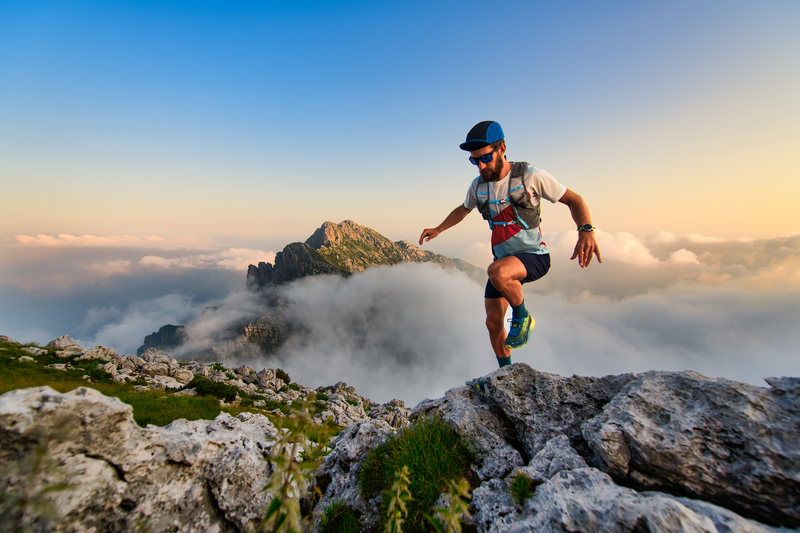
95% of researchers rate our articles as excellent or good
Learn more about the work of our research integrity team to safeguard the quality of each article we publish.
Find out more
ORIGINAL RESEARCH article
Front. Sustain. Energy Policy , 01 August 2023
Sec. Energy Quality and Efficiency
Volume 2 - 2023 | https://doi.org/10.3389/fsuep.2023.1204650
This paper constructs a comprehensive electricity market model in the context of China, highlighting the deviation caused by neglecting start-up costs from an engineering perspective. The model allows for the abandonment of excess wind and solar power generation, contributing to the achievement of research objectives in scenarios with a high proportion of renewable energy. Our method innovatively integrates fuel and carbon prices, clean energy expansion, and power system marginal prices according to the carbon trading rules of the Chinese power industry, providing a more accurate representation of market dynamics. Findings reveal that neglecting start-up costs can lead to significant biases in electricity prices. We demonstrate that the marginal price sometimes deviates from the fluctuation of the real value. While fuel and CO2 prices can be transmitted downstream, the value of new energy must be transmitted through its impact on the marginal unit. This insight is crucial for understanding the “missing money” problem in electricity markets. Based on these findings, we propose policy recommendations. We suggest considering fixed and average costs as pricing benchmarks and utilizing capacity utilization as a signal for demand response to adjust power pricing. Furthermore, we recommend trading different energy types separately in the spot market with different pricing benchmarks to ensure the homogeneity of marginal units.
With the increasing global concern over climate change, countries worldwide are accelerating clean development, addressing climate change, and promoting energy transition. China has actively responded and acted in this regard. President Xi Jinping, in his speech at the 75th session of the United Nations General Assembly on September 22, 2020, outlined China's carbon reduction goals—striving to peak carbon dioxide emissions by 2030 and achieve carbon neutrality by 2060. As the primary carbon-emitting industry, the electricity sector bears the responsibility of emissions reduction. Therefore, in order to respond to the “dual carbon” goals and drive the transformation and upgrading of the power system, China proposed important directions for deepening power system reforms and building a new power system predominantly based on renewable energy during the 9th meeting of the Central Financial and Economic Affairs Commission on March 15, 2021.
As the share of zero-marginal-cost variable renewable energy (VRE), such as wind and solar power, continues to rise, conventional power generation faces challenges of more frequent and rapid fluctuations in electricity system demand. To facilitate the transition toward a cleaner and more efficient power system, electricity pricing should be based on marginal cost principles, addressing the intermittent nature of VRE and appropriately compensating for the fixed costs of power generators.
To improve resource allocation efficiency in the electricity sector, China has committed to power market reforms. The reform was initiated in 2015, with a landmark policy being the issuance of the “Opinions on Further Deepening the Reform of the Power System.” This policy called for increased use of market mechanisms, improved energy efficiency, and increased utilization of renewable energy and distributed generation. Subsequently, in February 2022, the National Development and Reform Commission and the National Energy Administration issued the “Guiding Opinions on Accelerating the Construction of a National Unified Power Market System,” aiming to accelerate the formation of a unified, open, competitively ordered, safe, efficient, and well-governed power market system based on the principles of market operation and market economy. Currently, China has launched two batches of pilot electricity spot markets. In addition, many provinces have announced the cancellation of electricity price regulation for industrial and commercial sectors and are gradually increasing the proportion of floating electricity prices for industrial and commercial consumers. China's electricity market pricing mechanism is gradually transitioning from regulated pricing and catalog prices to a market-based price formation mechanism that restores the commodity attributes of electricity.
Inadequate adaptation of electricity prices to the market can lead to a failure to transmit the scarcity signal downstream, potentially resulting in power shortages and crises. For example, in 2021, China experienced electricity shortages, with over 20 provinces implementing power rationing and production restrictions. One of the reasons behind this issue was unfavorable weather conditions for renewable energy generation, while on the other hand, coal-fired power plants faced restricted fuel prices that couldn't be passed downstream, leading to production reductions. Similar situations have also occurred in the European Union, where rising natural gas prices, increasing carbon emission quota prices, and declining renewable energy output have resulted in soaring electricity prices. Additionally, with the establishment of the Chinese carbon emissions trading market, the fluctuation of carbon emission costs also affects generation costs, highlighting the need for attention. Therefore, the impacts of fuel prices, carbon emission costs, and renewable energy on the electricity market cannot be overlooked, and these factors should be transmitted downstream through electricity prices to provide economic incentives for demand-side balancing of electricity supply and demand.
