- Laboratory of Global Environmental Policy, Graduate School of Global Environmental Studies, Kyoto University, Kyoto, Japan
Introduction: In accordance with the government's pollution reduction policy, this study examines the acceptance and usage of improved fuel in a mandatory situation among citizens in Ulaanbaatar, Mongolia. The city struggles with air pollution due to high coal consumption, especially in its Ger districts.
Methods: Utilizing the Unified Theory of Acceptance and Use of Technology (UTAUT) model, the research explores the determinants influencing the behavioral intention and actual use of improved fuel. The study integrates additional factors, namely environmental impact, risk perception, and autonomous as well as controlled motivation, into the UTAUT framework. Data was collected through a cross-sectional online survey of 198 adult residents.
Results: The study identified performance expectancy, effort expectancy, and social influence as significant predictors of intention to adopt improved fuel. Performance expectancy had the strongest positive effect, while social influence negatively impacted intention, reflecting citizens' disapproval of improved fuel. Intention significantly predicted actual usage, but facilitating conditions were insignificant, likely due to the mandatory context. Autonomous motivation positively influenced intentions, highlighting the importance of understanding benefits. Controlled motivation, environmental impacts, and risks showed no significant effects.
Conclusion: The findings underscore the importance of targeted policies and public awareness in enhancing the adoption of cleaner technologies to mitigate air pollution and improve public health in the city.
1 Introduction
Despite being the most carbon-intensive fossil fuel, coal remains a crucial source of global energy production, supplying over a third of the total electricity generation. It is also a crucial energy source in the iron, steel, and cement industries (International Energy Agency, 2022). Due to its abundant availability, coal has historically played a pivotal role in global economic development. The latest data from 2022 shows that global coal prices reached a record level. Coal consumption continued to increase, amounting to 161 exajoules (EJ), the highest consumption level since 2014 (Energy Institute, 2023). China and India mainly triggered this increased demand. Global coal production reached a record high of 175 EJ in 2022, with China, India, and Indonesia being the major coal producers (Energy Institute, 2023). The share of coal consumption in various sectors differs and has changed over the past decades. While the residential sector was the top coal consumer roughly up until the end of the 90s, the sector's share in coal consumption has reached its minimum in recent years. For example, the global share of the residential sector in coal consumption in 2019 was 6.4%, compared to 19.6% in 1973 (International Energy Agency, 2021a). However, its adverse impacts on public health and the environment have become increasingly evident in recent years. In fact, coal combustion pollutants contribute to climate change, compromise air and water quality, and are linked to human morbidity and mortality (Hendryx et al., 2020).
Given the environmental and health risks associated with coal consumption, expanding access to clean fuels and technologies is crucial to mitigate household air pollution and safeguard public health. Targeted policies are crucial in low- and middle-income countries, where air pollution disproportionately affects populations. By addressing sources like fossil fuel combustion, such policies can significantly improve air quality and public health while also advancing climate change mitigation goals (WHO, 2022).
Coal is the dominant energy source in Mongolia, accounting for 60% of primary and 95% of secondary energy (Asian Development Bank Institute, 2018). Forty one percent of final coal consumption belongs to the residential sector in the country (International Energy Agency, 2021b), making it the most significant fossil fuel the households rely on for their daily activities. One of the latest policy countermeasures by the Mongolian government is Resolution No. 62, “Prohibition of the Use of Raw Coal,” enacted in 2019. This policy prohibits raw coal and mandates the adoption of improved fuel (hereafter IF), which can play a significant role in Mongolia's environmental pollution control measures. The policy significantly reduced PM2.5 and PM10 fine particles in air pollution, approaching the standard level specified by the Mongolian Agency for Standard and Metrology (Air Quality General Technical Requirements MNS4585:2016), which was previously three times higher. There was minimal change in CO pollution parameters; however, SO2 levels increased from 1.2 to 2.5 times the standard level (Mongolian Department against Air and Environmental Pollution, 2022).
Despite the potential benefits of new technologies, such as the introduction of IF, they can prompt diverse public responses, owing to the profound influence of energy within economic, political, and social frameworks, coupled with the extensive ramifications of energy-related decisions on ecological balance and public health. Understanding these responses and their underlying determinants is paramount, given their potential to shape the acceptance and utilization of novel technologies (Boudet, 2019). Scholars have voiced concerns regarding the fragmented nature of research findings in this domain, attributing this to the diverse theoretical underpinnings employed by researchers investigating perceptions of citizens and reactions to new energy technologies (Frankowski and Herrero, 2021; Boudet, 2019). This fragmentation impedes the development of a cohesive theoretical framework, as most studies tend to be descriptive rather than theoretically grounded (Frankowski and Herrero, 2021; Kang et al., 2022). Therefore, this research aims to explore Mongolian citizens' acceptance of the IF policy. To the author's knowledge, no previous literature has investigated this policy's acceptance levels and determinants as a direct government intervention aimed at citizens and individuals. Studying the acceptance of improved fuel after more than 5 years of its mandate is crucial for several reasons. First, mandates do not ensure genuine acceptance. Understanding ongoing compliance and satisfaction helps assess if the policy benefits are sustained over time. Second, negative perceptions, such as concerns over quality or health impacts, highlight areas for improvement. This study's insights from this study can guide enhancements to improve public satisfaction and compliance. Finally, lessons from this study can inform future policies and help shape more effective strategies for pollution reduction initiatives.
In order to analyze acceptance, this research will use the Unified Theory of Acceptance and Use of Technology (UTAUT). The key benefit of the UTAUT model is that it is already highly integrative, synthesizing eight disciplines and concepts, and quantitative studies have confirmed its predictive or explanatory power. Integrative theory can minimize bias, best fit a research topic or question, and tighten concepts through critical thinking (Sovacool and Hess, 2017). Other relevant theories lack such comprehensiveness. For example, the Technology Acceptance Model focuses primarily on perceived usefulness and ease of use but does not incorporate social or facilitating factors (Marikyan and Papagiannidis, 2023). Similarly, the Diffusion of Innovation theory emphasizes the characteristics of innovation but lacks a strong focus on individual behavioral intention (Dwivedi et al., 2019). Also, the Theory of Planned Behavior incorporates social norms and perceived behavioral control. However, it does not account for the role of performance or effort expectancy, which are key drivers in technology acceptance (Venkatesh et al., 2003).
Therefore, this research objectives (1) assess the level of public acceptance of the IF policy (Resolution No. 62) among Mongolian citizens, (2) identify the determinants influencing the acceptance of the IF policy in Mongolia, using the extended UTAUT framework, and (3) contribute to the development of a theoretical framework for understanding public responses to energy policy interventions in low- and middle-income countries. The research questions include: (1) What is the current level of public acceptance of the IF policy among Mongolian citizens? (2) How do the key constructs of the UTAUT model (performance expectancy, effort expectancy, social influence, and facilitating conditions) and the newly added variables influence Mongolian citizens' acceptance of the IF policy? (3) How can findings on public acceptance and determinants of IF adoption inform the design and implementation of future energy policies in Mongolia and similar contexts?
