- 1Urban Environment Observatory, School of Urban and Regional Planning, University of Alberta, Edmonton, AB, Canada
- 2Built Environment Lab, Department of Architecture and Planning, Indian Institute of Technology (IIT), Roorkee, India
There are thousands of pollution monitoring stations which are recording the data 24×7, the present research question is using this data to solve bring out a relationship between natural ventilation and air conditioning. Recently, WHO reported that 14 out of the top 15 most polluted cities are in India. Every year there is a loss of 6.2% to the global economy due to air pollution. The recent urban PM2.5 smog spread over the whole of north India covering about 50% of the country’s population. This event has been increasing the use of air purifiers and affecting the building energy performance. Most air purifiers (PM 10 and PM 2.5) are energy-intensive but are not always equipped with sensors. In commercial buildings, air purifiers are operated based on publicly relayed pollution information. The air pollutants that infiltrate into buildings are based on leaks, cracks, quality of building construction and pressure differences. Since indoor pollution levels are less than outdoor pollution levels, usage of air purifiers based on outdoor information leads to overperformance and hence energy wastage. Therefore, there is a need for optimization in sensitizing the performance of air purifiers at the building level. This study intends to assess the role of building airtightness and air purifier automation in lessening the air purifiers’ electricity consumption in urban areas. Transient building simulation tools do not account for infiltrated pollution levels directly. Virtually evaluating the energy savings through air purifier automation and the building’s airtightness would not be a straightforward assessment. The following paper uses EnergyPlus Energy Management System Class along with air pollution data monitored to model and simulate the Business-as-usual (BAU) and proposed Automation scenarios.
1 Introduction
There are pollution monitoring stations throughout the urban areas but the data from these stations are never used to monitor the HVAC (Heating, Ventilation, and Air Conditioning) usage practices. The air pollution is wide-spread, least addressed and yet one of the most endangering environmental health hazards of the world. The ambient and house-hold air pollution are observed to be the key environmental drivers for cardiovascular diseases, diarrheal diseases and lower respiratory infections (Neira and Prüss-Ustün, 2016; Barkjohn et al., 2020; Wang et al., 2023; Ma et al., 2022; Zhang et al., 2024). The cost of ambient air pollution on children’s health has been too high. WHO reported [World Health Organization (WHO), 2016] 1,69,250 deaths of children under five for the year 2012. In-addition to mortality, the neurodevelopment issues caused by air pollution in children has costing the developing countries up to 1 trillion USD every year (Attina and Trasande, 2013). According to 2019 World Air Quality Report by IQAir AirVisual, the 21 out of 30 world cities with worst air pollution are in India (Suri et al., 2023). It has been estimated that (Farrow et al., 2020; Budde et al., 2024) India experiences financial costs around 150 billion USD per year from fossil fuel air pollution alone. More than 70% of India’s electricity generation is through fossil fuels (NSDC, 2017; Production of Energy Resources, 2023). The Industry and Building sectors observed to be the first two primary stakeholders of incrementing energy consumption in India. Multiple policy initiatives have been taken to reduce the rising demand in both the sectors. Especially in Commercial building sector, energy conservation code (ECBC) (PNNL, 2018) have been launched by Ministry of Power, India to achieve energy efficiency by promoting high performance building envelope and efficient energy systems. However, the air pollution has been deteriorating the energy performance of the buildings. The rising pollutant levels in Indian cities has been forcing the citizens to procure the air purifiers for better health and well-being in Indoors. The air purifiers not only add-up to total energy consumption but also influence the heating, ventilating and air conditioning (HVAC) systems energy-use in buildings (Ummah, 2019; Bapat et al., 2012). At the times of outdoor pollution, occupants close the building fenestrations (windows and doors on facades) and switch on the HVAC and air purifiers. The latent heat load on the HVAC system becomes higher with almost no availability of fresh air. Thus, the energy use intensity becomes higher in buildings. Smart air purifiers available in the market could only reduce the operational period of air purifiers usage but can not contribute to the reduction of HVAC usage. It needs to be understood that the flow of air is not always from outside to inside. The indoor and outdoor pressure differences dictate the direction of flow. Opening the building fenestrations at the times with scope for exfiltration as well as predicted low pollutant infiltration could reduce the HVAC and Air purifiers operation period. The mathematical models predicting the air flow network are available and are being used for simulation studies. Novel systems determining the fenestrations, HVAC systems and air purifiers based on outdoor and indoor environmental conditions could be an efficient solution. The present-study illustrates the framework of such novel system conceptualized to sensitize performance of air purifiers for the buildings in urban core. The computational simulation-based approach has been chosen to demonstrate the performance of novel system, The similar system was proposed earlier using fuzzy intelligent computing model in estimating the overall health risk concerns using environmental monitoring data (Wang et al., 2024; Suri et al., 2023).
Air pollution and air conditioning go almost hand in glove. Where there is a high level of industrialization, as the world continues to be, so is air pollution in most towns and cities across the world. Air conditioning has been adopted and has become very common in homes, businesses, and other places as it gives comfort and relief from the hot weather. However, the relation between them could be complex and important to note knowing how they relate to each other.
1. Air pollution can have a huge effect on air conditioning systems. Outdoor air pollution can be drawn into the indoor spaces through the conditioning system, leading to poor quality of indoor air. This exposes occupants to a variety of health challenges, including respiratory problems, allergies, and asthma, among others. Besides, air pollution can damage the air conditioning system hence reducing its efficiency and increasing energy costs.
2. One of the ways in which air pollution can affect air conditioning systems is through the accumulation of particulate matter (Caciora et al., 2024). Particulate matter is a kind of air pollutant comprising minute particles that can be directly inhaled deep into the lungs (Budde et al., 2025). Once particulate matter gathers in the air conditioning system, it may block the filters, thereby become blocked and reduce the system’s performance. This can result in increased energy consumption and lower the system’s lifetime.
3. In addition to particulate matter, airborne pollution can also affect air conditioning systems through the accumulation of ozone. Ozone is a form of airborne pollutant which is created through a chemical reaction between nitrogen oxides and volatile organic compounds in the presence of sunlight. Ozone can also damage the rubber seals and plastic components of the air conditioning systems, which leads to leakages and losses in efficiency. It can also result in increased energy consumption and the life expectancy of the system.
