animal manures. Sci. Total Environ. 814, 152711. doi:10.1016/j.scitotenv.2021.152711
Flores-Orozco, D., Patidar, R., Levin, D., Kumar, A., Sparling, R., and Cicek, N. (2023). Metagenomic analyses reveal that mesophilic anaerobic digestion substantially reduces the abundance of antibiotic resistance genes and mobile genetic elements in dairy manures. Environ. Technol. Innov. 30, 103128. doi:10.1016/j.eti.2023.103128
Flores-Orozco, D., Patidar, R., Levin, D. B., Sparling, R., Kumar, A., and Çiçek, N. (2020). Effect of mesophilic anaerobic digestion on the resistome profile of dairy manure. Bioresour. Technol. 315, 123889. doi:10.1016/j.biortech.2020.123889
Fraikin, N., Goormaghtigh, F., and Van Melderen, L. (2020). Type II toxin-antitoxin systems: evolution and revolutions. J. Bacteriol. 202, 007633–e819. doi:10.1128/JB.00763-19
Harrison, E., Bonhotal, J., Schwarz Prepared, M., and Fiesinger, T. (2008). Using manure solids as bedding. Available at: https://ecommons.cornell.edu/handle/1813/44571 (Accessed April 26, 2023).
Hu, H. W., Han, X. M., Shi, X. Z., Wang, J. T., Han, L. L., Chen, D., et al. (2016). Temporal changes of antibiotic-resistance genes and bacterial communities in two contrasting soils treated with cattle manure. FEMS Microbiol. Ecol. 92, fiv169–13. doi:10.1093/femsec/fiv169
Jjemba, P. K. (2002). The potential impact of veterinary and human therapeutic agents in manure and biosolids on plants grown on arable land: a review. Agric. Ecosyst. Environ. 93, 267–278. doi:10.1016/S0167-8809(01)00350-4
Khafipour, A., Jordaan, E. M., Flores-Orozco, D., Khafipour, E., Levin, D. B., Sparling, R., et al. (2020). Response of microbial community to induced failure of anaerobic digesters through overloading with propionic acid followed by process recovery. Front. Bioeng. Biotechnol. 8, 604838. doi:10.3389/fbioe.2020.604838
Kumar, R. R., Lee, J. T., and Cho, J. Y. (2012). Fate, occurrence, and toxicity of veterinary antibiotics in environment. J. Korean Soc. Appl. Biol. Chem. 55, 701–709. doi:10.1007/s13765-012-2220-4
Li, D., Li, X. Y., Schwarz, S., Yang, M., Zhang, S. M., Hao, W., et al. (2019). Tn6674 is a novel enterococcal optrA-carrying multiresistance transposon of the Tn554 family. Antimicrob. Agents Chemother. 63, e00809–e00819. doi:10.1128/AAC.00809-19
Lima, T., Domingues, S., and Silva, G. J.Da (2020). Manure as a potential hotspot for antibiotic resistance dissemination by horizontal gene transfer events. Vet. Sci. 7, 110. doi:10.3390/VETSCI7030110
Liu, J., Zhao, Z., Avillan, J. J., Call, D. R., Davis, M., Sischo, W. M., et al. (2019). Dairy farm soil presents distinct microbiota and varied prevalence of antibiotic resistance across housing areas. Environ. Pollut. 254, 113058. doi:10.1016/j.envpol.2019.113058
Liu, Y., Cheng, D., Xue, J., Weaver, L., Wakelin, S. A., Feng, Y., et al. (2020). Changes in microbial community structure during pig manure composting and its relationship to the fate of antibiotics and antibiotic resistance genes. J. Hazard Mater 389, 122082. doi:10.1016/j.jhazmat.2020.122082
Lu, J., Breitwieser, F. P., Thielen, P., and Salzberg, S. L. (2017). Bracken: estimating species abundance in metagenomics data. PeerJ Comput. Sci. 2017, e104. doi:10.7717/peerj-cs.104
Ma, C., Lo, P. K., Xu, J., Li, M., Jiang, Z., Li, G., et al. (2020). Molecular mechanisms underlying lignocellulose degradation and antibiotic resistance genes removal revealed via metagenomics analysis during different agricultural wastes composting. Bioresour. Technol. 314, 123731. doi:10.1016/j.biortech.2020.123731
Mazzurco Miritana, V., Massini, G., Visca, A., Grenni, P., Patrolecco, L., Spataro, F., et al. (2020). Effects of sulfamethoxazole on the microbial community dynamics during the anaerobic digestion process. Front. Microbiol. 11, 537783. doi:10.3389/fmicb.2020.537783
Mulder, I., Siemens, J., Sentek, V., Amelung, W., Smalla, K., and Jechalke, S. (2018). Quaternary ammonium compounds in soil: implications for antibiotic resistance development. Rev. Environ. Sci. Biotechnol. 17, 159–185. doi:10.1007/s11157-017-9457-7