This research contributes to technology acceptance literature in three key ways. First, it extends the UTAUT model by incorporating additional variables such as environmental impacts, risk perception, and motivation (autonomous and controlled), tailored to a mandatory policy context. Second, the findings challenge traditional assumptions within the UTAUT, such as the positive influence of social influence on behavioral intention, by revealing its negative impact in this specific setting. This highlights the need to consider cultural and situational factors in technology adoption frameworks. Finally, the study provides empirical evidence from a developing country context, offering new insights into how socio-economic and policy-driven factors shape public acceptance.
Section 2 discusses the theoretical framework and hypotheses development, providing an overview of the UTAUT model and its extensions for this study. Section 3 outlines the methodology, including the survey design, data collection, and analytical techniques used. Section 4 presents the results, focusing on the key findings from the structural equation modeling analysis. Section 5 provides a discussion of the findings in light of existing literature, emphasizing theoretical and practical implications. Finally, Section 6 concludes the study by summarizing the key insights, highlighting limitations, and suggesting directions for future research.
2 The UTAUT model and research hypotheses
Venkatesh et al. (2003) reviewed and compared eight major technology acceptance models to create a unified perspective on user acceptance of technology. Through a thorough analysis of these models, they developed the UTAUT. This new model was designed to address the limitations of its predecessors and demonstrated superior empirical performance. The original UTAUT model distills the core factors in predicting behavioral intentions as the main factor determining technology use. These factors include performance expectancy, effort expectancy, social influence, and facilitating conditions.
Performance expectancy, a core construct in the UTAUT model, refers to the extent to which an individual believes using a system will help them achieve desired outcomes (Venkatesh et al., 2003). Studies on electric vehicle adoption (e.g., Abbasi et al., 2021; Jain et al., 2022) or renewable energy adoption (e.g., Ahn et al., 2016; Kaur et al., 2024) have shown that perceived performance benefits, such as fuel efficiency and environmental friendliness, significantly impact consumer adoption intentions. In the context of IF policy, this translates to the perceived benefits individuals associate with adopting IF, such as improved health, reduced emissions, or disease prevention. Based on this background, the current study hypothesizes that:
H1: Higher performance expectancy will have a positive effect on behavioral intention to accept IF.
Venkatesh et al. (2003) hypothesize that the effect of performance expectancy on intention will be moderated by gender and age to make the effect stronger for younger males. Thus, this study also assumes that:
H1a: The influence of performance expectancy on intention will be moderated by age and gender, being stronger for younger men.
Effort expectancy refers to the system's ease of use (Venkatesh et al., 2003). The significant effect of effort expectancy on intentions has been supported in previous sustainability studies, such as the study of Pak et al. (2023) on demand-responsive transportation and Kaur et al. (2024) on solar water heater adoption. In the case of IF, effort expectancy could relate to the perceived convenience of accessing and using IF and the ease of integrating IF into existing fuel infrastructure. Against this background, the current study assumes that:
H2: Greater effort expectancy will have a positive effect on behavioral intention to accept IF.
The influence of effort expectancy is moderated by gender, age, and experience so that the effect will be stronger for younger females and at early stages of experience (Venkatesh et al., 2003). The study thus assumes that:
H2a: This influence will be moderated by gender, age, and experience, being stronger for younger women at their earlier stages of experience.
Social norms, opinions of peers, and endorsements from influential figures can sway individual attitudes and behaviors (Venkatesh et al., 2003). Social influence has been consistently a significant predictor of technology adoption intentions (e.g., Pak et al., 2023; Kaur et al., 2024; Dwivedi et al., 2022; Liu et al., 2023). For IF adoption, social influence could manifest as community support for environmentally friendly fuel options or endorsements from trusted individuals. Thus, it is hypothesized that:
H3: Favorable social influence will have a positive effect on behavioral intention to accept IF.
The effect of social influence on intention is moderated by all four variables, such that it will be stronger for elder women in a mandatory setting and at early stages of experience (Venkatesh et al., 2003). Thus:
H3a: This effect will be moderated by gender, age, and experience, being stronger for older women with experience in using IF.
Facilitating conditions refer to the degree to which an individual believes that there are supporting infrastructures to use the system (Venkatesh et al., 2003). This can include access to information, training, technical infrastructure, or organizational support. Studies have shown that strong facilitating conditions can overcome adoption barriers and accelerate the diffusion of new technologies (e.g., Jain et al., 2022; Abbasi et al., 2021). Thus:
H4: Good facilitating conditions will have a positive effect on actual usage to accept IF.
Facilitating conditions are more impactful for older, experienced users who rely on established routines (Venkatesh et al., 2003). Therefore:
H4a: This effect will be moderated by age and experience, being stronger for older users with more experience.
Despite the vast applicability of the UTAUT model in different contexts, the low external validity of the model has motivated researchers to further extend it by including additional determinants of behavior (Marikyan and Papagiannidis, 2023). The present study adapts the UTAUT model based on its objectives. As mentioned in the previous sections, IF was introduced in response to the heightened concerns about the environmental risks attributed to coal consumption. It is expected that IF would result in environmental and health benefits. Therefore, the present study assumes that expected environmental benefits will affect the IF adoption intention and adds the new variable “environmental impact” to the original UTAUT model:
H5: Lower negative environmental impacts associated with IF will positively affect the adoption intention for IF.
Additionally, several previous studies point out the importance of risk and safety perception in deterring the adoption of new technologies (e.g., Widyanti et al., 2024; Jain et al., 2022; Abbasi et al., 2021). Considering the significant increase of SO2 emissions in IF (Mongolian Department against Air and Environmental Pollution, 2022), it is assumed that such health risks might negatively affect the adoption intention of IF:
H6: In the adoption of IF, higher risk will negatively influence acceptance
According to the UTAUT model, behavioral intention is a directly predicts of actual usage (Venkatesh et al., 2003). Thus:
H7: Behavioral intention to use IF significantly and positively will affect the actual use of IF.
Finally, based on research conducted by Guo (2022) in a similar mandatory context, this study adds autonomous and controlled motivation variables to understand how motivations, either controlled reasons or self-governed reasons, affect the intention. Autonomous motivation, a central concept in Self-Determination Theory (SDT; Deci and Ryan, 2000), refers to engaging in a behavior because it aligns with one's values, interests, or sense of self. This contrasts with controlled motivation, where behaviors are driven by external pressures or rewards. Autonomous motivation, aligned with self-determination theory, has been linked to higher acceptance of mandatory policies when individuals understand and internalize the benefits (Guo, 2022; Deci and Ryan, 2000). Controlled motivation, while less impactful than autonomous motivation, can influence intentions in settings with external contingencies like fines or rewards (Hagger et al., 2014). Thus, the study hypothesizes that:
H8: Autonomous motivation will have a significant positive effect on intention.