Air pollution and air conditioning are closely related, and it is important to understand how they impact each other. Air pollution can strongly reduce efficiency and shorten the life of air conditioning equipment. While air conditioning equipment can increase air pollution through the emission of refrigerants. By reducing air pollution and properly maintaining air conditioning equipment, we can assure better indoor air quality (Sun et al., 2024), reduce energy costs, and protect both health and the environment, the generalization is done about findings from the various papers regarding the increase/decrease in indoor air quality due to the outdoor pollution. In all the three cases, i.e., Beijing (Cui et al., 2020), Central India (Hou et al., 2019; Matawle et al., 2017) and AIT Bangkok (Klinmalee et al., 2009), the results showed that whenever there is an increase in outdoor pollution, the indoor pollution is also increased.
The four factors—occupancy, PM2.5 and PM10 concentration, dry bulb temperature, and incident solar radiation as shown in Table 1 and Figure 1—can each independently influence the quality of in-building air and performance of HVAC systems. Occupancy in buildings may change the amount of pollutant discharge to the air, as human activity can create emissions like CO2, volatile organic compounds, and particulate matter. Result in higher levels of PM2.5 and PM10 concentrations, which are a measure of the amount of small and large airborne particles, respectively. Increasing occupancy in space can also raise the temperature and humidity, thereby affecting the performance of the HVAC system.
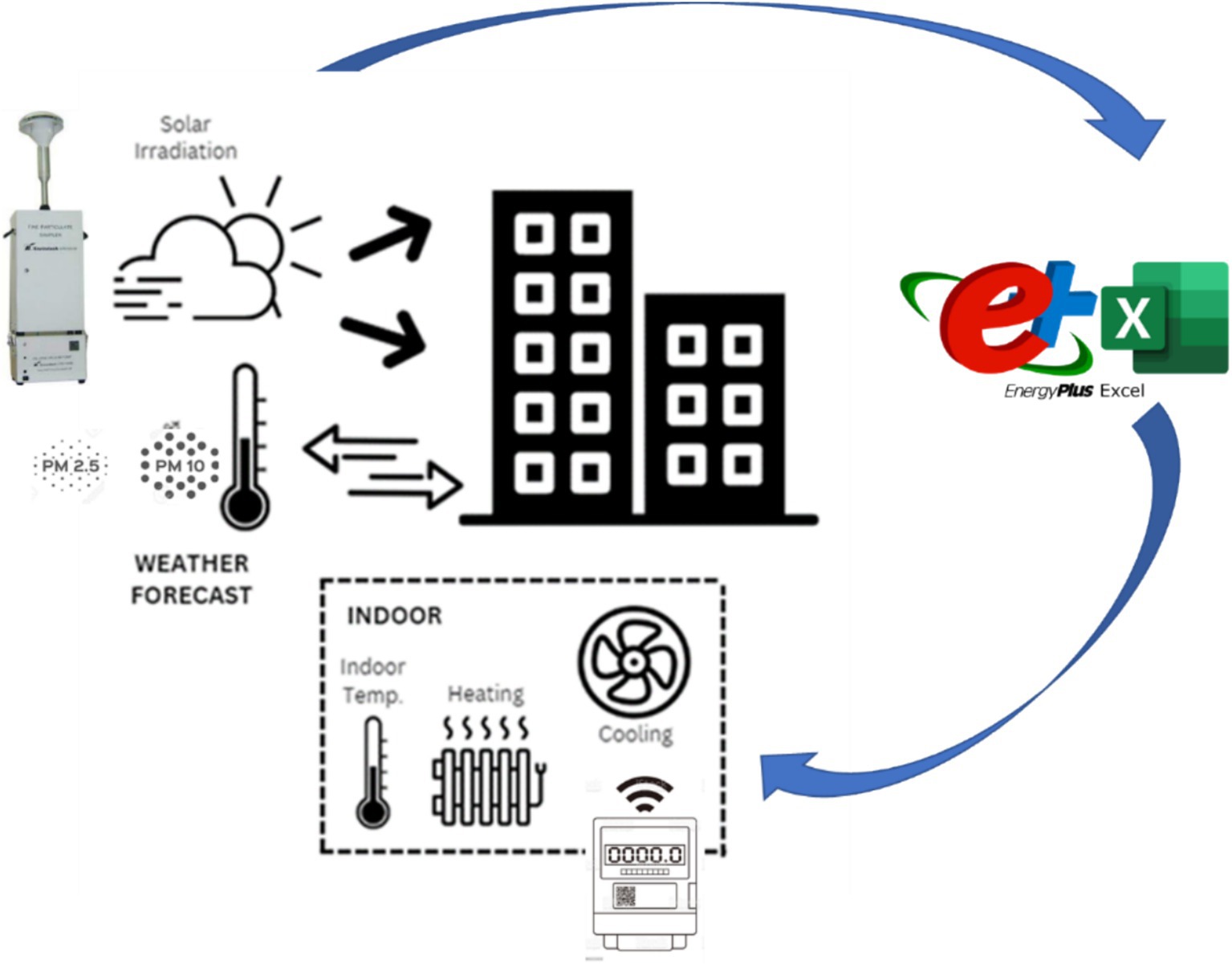
Figure 1. Pictorial problem statement explaining data from PM station outdoor, energy plus tool and indoor heating/cooling attached to the smart meter.
Factors that affect the levels of PM2.5 and PM10 concentrations differ from Occupancy levels and activities in the buildings, such as cooking or smoking, may increase these particulate matter concentrations, which adversely affect indoor air quality and the efficiency of HVAC systems by clogging filters and lowering system efficiency. High levels of particulate matter can also have health implications, especially for people with respiratory problems. Dry bulb temperature is a physical quantity defining the temperature of the air in a space, and it can be affected by a variety of reasons which will depend on a lot of factors, level of occupancy, the outdoor air temperature, performance of the HVAC systems. If the value for DBT is too high, it will impact IAQ and system performance since a comfortable temperature and humidity level cannot be easily maintained. A too-low DBT may result in poor system performance because equipment works harder than necessary.
Incident solar radiation refers to the radiation entering a building, which may be influenced by building orientation, window size and location, and other external shading devices. The ISR may further impact indoor air quality and HVAC system performance through raising both the temperature and humidity levels within space. In case ISR is excessive, it may give conditions that would make the indoor environment less comfortable to maintain; it can also increase workload on the HVAC system.