Pal, C., Bengtsson-Palme, J., Rensing, C., Kristiansson, E., and Larsson, D. G. J. (2014). BacMet: antibacterial biocide and metal resistance genes database. Nucleic Acids Res. 42, D737–D743. doi:10.1093/nar/gkt1252
Pitta, D. W., Indugu, N., Toth, J. D., Bender, J. S., Baker, L. D., Hennessy, M. L., et al. (2020). The distribution of microbiomes and resistomes across farm environments in conventional and organic dairy herds in Pennsylvania. Environ. Microbiome 15, 21. doi:10.1186/s40793-020-00368-5
Sabino, Y. N. V., Santana, M. F., Oyama, L. B., Santos, F. G., Moreira, A. J. S., Huws, S. A., et al. (2019). Characterization of antibiotic resistance genes in the species of the rumen microbiota. Nat. Commun. 10, 5252–5311. doi:10.1038/s41467-019-13118-0
Shintani, M., and Nojiri, H. (2013). “Mobile Genetic Elements (MGEs) carrying catabolic genes,” in Management of microbial Resources in the environment (Netherlands: Springer Netherlands), 167–214. doi:10.1007/978-94-007-5931-2_8/TABLES/6
Singer, R. S., Porter, L. J., Thomson, D. U., Gage, M., Beaudoin, A., and Wishnie, J. K. (2019). Raising animals without antibiotics: U.S. Producer and veterinarian experiences and opinions. Front. Vet. Sci. 6, 452. doi:10.3389/fvets.2019.00452
Smith, T. C., Gebreyes, W. A., Abley, M. J., Harper, A. L., Forshey, B. M., Male, M. J., et al. (2013). Methicillin-resistant Staphylococcus aureus in pigs and farm workers on conventional and antibiotic-free swine farms in the USA. PLoS One 8, e63704. doi:10.1371/journal.pone.0063704
Stoica, C., and Cox, G. (2021). Old problems and new solutions: antibiotic alternatives in food animal production. Can. J. Microbiol. 67, 427–444. doi:10.1139/cjm-2020-0601
Sukhum, K. V., Vargas, R. C., Boolchandani, M., D’Souza, A. W., Patel, S., Kesaraju, A., et al. (2021). Manure microbial communities and resistance profiles reconfigure after transition to manure pits and differ from those in fertilized field soil. mBio 12, e00798-21. doi:10.1128/mBio.00798-21
Sun, L., Han, X., Li, J., Zhao, Z., Liu, Y., Xi, Q., et al. (2020). Microbial community and its association with physicochemical factors during compost bedding for dairy cows. Front. Microbiol. 11, 254. doi:10.3389/fmicb.2020.00254
Sun, W., Qian, X., Gu, J., Wang, X. J., and Duan, M. L. (2016). Mechanism and effect of temperature on variations in antibiotic resistance genes during anaerobic digestion of dairy manure. Sci. Rep. 6, 30237. doi:10.1038/srep30237
Tran, T. T., Scott, A., Tien, Y. C., Murray, R., Boerlin, P., Pearl, D. L., et al. (2021). On-farm anaerobic digestion of dairy manure reduces the abundance of antibiotic resistance-associated gene targets and the potential for plasmid transfer. Appl. Environ. Microbiol. 87, e0298020–20. doi:10.1128/AEM.02980-20
Vats, P., Kaur, U. J., and Rishi, P. (2022). Heavy metal-induced selection and proliferation of antibiotic resistance: a review. J. Appl. Microbiol. 132, 4058–4076. doi:10.1111/jam.15492
Veech, J. A. (2013). A probabilistic model for analysing species co-occurrence. Glob. Ecol. Biogeogr. 22, 252–260. doi:10.1111/J.1466-8238.2012.00789.X
Wallace, J. S., Garner, E., Pruden, A., and Aga, D. S. (2018). Occurrence and transformation of veterinary antibiotics and antibiotic resistance genes in dairy manure treated by advanced anaerobic digestion and conventional treatment methods. Environ. Pollut. 236, 764–772. doi:10.1016/j.envpol.2018.02.024
Wang, F., Han, W., Chen, S., Dong, W., Qiao, M., Hu, C., et al. (2020). Fifteen-year application of manure and chemical fertilizers differently impacts soil ARGs and microbial community structure. Front. Microbiol. 11, 62. doi:10.3389/fmicb.2020.00062
WHO (2018a). Critically important antimicrobials for human medicine. Available at: https://apps.who.int/iris/bitstream/handle/10665/312266/9789241515528-eng.pdf (Accessed June 1, 2023).