H9: Controlled motivation will have a significant positive effect on intention.
Figure 1 depicts the study's extended UTAUT model and hypotheses.
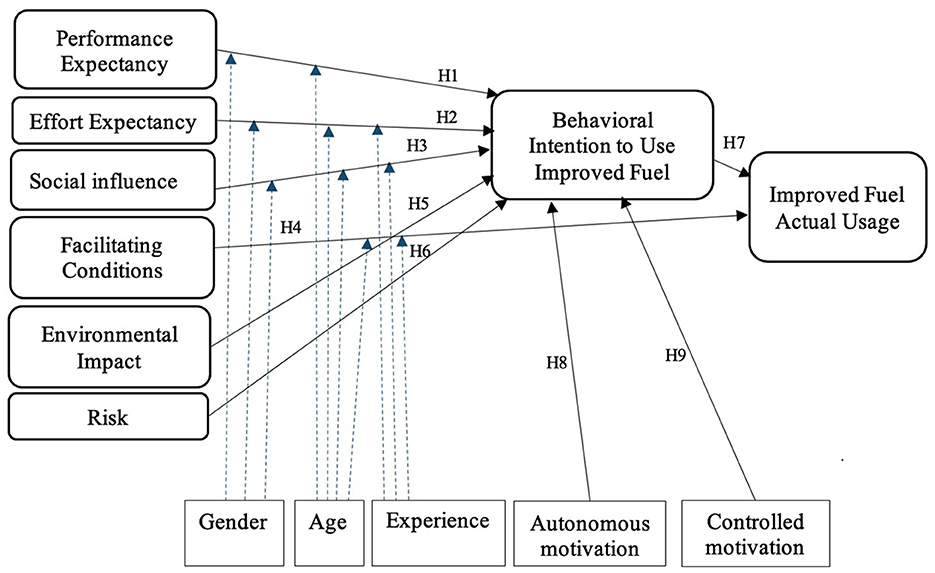
Figure 1. Adapted UTAUT model. The solid lines represent direct hypothesized relationships (e.g., performance expectancy → behavioral intention, H1). The dashed lines represent moderation effects (e.g., gender moderating performance expectancy → behavioral intention, H1a). Labels of moderating effects are omitted from the figure to maintain a clean and uncluttered look for the figure.
3 Methods and materials
3.1 Case study: Ulaanbaatar city
The capital city of Ulaanbaatar contains more than 40% of the country's total population. The city consists of a dense downtown core area and a ring of quasi-informal dwellings called Ger (around 60% of the city's population; Carlisle and Pevzner, 2019). The city is renowned as the world's coldest capital, positioned in a high-latitude basin at an altitude of 1,320 meters. It experiences extreme seasonal temperature variations, with winters averaging −25°C and summers reaching 30°C. The city grapples with prolonged, cold, and dry winters, contrasted by brief cool summers, with an average annual rainfall of 242.7 mm and 69% humidity. The geographical and climatic factors significantly impact air quality, especially in winter, due to the influence of the Siberian High on air circulation, resulting in stagnant and polluted air in the urban basin areas [National Statistics Office of Mongolia (NSO), 2024]. Over the years, coal has been the city's predominant source of energy provision, being burned at three levels: at centralized power plants, smaller heat plants and heat boilers, and hundreds of thousands of individual dwellings (Carlisle and Pevzner, 2019). Consequently, the air pollution problem due to the use of coal is severe in Ulaanbaatar. In fact, in 2015 and 2016, coal-dependent cities like Ulaanbaatar ranked as the most polluted city globally, surpassing industrialized metropolises like Beijing and New Delhi (Battulga and Dhakal, 2024). The primary source of winter air pollution is categorized into four main contributors. Approximately 80% of the air pollution stems from the annual consumption of 1 million tons of coal by stoves in the Ger districts and 3,000 heating stoves owned by enterprises. Another 10% comes from 238,000 vehicles using fuel for over a decade, 6% from coal in thermal power plants, and 4% from other sources [Unified Legal Information System (Mongolia), 2024]. The current toll of air pollution-related illnesses in Mongolia is dire, with 300 deaths annually, including 240 children under 5 years old. This stark statistic underscores the disproportionate impact of air pollution on children, highlighting the urgent need for action to protect their health (UNICEF Mongolia, 2023). Given the challenges faced, addressing Ulaanbaatar's heat supply and energy concerns is imperative to mitigate the impacts of severe winter conditions (National Statistics Office of Mongolia, 2024).
3.2 Survey design and data collection
This research utilizes an observational, cross-sectional survey method to assess the behavioral intention and actual usage of IF among Ulaanbaatar residents who have experience using this fuel type and reside in the Ger districts. The survey was designed using Google Forms. Online surveys offer time efficiency, enabling faster data collection and feasibility in situations with limited budgets. Mongolia has a relatively high internet penetration rate among urban populations, with the most significant coal consumption and pollution levels. Therefore, conducting an online survey aligns well with the study's aim to capture views primarily from citizens most impacted by the IF policy, particularly those residing in urban areas like Ulaanbaatar, where the impact on air quality is most apparent. Questionnaires were sent out through Facebook social media networks to recruit respondents. The target participants were Mongolian citizens over 18 years old who reside in the Ger district and have had experience with IF. One hundred and ninety-eight responses were collected from March 7th to April 6th, 2023. There are various opinions about the proper sample size. Some authors suggest a minimum of 100 (Boomsma, 1985 in Gana and Broc, 2018) to 200 (Kline, 2016 in Gana and Broc, 2018) participants for a non-complex model. Therefore, the study meets the minimum sample size based on these suggestions and some of the previous studies (e.g., Guo, 2022; Kang et al., 2022).
There were 2.5 million Mongolian Facebook users at the beginning of 2022, accounting for 85% of the total population. According to the 2020 population and housing census, 91.2% of people aged six and older in Ulaanbaatar use mobile phones, 76.0% use the internet, and the literacy rate for citizens over the age of 15 is 99.5%. The questionnaire included 13 demographic questions and 33 theoretical questions covering dependent, independent, and mediator variables. These questions were formulated based on previous research (e.g., Venkatesh et al., 2003; Madigan et al., 2017; Guo, 2022) and specifically adapted for the case of IF. The validity and reliability of the questionnaire were both above 0.70. The questions were developed based on theoretical concepts and an earlier UTAUT-based survey. Each item was rated on a five-point Likert scale, where respondents expressed their level of agreement with the statements from strongly agree (5) to strongly disagree (1). Two independent translators did the first translation from the source language into Mongolian. Bilingual translators translated the questionnaire into their native language to accurately capture the complexity of the target language (Guillemin et al., 1993). Next, a third neutral bilingual translator who was not involved in the previous translation discussed and resolved disagreements between the two translators.