In summary, the factors likely to affect indoor air quality and HVAC system performance are occupancy level, PM2.5 and PM10, DBT, and ISR and can be used as per Table 1. These factors should be measured, and policies formulated that would such measures include increasing ventilation rates or installing air-cleaning technologies to mitigate adverse impacts on a healthy and comfortable indoor environment.
Air purifiers are innovative devices which help in improving the air quality with removal of contaminants and pollutants from the air. These are generally used indoors. They use advanced filtration technologies to purify the air from airborne particles. These particles generally include dust, pollen, microscopic bacteria, viruses and more. The air is let to pass through the devices, which contains multiple filters which clean the air, then the clean sir is released back into the space.
In the above mentioned, the primary filter used is a High-Efficiency Particulate Air (HEPA) filter, which has the highest efficiency to capture a particle of 0.3 microns. Some air purifies also include additional filters, such as activated carbon filters and more, these help in the removal of odors and absorb harmful gasses and chemicals.
These have many other benefits such as improving sleep quality, providing a sense of freshness in enclosed spaces. One thing to note, air purifiers are effective, but they are not a cure to all solution for poor indoor air quality. Other measures must be taken to optimal air quality indoors, as in the recent years, 80% of the time is being spend indoor. Other measures include regular cleaning, proper ventilation, minimizing the use of pollutants indoors and more.
These pollutants contribute to the formation of harmful smog and ground-level ozone. Which in turn causes severe health effects, mainly on the respiratory and cardiovascular system. Long term exposure has resulted many health problems, such as reduced lung function, cardiovascular problems and premature death (Barkjohn et al., 2020; Wang et al., 2023; Ma et al., 2022; Zhang et al., 2024). It also has detrimental effects on the environment, such as acid rain and more. Particulate matter and toxic pollutants can also settle on soil and water, contaminating them and affecting the overall ecological balance.
Urban planning strategies have been prioritizing sustainable transportation, pedestrian-friendly infrastructure and green spaces in term to reduce pollution levels. In fighting urban air pollution, there is a need to adopt a multi-faceted approach that captures government policies and technological advancement accompanied by individual responsibility. The future of Smart building guidelines provides a basic framework with respect to designing, constructing, and operating buildings with advanced technologies in a way that makes them efficient, sustainable, comfortable, and safe by optimizing energy use, reducing environmental impact, and improving the occupants’ experiences. One.
One of the key aspects would be energy efficiency. Another critical aspect dealt with in smart building guidelines is sustainability. They can support increased uses of renewable energy sources. It provides indoor air quality monitoring and filtration systems, natural lighting, acoustics.
Optimization and ergonomic design principles are also taken into consideration. Smart technologies can be used in creating customized environments that would adjust based on the users’ individual preferences through its automated temperature control, adjustable lighting, and smart shading systems. Smart building guidelines also consider safety and security considerations. These guidelines play an important role in fomenting sustainable development, reducing operational costs, improving.
It enhances the quality of life of occupants and helps in the vision of smart, connected cities. Co-simulation mode refers to a collaborative approach to engineering and simulation that performs the integration of two or more systems or domains into one simultaneous simulation. Co-simulation requires the coordination of at least two software tools or models that are experts in some domain, to be combined into a single simulation environment. It makes Co-simulation mode makes it easy to understand complex systems that involve interactions between elements of different natures or disciplines. One key advantage of the mode is its allowance for modeling and analyzing systems that have multiple physical domains or disciplines. Co-simulation mode further allows integration of different levels of details or fidelity in the description of a system. High-fidelity models can be made to use for critical components.
Either subsystems can be used or simplified/reduced-order models can be used for less critical parts or computationally intensive ones. Lastly, co-simulation mode is an enabling technique for complex systems analysis in that several domains or subsystems modeled and simulated in one process are possible. It enables system understanding of behavior at a holistic level. It provides integration of various resolutions and hence supports cooperation among experts from different domains. Engineers and researchers can use co-simulation modes to gain an overview.
It can be used to optimize system performance and drive informed decisions for applications that range from building and energy systems to transportation and manufacturing.
EnergyPlus: Developed by the U.S. Department of Energy, it is a very strong, widely applied building energy simulation tool. EnergyPlus is a vigorous, detailed, and comprehensive methodology applied to simulate different parameters of building energy consumption. Flexibility and extensibility are the two main benefits that are associated with the tool called EnergyPlus. EnergyPlus provides sophisticated simulation capabilities related to energy performance. Apart from that, EnergyPlus also integrates other tools or databases to extend its software coverage. It has the ability to import models from CAD software, get sources of weather data, and integrate with whole-building energy analysis programs.
EnergyPlus is the industry’s most used, full-featured, and flexible energy simulation software. It allows detailed modeling, simulation, and integration capabilities that become very useful in the analysis and improvement of building energy performance. With its capacity to support a wide array of building types, HVAC systems, and energy conservation measures, EnergyPlus has come out to be a key component in supporting the design and operation of energy-efficient and sustainable buildings.
2 Problem statement
The HVAC systems comprise a prefilter that obscure pet hair, dust and large size microbes to flow into the main filter. The main filter could be HEPA or HEPA-like or HEPA-type filters to obscure bacteria, car emissions, spores and mold before air reaching evaporator for cooling. The HEPA grade filters are capable of handling PM2.5 and PM10 pollutant concentrations but only in rural environment (Shi et al., 2020). Dedicated air filters are necessary for built zones in urban environment. The recently innovated smart air filters are operated based on the pollutant concentration monitored by PM2.5 and PM10 sensors integrated with air filters. The PM2.5 and PM10 monitoring devices inside the air filter may not detect the concentration of pollutants all the time. The smart air filters house the sensors in the separate compartment that is cleaner than the rest of the system (Suri et al., 2023). The integrated sensor could not read the pollutant concentration until it reaches the room where PM2.5 and PM 10 sensors are hosted. The performance study (Junaid et al., 2018) conducted on air filters has deduced that the smart air filters should not be run on auto mode, i.e., operation based on PM2.5 and PM10 sensors. The problem statement is explained in Figure 1.
3 Case area selection
Vikas Minar (DDA HQ) (Figure 2), that falls in the urban core of Delhi has been chosen as a sample for this pilot study with the floor plan details as shown in Figure 3. The reconnaissance survey proves that the air conditioners are run without consideration of the indoor PM2.5 level. The Business-as-usual scenario operates the air purifier based on the outdoor air pollution information. Erroneous operation of air purifiers in unoccupied and unrequired hours have not to be included. In the Automation scenario, the air purifiers operations are based on indoor air pollution. The preliminary analysis has shown that energy saving up to 25% could be possible by operating air purifiers based on the indoor air pollution level. In addition to energy savings, the operational expenditure could be reduced by lessening the hours of operation by automation scenarios (Lat and long) (Climate details).
