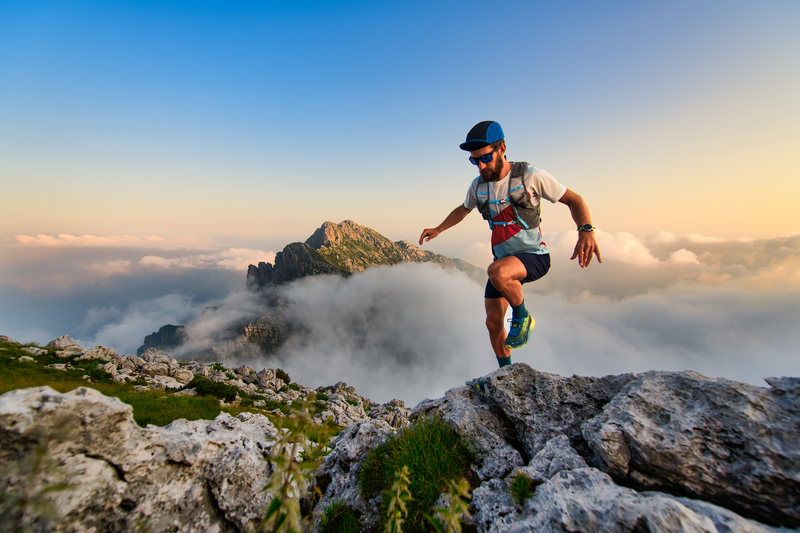
94% of researchers rate our articles as excellent or good
Learn more about the work of our research integrity team to safeguard the quality of each article we publish.
Find out more
ORIGINAL RESEARCH article
Front. Sustain. Tour. , 17 December 2024
Sec. Tourism, Climate and Global Environmental Consequences
Volume 3 - 2024 | https://doi.org/10.3389/frsut.2024.1446528
Accurate evaluation of the spatiotemporal variability of people's interest in seasons (i.e., their “sense of seasons”) is required to deeply understand temporal changes in the relationship between people and landscape under climate and societal changes. To this end, it is useful to analyze the time series of relative search volume (RSV) for relevant search terms extracted from Google Trends (GT) for any target period and target area. We examined the presence and absence of 12-month seasonality of the time series of RSV for about 300 representative search terms for Hokkaido in Japan, which has vast natural resources and is visited year-round by many tourists from Japan and abroad. In the case of searchers in both all of Japan and Hokkaido alone, search terms categorized into the foods, leisure, natural scenic spots, and seafood fields showed a strong trend toward 12-month seasonality of the time series of RSV, whereas those categorized into the Ainu (indigenous people) and people fields had weak trends for 12-month seasonality. People's sense of seasons in all of Japan or Hokkaido alone was likely associated with tourism (search terms in the animals, festivals, foods, leisure, natural scenic spots, seafood, tourist spots, and vegetation fields), as well as with animal and plant phenology (terms in the animals and vegetation fields), provisioning ecosystem services (terms in the foods and seafood fields), climate (terms in the climate field), and cultural ecosystem services (terms in the animals, festival, foods, leisure, natural scenic spots, seafoods, and vegetation fields). However, search terms with no 12-month seasonality were included in the tourist spots fields: they included many tourist spots visited by tourists all year around. Despite the need to appropriately select a huge number of representative search terms to reduce the effect of GT limitations and the uncertainty caused by unexpected and rapid temporal changes in RSV, the GT analysis allowed us to evaluate the spatiotemporal variability of people's sense of seasons regarding various objects in the landscape, including those related to tourism, under climate and societal changes.
To understand the temporal changes in the relationship between people and landscape in the face of climate and societal changes, we require accurate evaluation of the spatiotemporal variability of people's interest in seasons (hereafter, their “sense of seasons”). A landscape consists of multiple layers, such as the atmosphere, water, living things, nutrients, livelihoods, society, and information that change seasonally and annually (Shin et al., 2024). In prehistoric times, when people obtained various kinds of seasonal foods, clothes, and residences through the hunting and gathering of animals and plants, people's sense of seasons was likely associated with plant and animal phenology (in relation to provisioning ecosystem services) and climate. From the agricultural era onward, when people lived their daily lives according to the crop calendar, people's sense of seasons likely became associated with not only traditional plant and animal phenology and climate but also beliefs and religious ceremonies (cultural ecosystem services) as society and philosophical thought developed. Currently, direct interaction between people and nature has decreased markedly because of urbanization and globalization. However, tourism is expanding as people seek to enjoy beautiful natural scenery, gourmet foods, festivals and events, presentations of artworks, and leisure. The recent spread and development of the Internet—especially social networking services and online video-sharing platforms such as YouTube—have accelerated the quality and diversity of tourism. Hence, people's sense of seasons never fades, but rather creates new values (i.e., cultural ecosystem services). These new values have improved people's lives and enhanced their mental calm. Ultimately, tourism is likely becoming important to people's sense of seasons in the landscape.