Research has shown that China's transition from “three equity” dispatch principles to market-oriented economic dispatch can significantly reduce the operational costs of the power system and improve the system integration capacity of wind and solar power generation (IEA, 2018). Given the ongoing reforms in China's power industry, it is of significant importance to study the price formation under market-oriented economic dispatch, considering factors such as renewable energy, start-up costs, coal-fired power prices, and carbon emission costs. Therefore, this paper aims to study the price modeling of non-convex electricity markets through models and scenario simulations, elucidating the challenges posed by the increasing penetration of VRE and proposing corresponding solutions and policy recommendations.
The driving force behind conducting this particular study is the urgent need to address the challenges and complexities posed by the increasing penetration of renewable energy, the pricing dynamics influenced by fuel and carbon costs, and the need for efficient and incentive-compatible pricing mechanisms in the electricity market. By studying the price modeling of non-convex electricity markets, this research aims to contribute to the development of effective pricing policies, resource allocation efficiency, and the successful transition to a cleaner and more sustainable power system in China.
The research gaps identified in the study are the overlooked aspect of start-up costs in electricity price modeling and the need for a more accurate representation of market dynamics by integrating various factors such as fuel and carbon prices, clean energy expansion, and power system marginal prices. So, we focus on the following questions in this study: (1) Why is a non-convex model that considers start-up cost closer to reality? (2) What are the effects of fuel costs, CO2 emission costs, and intermittent renewables on the marginal price of electricity? and, (3) Why is the marginal pricing insufficient especially as intermittent renewable energy sources expand?
We contribute to the field of electricity pricing and market modeling by developing an optimization model that considers variable renewable energy sources and the start-up costs, thereby providing a more accurate representation of electricity market dynamics. The current research is important due to its relevance in addressing key challenges and advancing knowledge in the field of electricity price modeling in non-convex markets, particularly in the context of China's power sector reforms and its commitment to clean development.
Electricity pricing is central to the study of electricity markets (Joskow and Wolfram, 2012; Dutta and Mitra, 2017). In deregulated markets, electricity suppliers submit their price and quantity bids to the market operator, which determines the optimal dispatch and resulting payments to suppliers. There is a large body of research addressing the design and implementation of auctions in these markets, focusing on aspects of bidding behavior, market price calculations, and resulting dispatch efficiency (Parker et al., 2019). As a result, scholars have attempted to understand the conditions under which consumers pay enough for electricity to allow generating companies to recover their fixed costs. The general conclusion is that traditional marginal cost pricing on the supply side of the “energy only” market is inadequate due to factors such as start-up cost, outage costs, and minimum load requirements. Marginal suppliers cover the long-term investment costs by obtaining net revenues, but still some firms are unable to recover fixed costs, the so-called “missing money” problem (Cramton et al., 2013). As a solution, many argue that generating companies should be compensated for their generating capacity in addition to the energy gains from the sale of electricity (Cramton and Stoft, 2005). However, the increase in zero marginal cost renewables has again exacerbated the “missing money” problem. This is because high penetration of wind and solar resources will require frequent loading and unloading of conventional generating units to cope with the fluctuations of intermittent generation (Wu and Kapiscinski, 2013). Another option for dealing with intermittent generation is the use of energy storage devices, which will soon become an economically viable option for supporting the integration of high percentages of renewable energy.
Demand response electricity pricing is now becoming an actively evaluated area (Patnam and Pindoriya, 2021), resulting in dynamic electricity prices such as time-of-use (TOU) pricing and real-time pricing (RTP) that allow scarcity of generation in the wholesale market to be communicated to end consumers (Joskow and Wolfram, 2012; Lin et al., 2022; Wesseh and Lin, 2022). However, their pricing benchmarks are still inseparable from the average cost of electricity and the marginal price of electricity in the wholesale market. The literature on marginal cost pricing models for electricity markets dates directly back to the mid-20th century (Joskow, 2019), and as market elements continue to be added, extensions to the model will bring new applications.
The analysis of marginal pricing in wholesale electricity markets can be divided into four main categories: optimization models, equilibrium models, simulation, and empirical studies. Optimization models use linear programming, mixed-integer linear programming, and heuristic algorithms designed to bring mathematical models close to real system dispatch and are applied to investment planning for generation equipment and transmission lines, as well as to the calculation of marginal node tariffs for the system, and to predict the possible outcomes of future power system planning (van Ouwerkerk et al., 2022). The main advantage of optimization models is that they can introduce a large number of technical and economic constraints for large-scale models. However, the focus on a single objective simplifies the complexity of market behavior, making it difficult to integrate price-demand response on the consumer side with large-scale system optimization problems and their shadow prices. In optimization models, there is significant attention given to transforming the non-convex original problem into a convex optimization problem through mathematical derivations in pricing scheme design (Daraeepour et al., 2022). Equilibrium models satisfy both the first-order conditions (Karush-Kuhn-Tucker, KKT) for maximizing the profits of market participants and the market clearing condition of equal supply and demand. In equilibrium models, the supply side and the demand side are linked through price variables and solved as a mixed complementarity problem (MCP), which can be used for government pricing, such as the formulation of time-of-use pricing policies. This requires the original problem to be a convex optimization problem (Wesseh and Dogah, 2022; Wesseh et al., 2022), whereas for many specific issues in electricity markets, such as start-up cost (which require binary decisions), the convexity assumption is incorrect. Simulation models model market participants as goal-oriented and adaptive computational agents, which facilitate dynamic analysis (Richiardi, 2003), but the use of a specific learning algorithm is rarely justified explicitly, and most of the literature lacks validation of the empirical model and interpretation of the results. There are also a number of studies (e.g., Reguant, 2014) that empirically investigate the relationship between pricing mechanisms and marginal costs, as well as start-up costs and the bias of ignoring start-up costs in electricity markets, but the empirical analysis requires high data levels and there is a lack of bidding data for the liberalized market as China is in the initial stage of the electricity spot market.
Based on the existing research mentioned above, this paper provides an engineering perspective to elucidate the bias of neglecting startup costs and constructs a comprehensive electricity market model within the context of China. One minor innovation of the model is its allowance for the curtailment of excess wind and solar power generation, which facilitates the achievement of research objectives in scenarios with high levels of renewable energy integration.