4 Framework and methodology
The simulation-based study has been performed on an office building located in New Delhi. Two cases have been synthesized detailing the differences of operation in fenestrations, air filtering systems, Heating Ventilating and Air Conditioning (HVAC) systems for business-as-usual scenario (basecase) and for novel system scenario (smart case). In the basecase, the air filter operates when the occupants exist and outdoor PM2.5 and PM10 pollutants concentrations are greater than 100 ppm and 60 ppm, respectively, (Figure 4A). The windows and air conditioners (ACs) of base case are operated based on
1. Occupancy
2. PM2.5 and PM 10 concentration (3 years hourly data)
3. Dry bub temperature (DBT)
4. Incident Solar Radiation (ISR)
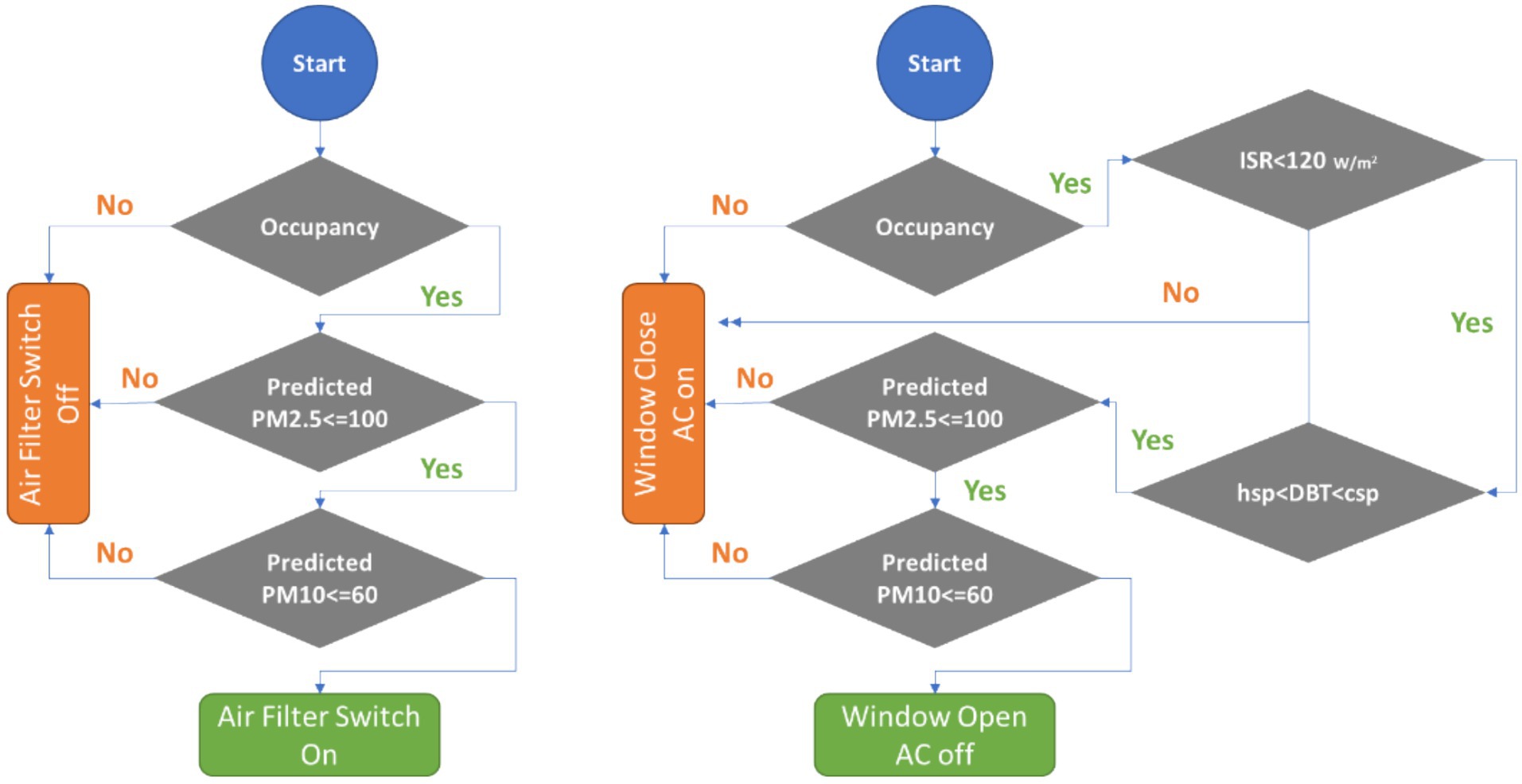
Figure 4. Smart case windows, ACs and air filters operation logic. (A) Air filter operation logic. (B) Window operation logic.
The occupancy and pollutant concentration conditions for windows opening are same as air filters operation conditions. The ISR less than 120 W/m2 and DBT between the cooling setpoint (csp) (Equation 2) and heating setpoint (hsp) (Equation 3) are required in the basecase to open the windows in addition to occupant and pollution concentration conditions as shown in Figure 4B. The csp (cooling set point) and hsp (heating set point) have been computed from Indian Model for Adaptive thermal Comfort (IMAC) mixed-mode building equation (Equation 1) provided in Energy Conservation Building Code 2017 (PNNL, 2018).
In the smart case, the air filter has been operated based on the predicted indoor PM2.5 and PM10 limits (Figure 4). The pollutant infiltration to the zone per individual window has been computed based on the indoor and outdoor pressure differences. In the real world, the smart system being proposed scraps weather forecasts from open-source websites. The indoor weather monitored by sensing devices in smart system predicts the pressure difference using validated mathematical models of computational fluid dynamics. The volume mass flow rate of air for provided fenestrations in the zone would also be accounted by the smart system to operate the windows. Other than pollutant concentrations, the occupancy, DBT and ISR have also been considered similar to basecase. Figure 4B shows the operation logic of window and ACs for smart case. The base case and smart case operational logics have been scripted in EnergyPlus (EP) Energy Management System (EMS) class through EnergyPlus Runtime Language (Erl). The EP is an open-source tool (Invidiata and Ghisi, 2016) that simulates the air flow network, thermal performance and energy performance for the virtually modeled building. The EnergyPlus Weather File (EPW) file of Delhi has been used as representative weather dataset for simulation. The PM2.5 and PM10 hourly data for 3 years has been extracted from Central Pollution Control Board of India data repository (CPCB, 2016; Mishra et al., 2021) and averaged out to create typical pollution dataset for Delhi. The weather and pollution data has been used to simulate and predict pollutants infiltration per window.