An expert carefully assessed each version of the translation to ensure consistency in meaning, expression, and concepts compared to the original text. Disagreements were resolved collaboratively, resulting in the final translated questionnaire. Five individuals tested this final version, also used to create a new questionnaire. Interviewers verbally prompted respondents to share their thoughts on each item, ensuring clarity and fidelity to the original meaning in the translated questionnaire.
3.3 Statistical analyses
Structural Equation Modeling (SEM) analysis was conducted to examine the factors affecting the acceptance of IF. First, following the suggestions of Anderson and Gerbing (1988), Confirmatory Factor Analysis (CFA) was used to ensure the validity of measures before testing the whole model. Additionally, in order to further evaluate the reliability of the scales and construct validity, several quantitative indicators including Cronbach's alpha, composite reliability (Hair et al., 2017), rho_a (Dijkstra and Henseler, 2015), Average Extracted Variance (AVE) and the square root of AVE (Fornell and Larcker, 1981), and Heterotrait-Monotrait ratio (HTMT) of correlations (Henseler et al., 2015) for assessing convergent validity and discriminant were calculated. HTMT is a measure used to assess discriminant validity in structural equation modeling (SEM). It compares the correlations between constructs (heterotrait correlations) to correlations within constructs (monotrait correlations; Henseler et al., 2015).
“Convergent validity is the degree to which multiple attempts to measure the same concept agree. The idea is that two or more measures of the same thing should covary highly if they are valid measures of the concept” (Bagozzi et al., 1991, p. 425). When the AVE value is greater than or equal to the recommended value of 0.50, items converge to measure the underlying construct, and hence convergent validity is established (Fornell and Larcker, 1981). “Discriminant validity is the degree to which measures of different concepts are distinct. If two or more concepts are unique, then valid measures of each should not correlate too highly” (Bagozzi et al., 1991, p. 425). According to Fornell and Larcker's (1981) criterion, discriminant validity is established when the square root of AVE for a construct is greater than its correlation with all other constructs.
After evaluating the measurement models' quality and validity, the whole model's predictive validity was assessed in the SEM stage of the process. Statistical analyses were conducted in SmartPLS version 4 (Ringle et al., 2021) and IBM SPSS Statistics version 28.0 (IBM Corp, 2021), utilizing Cronbach's Alpha (≥0.70), Composite Reliability (≥0.70), Average Variance Extracted (≥0.50), and Heterotrait-Monotrait Ratio (<0.85) to confirm the model's goodness of fit.
4 Results
4.1 Descriptive statistics
Figures 2A–D displays some main sample characteristics. Around 80% of respondents were female. Fifty four percent of respondents are between 30 and 40 years old, 20% are in their 40s, 14% are in their 50s, 8% are in their 20s, and 4% are over 60. The sample is rather educated, with 50% of the respondents having a high school education and around 40% having an undergraduate or graduate level of education. In addition, a significant % of respondents, 96%, have experience using IF as their primary energy source.
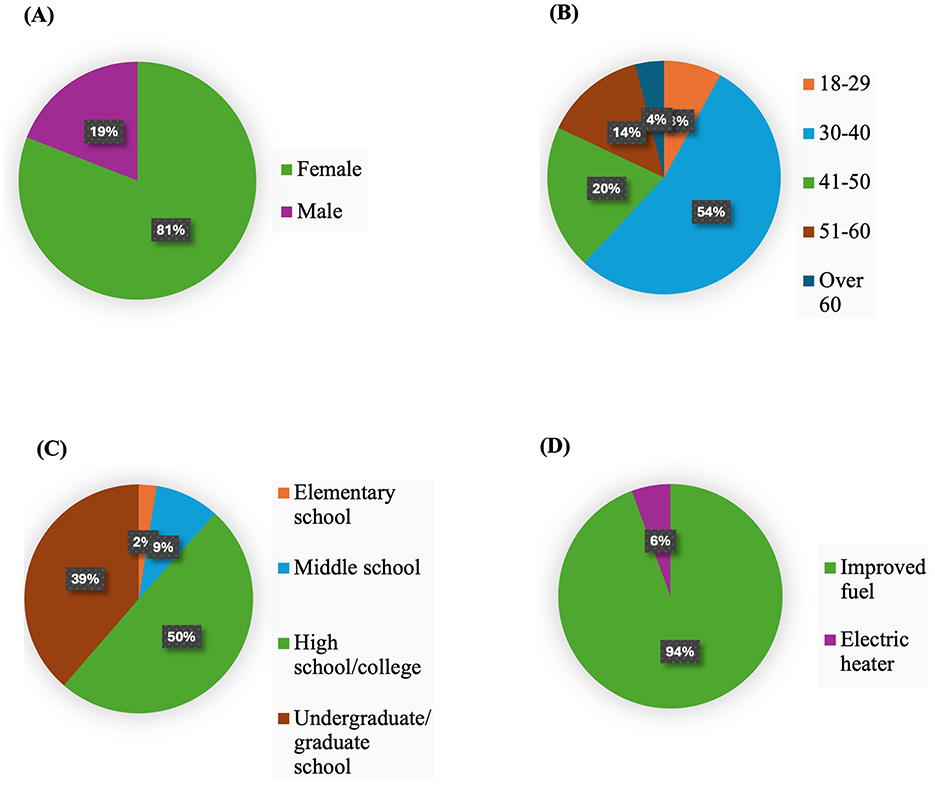
Figure 2. Demographic characteristics of the sample participants (%). (A) Gender. (B) Age. (C) Education level. (D) Household energy source.
4.2 Measurement models
The measurement models were assessed to establish the reliability and validity of the constructs (Table 1). First, the factor loadings of all the items in the model have a value greater than the minimum acceptable value of 0.50 mentioned in the previous research (e.g., Hair et al., 2017). Although a factor loading over 0.7 is desirable (Esposito Vinzi et al., 2010), researchers frequently obtain weaker outer loadings in social science studies. Rather than automatically eliminating indicators, the effects of removing the item on composite reliability, content, and convergent validity should be examined. In the current study, items Social Influence 4 (SI4) and Controlled Motivation 1 (CM1) were eliminated due to factor loadings lower than the acceptable amount of 0.5. In addition, items Controlled Motivation 3 (CM3), Facilitating Conditions 3 (FC3), and Performance Expectancy 3 (PE3) were removed because their HTMT was higher than the suggested ratio, implying that discriminant validity was not confirmed. Reliability was assessed using Cronbach's alpha, composite reliability, and rho_a. Statistics for the first two tests exceeded the recommended value of 0.700 (Wasko and Faraj, 2005). The rho_a value was between Cronbach's alpha and composite reliability (Sarstedt et al., 2022) and over 0.70, indicating good reliability (Henseler et al., 2016). Convergent validity was confirmed since the AVE for all constructs was higher than 0.500. In addition, the square root of AVE was higher than the correlations among the latent variables, and the heterotrait-monotrait ratio (HTMT) of correlations was below the (conservative) threshold of 0.85. Hence, discriminant validity was also confirmed (see Tables 1, 2).