Stephens-Davidowitz and Varian (2015) indicated the utility of Google Trends (hereafter “GT”; Google LLC, 2024) for the quantitative evaluation of temporal changes in people's interests. We can retrieve the temporal changes in people's interests in any search term in any target period (from 2004 to the current day) and target area (country or local administrative area) as a time series of the relative search volume (RSV). By analyzing the time series of RSV, we can detect long-term trends, interannual changes in the seasonal peak, and unexpected and rapid temporal changes—stirring—of people's interests. Conventional examinations of people's sense of seasons have been limited to questionnaire surveys and interviews (e.g., Naumov et al., 2020; Gotow et al., 2022; Régnier et al., 2022). In labor-intensive interviews, it is difficult to gather data on various kinds of items in multiple locations for long periods of time. For this reason, the sampling data of collected by conventional questionnaire surveys and interviews is problematic, because they include uncertainty caused by representational (i.e., sampling limitation and bias), spatial, and temporal gaps (Shin et al., 2024). In contrast, GT has merit in that we can use it to examine the spatiotemporal variability of people's interests with sufficient representativeness, because the time series of the RSV for any search term is retrieved from sampling data that are part of a larger population. Many previous analyses of the time series of RSV can be found in infodemiology (e.g., influenza: Dugas et al., 2013; Kang et al., 2013; Yang et al., 2015; COVID-19: Mavragani and Gkillas, 2020; Anastasiou et al., 2021; Amusa et al., 2022; melanoma: Zhang et al., 2021) and in human activities and economics under COVID-19 (Ahundjanov et al., 2020; Lee, 2020; Strzelecki et al., 2020; Brimblecombe and Lai, 2021; Kardeş, 2021). However, there is only a limited number of such studies of people's sense of seasons (timing of appearance of insects: Takada, 2012; usage of berries: Kotani et al., 2021; Shin et al., 2022a; cherry-flowering phenology: Shin et al., 2022b; demand for mushrooms: Diaz-Balteiro et al., 2023; fashion: Silva et al., 2019). In addition, Shin et al. (2024) examined people's sense of seasons in Russia by using multiple search terms (dozens or more) categorized into various fields, but they did not provide a detailed consideration of their results.
To develop a method for evaluating people's sense of seasons by using GT, here, as a case study, we examined the presence and absence of 12-month seasonality of the time series of RSV for about 300 search terms that were categorized into various fields and that represented Hokkaido in Japan. We can expect nature's contributions to people (Díaz et al., 2018) to be large in Hokkaido, which is endowed with an abundance of natural features. Many people from Japan and abroad visit Hokkaido throughout the year. For these reasons, we considered Hokkaido to be a suitable area for validating our study aims. In addition, for us to understand the background of people's sense of seasons, it was important to be familiar with the geography, traditions, and culture of the target area. For this reason, we selected our native country, Japan. Our aims were to clarify (1) the search terms with 12-month seasonality and the characteristics of their seasonal peaks; and (2) the usefulness, limitations, and perspectives of evaluating people's sense of seasons by using GT.
Our target area was Hokkaido in northern Japan, which accounts for about 22% of the country's total area (83,424 km2 as of 2020; Figure 1; Hokkaido Prefecture, 2023b). Indigenous Ainu people created their own lifestyle and culture in this area fifteen centuries ago, and Hokkaido was called Ezo by the Japanese people during this period. After fifteen centuries, interaction, trade, and conflict arose between Ainu and the Japanese people. In 1869, the Japanese government established the land development bureau and renamed the area Ezo Hokkaido (Hokkaido Regional Development Bureau, Ministry of Land, Infrastructure, Transport and Tourism, 2021). The population of Hokkaido is about 5.09 million (as of 1 January 2024; Hokkaido Prefecture, 2024a), of whom 13,000 identify as Ainu (as of 2017; Comprehensive Ainu Policy Office, Cabinet Secretariat, Government of Japan, 2012). Primary industry employs 4.7% of workers in Hokkaido, secondary industry 17.6%, and tertiary industry 73.9% (as of 2017; Hokkaido Prefecture, 2023a). Hokkaido, which is classified as Dfb (warm-summer humid continental climate) according to the Köppen climate classification, has four distinct seasons. However, climate varies locally because of the influence of the sea and mountains (Japan Meteorological Agency, 2024). Agricultural land (rice paddies and cropland) and natural forests (mixed forests of deciduous broad-leaved trees and evergreen coniferous trees) are widely distributed in Hokkaido, except in the metropolitan areas. Many people from Japan and abroad visit Hokkaido for recreation in the natural environment or to savor the abundant seafood and other gourmet foods, enjoy seasonal leisure activities, and attend festivals: a total of 46.01 million people from Hokkaido traveled within the prefecture in 2018, along with a total of 6.07 million Japanese people from outside Hokkaido and a total of 3.12 million people from overseas (before the COVID-19 era; Hokkaido Prefecture, 2024b).
Figure 1. (A) Location and (B) topographic map of Hokkaido in Japan. The map was sourced from Natural Earth's 1:10 m cultural vectors (Natural Earth, 2024) and Global 30 Arc-Second Elevation (GTOPO30; USGS, 2024).
