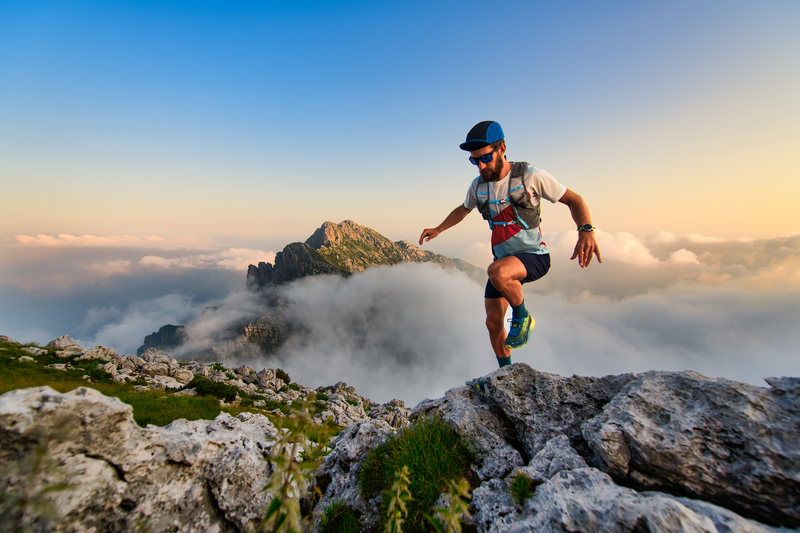
94% of researchers rate our articles as excellent or good
Learn more about the work of our research integrity team to safeguard the quality of each article we publish.
Find out more
ORIGINAL RESEARCH article
Front. Sustain. Cities
Sec. Cities in the Global South
Volume 7 - 2025 | doi: 10.3389/frsc.2025.1511761
The final, formatted version of the article will be published soon.
You have multiple emails registered with Frontiers:
Please enter your email address:
If you already have an account, please login
You don't have a Frontiers account ? You can register here
This study aims to investigate the influence of property value, property characteristics, cost of living, political stability, essential services, and environmental factors on residential property rental prices in Baidoa city. The research provides a comparative analysis of different modeling approaches to improve rental price forecasting. The study employs a dual-method approach, integrating hedonic regression analysis and artificial neural network (ANN) models to analyze rental values. The dataset includes key variables such as the number of bedrooms, essential services, cost of living, and environmental conditions. The predictive performance and interpretability of both models are compared to assess their effectiveness in rental price estimation. The findings indicate that rental prices are significantly influenced by the number of bedrooms, essential services such as electricity, cost of living, and environmental conditions. However, political stability and displacement were found not to have significant effects. While hedonic regression models provided clear and interpretable insights into direct predictors, ANN models demonstrated superior prediction accuracy by capturing nonlinear interactions. The ANN model's mixed performance, with 53% of cases underperforming and 47% exceeding predictions, highlights the need for improved precision in forecasting. The study underscores the importance of a mixed-method approach for rental price forecasting. Policymakers should integrate econometric and machine learning models to refine housing policies and ensure fair market regulations. Investors and property owners can utilize these findings to optimize rental pricing strategies. Additionally, real estate practitioners can benefit from data-driven decision-making, enhancing investment outcomes in emerging markets. This research contributes to the real estate valuation literature by bridging traditional econometric analysis with advanced machine learning techniques. The study validates the applicability of hedonic pricing and information asymmetry theories within an emerging market context. By incorporating economic and environmental factors into both models, the research provides a more comprehensive understanding of rental price determinants.
Keywords: Climate-Driven Real Estate, Hedonic regression, Artificial neural networks (ANN), Rental Price Forecasting, Displacement and Political Stability
Received: 15 Oct 2024; Accepted: 03 Mar 2025.
Copyright: © 2025 Nor, Audu and Mohamed. This is an open-access article distributed under the terms of the Creative Commons Attribution License (CC BY). The use, distribution or reproduction in other forums is permitted, provided the original author(s) or licensor are credited and that the original publication in this journal is cited, in accordance with accepted academic practice. No use, distribution or reproduction is permitted which does not comply with these terms.
* Correspondence:
Mohamed Ibrahim Ibrahim Nor, SIMAD University, Mogadishu, Somalia
Disclaimer: All claims expressed in this article are solely those of the authors and do not necessarily represent those of their affiliated organizations, or those of the publisher, the editors and the reviewers. Any product that may be evaluated in this article or claim that may be made by its manufacturer is not guaranteed or endorsed by the publisher.
Research integrity at Frontiers
Learn more about the work of our research integrity team to safeguard the quality of each article we publish.