4.3 Structural model assessment
Table 3 shows the results of the SEM analysis. The results reveal that performance expectancy (B = 0.431, t = 2.209, p < 0.05), effort expectancy (B = 0.259, t = 1.738, p < 0.05), and autonomous motivation (B = 0.464, t = 4.273, p < 0.05) have significant and positive effects on behavioral intentions to accept the IF among respondents. Therefore, hypotheses H1, H2, and H9 are supported. While favorable social influence (B = −0.337, t = 1.715, p < 0.05) was statistically significant, unlike hypothesized, its effect on the intention was negative. Thus, H3 is not confirmed regarding the direction of its effect. The effect of good facilitating conditions (B = 0.115, t = 1.546, p > 0.05) is insignificant on actual usage, and H4 is not confirmed.
The current study also added two factors, risk (B = 0.079, t = 1.039, p > 0.05) and environmental impacts (B = 0.012, t = 0.174, p > 0.05), to investigate whether they can affect the intentions of the respondents to accept the IF. The findings show that neither of these two factors is significantly connected with intentions. In other words, higher risks and environmental impacts do not affect acceptance. Thus, H5 and H6 are not confirmed. Expectedly, behavioral intention is a strong and significant predictor of actual usage (B = 0.676, t = 9.866, p < 0.05) and H7 is supported. Experience (B = 0.094, t = 1.197, p > 0.05) and controlled motivation (B = 0.059, t = 0.840, p > 0.05) are insignificant predictors of intentions and H8 and H10 are not supported.
R2 represents the variance explained in each endogenous construct and measures the model's explanatory power (Shmueli and Koppius, 2011). The R2 ranges from 0 to 1, with higher values indicating a greater explanatory power. As a general guideline, Cohen (1988) suggested that R2 values for endogenous latent variables are assessed as follows: 0.26 (substantial), 0.13 (moderate), 0.02 (weak). The results (Table 4) show that the R2 for all the endogenous constructs is over 0.26, indicating a substantial explanatory power. To better estimate the explanatory value of each exogenous variable in the model, the effect size (f2) can be tested. The effect size measures the influence of each independent variable on the dependent variable. The impact of the predictor variable is high at the structural level if f2 is 0.35, medium if f2 is 0.15, and minor if f2 is 0.02 (Cohen, 1988). The results (Table 4) reveal that f2 effect size ranges from 0.000 (negligible) for EI on BI to 1.019 (high) for BI on AU. Finally, Q2 values for the endogenous constructs were over 0; hence, predictive relevance was established (Hair et al., 2017).
To assess a model's predictive power, the root-mean-square error (RMSE) values were calculated, and the LM benchmark values were obtained by running a linear regression of each of the dependent construct's indicators on the indicators of the exogenous constructs in the PLS path model (Danks and Ray, 2018). The comparison between the RMSE and the LM values (Table 5) shows that all indicators in the PLS-SEM analysis have lower RMSE values than the naïve LM benchmark. Hence, the model has high predictive power.
4.4 Moderation analysis
Table 6 shows the results of the moderation analysis. Based on this analysis, only two significant moderating effects were identified. First, results concerning the role of gender on the relationship between SI and BI (p-value = 0.024) reveal that without gender being included, 47.9% of the variance in BI is explained by SI. After including gender in the analysis, the explained variance increases to 65.7%. This analysis demonstrates that social influence is a significant predictor of intentions, and this effect is significantly stronger among female participants. Second, age negatively and significantly moderates the relationship between FC and AU (p-value = 0.034). In other words, the effect of FC on AU is stronger among younger participants. According to Cohen's (1988) rule of effect size (f2), results reveal that moderating effects do not contribute significantly to explaining the endogenous construct (Table 6).
4.5 Respondents' opinions on IF
Sixty-seven individuals left a comment on how they think about IF. Fifty-three respondents believed that burning produces excessive soot and ash, which affects the air quality and causes inconvenience and health concerns. Twenty people raised the issue of poor combustion, noting that the fuel neither ignites well nor burns completely, causing excessive smoke. Among these groups, some even mentioned that fuel quality has deteriorated. Twenty-five respondents reported headaches and respiratory issues due to the unpleasant fuel odor and expressed concerns about persistent colds and irritation of the lungs. Eleven people expressed concern for carbon monoxide poisoning or other dangerous effects from the fuel, mentioning symptoms like dizziness and nausea. There was significant concern that the fuel might be a silent danger in their homes. Six users suggested that traditional coal, despite its environmental drawbacks, was more efficient and less problematic for health and daily use. Finally, 18 users suggested that the IF needs better quality control and further research. They believed that the fuel composition could be adjusted to address the issues of soot, odor, and combustion efficiency. These opinions reflect a significant dissatisfaction with the IF among the participants.
5 Discussion
This study analyzed the factors influencing Ulaanbaatar citizens' acceptance of the IF using the UTAUT model. The findings indicated that the model could explain around 68% of the variance in behavioral intentions and 66% in actual usage in a mandatory situation. Performance expectancy, effort expectancy, and social influence were all significant predictors of adoption intention. While the former two factors were positively connected to intentions, the latter negatively affected the intentions. The study also confirmed that intention is the significant and direct predictor of actual usage; however, facilitating conditions were insignificant. These factors have been commonly, but not consistently, mentioned as significant predictors of intentions in other sustainability research areas (e.g., Dwivedi et al., 2022; Pak et al., 2023; Liu et al., 2023; Kaur et al., 2024).
5.1 Theoretical implications
This study advances the UTAUT model by incorporating variables specific to sustainability and policy-driven contexts, such as environmental impacts, risk perception, and motivation (autonomous and controlled). These additions address gaps in the literature by refining the model's applicability to mandatory settings and developing countries. The negative impact of social influence on behavioral intention provides a novel insight, challenging the UTAUT's assumption that social pressures always positively influence adoption. This finding underscores the need to consider cultural and contextual factors in technology acceptance models. Furthermore, the strong role of autonomous motivation in a mandatory policy scenario highlights the importance of intrinsic factors, expanding the theoretical basis for understanding behavioral responses in sustainability research.
Koh et al. (2010) argue that in mandatory settings, people will pay more attention to the usefulness of the new system. Therefore, performance expectancy has a greater effect on intentions than effort expectancy. Similarly, in the current study's mandatory context, the effect of performance expectancy was more significant than that of effort expectancy. Social influence had a negative effect on intentions to adopt IF. This finding is intriguing as it contrasts with most previous studies and the assumption of the UTAUT model. Sledgianowski and Kulviwat (2009) find a negative relationship between normative pressure and the intent to use. They discuss that normative pressure might only be influential in the initial stages, or sometimes, pressure from significant others can result in a reverse effect. Opinions expressed by over one-third of the respondents in the present study show that many people are concerned about the impacts of the IF and are not content with the current quality of the fuel. This might imply that citizens generally disapprove of the IF and its adoption. Consequently, people's significant others' disapproval might decrease an individual's motivation and intention to engage in the behavior and lead to the negative effect of social influence on the adoption intention of the IF.