We examined a total of 319 search terms representing Hokkaido. They were categorized into 11 fields (Ainu, animals, climate, festivals, foods, leisure, natural scenic spots, people, seafood, tourist spots, and vegetation). The number of search terms in each field ranged from 11 (climate and leisure) to 44 (foods). The average number of search terms per field was 29. To accurately evaluate the sense of seasons in Hokkaido, we assumed that we needed to select search terms that were both specific to Hokkaido and common to other regions. These terms were selected by coauthor HN, who is a native of Hokkaido. She selected these terms in as unbiased a way as possible by using her experience and knowledge, as well as relevant Internet websites. The location of each search term categorized into the festivals, natural scenic spots, and tourist spots fields is shown in Supplementary Figure 1.
In GT, a search can be done in two ways, namely (a) by “topic” (a group of terms preliminarily registered by Google that share the same concept in any language) or (b) by “keyword” (a literal term that includes data only from the language that is selected). To clarify the target field of the search term, we selected the “topic” search as much as possible. For instance, when we searched for “cherry blossom” as vegetation, we selected the “topic” search in order to remove people's interest in items other than vegetation, such as people's names and product names. When we could not select a “topic” search (i.e., when there was no preselected GT registration of the corresponding topic), we selected the “keyword” search. The accuracy of GT has been developed through three specification changes (1 January 2011; 1 January 2016; and 1 January 2022; Google LLC, 2024). We set the search period from 1 January 2018 to 31 December 2022. Therefore, the time series of RSVx (x = 1, 2, 3, …., 319) at a weekly time step consisted of 260 values (0 ≤ RSVx ≤ 100).
To validate the characteristics of these RSVx values that were associated with the geographic distribution of Google searchers, we set up two search areas: (1) the whole of Japan (henceforth referred to simply as “Japan”) and (2) Hokkaido only. We obtained 308 time series of RSVx for searchers in Japan and 301 time series of RSVx for searchers in Hokkaido. To confirm the validity of the RSVx values for each search term, we checked three attributes of the RSVx values, namely “Interested by sub-region,” “Top related topics,” and “Top related queries.” We checked the top 5 for each of the attributes (please see details in Shin et al., 2022a; Google LLC, 2024).
Previous studies have reported that systematic error of RSV values can be caused by differences in the search dates (Rovetta, 2021; Cebrián and Domenech, 2023). However, we assumed that this error would not have a substantial influence on the results of our analysis because we evaluated only the presence and absence of 12-month seasonality of the time series of RSV. Each term was accessed only once from 11 June 2023 to 3 July 2023.
To detect the presence and absence of 12-month seasonality of the time series of RSVx, we calculated the autocorrelation coefficient explained by Equation 1, where s is the time lag in weeks and t is the time in weeks.
where n = 260 (52 weeks × 5 years) and .
When a statistically significant peak value of the autocorrelation coefficient occurred at about s = 51, 52, or 53, we defined the time series of RSVx as having 12-month seasonality. Typical examples of the presence and absence of 12-month seasonality are shown in Supplementary Figure 2. The peak season of the time series of RSVx was detected by visual inspection. The analysis was conducted in R ver. 3.6.3 (The R Foundation, 2024) and LibreOffice ver. 7.5.0.3.
Table 1 shows the presence and absence of 12-month seasonality for each search term. In the case of searchers in both Japan and Hokkaido combined, the number of search terms with 12-month seasonality (total from the “topic” and “keyword” searches combined) in the Ainu field was 0; animals, 2; climate, 2; festivals, 4; foods, 12; leisure, 4; natural scenic spots, 14; people, 0, seafood, 15; tourist spots, 7; and vegetation, 8. In the case of searchers in either Japan or Hokkaido, some search terms with 12-month seasonality were found. The total number of search terms in the “topic” search was 250. The percentage of search terms in the “topic” search for each field ranged from 45% (climate and festivals) to 98% (natural scenic spots). The average was 75% and the standard deviation (SD) was 19%.
Table 1. Presence and absence of 12-month seasonality of the time-series of relative search volume for each search term.
We cross-tabulated the presence and absence of 12-month seasonality of the time series of RSV categorized into the 11 fields (Figure 2). In the case of searchers in Japan, the percentage of search terms with 12-month seasonality ranged from 0% (Ainu) to 64% (climate). The average was 34% (SD = 19%). In the case of searchers in Hokkaido, the percentage of search terms with 12-month seasonality ranged from 0% (people) to 52% (seafood). The average was 28% (SD = 16%). In the case of both searchers in Japan and searchers in Hokkaido, the percentages of search terms with 12-month seasonality in the foods, leisure, natural scenic spots, and seafood fields were 33% or more. The percentages of search terms with 12-month seasonality in the animals, climate, festivals, and vegetation fields were higher among searchers in Japan than among those in Hokkaido. In the case of both searchers in Japan and searchers in Hokkaido, the percentage of search terms with 12-month seasonality in the Ainu field was either zero or under 11%; this was also the case in the people field.
Figure 2. Cross-tabulation of the 12-month seasonality of the time series of RSV in (A) Japan, (B) Hokkaido for search terms categorized into 11 fields. AIN, Ainu; ANI, animals; CLI, climate; FES, festivals; FOO, foods; LEI, leisure; NSS, natural scenic spots; PEO, people; SEA, seafood; TOU, tourist spots; VEG, vegetation. Values on the figure show the percentages of search terms with or without 12-month seasonality in each field.