To achieve the above objectives, this paper adopts the optimization model of literature (Weigt, 2009). The difference is that this paper focuses on the generation of renewable resources and includes them in the model instead of subtracting them directly from the demand. Our model allows for the abandonment of excess wind and solar power generation, contributing to the achievement of research objectives in scenarios with a high proportion of renewable energy. In addition, the model is based on the carbon trading rules of the Chinese power industry. The model does not account for losses and blockages, so the market clearing price is the marginal price. The model uses a minimized total system cost model to estimate the marginal price level under a perfectly competitive market, with the objective function of
where is the marginal cost of unit i in hour t, is the actual output of unit i in hour t, is the start-up cost of unit i in hour t when it has to come online, is the cost of the unit to ramp up and ramp down, CFi is the emission factor per MW of the particular technology, is the emission intensity benchmark/quota for the carbon trading scheme, and is the carbon price. The last term indicates the climate cost, i.e., the penalty/allowance for increasing/reducing carbon emissions.
The unit generation cost of the unit is expressed as
In hour t, includes fuel cost based on fuelconsumpi and , and operation and maintenance cost O&M costi.
Unit start-up costs are expressed as a function containing binary decision variables.
is the start-up fuel cost required for heating, driven by the fuel price that varies with time t. indicates whether the unit starts, 1 if it does, 0 otherwise. The values of are 0, 1, and −1 for the unit start-up states remain constant, from offline to on, and from online to off, respectively. A value of −1 from online to off has no effect because the actual output is 0, making equal to 0. The initial value of unit state is the unit state of the last period of the last calculation cycle.
The cost function of a thermal power unit is not only related to the unit output, but also has a direct relationship with the ramping rate of the unit at adjacent moments. In this paper, the ramping cost of thermal units is measured as a linear function of the climbing rate, and the calculated function is
where rampfac is the ramping cost factor for thermal units, is the change of power in neighboring hours, i.e., ramp up or ramp down rate. The ramping cost factor is 25 for coal units and 35.5 for nuclear units.
(1) Capacity constraint
indicates that the power generation is limited by the upper and lower boundaries. Where is the maximum available power output, is the minimum necessary output, and is a binary conditional variable with the value of 1 when the unit is online and 0 when it is offline.
(2) Start-up constraint
indicates the necessary start-up time of the unit from shutdown to start-up. Where li is the necessary start-up time of unit i. If the initial state of the unit is “online”, when the unit comes offline, the left side of the equation is 1, and then the condition variable must be 0 at τ hours to restrict the unit to start. If the initial state of the unit is “offline”, the unit can be ready to start at any time in advance, and the constraint does not create a restriction.
(3) Energy balance
where dt is the demand d in hour t, is the process of pumping water from the pumped storage facility to store energy, and is the process of releasing water from the pumped storage facility to release energy. The dual variable of this equation is the marginal price of electricity generation.
(4) Storage equation
The storage process of the pump is the inter-period condition, where is the available storage capacity of the pumped storage facility for t hours. It is assumed that the efficiency of the pumped storage facility is 75%, that is, if it is in pumping mode (), 75% of the energy consumed by the pump during pumping, by converting into gravitational potential energy of water and then into electrical energy for any time needed, can finally be used in the next stage of power generation. If in power generation mode (), the appropriate amount of energy will be removed from the pumped storage facility. The initial storage is assumed to be 0.
(5) PSP constraint 1
The process of energy storage and power generation of the pumped storage equipment is limited by capacity, PSPmax is the maximum capacity of the pumped storage power plant, which is the maximum value that can be achieved by the total amount of storage and extraction.
(6) PSP constraint 2
The pumped storage facilities do not generate more power than the available storage capacity.
(7) Wind, solar, and hydro constraint
denotes the actual contribution of wind power generation, denotes the available output according to the wind resource status, grid connection conditions, etc., and the same for solar power. Although the marginal cost of wind and solar power generation is zero, in order to balance their intermittent generation, additional start-stop and climbing of other units may be required. Given the negative externality of wind and solar power generation, their generation can be discarded when the value of using resources for balancing them is higher than the value they bring by themselves. Hydropower has a certain regulation ability, because it can control the valve for water storage or abandonment based on the demand of the power system on the basis of the hydraulic resources in that period of time, and its upper limit is set as a factor γmax multiplied by the average hourly generation of hydropower in that month. However, the total amount of hydropower generation needs to be within the range of available hydraulic resource conditions over a period of time.
Finally, considering the possibility that the demand for electricity exceeds the supply, in order to make the model feasible to solve and calculate the shortage of electricity under the optimal conditions, so as to facilitate the early warning of electricity shortage and the prediction of “orderly electricity consumption” policy, or to further the pricing of electricity scarcity, this paper introduces a positive variable xt to represent electricity shortage. In the power shortage scenario, the objective function should also take into account the losses caused by the power shortage:
where ρt is the social loss per unit of power shortage, and costs′ denotes the cost of the power shortage scenario, which is the cost of the original objective function plus the power shortage loss.
The balancing constraint requires consideration of the power shortage component as a demand that the system cannot meet:
The model is implemented in GAMS as a combination of a mixed integer problem for the unit commitment and an optimization problem with fixed binary condition variables for the actual dispatch. The dual variable on the energy balance constraint is considered to represent the hourly market price.
Since the import and export of electricity in Fujian Province, China, are basically the same, and there is no need to consider too much the inter-provincial transmission, this paper takes Fujian Province, as an example, to model the main generating units. Conventional energy includes 59 coal thermal power units and 12 combined cycle gas and steam (CCGT) units, whose capacity and pressure parameters are public data for classifying various performance parameters of different units. For new energy (nuclear power, wind power, solar power) this paper uses the total installed capacity data of Fujian Provincial Bureau of Statistics in 2021, which means the summation of the capacity of all units. There is 698332 GW of installed power generation capacity in Fujian Province at the end of the year in 2021, and the share of thermal, hydro, nuclear, wind, solar, and other installed power capacities are 51.50, 19.84, 14.12, 10.53, 3.97, and 0.04%, respectively.
The basic model involves two scalars, the pumped storage capacity and CO2 price. The former is based on the fact that there are currently four pumped storage power plants with a total of 5,000 MW in operation in Fujian province, the latter is based on the fact that the carbon price is basically around 50 yuan without major fluctuations, despite the carbon emissions trading in the national power sector starting on July 16, 2021. The decision variables are the binary decision variable for each thermal unit and the generation capacity of each unit (including the pumped storage).