The Figure 4 shows cases the virtual modeling of smart system operating windows as well as air filter modeling in EMS of EP. The state-of-art air filter’s electric power density (EPD) i.e., air filter wattage per unit area covered was discovered and wattage for representative floor plate has been calculated. The indoor pollutants concentration (conPM25 & conPM10) has been predicted by multiplying volume flow rate of air per window with outdoor pollutant concentration. The airflow network (AFN) class in EP accounts the volumetric flow rate of air through windows. The occupants generally assume that the direction of air flow is always from outside to inside. However, the direction of flow depends on pressure difference between outdoors and indoors. The regular window opening can reduce the sensible as well as latent load on cooling and heating systems (ACs). Figures 4A,B shows that the AC is switched on when windows are closed. But cooling and heating process in ACs is initiated when zone air temperature is above csp and below hsp, respectively. Therefore, the AC on/off ion this study represents operational availability of AC.
5 Analysis and discussions
The smart case and base case scanarios are human centric as the logic depends on the occupancy. When the data from the nearest pollution monitoring station is missing the logic can be run based on the other nearest station in automated form by following euclidean distance principle. The potentiality of smart system in this study has been accounted by comparing the energy performance of building in both cases. Firstly, the total energy consumption of building in both the cases has been compared. The air filters and ACs energy consumption for both cases have been compared later. As shown in Figure 5A, the total energy consumption in smart case is reduced by 64% over base case. The major contribution for reduction in total energy consumption is from reduced ACs energy consumption followed by reduced air filters energy consumption in smart case. The ACs energy consumption in smart case is about 75% less than that of the base case. The air filters energy consumption in smart case observed to be 32% less than the base case. The ACs energy savings in smart case seemed to be higher than air filters. Thus, the heat addition and removal from windows in base case and smart case has been compared. The quantity of heat addition per square meter area of modeled building has reduced from 78 kWh/m2/year in base case to 71 kWh/m2/year in smart case as shown in Figure 5B. Additionally, heat removal in smart case increased to 27 kWh/m2/year heat from 17 kWh/m2/year in base case. Although the change seems to be small in terms of energy, the load on ACs is large. In less ventilated building spaces, the latent load from occupants increases gradually. The ACs require additional enthalpy to address the latent load over a sensible load. The air changes due to natural ventilation displaces the warm and humid air in the zone with windows open/ close scheduling as per Figure 6. In addition to energy benefit, the air filter life can be increased by lowering the usage of air purifiers. In the studies conducted earlier highlighted the use of decision-making algorithms such as fuzzy logic, generic algorithms (Bardhan et al., 2018; Sarkar and Bardhan, 2020) and other relevant machine learning models will be helpful to guide the policymakers and relate it with energy studies for further design. The study is limited to one station data and occupancy data is considered as general office timings (9 am-5 pm), With the future improvement in IoT, There could be real time occupancy data and modeling which could be developed in understanding the space usage patterns for better energy usage prediction.
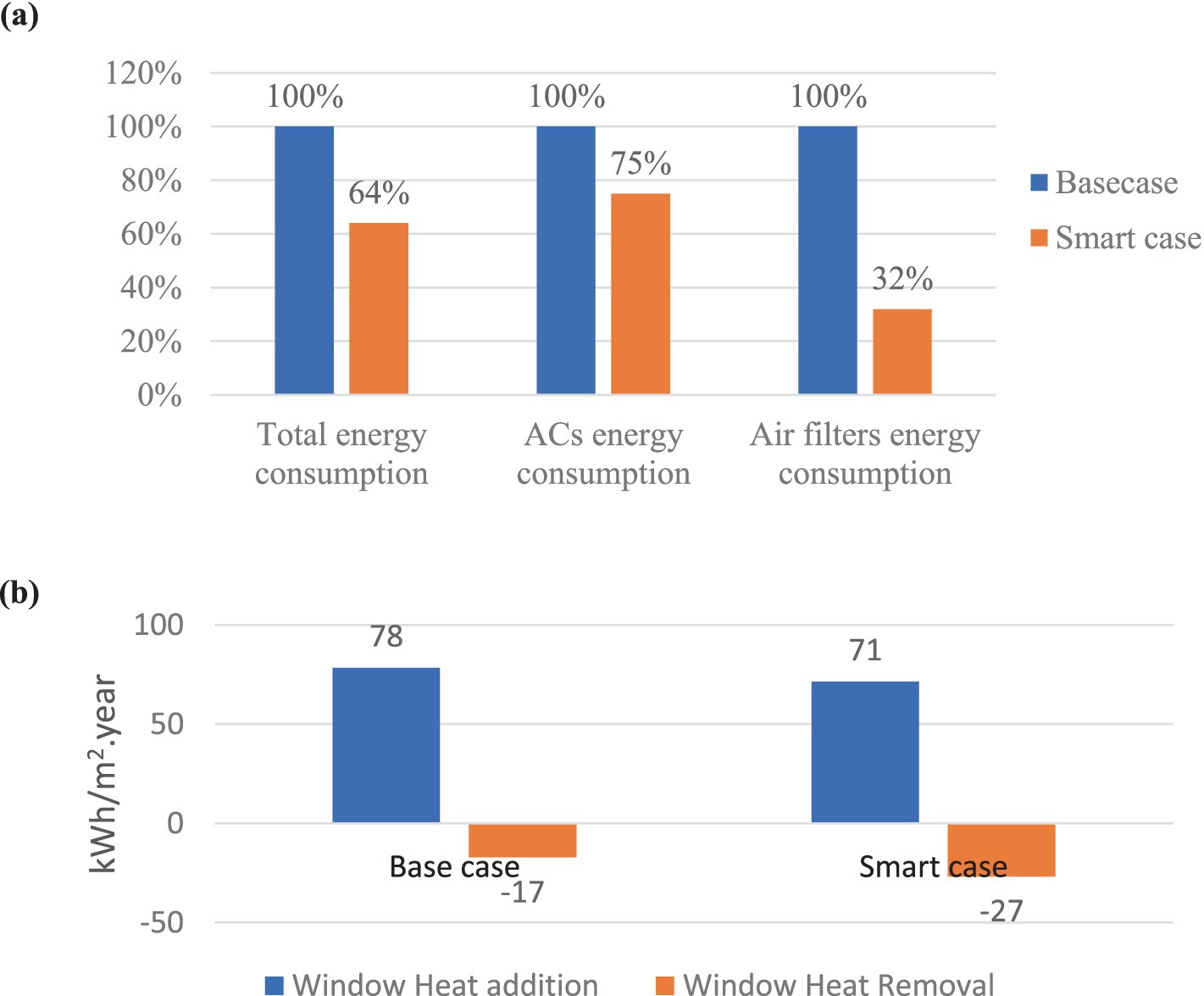
Figure 5. (A) Comparison of energy performance between base case and smart case. (B) Comparison of heat transfer through windows in base case and smart case.
