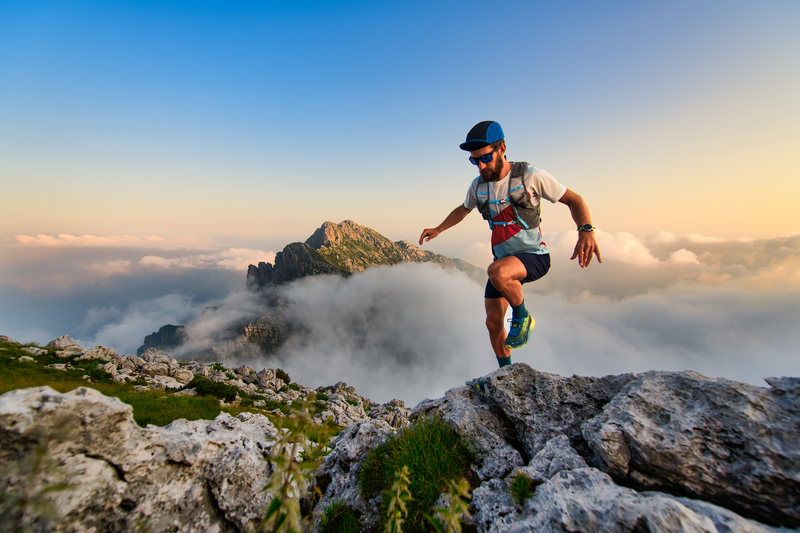
94% of researchers rate our articles as excellent or good
Learn more about the work of our research integrity team to safeguard the quality of each article we publish.
Find out more
SYSTEMATIC REVIEW article
Front. Sustain. Cities
Sec. Climate Change and Cities
Volume 7 - 2025 | doi: 10.3389/frsc.2025.1552994
The final, formatted version of the article will be published soon.
You have multiple emails registered with Frontiers:
Please enter your email address:
If you already have an account, please login
You don't have a Frontiers account ? You can register here
With increasing urbanization, the frequency of extreme weather events, and the intensification of the urban heat island (UHI) phenomenon, there is a growing concern about outdoor thermal comfort (OTC) in urban and rural spaces. However, previous OTC studies have been dominated by empirical case studies of regional sample points, and have lacked systematic large-scale exploration within a certain region. This study used the preferred reporting items for systematic reviews and meta-analyses (PRISMA) method and bibliometric tools to statisticians the sources, journals, disciplines, keywords, and highly cited papers of OTC studies. Based on the quantitative results, this study sorts and organizes OTC research from characterization, methods, and research trends, and summarizes the following results:1) Universal thermal climate index (UTCI) is relatively suitable for large-scale OTC research; 2) The combination of subjectivity and objectivity with the application of Artificial Intelligence (AI) is the current cutting-edge research method of OTC; 3) Local climate zone (LCZ) classification system has a potential to be used in future large-scale OTC research has application potential. Based on the collated results of previous studies, this study proposes a framework for large-scale OTC study to provide necessary theoretical support and practical guidance for future urban planning and construction, which will help optimize the urban environment and improve the thermal comfort and quality of life of residents.Keywords:Outdoor thermal comfort 1 , Large-scale 2 , Universal thermal climate index 3 , Local climate zone 4 , Systematic reviews 5 .
Keywords: Outdoor thermal comfort, large-scale, Universal thermal climate index, Local Climate Zone, Systematic reviews
Received: 19 Jan 2025; Accepted: 03 Mar 2025.
Copyright: © 2025 Li, Fei, Yang, Wang, Du and Wang. This is an open-access article distributed under the terms of the Creative Commons Attribution License (CC BY). The use, distribution or reproduction in other forums is permitted, provided the original author(s) or licensor are credited and that the original publication in this journal is cited, in accordance with accepted academic practice. No use, distribution or reproduction is permitted which does not comply with these terms.
* Correspondence:
Mengsheng Yang, Xiamen University, Xiamen, China
Disclaimer: All claims expressed in this article are solely those of the authors and do not necessarily represent those of their affiliated organizations, or those of the publisher, the editors and the reviewers. Any product that may be evaluated in this article or claim that may be made by its manufacturer is not guaranteed or endorsed by the publisher.
Research integrity at Frontiers
Learn more about the work of our research integrity team to safeguard the quality of each article we publish.