The coal consumption parameters of thermal power units are based on the values of the latest national standard documents. The average carbon intensity of the corresponding type of units in the literature is referred to, and the literature divides the monitoring of carbon intensity of coal units into three scenarios: bituminous coal case, balanced case, and default case. The average CO2 intensity of the units in this paper is based on the “most likely” balanced case, which assumes that small units (circulating fluidized bed, high pressure, and subcritical units below 600 MW) will use the default value of 123 kgCO2/GJ due to lack of reporting and verification (MRV) capability. Supercritical and ultra-supercritical units, as well as large subcritical units above 600 MW, monitor their CO2 fuel factor and apply the IPCC value of 95 kgCO2/GJ. The carbon quotas are based on the latest quota standards (IEA, 2020a,b).
The data in this paper are mainly integrated using historical data, and the specific integration process is described in this section. It should be emphasized that if the model is to be applied in practice, these data should be predicted values measured by professional methods. The forecasts are assumed to be known and used as input parameters in this paper. In addition, the analysis in this paper is performed on a system-wide basis, where transmission constraints are not considered, and future research could take transmission constraints into account.
The total social electricity consumption in Fujian Province in 2021 is 283.666 billion kWh. Since some small thermal power units are not publicly available, the units involved in this paper cover 96.367% of the installed capacity, so the simulated total demand is also multiplied by that ratio. Based on the month-by-month electricity consumption in Fujian Province in 2021 and the electricity consumption curve of a region with 8,760 h, the electricity consumption curve of Fujian Province in 2021 with 8,760 h is simulated.
In 2021, fuel prices for power generation (gas and nuclear) are relatively stable, except for the volatile market price of power coal (see Figure 1). Therefore, except for coal-fired power plants using coal prices and coal consumption, other power plants are substituted with average marginal cost data.
Thermal and nuclear power are dispatchable power generation facilities, but solar power generation, wind power generation, and hydropower generation, which depend on the natural environment, cannot be fully controlled artificially, and have limited decision-making space, and need to be simulated based on meteorological data and historical power generation data. It is worth noting that the region has more wind energy and less solar energy in winter, and more solar energy and less wind energy in summer. With the increase in solar power scale, wind and solar can complement each other to a certain extent.
For solar photovoltaic power plants with defined engineering characteristics such as modules, specifications, and installation methods, the power output mainly depends on meteorological conditions, among which the dominant factors are solar radiation irradiance and temperature. On the one hand, the irradiance is proportional to the photocurrent of the solar photovoltaic cell, and the photocurrent always increases linearly with the irradiance in the range of 100–1,000 W/m2, while the irradiance has little effect on the photovoltage; on the other hand, when the temperature increases, the shape of the current-voltage curve will change, leading to a decrease in the photoelectric conversion efficiency. The hourly solar radiation data in this paper were obtained from Meteonorm software, and the month-by-month data of total solar power generation were obtained from the Bureau of Statistics and “Wind Database”, respectively, by weighting the total solar power generation of each month to each hour of that month.
Wind power generation is highly intermittent, volatile, and uncertain. In systems with high wind generation penetration, this can lead to the use of frequent unit commitment decisions to maintain acceptable unit commitment risk, and therefore, it is important to include wind generation in the model. Similar to solar power, the hourly wind speed data in this paper are obtained from Meteonorm software, and the month-by-month data for total wind power generation are obtained from the Bureau of Statistics and the “Wind Database”. The difference is that the wind speed is not linearly related to the wind turbine power, and the wind speed needs to be within a certain range to generate electricity. According to hydrodynamics, the correspondence between the output mechanical power PM and wind speed v is as follows:
Where CP is the wind energy utilization coefficient; vin is the wind speed cut in; vn is the rated wind speed; vout is the wind speed cut out; ρ is the air density; S is the impeller swept area; Pn is the rated power of wind turbine.
The parameter setting of this paper refers to the 1.5 MW wind turbine produced by a leading wind power company. The cut-in wind speed is 3 m/s, the rated wind speed is 12 m/s, and the cut-out wind speed is 25 m/s. The curves are combined with the actual wind speed data in the area to derive the hourly power data, and then estimate the grid-connected wind power capacity of 735.02 million kilowatts in Fujian Province.
Hydropower has a certain amount of water storage and regulation. The total amount of hydropower generation is obtained from the statistics bureau and “Wind Database” on a month-by-month basis. It is assumed that the water flow is smoothly generated in a month with a 30% free adjustment.
In this paper, a week of the representative month of each season (January, April, July, and October represent winter, spring, summer, and autumn, respectively) is selected as the representative week, and the data representing 168 h of each season are applied into the model separately, i.e., the results of 4 × 168 h under the assumption of a perfectly competitive market are obtained. This case represents a typical scenario in the Chinese electricity market, encompassing various types of power generation units, including coal-fired, gas-fired, nuclear, and renewable energy. The case also focuses on factors such as startup costs of power units, carbon trading rules in the Chinese electricity industry, and fluctuations in fuel prices, all of which have significant impacts on the dynamic pricing in the comprehensive electricity market.
The marginal prices with and without start-up costs are compared to reflect the impact of the model start-up cost variable.
Figure 2 shows the hourly marginal electricity price with and without start-up cost model, and Figure 3 shows the number of hourly units online for each of these two models, respectively.
Fall has the highest marginal price, which is due to the selected 2021 spike in coal prices, resulting in higher fuel costs. Summer is the next highest; this is because it is at peak load and most units (regardless of efficiency) will be deployed. The highest demand is essentially when electricity prices peak, and vice versa. In the absence of dramatic fluctuations in fuel prices, generally the higher the electricity use, the higher the marginal price, in line with the basic intuition of price-demand trends. However, since units have maximum and minimum generation limits and the supply curve is stepped, demand may correspond to the same price for small fluctuations within a certain range.