6 Conclusion
The following study uses real time data from pollution monitoring stations which makes it easy for study application in across south Asia and can be used in general not only for commercial buildings but for also residential and mixed use buildings in the urban areas, Building energy consumption is the main subject of interest for the carbon foot print reduction, chief utilizing potentials of the air purifiers though the 30 W-60 W air purifier is not energy intensive but when coupled with air conditioners there would be better energy savings in the following base case and smart case scanarios. In the majority of offices, the air purifiers are locally made and do not comprise any automation capability. Additionally, the air purifiers are operated based on pollution information available from public broadcasters. Occupants tend to initiate the air purifiers in the morning once they enter their office spaces and shutdown the fenestration completely. Though the external conditions are favorable, occupants do not tend the operation mode with which there is a rise in cooling energy demand. Today, transient simulation models are being allied with real time building models to pitch dynamic solutions for energy and IAQ conservation based on predictions. Although CONTAM tool (Fellini et al., 2019; Andra et al., 2015; Thouron et al., 2017; Mendoza et al., 2021; Daly et al., 2012) was available to couple with energy plus, it is only available for older versions. Therefore, Airflow Network class in Energy Plus was used to predict the concentration would be infused if the window is opened. Based on the number of windows, the limit of pollution at which the window should be opened was set. Although in our preliminary analysis, the savings showed up to 25%. After the revision of logic, we observe savings in cooling energy by 75% which is large. In smart case, reduction in heat addition and increase in heat removal aided the Ideal HVAC system assumed to reduce the load. Secondly, we assume that reduction in latent load may also be the additional cause. The necessity-based approach on electricity consumption needs to be understood from the user side. There is 75% reduction in operational costs observed in smart case scenario over base case scenario.
The logic framework of scenarios had been coded inside EnergyPlus through EnergyPlus Runtime language. The novel predictive based smart systems have shown better energy efficiency than conventional system in simulation-based analysis. The initiation of novel system development and real-time performance testing is on-going. The logic assembly can be used for different built typologies for buildings within different local climate zoning (Han et al., 2022; Abougendia et al., 2020; Zheng et al., 2018), India is going to have more than 2,500 pollution monitoring stations by 2030 and if the local station generated data if used in IoT will help in creating smart air purifiers and a real time human occupancy based energy usage.
Data availability statement
The original contributions presented in the study are included in the article/Supplementary material, further inquiries can be directed to the corresponding author.
Author contributions
SB: Conceptualization, Data curation, Formal analysis, Investigation, Methodology, Software, Writing – original draft, Writing – review & editing, Visualization. PC: Conceptualization, Funding acquisition, Investigation, Methodology, Project administration, Resources, Supervision, Validation, Writing – original draft, Writing – review & editing. SA: Funding acquisition, Project administration, Resources, Supervision, Validation, Writing – original draft, Writing – review & editing.
Funding
The author(s) declare financial support was received for the research, authorship, and/or publication of this article. The study received grant from Ministry of Human Resources Development, File no:18902022, 2018.
Conflict of interest
The authors declare that the research was conducted in the absence of any commercial or financial relationships that could be construed as a potential conflict of interest.
Publisher’s note
All claims expressed in this article are solely those of the authors and do not necessarily represent those of their affiliated organizations, or those of the publisher, the editors and the reviewers. Any product that may be evaluated in this article, or claim that may be made by its manufacturer, is not guaranteed or endorsed by the publisher.
Supplementary material
The Supplementary material for this article can be found online at: https://www.frontiersin.org/articles/10.3389/frsc.2024.1469803/full#supplementary-material
Abbreviations
PM, Particulate Matter; IAQ, Indoor Air Quality; HVAC, Heating, Ventilation, and Air Conditioning; ECBC, Energy Conservation Building Code; DBT, Dry Bulb Temperature; ISR, Incident Solar Radiation; HEPA, High-Efficiency Particulate Air; TVOC, Total volatile organic compounds; Csp, Cooling Setpoint; Hsp, Heating Setpoint; IMAC, Indian Model for Adaptive thermal Comfort; EPW, EnergyPlus Weather File; EP, EnergyPlus; EMS, Energy Management System; BAU, Business-as-usual; AFN, The airflow network; EPD, Electric power density; Erl, EnergyPlus Runtime Language; IoT, Internet of Things.
References
Abe, C. F., Dias, J. B., Notton, G., and Faggianelli, G. A. (2020). Experimental application of methods to compute solar irradiance and cell temperature of photovoltaic modules. Sensors 20:2490. doi: 10.3390/s20092490
Abougendia, S. M., Ayad, H. M., and El-Sayad, Z. T. (2020). Classification framework of local climate zones using world urban database and access portal tools: case study of Alexandria city, Egypt. WIT Trans. Ecol. Environ. 241, 309–322. doi: 10.2495/SDP200251
Andra, S. S., Charisiadis, P., Arora, M., van Vliet-Ostaptchouk, J. V., and Makris, K. C. (2015). Biomonitoring of human exposures to chlorinated derivatives and structural analogs of bisphenol a. Environ. Int. 85, 352–379. doi: 10.1016/j.envint.2015.09.011
Attina, T. M., and Trasande, L. (2013). Economic costs of childhood lead exposure in low- and middle-income countries. Environ. Health Perspect. 121, 1097–1102. doi: 10.1289/ehp.1206424
Bapat, S., Chaturvedi, A., Drewery, W., Fei, F., and Hepfer, E. (2012). Microeconomics of competitiveness Tamil Nadu automotive cluster Harvard Business School – Spring 2012 section. Harvard Business School.