We examined the peak seasons of the time series of RSVx for each search term in the case of searchers in Japan (Table 2) and those in Hokkaido (Table 3). The following eight characteristics were observed: (1) RSV peaks in the animals (mainly in the case of searchers in Japan), festivals, and tourist spots fields were observed in three seasons: December–February, March–May, and June–August. (2) RSV peaks in the climate field were observed in two seasons: December–February and September–November. (3) RSV peaks in the foods and seafood fields were observed in all seasons. (4) RSV peaks in the natural scenic spots field were observed mainly in two seasons: June–August and September–November. (5) RSV peaks in the leisure field were observed in two seasons: December–February and June–August. (6) RSV peaks in the vegetation field were observed in three seasons: March–May, June–August, and September–November. (7) In the case of searchers in either Japan or Hokkaido, RSV peaks for several search terms (Lake Akan and Mt. Hakodate in the natural scenic spots field; Taiho Koki in the people field; Abashiri Prison Museum, Northern Horse Park, and Ekorin Village in the tourist spots field; salmon in the seafood field; and Japanese white birch in the vegetation field) were observed in multiple seasons. (8) The number of search terms with obscure or unknown RSV peak seasons was greater in the case of searchers in Japan than for searchers in Hokkaido.
Table 2. Peak seasons of the time series of relative search volume for each search term in the case of searchers in the whole of Japan.
Table 3. Peak seasons of the time series of relative search volume for each search term in the case of searchers in Hokkaido only.
In the case of both searchers in Japan and those in Hokkaido, a high trend was observed in the percentages of search terms with 12-month seasonality in the foods, leisure, natural scenic spots, and seafood fields, whereas a low trend was observed in the percentages in the Ainu and people fields (Figure 2, Table 1). The RSV peak seasons in each field (Tables 2, 3) may have been associated with the following. Search terms categorized into the animals field were likely associated with the peak appearance of each animal. Search terms categorized into the climate field were likely associated with snowfall (studless tires, snow shovel, road heating, powder snow, and ice burn), coldness (stoves and water pipes), and winter events (drift ice). Search terms categorized into the festivals field were likely associated with the dates of each festival (see details in Supplementary Table 4). Search terms categorized into the foods field were likely associated with the dates of traditional events (New Year's Eve: dried fileted herring and soba noodle soup with herring; New Year: izushi fermented sushi and kazunoko herring roe; Valentine's Day: nama chocolate ganache), harvest time (alpine leek, Densuke watermelon, Yubari melon, and beetroot), and seasonal foods (e.g., Matsumae pickles, ishikari-nabe Hokkaido salmon hot pot, chanchan yaki Hokkaido-style salmon and vegetable hotpot, and sujiko salmon roe). Despite advances in refrigeration and transportation technology, as well as increased satiation and globalization, it was interesting to find that a clear sense of seasons was observed for search terms associated with traditional food culture (e.g., kazunoko herring roe and izushi fermented sushi). Search terms categorized into the leisure field were likely associated with summer (rafting) and winter (skiing, snowboarding, snowshoeing, and ice hockey) sports. Search terms categorized into the natural scenic spots field were likely associated with the high tourism seasons (summer and autumn). Searches for Taiho Koki (a highest-ranking yokozuna sumo wrestler from Hokkaido, 1940–2013), categorized into the people field, were likely associated with the period of the year when the six annual sumo tournaments are held (each lasting 15 days). Many search terms categorized into the seafood field were likely associated with the peak commercial fishing season (see details in Supplementary Table 5). Search terms categorized into the tourist spots field were likely associated with Shinto ritual (Hokkaido Shrine), winter events (cruises of the icebreaker Garinko II), a cherry flowering spot (Nijukken Road), a moss phlox flowering spot (Higashimokoto Shibazakura Park), and high tourism seasons (spring and summer). Search terms categorized into the vegetation field were likely associated with flowering (all search terms, excluding Japanese white birch and ginkgo), new green leaves (Japanese white birch), and leaf coloring (Japanese white birch and ginkgo).
People's sense of seasons may have been associated with a variety of objects in the landscape. The search terms with 12-month seasonality in the animals and vegetation fields were likely associated mainly with animal and plant phenology and cultural ecosystem services. Those in the climate field were associated mainly with climate. Those in the festivals field were likely associated mainly with cultural ecosystem services. Those in the foods and seafood fields likely associated mainly with the provisioning ecosystem services caused by animal and plant phenology, and with cultural ecosystem services. Those in the leisure, natural scenic spots, and tourist spots field were likely associated mainly with tourism. The leisure and natural scenic spots fields also included search terms that were likely associated with cultural ecosystem services.
Notably, the search terms with 12-month seasonality in the animals, festivals, foods, seafood, and vegetation fields also appeared to be associated with tourism for the enjoyment of nature, living things, culture, festivals, and gourmet foods. All of these findings suggest that the spatiotemporal variability of people's sense of seasons in Hokkaido is derived from tourism, as well as from animal and plant phenology, provisioning ecosystem services, cultural ecosystem services, and climate. There were, however, search terms with no 12-month seasonality in the tourist spots field; they included many tourist spots visited by tourists all year around. In addition, people have a low sense of seasons in the Ainu and people fields in Hokkaido, despite the inclusion of tourism factors in these fields.