The marginal price volatility calculated by a model that ignores start-up cost would be biased downward because the unit start-up decision would be able to produce more frequent changes to fit the objective function according to the optimization objective, smoothing the supply “ladder” curve, but in reality, the flexibility of the unit start-up is not cheap, and it is not advisable to start-up frequently. This is particularly evident in October and not in July. Because in October, the model that ignores start-up cost has a large fluctuation as the marginal unit is constantly switching between coal-fired and gas-fired units, but it actually has much less flexibility. In July, the units are almost in full load, the space of flexibility is very small, and marginal price and volatility of the two models (with and without start-up cost) are almost indistinguishable. In addition, the deviation in the size of the marginal price depends on whether a start-up actually occurs (a large online unit capacity utilization would increase the likelihood of starting a new unit) and the cost of the more flexible marginal unit.
The marginal price calculated by a model that ignores start-up cost will produce an upward or downward bias. One situation is that overestimating the start-up frequency will bias the marginal price of the ignored start-up cost model downward. In the other case, since the units have minimum generation requirements, ignoring start-up cost would result in more use of smaller units with lower minimum generation requirements, greater flexibility, and slightly higher costs, with little or no deviation or a slight increase in marginal prices.
If the model ignores start-up cost, it is clearly not common sense that the optimization results will have unlimited free start-ups and shutdowns for each unit. However, excluding the start-up and shutdown of each unit, the other values are basically consistent with common sense, so some literature is directly used to simplify the analysis. In this paper, we analyze this slight deviation, and how it expands.
The results of the model with and without start-up cost show the average marginal price, average cost, average capacity utilization, and generator profitability for the study period, as shown in Table 1. Capacity utilization here refers to thermal and nuclear generation/online unit capacity and is used to characterize online unit load utilization.
As mentioned earlier regarding the average marginal price, ignoring the start-up cost may produce upward or downward bias. The downward bias is due to the overestimation of the frequency of startups occurring during the study period, allowing free choice of low-cost units. The upward bias is due to the fact that low-cost advanced units tend to have larger minimum loads and may not meet minimum generation requirements despite relaxed start-up conditions. The model selects the latter between advanced units with low-capacity utilization and advanced units + flexible units combined with high-capacity utilization, with marginal units likely to be those with smaller minimum loads, more flexible, and higher marginal costs such as high-pressure units and gas-fired units. Although start-up costs are a low percentage of total costs, they are an essential variable in generation decisions. The average cost of a unit without start-up cost will produce an overall downward bias, but the actual cost to the plant will be much more than that. Ignoring the start-up cost model's wrong decision makes the already existing “missing money” problem even worse, and severely overestimates capacity utilization.
In order to verify the “missing money” problem in the deregulated market and the effect of start-up cost on the model results, the net income of each unit is compared in the results of the model with start-up cost (Model 1 net income), the model with start-up cost but minus start-up cost in result (Model 1 net income except start-up cost), and the model without start-up cost (Model 2 net income). The figure shows the net income of each unit during the study period. The horizontal coordinates indicate the different thermal units, the first 33 units are coal units above 600 MW, the numbers 34–59 are units below 600 MW, and the numbers 60–71 are gas units.
As can be seen from Figure 4, without a start-up cost model net income will produce a downward or upward bias and is not the effect of start-up cost itself (compared to model 1 except for start-up cost, start-ups are infrequent and start-up costs are small), but rather the result of different generation decisions leading to different marginal prices. Small capacity units have some losses in January and April, which means that not only do these units not recover their fixed costs, they do not earn enough revenue from generation to cover the variable costs. With sufficient generation supply, gas-fired units (except for the October coal price increase when gas was used heavily as a substitute) and some inefficient coal units generated very little revenue, clearly not enough to cover fixed costs. This is the problem of “missing money” in the “energy only” market. Therefore, a capacity market is necessary to cover the fixed costs of spare capacity.
In addition, the net income from nuclear and new energy should also be taken into account, as shown in Figure 5. The unit generation cost of nuclear power is slightly higher than that of coal power, and lower than gas power. When the demand is low and thermal fuel costs are normal, nuclear power competing with coal power in the same market will incur losses, and conversely, in higher demand (July, compete with gas power) or higher thermal fuel cost prices (October) can be profitable, thus generators may take contractual measures to hedge uncertainty. Zero marginal cost hydropower, wind power, and solar power in the absence of abandoned power net income are directly related to the generation and market prices for offsetting fixed costs and profits.
In order to better understand the sensitivity of the marginal price of electricity to important drivers, a sensitivity analysis was conducted on a specific set of parameters. The base scenarios for coal price, CO2 price, and CO2 free quota are: 750 yuan/t, 50 yuan/t, 100%, respectively.
As can be seen from Figure 6, the marginal price of power generation varies regularly under different coal prices, and the marginal price converges in some periods because the marginal unit in that period is a gas-fired unit.
As can be seen from Figure 7, coal prices and average unit costs are linearly related, with each 100 yuan increase in coal prices increasing average unit costs by 0.025 yuan/kWh. In general, the higher the coal price, the higher the average marginal price of electricity, but not strictly linear, because marginal cost is not the average cost, but only the shadow price of the last unit of electricity consumed, and where this unit falls is derived from the overall optimization results. When the cost of coal increases to a certain level it will change the generation sequence of the units so that their economics are surpassed by nuclear and gas power, and the marginal units then change, especially when the electricity consumption is high. This is not the case with average unit costs, but the price difference between average costs and marginal prices is actually reflected in producer surplus, and the slowdown in marginal prices results in a significant reduction in generator earnings.
In addition, we can see that at a coal price of 500 yuan/t, generators have negative returns in January and April, and are “missing money” without counting fixed costs. This is due to the optimal decision to meet demand with low-capacity utilization of operating units (about 72%), marginal units that can be advanced units, low marginal prices, nuclear power, and higher cost units that must generate power with insufficient revenue to cover costs. This is also intuitively understood because once a new unit is turned on to meet at least the minimum load requirements, the original unit may generate less power, and the unit's idle capacity results in a waste of producer resources. Carbon intensity decreases with higher coal prices as more alternatives to coal-fired units such as gas-fired units and nuclear power will be used due to higher coal prices.
As can be seen from Figure 8, there is no certain pattern of marginal price change at different CO2 prices because some units need to buy allowance and some units have an extra allowance for sale. The marginal price is the highest when the carbon price is zero in July, because more than half of the units have an excess allowance which has priority due to their advanced generation, and all units will be turned on in July, so there will be excess allowances for sale from the whole generators.