Bardhan, R., Debnath, R., Malik, J., and Sarkar, A. (2018). Low-income housing layouts under socio-architectural complexities: a parametric study for sustainable slum rehabilitation. Sustain. Cities Soc. 41, 126–138. doi: 10.1016/j.scs.2018.04.038
Barkjohn, K. K., Norris, C., Cui, X., Fang, L., He, L., Schauer, J. J., et al. (2020). Children’s microenvironmental exposure to PM2.5 and ozone and the impact of indoor air filtration. Epidemiology 30, 971–980. doi: 10.1038/s41370-020-00266-5
Budde, S., Agrawal, S., and Chani, P. S. (2024). Utilising GIS for studying urban entropy, population dynamics, and ventilation disparity: a case study of changing land use, land cover, and socially vulnerable hotspots in Hyderabad, India. Phys. Chem. Earth 136:103748. doi: 10.1016/j.pce.2024.103748
Budde, S., Chani, P. S., and Agrawal, S. (2025). Framework for Evaluating and Mitigating Industrial Air Pollution in India: Systematic Review of Concepts and Unmet Needs. J. Hazard. Toxic Radio. Waste 29, 1–12. doi: 10.1061/JHTRBP.HZENG-1356
Caciora, T., Ilieş, A., Berdenov, Z., al-Hyari, H. S.'., Ilieş, D. C., Safarov, B., et al. (2024). Comprehensive analysis of classroom microclimate in context to health-related national and international indoor air quality standards. Front. Public Health 12. doi: 10.3389/fpubh.2024.1440376
CPCB. (2016). Action plan for non attainment cities /area(AirPollution) of Telangana state. Central Pollution Control Board.
Cui, L., Wu, Z., Han, P., Taira, Y., Wang, H., Meng, Q., et al. (2020). Chemical content and source apportionment of 36 heavy metal analysis and health risk assessment in aerosol of Beijing. Environ. Sci. Pollut. Res. 27, 7005–7014. doi: 10.1007/s11356-019-06427-w
Daly, A., Zannetti, P., and Echekki, T. (2012). A combination of fire and dispersion modeling techniques for simulating a warehouse fire. Int. J. Safety Secur. Eng. 2, 368–380. doi: 10.2495/SAFE-V2-N4-368-380
Doukas, H., Patlitzianas, K. D., Iatropoulos, K., and Psarras, J. (2007). Intelligent building energy management system using rule sets. Build Environ, 42, 3562–3569. doi: 10.1016/j.buildenv.2006.10.024
Farrow, A., Miller, K. A., and Myllyvirta, L. (2020). Toxic air: the Price of fossil fuels. Greenpeace Southeast Asia, 1–44.
Fellini, S., Salizzoni, P., Soulhac, L., and Ridolfi, L. (2019). Propagation of toxic substances in the urban atmosphere: a complex network perspective. Atmos. Environ. 198, 291–301. doi: 10.1016/j.atmosenv.2018.10.062
Han, B., Luo, Z., Liu, Y., Zhang, T., and Yang, L. (2022). Using local climate zones to investigate Spatio-temporal evolution of thermal environment at the urban regional level: a case study in Xi'an, China. Sustain. Cities Soc. 76:103495. doi: 10.1016/j.scs.2021.103495
Hou, S., Zheng, N., Tang, L., Ji, X., Li, Y., and Hua, X. (2019). Pollution characteristics, sources, and health risk assessment of human exposure to cu, Zn, cd and Pb pollution in urban street dust across China between 2009 and 2018. Environ. Int. 128, 430–437. doi: 10.1016/j.envint.2019.04.046
Invidiata, A., and Ghisi, E. (2016). Impact of climate change on heating and cooling energy demand in houses in Brazil. Energ. Buildings 130, 20–32. doi: 10.1016/j.enbuild.2016.07.067
Junaid, M., Syed, J. H., Abbasi, N. A., Hashmi, M. Z., Malik, R. N., and Pei, D. S. (2018). Status of indoor air pollution (IAP) through particulate matter (PM) emissions and associated health concerns in South Asia. Chemosphere 191, 651–663. doi: 10.1016/j.chemosphere.2017.10.097
Klinmalee, A., Srimongkol, K., and Kim Oanh, N. T. (2009). Indoor air pollution levels in public buildings in Thailand and exposure assessment. Environ. Monit. Assess. 156, 581–594. doi: 10.1007/s10661-008-0507-z
Kolokotsa, D., Pouliezos, A., and Stavrakakis, G., and Lazos, C. (2009). Predictive control techniques for energy and indoor environmental quality management in buildings. Build Environ, 44, 1850–1863. doi: 10.1016/j.buildenv.2008.12.007
Kumar, R., Nagar, J. K., Goel, N., Kumar, P., Kushwah, A. S., and Gaur, S. N. (2015). Indoor air quality and energy management through real-time sensing in commercial buildings. Energy Build. 111, 145–153. doi: 10.1016/j.enbuild.2015.11.037
Ma, Z., Meng, X., Chen, C., Chao, B., Zhang, C., and Li, W. (2022). Short-term effects of different PM2.5 ranges on daily all-cause mortality in Jinan, China. Sci. Rep. 12, 5665–5668. doi: 10.1038/s41598-022-09057-4
Martani, C., Lee, D., Robinson, P., Britter, R., and Ratti, C. (2012). ENERNET: Studying the dynamic relationship between building occupancy and energy consumption. Energy Build, 47, 584–591. doi: 10.1016/j.enbuild.2011.12.037
Matawle, J. L., Pervez, S., Shrivastava, A., Tiwari, S., Pant, P., Deb, M. K., et al. (2017). PM2.5 pollution from household solid fuel burning practices in Central India: 1. Impact on indoor air quality and associated health risks. Environ. Geochem. Health 39, 1045–1058. doi: 10.1007/s10653-016-9871-8
Mendoza, D. L., Benney, T. M., and Boll, S. (2021). Long-term analysis of the relationships between indoor and outdoor fine particulate pollution: a case study using research grade sensors. Sci. Total Environ. 776:145778. doi: 10.1016/j.scitotenv.2021.145778
Milenkovic, M., and Amft, O. (2013). Recognizing energy-related activities using sensors commonly installed in office buildings. Procedia Computer Science, 19(Seit), 669–677. doi: 10.1016/j.procs.2013.06.089
Mineno, H., Kato, Y., Obata, K., Kuriyama, H., Abe, K., Ishikawa, N., et al. (2010, January). Adaptive home/building energy management system using heterogeneous sensor/actuator networks. In 2010 7th IEEE Consumer Communications and Networking Conference (pp. 1–5). IEEE. doi: 10.1109/CCNC.2010.5421762
Missaoui, R., Joumaa, H., Ploix, S., and Bacha, S. (2014). Managing energy smart homes according to energy prices: analysis of a building energy management system. Energy Build. 71, 155–167. doi: 10.1016/j.enbuild.2013.12.018
Mishra, R. K., Agarwal, A., and Shukla, A. (2021). Predicting ground level PM2.5Concentration over Delhi using Landsat 8 satellite data. Int. J. Remote Sens. 42, 827–838. doi: 10.1080/2150704X.2020.1832279
Neira, M., and Prüss-Ustün, A. (2016). Preventing disease through healthy environments: a global assessment of the environmental burden of disease. Toxicol. Lett. 259:S1. doi: 10.1016/j.toxlet.2016.07.028
NSDC. (2017). Human resource and skill requirements in the construction material and building hardware sector (p. 67). Ministry of Skill Development India.