Overall, the time series of RSV detected as RSV peak seasons had the following three patterns (Supplementary Figure 3). Type 1: Rapid increases and decreases were observed in a short period of time (mainly in the festivals and climate fields, and partly in the foods field). This may have been caused by the fact that festival events and the consumption of special foods in relation to events are limited to short periods. In addition, drift ice and ice burn (in the climate field) are natural phenomena that occur over short periods of time. Type 2: Bell-shaped increases and decreases were observed in certain periods (mainly in the animals, climate, foods, seafood, and vegetation fields). This may have been caused by the fact that the peak of appearance of animals, the initial yearly need for cold protection, the peak commercial fishing season, and flowering are observed over periods of 1 week to 1 month. Type 3: Compared with type 2, more gradual increases and decreases, with mountain-shaped peaks in the graph, were observed (mainly in the natural scenic spots field and partly in the tourist spots field). This may have resulted from the fact that the high seasons for natural scenic and tourist spots generally continue for multiple months.
Although 12-month seasonality was detected in our analysis of the autocorrelation coefficient of the time series of RSV, there were cases in which an RSV peak season could not be detected successfully by visual inspection (Tables 2, 3). This may have been caused by the following. The time series of RSV included 12-month periodic components. However, it had a complex pattern because it included non-periodic components. As a result, in these cases it was difficult to detect the RSV peak season. In contrast, in other cases, although 12-month seasonality in multiple years was detectable by visual inspection, some search terms did not detect it through analysis of the autocorrelation coefficient (examples are, in the case of searchers in Japan: heavy snow categorized into the climate field, and Toyohira River Fireworks Festival, Hakodate Port Festival, and Yosakoi Soran Festival categorized into the festivals field; in the case of searchers in Hokkaido: Otaru Tide Festival, Katsumai Fireworks Festival, and Hakodate Port Festival categorized into the festivals field, and Asahiyama Zoo categorized into the tourist spots field; these terms therefore do not appear in Tables 2, 3). Many of these time series of RSV did not show clear peak seasons in 2020 and 2021. This may have been caused by a substantial decrease in the number of Google searches because of the cancellation of festivals and events during the COVID-19 epidemic.
Unexpected and rapid temporal changes in RSV values in a short time period with no relationship to 12-month seasonality may have hindered our detection of the 12-month periodic component of the time series of RSV. The time series of RSV for the Shiretoko Peninsula (categorized into the natural scenic spots field) had an unexpected and rapid increase from 24 April to 30 April 2022 because of a marine accident (Fire Disaster Management Agency, 2022). For this reason, the 12-month periodic component that peaked in about August every year was not detected by our analysis of the autocorrelation coefficient. A rapid increase in people's interest in a short time period often occurs in response to movies, TV programs, animations, and news reports. We can guess the reason for unexpected and rapid temporal changes in the RSV values to some extent by carefully validating the attributes of RSV values (i.e., “Top related topics” and “Top related queries”; Shin et al., 2022a). However, in the case of the analysis of huge numbers of search terms, it is unrealistic to verify all attributes. For this reason, the inclusion of unexpected and rapid temporal changes in RSV values that have no relationship to 12-month seasonality (i.e., that can be considered to be systematic errors) will mislead any evaluation of people's sense of seasons.
Our approach included the following three limitations on the representativeness of selected search terms: (1) The terms included bias associated with the GT searcher's generation, gender, knowledge, experience, and skill. (2) The terms included various levels of specificity and generality. (3) We could not identify both the attributes of Google searchers (e.g., generation, gender, and nationality) and the absolute, rather than relative, volumes of Google searchers. Regarding the first limitation, comprehensive investigation of printed guidebooks and brochures and of the websites of tourism associations and local governments may be preferable. However, the information retrieved from these media also includes bias due to the intent or purpose of the publisher or sender and the availability of printed pages. Regarding the second limitation, in a certain search area, more specific terms are likely easier to observe for 12-month seasonality, whereas more general terms may make it difficult to detect such patterns. In fact, the percentages of search terms with 12-month seasonality in the animals, climate, and festivals fields in the case of searchers in Japan were higher than those in the case of searchers in Hokkaido (Figure 2). This may have been because these fields included typical search terms with 12-month seasonality in areas other than Hokkaido (e.g., small stag beetle in the animals field, and rose of Sharon in the vegetation field; Table 1). By referring to the “Interest by sub-region” attribute (e.g., Japanese tree frog in Akita, Gifu, and Tottori, and snow shovel in Akita, Yamagata, and Niigata), we confirmed that the top 5 areas of search terms included areas other than Hokkaido. In other words, these search terms were not specific to Hokkaido. Regarding the third limitation, a search term with high 12-month seasonality but a low search volume (small population) may not be as significant as a search term with low 12-month seasonality but a high search volume (large population). In the case of Google searchers in Hokkaido, the RSV values might have included the search results from both local people in Hokkaido and many tourists from Japan and abroad. Moreover, with regard to the second limitation, the sensitivity of the sense of seasons may vary between local people in Hokkaido and both tourists and Japanese people residing outside Hokkaido. For this reason, in some cases, it is difficult to use search terms with 12-month seasonality to accurately define the sense of seasons of local people, specifically, in Hokkaido. To remove these three limitations as far as possible, increasing the number of search terms is of primary importance from a statistical perspective, because increasing the sample size causes the sample mean to converge to the population mean (Law of Large Numbers). This would require an automatic analysis system that identifies huge numbers of representative search terms on the Internet and then examines the 12-month seasonality of downloaded time series of RSV values for each search term.