As can be seen from Figure 9, for every 100 yuan increase in CO2 price, the average unit cost decreases by 0.004–0.007 yuan/kWh, where the average unit cost decreases by a smaller amount in July. This is because under the current power sector carbon quota, more than half of the units generating have allowances left over and the other part needs to purchase additional allowances for emissions. In July, units with higher carbon emissions will also come in handy due to high loads. The marginal price fluctuates within a small range, as the more advanced units are ahead in the generation sequence and the more backward units are behind, and the marginal unit may swing between the two, with the trade-off between the two forces forming the marginal price.
However, the price at this point does not reflect the true cost of carbon emissions, as the free emission allowances (benchmarks) are different, and the cost of CO2 emissions will be small after allowances are subtracted. Units with excess allowances for sale are inherently dominant in a perfectly competitive market due to their technological sophistication. A further increase in CO2 prices would simply increase the already existing incentive for unit improvement and do little to promote low-carbon energy. Adjustments to the free quota amount are therefore needed.
As can be seen from Figure 10, different CO2 free quota will cause different generation decisions and sometimes not. If the decision is the same, then the difference is the reduced allowance itself which has little difference, otherwise will change to the unit that is more environmentally friendly with the different marginal price.
As can be seen from Figure 11, when the CO2 price is 50 yuan, the average unit cost increases by 0.003 yuan/kWh for every 10% reduction in CO2 free quota. The average marginal cost roughly follows this trend, except that in January and April there are fluctuations. This is because with the reduction of free credits, gas-fired units gradually show the advantage of fewer carbon emissions than coal-fired units, which may be selected for generating units even if the demand is low and the marginal units swing between the two. July and October are used by gas-fired units, so the impact is less. The trend of profitability is the same as the marginal price. The lower the free allowance, the lower the carbon intensity, but this trend is almost negligible. Therefore, the current CO2 price and free allowances are too relaxed if carbon trading is to play a role in decarbonizing the power system.
The analysis of large-scale intermittent renewable energy sources is essential to better assess the future electricity market. This paper analyzes the possible scenarios of Renewable extension. We simulate the baseline scenario based on the installed share of wind and solar energy at 30, 50, and 80%, and the energy storage ratio is 10% of the installed wind and solar energy. The results of the start-up cost model and the ignored start-up cost model are shown in Figures 12, 13.
With the expansion of installed scenery, occasionally there will be 0 marginal tariffs, meaning that the marginal condition of consuming one more unit of electricity used is wind or solar power, that is to say, there is abandoned wind and solar. This is because during the time period for both the power consumption valley and capacity utilization valley, there is more intermittent renewable energy generation, and optimal cost objectives do not allow the shutdown of the unit and then restarting for consuming new energy temporarily. With the expansion of wind and solar capacity, the average cost and CO2 intensity of the power market gradually decrease, and the difference between the model with and without start-up cost is not significant, except that the zero marginal cost during the abandoned wind and solar time will pull down the average marginal price. The marginal price goes down too, but is still decided by the marginal unit.
The wind and solar abandonment rate for the 80% wind and solar installation scenario can be up to 15%, while ignoring start-up costs will underestimate wind and solar abandonment. The increase in energy storage will reduce the wind and solar abandonment rate, while relieving electricity tensions during peak usage. However, due to the wind and solar abandonment rate and the seasonal imbalance in electricity demand, it is difficult to determine how much energy storage is needed to both reduce wind and solar abandonment to ease power supply tensions and not sit idle when it is not needed. In order to reduce the waste of energy storage capacity, the development of multi-purpose flexible energy storage such as electric vehicle charging piles, batteries, etc. can be considered.
The net income of the models with and without start-up costs also show some differences. With the expansion of the wind and solar power scale, the net income of the market will gradually decrease, and the income of wind and solar power will account for an increasingly higher proportion, squeezing the net income of coal power and nuclear power, resulting in a more serious “missing money” problem. Although ignoring start-up costs makes the problem worse, factoring in start-up costs does not avoid it, so other means of adjusting prices may be needed. Pumped storage and energy storage net income will also become significant. Ignoring the start-up cost model will underestimate the abandonment rate of wind and solar and will overestimate the share of wind and solar generation. The model's net revenue falls and then rises, which is related to the initial overestimation of the rate of decline in thermal net income and the final overestimation of wind and solar generators' net income. In addition, the role of energy storage is underestimated when ignoring start-up costs because of the overestimation of start-stop flexibility.
Certainly, taking into account the reality that the installed plan should match the demand, the growth of wind and solar installation is accompanied by the growth of demand, and the growth of demand will, to a certain extent, improve the marginal price and ease the “missing money” problem of thermal power, especially nuclear power, and ease the amount of abandoned wind and solar. Energy storage will also change its role from reducing abandoned wind and solar energy when electricity is loose to the role of adjusting peak when electricity is tight, but it is undeniable that wind and solar will take a large part of the total revenue, and the “missing money” problem in the market cannot be solved. New price mechanisms, policies, and market design are needed.
This paper constructs a comprehensive electricity market model in the context of China, highlighting the deviation caused by neglecting start-up costs from an engineering perspective. The model allows for the abandonment of excess wind and solar power generation, contributing to the achievement of research objectives in scenarios with a high proportion of renewable energy. The model can be used to predict marginal electricity prices and highlights the importance of non-convex modeling of start-up cost, showing trends in key indicators such as electricity prices, costs, and benefits under different coal prices, CO2 prices, CO2 free quotas, and a large percentage of intermittent renewable energy resources scenarios. Finally, the issue of “missing money” is highlighted as new energy sources expand.
By comparing the model with start-up cost and the model without start-up cost, the model ignoring start-up cost for power generation will result in an upward or downward deviation in marginal prices and a downward deviation in average costs, but the actual cost to the plant will be much more than that. This bias has some volatility because the model bias is not brought about by start-up cost itself, but by different decisions leading to changes in marginal units (trade-offs between high-capacity utilization of advanced units and advanced units + flexible units combinations). In addition, ignoring the start-up cost model will underestimate the rate of wind and solar abandonment and underestimate the utilization of energy storage under massive new energy expansion.