PNNL (2018). Energy conservation building directive – 2018 (based on ECBC 2017), vol. 2018. Alliance for Energy Efficient Economy. [Epub ahead of print].
Polo, J., Fernandez-Neira, W. G., and Alonso-García, M. C. (2017). On the use of reference modules as irradiance sensor for monitoring and modelling rooftop PV systems. Renew. Energy 106, 186–191. doi: 10.1016/j.renene.2017.01.026
Remund, J., Müller, S. C., Schilter, C., and Rihm, B. (2011). The use of Meteonorm weather generator for climate change studies. EMS Annual Meet. Abs. 7:46625.
Rhiat, M., Karrouchi, M., Hassari, A., Melhaoui, M., Atmane, I., Azzaoui, H., et al. (2023). Design and simulation of a low-cost solar irradiance meter for PV applications. E3S Web Conf. 469, 3–8. doi: 10.1051/e3sconf/202346900076
Sarkar, A., and Bardhan, R. (2020). Improved indoor environment through optimised ventilator and furniture positioning: a case of slum rehabilitation housing, Mumbai, India. Front. Arch. Res. 9, 350–369. doi: 10.1016/j.foar.2019.12.001
Shi, G., Lu, X., Lu, X., Lu, X., Deng, Y., Urpelainen, J., et al. (2020). Air pollutant emissions induced by population migration in China. Environ. Sci. Technol. 54, 6308–6318. doi: 10.1021/acs.est.0c00726
Snyder, E. G., Watkins, T. H., Solomon, P. A., Thoma, E. D., Williams, R. W., Hagler, G. S., et al. (2013). The changing paradigm of air pollution monitoring. Environ Sci Technol, 47, 11369–11377. doi: 10.1021/es4022602
Sun, D., Tang, L., Long, K., Sun, W., and Zhang, Z. (2024). Bacterial contamination in the different parts of household air conditioners: a comprehensive evaluation from Chengdu, Southwest China. Front. Public Health 12. doi: 10.3389/fpubh.2024.1429626
Suri, R. S., Jain, A. K., Kapoor, N. R., Kumar, A., Arora, H. C., Kumar, K., et al. (2023). Air quality prediction-a study using neural network based approach. J. Soft Comp. Civil Eng. 7, 93–113. doi: 10.22115/SCCE.2022.352017.1488
Thouron, L., Seigneur, C., Kim, Y., Legorgeu, C., Roustan, Y., and Bruge, B. (2017). Simulation of trace metals and PAH atmospheric pollution over greater Paris: concentrations and deposition on urban surfaces. Atmos. Environ. 167, 360–376. doi: 10.1016/j.atmosenv.2017.08.027
Wang, W., Guan, X., Peng, X., Wang, Z., Liang, X., and Zhu, J. (2024). Urban environmental monitoring and health risk assessment introducing a fuzzy intelligent computing model. Front. Public Health 12, 1–11. doi: 10.3389/fpubh.2024.1357715
Wang, P., Liu, D., Mukherjee, A., Agrawal, M., Zhang, H., Agathokleous, E., et al. (2023). Air pollution governance in China and India: comparison and implications. Environ Sci Policy 142, 112–120. doi: 10.1016/j.envsci.2023.02.006
World Health Organization (WHO). (2016). Health as the pulse of the new urban agenda: United Nations conference on housing and sustainable urban development. United Nations Conference on Housing and Sustainable Urban Development Quito – 2016, 56.
Zhang, J., Zhang, J., Duan, Z., Nie, J., Li, X., Yu, W., et al. (2024). Association between long-term exposure to PM 2. 5 chemical components and metabolic syndrome in middle-aged and older adults. Front. Public Health. 12. doi: 10.3389/fpubh.2024.1462548
Zhang, X., Yan, J., Feng, S., Lei, Z., Yi, D., and Li, S. Z. (2012, September). Water filling: Unsupervised people counting via vertical kinect sensor. In 2012 IEEE ninth international conference on advanced video and signal-based surveillance (pp. 215–220). IEEE. doi: 10.1109/AVSS.2012.82
Keywords: air purifiers, urban air pollution, smart building guidelines, co-simulation mode, energy plus
Citation: Budde S, Chani PS and Agrawal S (2025) Sensitizing performance of air purifiers for the high-rise commercial buildings in urban core. Front. Sustain. Cities. 6:1469803. doi: 10.3389/frsc.2024.1469803
Edited by:
Nishant Raj Kapoor, Academy of Scientific and Innovative Research (AcSIR), IndiaReviewed by:
Hashem Jahangir, University of Birjand, IranSarkawt Hama, University of Sulaymaniyah, Iraq
Anant Mishra, Birla Institute of Technology and Science, India
Copyright © 2025 Budde, Chani and Agrawal. This is an open-access article distributed under the terms of the Creative Commons Attribution License (CC BY). The use, distribution or reproduction in other forums is permitted, provided the original author(s) and the copyright owner(s) are credited and that the original publication in this journal is cited, in accordance with accepted academic practice. No use, distribution or reproduction is permitted which does not comply with these terms.
*Correspondence: Sandeep Budde, c2J1ZGRlQHVhbGJlcnRhLmNh