On GT, we can extract the time series of RSV at a daily time step by setting the search period to within 8 months (Google LLC, 2024). For this reason, year-to-year variability in the RSV peak season could be examined accurately by extracting the RSV values every year for the same period. In fact, the daily RSV time series of blossoming of the three Great Cherry Trees of Japan show year-to-year variability of the RSV peak season, which is caused by variations in the dates of full bloom (Shin et al., 2022b). The time series of RSV associated with the peak commercial fishing season, the peak of appearance of animals, and flowering can be viewed as proxy data for animal and plant phenology. The time series of RSV associated with seasonal gourmet food consumption, leisure, and visits to natural scenic and tourist spots can be seen as proxy data for seasonal human activities. An analysis of the year-to-year variability of the RSV peak for various search terms in multiple countries and regions could deepen our understanding of the sensitivities of animal and plant phenology and seasonal human activities to climate change. The analysis of texts on X and Facebook, of videos on Instagram, TikTok, and YouTube, and of human flow data with time stamp and geolocation information will provide supporting evidence of animal and plant phenology and seasonal human activities. By verifying these data, we will be able to improve the accuracy of our evaluation of people's sense of seasons by using GT.
Our analysis of the time series of RSV for over 300 search terms representing Hokkaido, in Japan, allowed us to detect the presence and absence of 12-month seasonality and the characteristics of RSV peak seasons. In the case of searchers in both Hokkaido and the whole of Japan, many search terms with 12-month seasonality were observed in the foods, leisure, natural scenic spots, and seafood fields. In contrast, search terms with 12-month seasonality were barely observed in the Ainu and people fields. In Hokkaido, people's sense of seasons was likely associated with tourism, as well as with animal and plant phenology, provisioning ecosystem services, climate, and cultural ecosystem services. However, unexpected and rapid temporal RSV value changes that had no relationship to seasonal changes, together with limitations on the representativeness of selected search terms, caused uncertainty in the evaluation of people's sense of seasons on the basis of the time series of RSV. Although there remains a need for the appropriate selection of huge numbers of representative search terms, we recommend further studies of people's sense of seasons by using GT of various search terms in countries and regions of interest to deepen our understanding of the temporal changes in the relationship between people and various objects of landscape. From the perspective of people's sense of seasons, we will be able to evaluate the spatiotemporal variability of the relationship between people and tourism under climate and societal changes.
Publicly available datasets were analyzed in this study. This data can be found at: https://trends.google.com/trends/.
NS: Conceptualization, Data curation, Formal analysis, Funding acquisition, Investigation, Methodology, Validation, Visualization, Writing – original draft. HN: Data curation, Investigation, Validation, Writing – review & editing. HM: Methodology, Writing – review & editing. AK: Validation, Writing – original draft.
The author(s) declare financial support was received for the research, authorship, and/or publication of this article. This research was supported by KAKENHI grants (JP21H05178, JP22H04457, and JP24H00935) from the Japan Society for the Promotion of Science.
We thank the editors and two reviewers for their constructive comments.
The authors declare that the research was conducted in the absence of any commercial or financial relationships that could be construed as a potential conflict of interest.
All claims expressed in this article are solely those of the authors and do not necessarily represent those of their affiliated organizations, or those of the publisher, the editors and the reviewers. Any product that may be evaluated in this article, or claim that may be made by its manufacturer, is not guaranteed or endorsed by the publisher.
The Supplementary Material for this article can be found online at: https://www.frontiersin.org/articles/10.3389/frsut.2024.1446528/full#supplementary-material
Supplementary Figure 1 | Location maps of search terms categorized into the (A) festivals, (B) natural scenic spots, and (C) tourist spots fields. The correspondence between numbers and search terms on each map is shown in (A) Supplementary Table 1, (B) Supplementary Table 2, (C) Supplementary Table 3.
Supplementary Figure 2 | Examples of the time series of relative search volume (RSV) and its autocorrelation coefficient with 12-month seasonality (A, C) and with no 12-month seasonality (B, D). The red and green dotted lines show 95% significance and time lag (s) = 52, respectively.
Supplementary Figure 3 | Examples of the three patterns of the peak season of the times series of relative search volume (RSV): (A) type 1, (B) type 2, and (C) type 3. See text for details.
Supplementary Table 1 | List of the correspondence between numbers on the map in Supplementary Figure 1A and search terms in the festivals field.
Supplementary Table 2 | List of the correspondence between numbers on the map in Supplementary Figure 1B and search terms in the natural scenic spots field.
Supplementary Table 3 | List of the correspondence between numbers on the map in Supplementary Figure 1C and search terms in the tourist spots field.
Supplementary Table 4 | List of relevant websites and event dates for search terms categorized into the festivals field. All relevant websites were accessed on 6 September 2024. (J) Results for searchers in the whole of Japan; (H) results for searchers in Hokkaido only; (JH) results for searchers in both areas combined.