A sensitivity analysis of the factors influencing electricity prices shows that electricity prices are sensitive and relatively stable to changes in fuel prices, with an average increase of 0.0245 yuan for every 100 yuan increase in coal prices. The sensitivity of electricity price to CO2 price and CO2 free quota amount is negligible unless both change at the same time. Since the marginal price is directly related to the marginal unit, which has a minimum load requirement, the trend of marginal price changes is unstable, and its difference from the stable average price constitutes the unstable net income of the generator.
In the electricity pricing containing large renewable energy, although the marginal price of renewable energy is 0, the marginal price in the electricity market will not be lower than the cost of generation of advanced coal units if there is no abandonment of wind and solar. If electricity demand and new energy capacity grow in tandem (more in line with reality), the marginal price will not be significantly lower. Because the final marginal price of electricity depends on the price of the last generating unit, the small difference in income and significantly lower costs constitute the excess profits of renewable generators, the actual value of renewable is not a high level of transmission to users, and will squeeze the profit of thermal power, exacerbating the “missing money” problem of thermal power units. There are two solutions: (1) Encourage renewable energy to be traded separately and consumed nearby. Renewable energy pricing should provide a more significant demand response mechanism, such as local electricity markets; and, (2) Price correction for different generation technologies based on capital investment, average cost, marginal cost, capacity utilization, and other indicators, thus allowing power generators to obtain reasonable profits while reflecting the marginal generation and capacity value of different time periods.
In this paper, the marginal price is calculated under the assumption of a perfectly competitive market. In reality, there are contractual agreements, different power plant generation requirements, and even financial contracts that are not taken into account. Nuclear power is considered to be stable, whether loss or profit will be a huge amount. Since the cost of nuclear power is between advanced thermal units and backward thermal units, the marginal price is low for nuclear power losses when market demand is low (generate low marginal price) and high for nuclear power profits when demand is high (generate high marginal price). Therefore, the choice of entry of nuclear power plants into the market or the signing of contracts depends on the level of electricity tension and risk tolerance. On the other hand, the lack of shutdown options increases the flexibility of nuclear power peaking to a certain extent. With the development of technology, nuclear power will play an important role in peaking in the future, not only as a base load. The problem of “missing money” cannot be solved in the market, and new mechanisms, policies, and market designs are needed.
This paper constructs a power market pricing model that focuses on the start-up cost of generating units and new energy utilization, evaluates the sensitivity of coal prices, CO2 prices, and free CO2 quotas based on China, and predicts the electricity prices, wind and solar abandonment, and net income of different power sources under new energy expansion. The model is used as a policy analysis tool to inform tariff makers about marginal and average prices, profitability, and CO2 emission costs of generators in different scenarios, and to highlight the fact that the “missing money” problem of thermal units in liberalized markets will be exacerbated by the expansion of new energy sources.
In general, the marginal price in the electricity market is inadequate. The average cost varies smoothly according to the optimization objective, and the trend of marginal price is related to the average cost but with some volatility because the marginal price is directly related to the marginal unit. This is caused by the minimum load limits and start-up decisions of thermal units. The unstable marginal price and the stable average cost constitute the net income of the generators, which is not sufficient to cover the fixed costs, even if the net income is negative, resulting in a “missing money” problem. With the expansion of zero marginal cost intermittent renewable resources, marginal prices are constant or slightly lower (determined by demand) depending on the marginal unit, and thermal units will be taking away an increasing share of net income. Nuclear revenues face the same awkward situation of being dependent on demand-generating marginal units. Wholesale market design is therefore not appropriate to apply a uniform market price for different generation technologies, and new mechanisms, policies, and market designs are needed to establish and promote better long-term contracts.
The results of this study underscore several findings and policy advice. The marginal price sometimes deviates from the fluctuation of the real value; the fuel price and CO2 price basically can be transmitted downstream, but the new energy value must be transmitted through the impact on the marginal unit; it is difficult to control the excess profit or “missing money” problem, while the average cost change is more stable. In terms of the pricing mechanism, fixed cost and average cost can be considered as pricing benchmarks, and marginal prices are supplemented by capacity utilization, which can be used as a signal for demand response to adjust power pricing. In the spot market, it is recommended that different types of energy be traded separately, with different pricing benchmarks, so that the marginal units in the same market are homogeneous, and a better market design is needed to provide perfect long-term contract rules for power sources that are not suitable to enter the market.
The significance of this study lies in the understanding of the importance of accurate electricity price modeling in the context of China's power sector reforms, clean development, and carbon neutrality commitments. With the increasing penetration of renewable energy, the influence of fuel and carbon costs on pricing dynamics, and the need for efficient and incentive-compatible pricing mechanisms in the electricity market, conducting this research is both urgent and complex. By studying the price modeling of non-convex electricity markets, this research aims to contribute to the development of effective pricing policies, resource allocation efficiency, and the successful transition to a cleaner and more sustainable power system in China.
Although the research findings are based on the Chinese electricity market, their applications are universal. First, the optimization model considers the non-convexity and startup costs of the electricity market, which are challenges faced by all electricity markets. Second, the research reveals the issue of “missing money,” which stems from the “energy-only” characteristic of electricity markets, where only energy prices are considered while ignoring the fixed costs of electricity units. This problem exists in all electricity markets. Last, the research results demonstrate that to address the “missing money” issue, it is necessary to establish a capacity market to compensate for the fixed costs of backup capacity. This recommendation can also be applied to other economies to help them address their electricity market issues. Different electricity markets may have different installed capacity structures, but the model presented in this paper can still be applied by modifying the technical and economic parameters and conducting sensitivity analysis.
The disadvantage of this pricing model is that it does not consider the variation of demand with price, further iterative demand-price calculations can be considered in the future, along with modeling of renewable energy stochasticity to reflect the impact of new energy volatility on electricity prices. In addition, for the simulation in the long term (2050–2060), a combination of CCUS + thermal power can be considered. Finally, deviations of perfectly competitive markets from reality can also be considered and corrected, e.g., by studying the price markup in monopolistically competitive markets in conjunction with actual transactions.