In this study's mandatory situation, autonomous motivation significantly and positively influenced behavioral intentions to use the IF. This result shows that respondents with autonomous motivation recognize the benefits of using IF better, even as a mandatory behavior, and respond more positively to this mandatory policy. A similar result regarding the role of autonomous motivation was found in Guo (2022) in a study about factors influencing students' acceptance of sports apps in a mandatory situation. Similar to some previous studies (e.g., Hagger et al., 2014; Leo et al., 2022), controlled motivation had no effect on intentions to use IF. According to the self-determination theory (Deci and Ryan, 2000), individuals who engage in certain behaviors for controlled reasons feel pressure and obligation and are likely to persist with the behavior as long as the external contingency, such as a fine or punishment, exists. Thus, the effect of controlled motivation on intentions is smaller than that of autonomous motivation. The results of this study also align with the premises of self-determination theory.
Facilitating conditions had no significant effect on the actual use. This might be due to the fact that the situation is mandatory, and whether there are facilitating conditions or not, people are left with no other choice. In addition, households are well-equipped and familiarized with how to use the IF. Moreover, Venkatesh et al. (2003) state that facilitating conditions are only significant when the moderating effects of experience and age are also analyzed. This study assessed the moderating effect of experience on the relationship between facilitating conditions and actual use. Still, the moderating effect was insignificant. Finally, this study did not confirm any significant effects of the two newly added variables, i.e., environmental impacts and risks, on the intentions.
The study found that moderating effects, such as gender, age, and experience, on the relationships between key predictors and behavioral intention or actual usage were statistically insignificant. This finding contrasts with previous research using the UTAUT model (Venkatesh et al., 2003), highlighting these factors' moderating influence. Several contextual and methodological reasons may account for these results. First, the mandatory nature of the IF policy likely minimized the impact of individual differences. In such contexts, citizens may have limited autonomy in decision-making, reducing the variability in responses based on gender, age, or experience. This aligns with findings from Koh et al. (2010), which suggest that mandatory adoption diminishes the influence of personal and demographic moderators. Second, the sample's demographic characteristics may have contributed to the lack of significant moderation effects. The participants' education and experience with IF were relatively homogeneous, which could explain why these moderators did not show significant variance. For example, nearly all respondents had prior experience using IF, limiting the potential impact of “experience” as a moderator. Third, the theoretical framework of the UTAUT assumes that moderating effects become more pronounced in voluntary contexts where individual preferences play a larger role. In this study, the mandatory adoption setting likely reduced the salience of these moderators, as citizens were compelled to adopt IF regardless of their demographic characteristics or prior experience.
5.2 Practical implications
The findings provide actionable insights for policymakers aiming to increase citizen acceptance of mandatory environmental technologies like IF. First, addressing citizens' dissatisfaction with the quality of IF is essential. Policymakers should implement stricter quality control measures and provide transparent updates on product improvements. Second, public awareness campaigns focusing on IF's environmental and health benefits can enhance intrinsic motivation and reduce resistance. Additionally, tailored interventions, such as targeted messaging for older citizens accustomed to traditional fuels, can address demographic-specific concerns. Finally, fostering autonomous motivation through educational programs can align citizens' values with policy goals, ensuring greater acceptance and long-term compliance.
6 Conclusion
Coal is a major source of power production and fuels energy-intensive industries globally despite its proven negative impacts on climate change, air pollution, and subsequent health issues. Mongolia has a long history of coal reliance, with the residential sector being the top coal consumer. This dependence on coal has led to severe environmental and health problems in Mongolia. In the capital city of Ulaanbaatar, Ger dwellings burn over a million tons of raw coal annually, the main contributor to severe air pollution during the winter months.
To combat the issues arising from coal combustion, the Mongolian government banned the use of raw coal in 2019 and introduced IF. As energy policies are intertwined with many other socio-economic variables, introducing IF can trigger various public responses. Understanding the complexities of public response and acceptance of new energy technologies is crucial for achieving desirable policy outcomes. Thus, while such policies are significant steps toward environmental protection and climate mitigation, it is also essential to investigate the social aspects of new energy policies.
The current study aims to understand citizens' acceptance of IF, which was not thoroughly investigated in the years following the policy's introduction. To achieve this objective, the research adopts the UTAUT model. It extends it by incorporating environmental impacts, risks, and autonomous and controlled motivations based on the IF case in the Mongolian context. The results show that performance expectancy, effort expectancy, social influence, and autonomous motivation are significant predictors of the intention to adopt IF.
Policymakers should ensure that the high standards of IF, or any other energy-related technologies, are maintained to keep citizens satisfied with new technologies' performance and ease of use. Additionally, fostering positive attitudes among citizens regarding the benefits of IF can lead to better satisfaction and acceptance than punitive measures, which might provoke resistance.
6.1 Limitations and future directions
This study provides valuable insights into the factors influencing the acceptance and use of IF in Ulaanbaatar. However, several limitations should be noted. First, the data were collected using a cross-sectional survey, simultaneously capturing responses. As a result, the study cannot assess how perceptions and behavioral intentions may change over time, particularly as citizens become more accustomed to the mandatory use of IF. Future research could employ a longitudinal design to examine these dynamics. Second, while social media was a practical tool for survey distribution in this study, it presents potential biases, including self-selection and limited access to specific demographics. The high penetration rate of Facebook in Mongolia mitigates some of these biases, as most urban residents actively use the platform. However, future studies could address this limitation by combining social media surveys with alternative methods, such as in-person or random sampling, to reach underrepresented groups and ensure greater generalizability of the findings. Finally, the study did not include other potentially relevant factors, such as trust in government policies or perceptions of economic incentives. Future research could incorporate these variables to provide a more comprehensive understanding of citizens' acceptance of IF and other sustainable technologies.
Data availability statement
The original contributions presented in the study are included in the article/Supplementary material, further inquiries can be directed to the corresponding author/s.
Ethics statement
The studies involving humans were approved by Global Environmental Policy Research Ethics Committee. The studies were conducted in accordance with the local legislation and institutional requirements. The participants provided their written informed consent to participate in this study.
Author contributions
UB: Conceptualization, Data curation, Formal analysis, Investigation, Methodology, Project administration, Resources, Software, Supervision, Validation, Visualization, Writing – original draft, Writing – review & editing.
Funding
The author(s) declare that no financial support was received for the research, authorship, and/or publication of this article.
Acknowledgments
The authors would like to express her deep gratitude to Professor Makoto Usami of the Graduate School of Global Environmental Studies at Kyoto University for his invaluable guidance throughout the writing of this research. The author is also sincerely grateful to Dr. Bahareh Ghafouri for her insightful comments on the manuscript. Furthermore, the author would like to extend her heartfelt thanks to the Ministry of Education, Culture, Sports, Science, and Technology of Japan (MEXT) for their support.