WHO (2018b). Antibiotic resistance world health organization. Available at: https://www.who.int/news-room/fact-sheets/detail/antibiotic-resistance (Accessed March 19, 2019).
Wicks, M., and Keener, H. (2017). Manure processing technologies 3.2 separation | manure processing technologies 3.2 separation what is separation?
Wohde, M., Berkner, S., Junker, T., Konradi, S., Schwarz, L., and Düring, R. A. (2016). Occurrence and transformation of veterinary pharmaceuticals and biocides in manure: a literature review. Environ. Sci. Eur. 28, 23. doi:10.1186/s12302-016-0091-8
Wood, D. E., Lu, J., and Langmead, B. (2019). Improved metagenomic analysis with Kraken 2. Genome Biol. 20, 257–313. doi:10.1186/s13059-019-1891-0
Wu, X., Tian, Z., Lv, Z., Chen, Z., Liu, Y., Yong, X., et al. (2020). Effects of copper salts on performance, antibiotic resistance genes, and microbial community during thermophilic anaerobic digestion of swine manure. Bioresour. Technol. 300, 122728. doi:10.1016/j.biortech.2019.122728
Xu, M., Stedtfeld, R. D., Wang, F., Hashsham, S. A., Song, Y., Chuang, Y., et al. (2019). Composting increased persistence of manure-borne antibiotic resistance genes in soils with different fertilization history. Sci. Total Environ. 689, 1172–1180. doi:10.1016/j.scitotenv.2019.06.376
Zhang, J., Lu, T., Shen, P., Sui, Q., Zhong, H., Liu, J., et al. (2019). The role of substrate types and substrate microbial community on the fate of antibiotic resistance genes during anaerobic digestion. Chemosphere 229, 461–470. doi:10.1016/j.chemosphere.2019.05.036
Zhang, L., Li, L., Sha, G., Liu, C., Wang, Z., and Wang, L. (2020). Aerobic composting as an effective cow manure management strategy for reducing the dissemination of antibiotic resistance genes: an integrated meta-omics study. J. Hazard Mater 386, 121895. doi:10.1016/j.jhazmat.2019.121895
Zhang, R. M., Liu, X., Wang, S. L., Fang, L. X., Sun, J., Liu, Y. H., et al. (2021). Distribution patterns of antibiotic resistance genes and their bacterial hosts in pig farm wastewater treatment systems and soil fertilized with pig manure. Sci. Total Environ. 758, 143654. doi:10.1016/j.scitotenv.2020.143654
Zhang, T., Zhang, X. X., and Ye, L. (2011). Plasmid metagenome reveals high levels of antibiotic resistance genes and mobile genetic elements in activated sludge. PLoS One 6, 26041. doi:10.1371/journal.pone.0026041
Keywords: manure bedding recovery, manure management, antimicrobial resistance, microbial dynamics, metagenomics
Citation: Flores-Orozco D, Levin D, Kumar A, Sparling R and Cicek N (2023) Influence of three different manure treatments on antimicrobial resistance genes and mobile genetic elements. Front. Synth. Biol. 1:1301879. doi: 10.3389/fsybi.2023.1301879
Received: 25 September 2023; Accepted: 20 November 2023;
Published: 04 December 2023.
Edited by:
Na Wei, University of Illinois at Urbana-Champaign, United StatesReviewed by:
Ying Wu, Fred Hutchinson Cancer Center, United StatesYiran Dong, China University of Geosciences Wuhan, China
Copyright © 2023 Flores-Orozco, Levin, Kumar, Sparling and Cicek. This is an open-access article distributed under the terms of the Creative Commons Attribution License (CC BY). The use, distribution or reproduction in other forums is permitted, provided the original author(s) and the copyright owner(s) are credited and that the original publication in this journal is cited, in accordance with accepted academic practice. No use, distribution or reproduction is permitted which does not comply with these terms.
*Correspondence: David Levin, david.levin@umanitoba.ca; Nazim Cicek, nazim.cicek@umanitoba.ca