Publicly available datasets were analyzed in this study. This data can be found here: https://openstd.samr.gov.cn/bzgk/gb/newGbInfo?hcno=08B766F14E3B138C8DD068DF41D95123; https://www.iea.org/reports/chinas-emissions-trading-scheme; https://www.mee.gov.cn/xxgk2018/xxgk/xxgk03/202012/W020201230736907121045.pdf?fbclid=IwAR0lMkdsACwXsYopAMaLazAIyyceiYmSndrjRh221-QgYzu7Z-uO8yUoDLE; https://www.iea.org/reports/the-role-of-chinas-ets-in-power-sector-decarbonisation; https://sc.macromicro.me/charts/41892/china-carbon-trading-market; https://meteonorm.com/; https://www.wind.com.cn/portal/zh/EDB/index.html.
PW, JC, and BL contributed to conception and design of the study and wrote sections of the manuscript. PW organized the database and performed the statistical analysis. JC wrote the first draft of the manuscript. All authors contributed to manuscript revision, read, and approved the submitted version.
The authors declare that the research was conducted in the absence of any commercial or financial relationships that could be construed as a potential conflict of interest.
All claims expressed in this article are solely those of the authors and do not necessarily represent those of their affiliated organizations, or those of the publisher, the editors and the reviewers. Any product that may be evaluated in this article, or claim that may be made by its manufacturer, is not guaranteed or endorsed by the publisher.
Cramton, P., Ockenfels, A., and Stoft, S. (2013). Capacity market fundamentals. Econ. Energy Environ. Policy 2, 27–46. doi: 10.5547/2160-5890.2.2.2
Cramton, P., and Stoft, S. (2005). A capacity market that makes sense. Electr. J. 18, 43–54. doi: 10.1016/j.tej.2005.07.003
Daraeepour, A., Larson, E. D., and Greig, C. (2022). Investigating price formation enhancements in non-convex electricity markets as renewable generation grows. Energy J. 43, 161–179. doi: 10.5547/01956574.43.5.adar
Dutta, G., and Mitra, K. (2017). A literature review on dynamic pricing of electricity. J. Operat. Res. Soc. 68, 1331–1445. doi: 10.1057/s41274-016-0149-4
IEA (2020a). China's Emissions Trading Scheme: Designing Efficient Allowance Allocation. Paris: International Energy Agency.
IEA (2020b). The Role of China's ETS in Power Sector Decarbonisation. Paris: International Energy Agency.
Joskow, P. L. (2019). Challenges for wholesale electricity markets with intermittent renewable generation at scale: the US experience. Oxford Rev. Econ. Policy 2, 2. doi: 10.1093/oxrep/grz001
Joskow, P. L., and Wolfram, C. D. (2012). Dynamic pricing of electricity. Am. Econ. Rev. 102, 381–385. doi: 10.1257/aer.102.3.381
Lin, B., Chen, J., and Wesseh, P. K. (2022). Peak-valley tariffs and solar prosumers: why renewable energy policies should target local electricity markets. Energy Pol. 165, 112984. doi: 10.1016/j.enpol.2022.112984
Parker, G. G., Tan, B., and Kazan, O. (2019). Electric power industry: operational and public policy challenges and opportunities. Prod. Operat. Manage. 28, 2738–2777 doi: 10.1111/poms.13068
Patnam, B. S. K., and Pindoriya, N. M. (2021). Demand response in consumer-centric electricity market: mathematical models and optimization problems. Electric Power Syst. Res. 193, 106923. doi: 10.1016/j.epsr.2020.106923
Reguant, M. (2014). Complementary bidding mechanisms and startup costs in electricity markets. Cesifo Working Paper 81, 1708–1742. doi: 10.1093/restud/rdu022
Richiardi, M. (2003). The Promises And Perils Of Agent-Based Computational Economics. Laboratorio R Revelli Working Papers.
van Ouwerkerk, J., Hainsch, K., Candas, S., Muschner, C., Buchholz, S., Günther, S., et al. (2022). Comparing open source power system models - a case study focusing on fundamental modeling parameters for the German energy transition. Renew. Sustain. Energy Rev. 161, 112331. doi: 10.1016/j.rser.2022.112331
Weigt, H. (2009). Modeling Competition and Investment in Liberalized Electricity Markets. Technische Universität Dresden.
Wesseh, P. K., Benjamin, N. I., and Lin, B. (2022). The coordination of pumped hydro storage, electric vehicles, and climate policy in imperfect electricity markets: insights from China. Renew. Sustain. Energy Rev. 160, 112275. doi: 10.1016/j.rser.2022.112275
Wesseh, P. K., and Dogah, K. E. (2022). Dynamic tariffs and climate policy interaction: an economic analysis of welfare implications in retail electricity markets. Energy Res. Soc. Sci. 90, 102679. doi: 10.1016/j.erss.2022.102679
Wesseh, P. K., and Lin, B. (2022). A time-of-use pricing model of the electricity market considering systemflexibility. Energy Rep. 8, 1457–1470. doi: 10.1016/j.egyr.2021.12.027
Keywords: electricity pricing, renewable energy sources, energy shortage, electricity markets, start-up costs
Citation: Wesseh PK Jr, Chen J and Lin B (2023) Electricity price modeling from the perspective of start-up costs: incorporating renewable resources in non-convex markets. Front. Sustain. Energy Policy 2:1204650. doi: 10.3389/fsuep.2023.1204650
Received: 12 April 2023; Accepted: 08 June 2023;
Published: 01 August 2023.
Edited by:
Syed Ahsan Ali Shah, University of Salamanca, SpainReviewed by:
Yasir Ahmed Solangi, Jiangsu University, ChinaCopyright © 2023 Wesseh, Chen and Lin. This is an open-access article distributed under the terms of the Creative Commons Attribution License (CC BY). The use, distribution or reproduction in other forums is permitted, provided the original author(s) and the copyright owner(s) are credited and that the original publication in this journal is cited, in accordance with accepted academic practice. No use, distribution or reproduction is permitted which does not comply with these terms.
*Correspondence: Boqiang Lin, YnFsaW5AeG11LmVkdS5jbg==
Disclaimer: All claims expressed in this article are solely those of the authors and do not necessarily represent those of their affiliated organizations, or those of the publisher, the editors and the reviewers. Any product that may be evaluated in this article or claim that may be made by its manufacturer is not guaranteed or endorsed by the publisher.
Research integrity at Frontiers
Learn more about the work of our research integrity team to safeguard the quality of each article we publish.