Conflict of interest
The author declares that the research was conducted in the absence of any commercial or financial relationships that could be construed as a potential conflict of interest.
Generative AI statement
The author(s) declare that no Gen AI was used in the creation of this manuscript.
Publisher's note
All claims expressed in this article are solely those of the authors and do not necessarily represent those of their affiliated organizations, or those of the publisher, the editors and the reviewers. Any product that may be evaluated in this article, or claim that may be made by its manufacturer, is not guaranteed or endorsed by the publisher.
Supplementary material
The Supplementary Material for this article can be found online at: https://www.frontiersin.org/articles/10.3389/fsuep.2024.1508302/full#supplementary-material
References
Abbasi, H., Johl, S., Shaari, Z., Moughal, W., Mazhar, M., Musarat, M., et al. (2021). Consumer motivation by using Unified Theory of Acceptance and Use of Technology towards electric vehicles. Sustainability 13:12177. doi: 10.3390/su132112177
Ahn, M., Kang, J., and Hustvedt, G. (2016). A model of sustainable household technology acceptance. Int. J. Consumer Stud. 40, 83–91. doi: 10.1111/ijcs.12217
Anderson, J. C., and Gerbing, D. W. (1988). Structural equation modeling in practice: a review and recommended two-step approach. Psychol. Bull. 103, 411–423.
Asian Development Bank Institute (2018). Ulaanbaatar Air Quality Improvement Program: Report and Recommendation of the President, Sector Assessment: Energy. Available at: https://www.adb.org/projects/documents/mon-51199-001-rrp (accessed March 15, 2024).
Bagozzi, R. P., Yi, Y., and Phillips, L. W. (1991). Assessing construct validity in organizational research. Administr. Sci. Q. 36:421. doi: 10.2307/2393203
Battulga, S., and Dhakal, S. (2024). Stakeholders' perceptions of sustainable energy transition of Ulaanbaatar city, Mongolia. Renew. Sustain. Energy Rev. 189:114020. doi: 10.1016/j.rser.2023.114020
Boomsma, A. (1985). Non-convergence, improper solutions, and starting values in LISREL maximum likelihood estimation. Psychometrika 50, 229–242.
Boudet, H. S. (2019). Public perceptions of and responses to new energy technologies. Nat. Energy 4, 446–455. doi: 10.1038/s41560-019-0399-x
Carlisle, S., and Pevzner, N. (2019). Challenges of Radical Energy Sector Decarbonization. Philadelphia, PA: Kleinman Center for Energy Policy.
Cohen, J. (1988). Statistical Power Analysis for the Behavioral Sciences, 2nd Edn. New York, NY: Routledge.
Danks, N. P., and Ray, S. (2018). “Predictions from partial least squares models,” in Application of Partial Least Squares – Structural Equation Modeling (PLS-SEM) in Tourism and Hospitality Research, eds. F. Ali, S. M. Rasoolimanesh, and C. Cobanoglu (Leeds: Emerald Publishing Group), 35–52.
Deci, E. L., and Ryan, R. M. (2000). The “What” and “Why” of goal pursuits: human needs and the self-determination of behavior. Psychol. Inquiry 11, 227–268. doi: 10.1207/S15327965PLI1104_01
Dijkstra, T. K., and Henseler, J. (2015). Consistent and asymptotically normal PLS estimators for linear structural equations. Comput. Stat. Data Anal. 81, 10–23. doi: 10.1016/j.csda.2014.07.008
Dwivedi, A., Dwivedi, P., Joshi, K., Sharma, V., Sengar, A., Agrawal, R., et al. (2022). Local leader's impact on adoption of renewable energy generation technology by rural communities in the Himalayan region. J. Clean. Prod. 352:131479.
Dwivedi, Y. K., Rana, N. P., Jeyaraj, A., Clement, M., and Williams, M. D. (2019). Re-examining the Unified Theory of Acceptance and Use of Technology (UTAUT): towards a revised theoretical model. Inf. Syst. Front. 21, 719–734. doi: 10.1007/s10796-017-9774-y
Energy Institute (2023). Statistical Review of World Energy. Available at: https://www.energyinst.org/statistical-review (accessed September 6, 2024).
Esposito Vinzi, V., Chin, W. W., Henseler, J., and Wang, H. (Eds.). (2010). Handbook of Partial Least Squares: Concepts, Methods and Applications. Berlin: Springer Berlin Heidelberg.
Fornell, C., and Larcker, D. F. (1981). Evaluating structural equation models with unobservable variables and measurement error. J. Mark. Res. 18, 39–50. doi: 10.1177/002224378101800104
Frankowski, J., and Herrero, S. T. (2021). What is in it for me? A people-centered account of household energy transition co-benefits in Poland. Energy Res. Soc. Sci. 71:101787. doi: 10.1016/j.erss.2020.101787
Gana, K., and Broc, G. (2018). Structural Equation Modeling with Lavaan. Hoboken, NJ: John Wiley and Sons, Inc.
Guillemin, F., Bombardier, C., and Beaton, D. (1993). Cross-cultural adaptation of health-related quality of life measures: literature review and proposed guidelines. J. Clin. Epidemiol. 46,1417–1432. doi: 10.1016/0895-4356(93)90142-N
Guo, J. (2022). Influencing factors of college students' use of sports apps in mandatory situations: based on UTAUT and SDT. Biomed Res. Int. 2022, 1–12. doi: 10.1155/2022/9378860
Hagger, M. S., Hardcastle, S. J., Chater, A., Mallett, C., Pal, S., and Chatzisarantis, N. L. D. (2014). Autonomous and controlled motivational regulations for multiple health-related behaviors: between- and within-participants analyses. Health Psychol. Behav. Med. 2, 565–601. doi: 10.1080/21642850.2014.912945
Hair, J. F., Hult, G. T. M., Ringle, C., and Sarstedt, M. (2017). A Primer on Partial Least Squares Structural Equation Modeling (PLS-SEM), 2nd Edn. Los Angeles, CA: Sage.
Hendryx, M., Zullig, K. J., and Luo, J. (2020). Impacts of coal use on health. Annu. Rev. Public Health 41, 397–415. doi: 10.1146/annurev-publhealth-040119-094104
Henseler, J., Ringle, C. M., and Sarstedt, M. (2015). A new criterion for assessing discriminant validity in variance-based structural equation modeling. J. Acad. Mark. Sci. 43, 115–135. doi: 10.1007/s11747-014-0403-8
Henseler, J., Ringle, C. M., and Sarstedt, M. (2016). Testing measurement invariance of composites using partial least squares. Int. Mark. Rev. 33, 405–431. doi: 10.1108/IMR-09-2014-0304
International Energy Agency (2021a). Mongolia - Countries and Regions. Available at: https://origin.iea.org/countries/mongolia/coal (accessed March 14, 2024).