Supplementary Table 5 | List of the commercial fishing seasons for search terms categorized into the seafood field. (J) Results for searchers in the whole of Japan; (H) results for searchers in Hokkaido only; (JH) results for searchers in both areas combined.
Ahundjanov, B. B., Akhundjanov, S. B., and Okhunjanov, B. B. (2020). Information search and financial markets under COVID-19. Entropy 22:791. doi: 10.3390/e22070791
Amusa, L. B., Twinomurinzi, H., and Okonkwo, C. W. (2022). Modeling COVID-19 incidence with google trends. Front. Res. Metr. Anal. 7:1003972. doi: 10.3389/frma.2022.1003972
Anastasiou, M., Pantavou, K., Yiallourou, A., Bonovas, S., and Nikolopoulos, G. K. (2021). Trends of online search of COVID-19 related terms in Cyprus. Epidemiologia 2, 36–45. doi: 10.3390/epidemiologia2010004
Brimblecombe, P., and Lai, Y. (2021). Subtle changes or dramatic perceptions of air pollution in Sydney during COVID-19. Environments 8:2. doi: 10.3390/environments8010002
Cebrián, E., and Domenech, J. (2023). Is Google Trends a quality data source? Appl. Econ. Lett. 30, 811–815. doi: 10.1080/13504851.2021.2023088
Comprehensive Ainu Policy Office Cabinet Secretariat, Government of Japan. (2012). Summary of a Policy of Ainu. Available at: https://www.kantei.go.jp/jp/singi/ainusuishin/policy.html (accessed September 24, 2024).
Díaz, S., Pascual, U., Stenseke, M., Martín-López, B., Watson, R. T., Molnár, Z., et al. (2018). Assessing nature's contributions to people. Science 359, 270–272. doi: 10.1126/science.aap8826
Diaz-Balteiro, L., Alfranca, O., Voces, R., and Solinõ, M. (2023). Using google search patterns to explain the demand for wild edible mushrooms. For. Pol. Econ. 152:102993. doi: 10.1016/j.forpol.2023.102993
Dugas, A. F., Jalalpour, M., Gel, Y., Levin, S., Torcaso, F., Igusa, T., et al. (2013). Influenza forecasting with Google Flu Trends. PLoS ONE 8:e56176. doi: 10.1371/journal.pone.0056176
Fire Disaster Management Agency (2022). Tourist Boat Accident Off the Coast of Abashiri, Hokkaido (12 May 2022). Available at: https://www.fdma.go.jp/disaster/info/items/6b20c7c9f01bb6bf0e5eee8be693f985726c02ae.pdf (accessed September 24, 2024).
Google LLC (2024). Google Trends Web. Available at: https://trends.google.com/trends/ (accessed September 24, 2024).
Gotow, N., Nagai, Y., Taguchi, T., Kino, Y., Ogino, H., and Kobayakawa, T. (2022). Nostalgia evocation through seasonalityconscious purchasing behavior revealed by online survey using vegetable names. Sci. Rep. 12:5568. doi: 10.1038/s41598-022-09485-2
Hokkaido Prefecture (2023a). Economy in Hokkaido Data Book in 2020. Available at: https://www.pref.hokkaido.lg.jp/ss/tkk/databook/2020/0301.html (accessed September 24, 2024).
Hokkaido Prefecture (2023b). Geography in Hokkaido Data Book in 2021. Available at: https://www.pref.hokkaido.lg.jp/ss/tkk/databook/76967.html (accessed September 24, 2024).
Hokkaido Prefecture (2024a). Population and Households. Available at: https://www.pref.hokkaido.lg.jp/ss/tuk/900brr/index2.html (accessed September 24, 2024).
Hokkaido Prefecture (2024b). Survey Report on the Number of Tourists in Hokkaido. Available at: https://www.pref.hokkaido.lg.jp/kz/kkd/irikomi.html (accessed September 24, 2024).
Hokkaido Regional Development Bureau Ministry of Land, Infrastructure, Transport and Tourism. (2021). History of Hokkaido Development. Available at: https://www.hkd.mlit.go.jp/ky/ki/kouhou/70th/history/00-01.html (accessed September 24, 2024).
Japan Meteorological Agency (2024). Characteristics of the Weather in Hokkaido. Available at: https://www.data.jma.go.jp/cpd/j_climate/hokkaido/main.html (accessed September 24, 2024).
Kang, M., Zhong, H., He, J., Rutherford, S., and Yang, F. (2013). Using Google Trends for influenza surveillance in South China. PLoS ONE 8:e55205. doi: 10.1371/journal.pone.0055205
Kardeş, S. (2021). Public interest in spa therapy during the COVID-19 pandemic: analysis of Google Trends data among Turkey. Int. J. Biometeorol. 65, 945–950. doi: 10.1007/s00484-021-02077-1
Kotani, A., Shin, N., Tei, S., Makarov, A., and Gavrilyeva, T. (2021). Seasonality in human interest in berry plants detection by google trends. Front. For. Glob. Change 4:688835. doi: 10.3389/ffgc.2021.688835
Lee, H. S. (2020). Exploring the initial impact of COVID-19 sentiment on US stock market using big data. Sustainability 12:6648. doi: 10.3390/su12166648
Mavragani, A., and Gkillas, K. (2020). COVID-19 predictability in the United States using Google Trends time series. Sci. Rep. 10:20693. doi: 10.1038/s41598-020-77275-9
Natural Earth (2024). 1:10m Cultural Vectors. Available at: https://www.naturalearthdata.com/downloads/10m-cultural-vectors/ (accessed September 24, 2024).