International Energy Agency (2021b). Key World Energy Statistics. Available at: https://www.iea.org/reports/key-world-energy-statistics-2021 (accessed July 5, 2024).
International Energy Agency (2022). Coal in Net Zero Transitions: Strategies for Rapid, Secure and People-Centered Change. Paris: OECD.
Jain, N. K., Bhaskar, K., and Jain, S. (2022). What drives adoption intention of electric vehicles in India? An integrated UTAUT model with environmental concerns, perceived risk and government support. Res. Transport. Bus. Manag. 42:100730. doi: 10.1016/j.rtbm.2021.100730
Kang, H., Chen, G., and Luna-Reyes, F. (2022). Understanding citizens' perception of government fiscal information through a survey experiment. Govern. Inform. Q. 39:101661. doi: 10.1016/j.giq.2021.101661
Kaur, P., Rajveer, K. R., and Kaur, A. (2024). Unraveling consumer behavioral intentions to adopt solar water heaters in India using UTAUT model. Built Environ. Proj. Asset Manag. 14:312. doi: 10.1108/BEPAM-07-2023-0118
Kline, R. B. (2016). Principles and Practice of Structural Equation Modeling, 4th Edn. New York, NY: Guilford Press.
Koh, C., Prybutok, V. R., Ryan, S. D., and Wu, Y. (2010). A model for mandatory use of software technologies: an integrative approach by applying multiple levels of abstraction of informing science. Inform. Sci. Int. J. Emerg. Transdiscip. 13, 177–203. doi: 10.28945/1326
Leo, F. M., Mouratidis, A., Pulido, J. J., López-Gajardo, M. A., and Sánchez-Oliva, D. (2022). Perceived teachers' behavior and students' engagement in physical education: the mediating role of basic psychological needs and self-determined motivation. Phys. Educ. Sport Pedag. 27, 59–76. doi: 10.1080/17408989.2020.1850667
Liu, C., Xiong, K., Chen, X., and Huang, X. (2023). Consumer behavior patterns of carbon neutral label using the Unified Theory of Acceptance and Use of Technology. Chinese J. Popul. Resour. Environ. 21, 137–144. doi: 10.1016/j.cjpre.2023.09.002
Madigan, R., Louw, T., Wilbrink, M., Schieben, A., and Merat, N. (2017). What influences the decision to use automated public transport? Using UTAUT to understand public acceptance of automated road transport systems. Transport. Res. Pt. F Traff. Psychol. Behav. 50, 55–64. doi: 10.1016/j.trf.2017.07.007
Marikyan, D., and Papagiannidis, S. (2023). “Unified Theory of Acceptance and Use of Technology: a review,” in Theory Hub Book, ed. S. Papagiannidis. Available at: https://open.ncl.ac.uk (accessed July 20, 2024).
Mongolian Department against Air and Environmental Pollution (2022). Available at: https://aprd.ub.gov.mn (accessed October 26, 2022).
National Statistics Office of Mongolia (2024). Mongolian Statistical Information Service. Available at: https://www.1212.mn/en (accessed May 15, 2024).
Pak, T.-Y., Bae, B., Lee, C., Jung, I., and Jang, B.-J. (2023). Modeling public acceptance of demand-responsive transportation: an integrated UTAUT and ITM framework. J. Public Transport. 25:100067. doi: 10.1016/j.jpubtr.2023.100067
Ringle, C. M., Wende, S., and Becker, J. M. (2021). SmartPLS 4. Boenningstedt: SmartPLS GmbH; IBM Corp. Available at: http://www.smartpls.com.
Sarstedt, M., Ringle, C. M., and Hair, J. F. (2022). “Partial least squares structural equation modeling,” in Handbook of Market Research, eds. C. Homburg, M. Klarmann, and A. Vomberg (Cham: Springer Books), 587–632.
Shmueli, G., and Koppius, O. R. (2011). Predictive analytics in information systems research. MIS Q. 35, 553–572. doi: 10.2307/23042796
Sledgianowski, D., and Kulviwat, S. (2009). Using social network sites: the effects of playfulness, critical mass and trust in a hedonic context. J. Comp. Inform. Syst. 49, 74–83. doi: 10.1080/08874417.2009.11645342
Sovacool, B. K., and Hess, D. J. (2017). Ordering theories: typologies and conceptual frameworks for sociotechnical change. Soc. Stud. Sci. 47, 703–750. doi: 10.1177/0306312717709363
UNICEF Mongolia (2023). Impact of Air Pollution on Maternal and Child Health Project (2018-2023). Available at: https://www.unicef.org/mongolia/reports/impact-air-pollution-maternal-and-child-health-project-2018-2023 (accessed May 28, 2024).
Unified Legal Information System (Mongolia) (2024). Available at: https://legalinfo.mn (accessed April 10, 2024).
Venkatesh, V., Morris, M. G., Davis, G. B., and Davis, F. D. (2003). User acceptance of information technology: toward a unified view. MIS Q. 27, 425–478. doi: 10.2307/30036540
Wasko, M., and Faraj, S. (2005). Why should I share? Examining social capital and knowledge contribution in electronic networks of practice. MIS Q. 29, 35–57. doi: 10.2307/25148667
WHO (2022). Ambient (Outdoor) Air Pollution. Available at: https://www.who.int/news-room/fact-sheets/detail/ambient-(outdoor)-air-quality-and-health.
Widyanti, A., Erlanga, R., Sutarto, A. P., Trapsilawati, F., Ansori, N., Woodman, R., et al. (2024). Driving forward together: the common intention of indonesians in different residential areas to use autonomous vehicles. Transport. Res. Interdiscipl. Perspect. 24:101049. doi: 10.1016/j.trip.2024.101049
Keywords: Ulaanbaatar, citizens' acceptance, UTAUT model, improved fuel, air pollution
Citation: Batkhuyag U (2025) Citizens' acceptance and use of new technology: the case of improved fuel in Ulaanbaatar. Front. Sustain. Energy Policy 3:1508302. doi: 10.3389/fsuep.2024.1508302
Received: 09 October 2024; Accepted: 31 December 2024;
Published: 21 January 2025.
Edited by:
Hongguang Nie, Liaoning University, ChinaReviewed by:
Amin Vafadarnikjoo, The University of Sheffield, United KingdomIsa Ferrall-Wolf, National Renewable Energy Laboratory (DOE), United States
Copyright © 2025 Batkhuyag. This is an open-access article distributed under the terms of the Creative Commons Attribution License (CC BY). The use, distribution or reproduction in other forums is permitted, provided the original author(s) and the copyright owner(s) are credited and that the original publication in this journal is cited, in accordance with accepted academic practice. No use, distribution or reproduction is permitted which does not comply with these terms.
*Correspondence: Undrakh Batkhuyag, YmF0a2h1eWFnLnVuZHJha2guNjZ6QHN0Lmt5b3RvLXUuYWMuanA=