Naumov, A., Akimova, V., Elmanova, D., Velichko, E., Topnikov, M., Volkov, M., et al. (2020). Role of seasonality in the socio-economic development of rural areas of the southern part of the Republic of Karelia. Miscellanea Geogr. 24:51–61. doi: 10.2478/mgrsd-2020-0015
Régnier, F., Dalstein, A.-L., Rouballay, C., and Chauvel, L. (2022). Eating in season-A lever of sustainability? an interview study on the social perception of seasonal consumption. Sustainability 14:5379. doi: 10.3390/su14095379
Rovetta, A. (2021). Reliability of Google Trends: analysis of the limits and potential of web infoveillance during COVID-19 pandemic and for future research. Front. Res. Metr. Anal. 6:670226. doi: 10.3389/frma.2021.670226
Shin, N., Kotani, A., Maruya, Y., and Gavrilyeva, T. (2022a). Can Yandex statistics and google trends be used to detect people's interests in berries in the Russian far east? Polar Sci. 33:100871. doi: 10.1016/j.polar.2022.100871
Shin, N., Kotani, A., Tei, S., and Tsutsumida, N. (2022b). Monitoring of cherry flowering phenology with Google Trends. PLoS ONE 17:e0271648. doi: 10.1371/journal.pone.0271648
Shin, N., Saitoh, T. M., Kotani, A., Miura, T., Nakagawa, H., Katsumata, C., et al. (2024). Perspective and review: how to develop our understanding of temporal changes in the relationship between people and the landscape under societal and climate change in Northeast Asia? Front. Environ. Sci. 12:1236664. doi: 10.3389/fenvs.2024.1236664
Silva, E. S., Hassani, H., Madsen, D. Ø., and Gee, L. (2019). Googling fashion: forecasting fashion consumer behaviour using Google Trends. Soc. Sci. 8:111. doi: 10.3390/socsci8040111
Stephens-Davidowitz, S., and Varian, H. (2015). A Hands-on Guide to Google Data. Available at: https://people.ischool.berkeley.edu/~hal/Papers/2015/primer.pdf (accessed September 24, 2024).
Strzelecki, A., Azevedo, A., and Albuquerque, A. (2020). Correlation between the spread of COVID-19 and the interest in personal protective measures in Poland and Portugal. Healthcare 8:203. doi: 10.3390/healthcare8030203
Takada, K. (2012). Japanese interest in “Hotaru” (Fireflies) and “Kabuto-Mushi” (Japanese Rhinoceros Beetles) corresponds with seasonality in visible abundance. Insects 3, 424–431. doi: 10.3390/insects3020424
The R Foundation (2024). The R Project for Statistical Computing. Available at: https://www.r-project.org/ (accessed September 24, 2024).
USGS (2024). USGS EROS Archive—Digital Elevation—Global 30 Arc-Second Elevation (GTOPO30). Available at: https://www.usgs.gov/centers/eros/science/usgs-eros-archive-digital-elevation-global-30-arc-second-elevation-gtopo30 (accessed September 24, 2024).
Yang, S., Santillana, M., and Kou, S. C. (2015). Accurate estimation of influenza epidemics using Google search data via ARGO. Proc. Natl. Acad. Sci. U. S. A. 112, 14473–14478. doi: 10.1073/pnas.1515373112
Keywords: autocorrelation coefficient, people's interests, phenology, tourism, 12-month seasonality
Citation: Shin N, Nakagawa H, Morimoto H and Kotani A (2024) A Google Trends analysis of people's sense of seasons in Hokkaido. Front. Sustain. Tour. 3:1446528. doi: 10.3389/frsut.2024.1446528
Received: 10 June 2024; Accepted: 18 November 2024;
Published: 17 December 2024.
Edited by:
Wei-Ching Wang, Nanfang College of Sun Yat-sen University, ChinaReviewed by:
C. K. Ceng, National Taiwan University, TaiwanCopyright © 2024 Shin, Nakagawa, Morimoto and Kotani. This is an open-access article distributed under the terms of the Creative Commons Attribution License (CC BY). The use, distribution or reproduction in other forums is permitted, provided the original author(s) and the copyright owner(s) are credited and that the original publication in this journal is cited, in accordance with accepted academic practice. No use, distribution or reproduction is permitted which does not comply with these terms.
*Correspondence: Nagai Shin, bmFnYWlzQGphbXN0ZWMuZ28uanA=
†These authors have contributed equally to this work
Disclaimer: All claims expressed in this article are solely those of the authors and do not necessarily represent those of their affiliated organizations, or those of the publisher, the editors and the reviewers. Any product that may be evaluated in this article or claim that may be made by its manufacturer is not guaranteed or endorsed by the publisher.
Research integrity at Frontiers
Learn more about the work of our research integrity team to safeguard the quality of each article we publish.